The Invisible Backbone of AI Efficiency
Why Industrial AI Struggles Without Top-Notch Visual Data
Last updated:

Edited By
Mackenzie Ferguson
AI Tools Researcher & Implementation Consultant
Discover why quality visual data is not just a preference but a necessity for industrial AI success. From safety issues to eroded trust, learn about the perils of poor data and the steps industries are taking to rectify it.
Introduction to the Importance of Visual Data in Industrial AI
In the rapidly-evolving landscape of Industrial AI, the quality of visual data stands as a cornerstone for the sector's success. From enhancing operational efficiency to ensuring system safety, high-quality visual data plays a pivotal role. As outlined in the compelling analysis by RTInsights, the inadequacy in visual data quality can severely undermine the efficacy of AI models. This deficiency can lead to disruptive phenomena like model drift, erroneous predictions, and even critical safety lapses (source). The pursuit of excellence in visual data quality involves intricate measures ranging from upgrading hardware and software to adopting edge intelligence techniques. These improvements are not just optional add-ons but necessary steps to fully harness the potential of Industrial AI systems.
Underpinning the demand for superior visual data is the necessity for real-time data processing to meet the dynamic needs of industrial operations. Real-time visual data processing enables timely responses to ongoing processes and anomalies, a capability underscored by solutions such as Volt Active Data's platform. This platform is exemplary in its ability to offer real-time ingestion, processing, and response, significantly enhancing the integration with computer vision pipelines and empowering industries with the means for contextual decision-making (source). Moreover, the sophistication of Industrial AI is intimately linked with the quality of visual data it processes. Sophisticated AI models, no matter how advanced, are only as good as the data fed into them. As Salvatore Salamone from RTInsights points out, challenges such as poor lighting and camera misalignments can squander resources and pose safety risks. Thus, implementing a robust framework for ensuring high-quality visual data is indispensable for industrial sectors aiming to thrive in the AI-driven future (source).
Learn to use AI like a Pro
Get the latest AI workflows to boost your productivity and business performance, delivered weekly by expert consultants. Enjoy step-by-step guides, weekly Q&A sessions, and full access to our AI workflow archive.
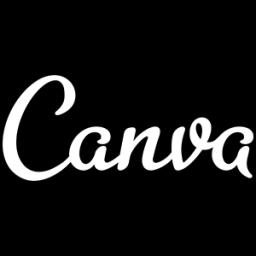
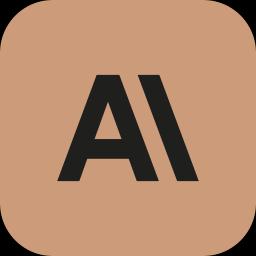
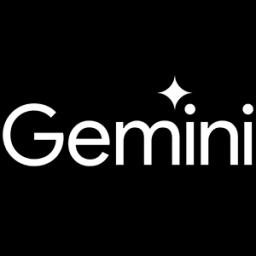
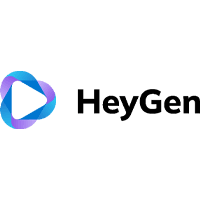
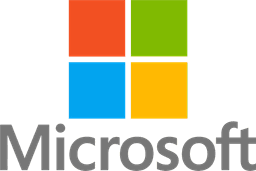
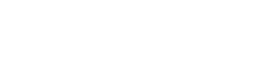
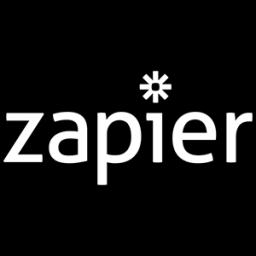
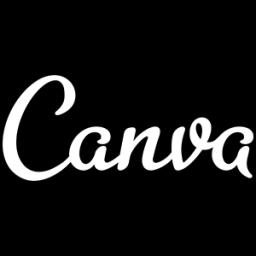
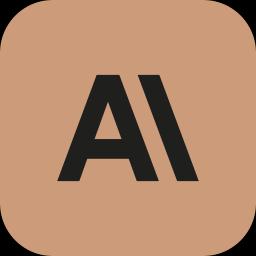
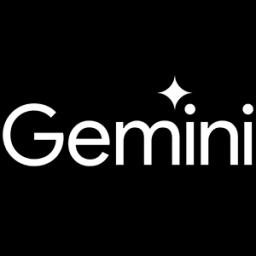
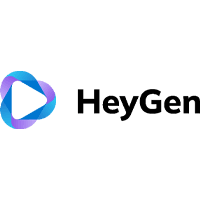
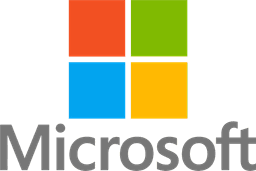
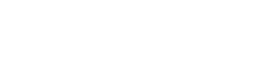
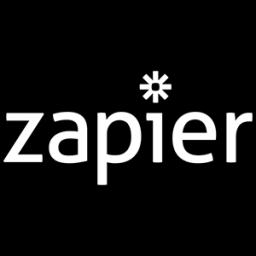
Furthermore, the implications of high-quality visual data extend beyond immediate operational benefits. They contribute significantly to broader economic, social, and political paradigms. Economically, there is a distinct trend towards greater investments in enhanced technologies to support high-quality visual data, as businesses are increasingly aware of the competitive edge such investments can provide. Socially, the improvements in safety and product quality are palpable, leading to enhanced consumer trust and satisfaction. Politically, there is an imperative need for creating and adhering to robust regulatory frameworks to manage aspects like data privacy, algorithmic accountability, and other ethical concerns that accompany the deployment of Industrial AI (source).
In light of these transformations, the responsible adoption of Industrial AI, underscored by the reliability of visual data, calls for strategic foresight and comprehensive planning. The interplay between technological advancements and industry standards shapes how effectively industries can leverage AI to optimize operations while mitigating potential risks associated with data mismanagement and ethical breaches. The journey towards realizing the full potential of Industrial AI is a complex yet rewarding endeavor that rests on the pillars of high-quality visual data and informed governance.
Challenges of Poor Visual Data Quality
The challenges posed by poor visual data quality in industrial AI systems are profound and multifaceted. One of the primary issues is model drift, where models that initially performed accurately start generating flawed predictions due to changes in data patterns not being effectively captured. This happens because low-quality data fails to provide a comprehensive and consistent representation of the real-world scenarios in which the AI operates. As a result, AI systems can produce increased false positives or negatives, leading to erroneous analyses that compromise operational decision-making and safety.[Read more]
Furthermore, poor visual data quality significantly heightens safety risks and erodes trust within industrial environments. Without accurate visual data, AI systems may overlook critical cues that indicate potential equipment failures or unsafe working conditions, failing to alert human operators to intervene promptly. This erosion of trust is detrimental, as stakeholders might become apprehensive about relying on AI systems, especially in safety-critical applications.[More details here]
Learn to use AI like a Pro
Get the latest AI workflows to boost your productivity and business performance, delivered weekly by expert consultants. Enjoy step-by-step guides, weekly Q&A sessions, and full access to our AI workflow archive.
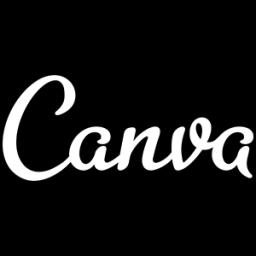
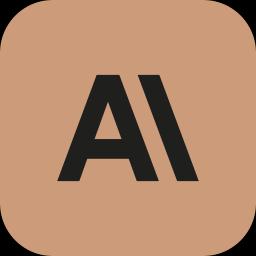
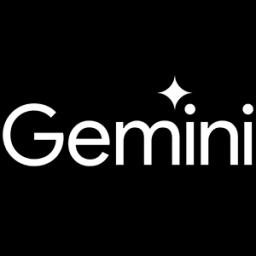
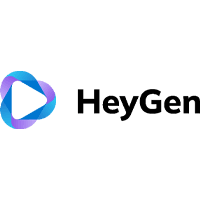
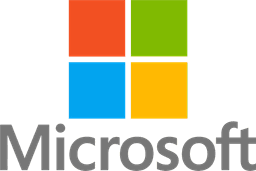
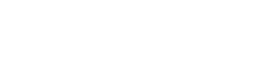
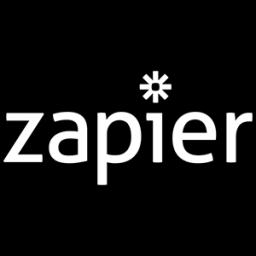
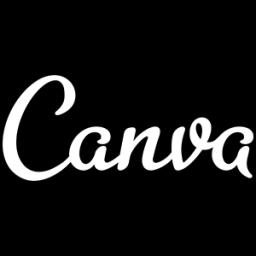
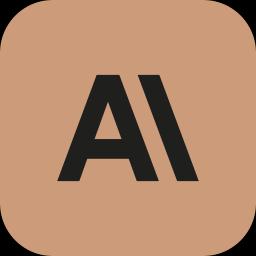
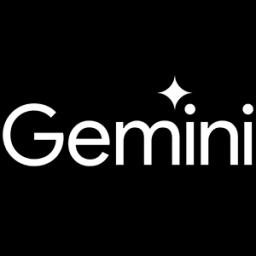
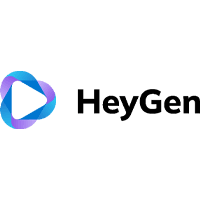
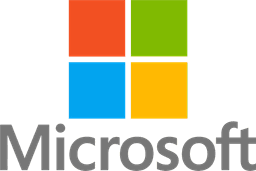
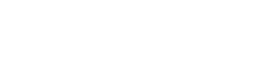
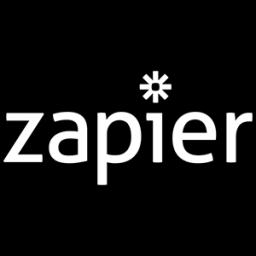
Another challenge linked to poor visual data quality is the increase in operational inefficiencies. Inaccurate data leads to misdirected maintenance efforts, as AI systems may signal alerts for normal variances as anomalies or fail to detect actual issues. This misalignment results in unnecessary maintenance procedures, wasting both time and resources, while genuine problems might go unnoticed, escalating into more significant issues.Learn more
Improving the quality of visual data requires comprehensive measures, including upgrading both hardware and software components. For instance, better resolution cameras and sophisticated image processing software can enhance data accuracy. Additionally, more intelligent data labeling practices, such as involving domain experts and utilizing advanced learning techniques, can refine the data that AI models learn from, reducing biases and improving model performance.Explore further
Moreover, integrating edge intelligence in industrial AI systems plays a crucial role in mitigating poor data quality issues. Edge computing allows for immediate filtering and processing of data at its source, reducing the burden on central systems and enabling quicker responses to irregularities. This approach not only improves the speed of data handling but also ensures data integrity and relevance before reaching the core processing units, thus preserving the quality of insights provided by the AI.Find out more
Strategies for Improving Visual Data Quality
Improving visual data quality is a cornerstone for the successful deployment of industrial AI systems. To this end, enhancing hardware capabilities by utilizing high-resolution cameras and advanced sensors is pivotal. These upgrades provide clearer and more precise data, significantly reducing the likelihood of errors. Additionally, the integration of edge computing solutions facilitates the local processing of data, which not only speeds up response times but also enables real-time data filtering to ensure that only the most accurate data is utilized for decision-making processes. This approach aligns with platforms like Volt Active Data that specialize in real-time data ingestion, offering infrastructure that can seamlessly integrate with existing computer vision pipelines for streamlined operations .
The role of smarter data labeling cannot be overstated in improving visual data quality. By involving domain experts in the labeling process, AI models become more contextually aware and accurate. Furthermore, the adoption of semi-supervised learning and active learning techniques can vastly improve the efficiency and effectiveness of data annotation. These methodologies ensure that models are trained on well-curated data, thus enhancing the overall quality of AI outputs. Periodic audits and continuous evaluation of data labeling processes serve as quality checks that prevent bias and maintain the integrity of the visual data utilized by AI systems .
Utilizing edge intelligence as part of a comprehensive strategy to improve visual data quality offers several advantages. By processing data locally, devices equipped with edge intelligence can filter out corrupted or irrelevant data, thereby reducing the cognitive load on central systems. The local inference ability of these devices ensures that decisions are made quickly, resembling near-immediate responsiveness, which is crucial in dynamic industrial environments. This technology not only enhances efficiency but also allows for the collection and analysis of data even in bandwidth-constrained settings, thereby optimizing the utilization of visual data resources .
Learn to use AI like a Pro
Get the latest AI workflows to boost your productivity and business performance, delivered weekly by expert consultants. Enjoy step-by-step guides, weekly Q&A sessions, and full access to our AI workflow archive.
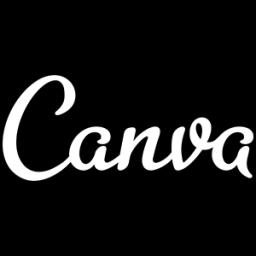
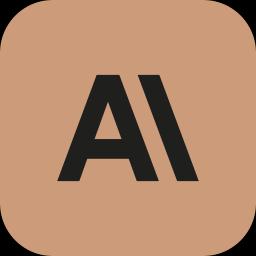
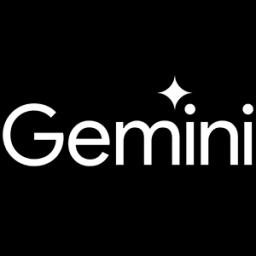
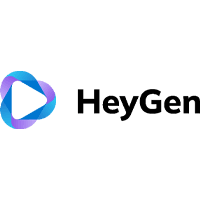
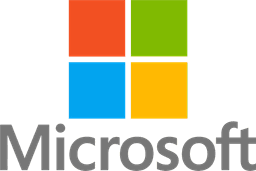
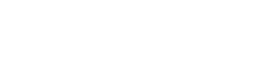
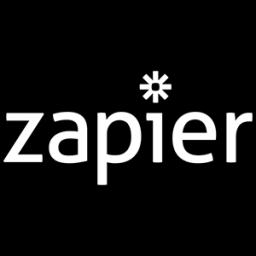
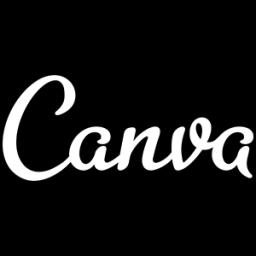
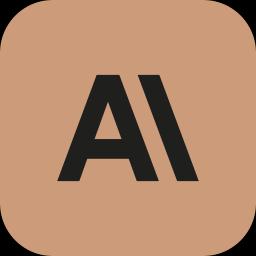
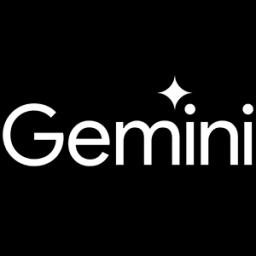
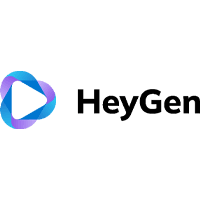
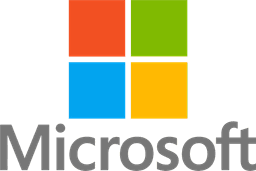
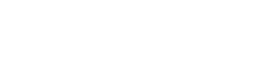
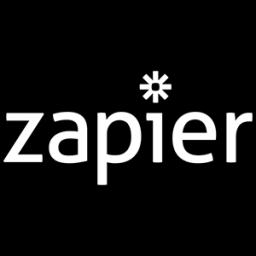
Another crucial strategy for enhancing visual data quality involves software improvements and innovations in data processing techniques. Implementing sophisticated algorithms for image processing and data analysis ensures that noisy or poor-quality data is swiftly identified and rectified before it can influence AI models. Platforms that support real-time visual intelligence, like the one offered by Volt Active Data, enable contextual decision-making by integrating seamlessly with various AI processes. The synergy achieved through such integrated solutions allows industries to leverage their AI systems to full potential, ensuring timely interventions and maintaining operational efficiency .
The Role of Real-Time Processing and Edge Intelligence
The integration of real-time processing and edge intelligence in industrial AI systems is critical for leveraging high-quality visual data in decision-making processes. In dynamic environments, where rapid responses are essential, real-time data processing allows systems to ingest and analyze data instantaneously, enabling timely and accurate interventions. Edge intelligence enhances this capability by processing data locally, minimizing the latency issues inherent in cloud-based systems. This local processing not only speeds up the analysis but also ensures that sensitive data remains secure on-site.
Furthermore, integrating edge intelligence with real-time processing is pivotal for improving the overall quality of visual data in industrial AI. By handling preprocessing tasks locally, such as filtering out noise and managing corrupted data, edge devices help maintain the integrity of the input data. This ensures that only the most relevant and high-quality images are analyzed, reducing the potential for errors in AI models. As referenced in the article "Why Industrial AI Fails Without Quality Visual Data", edge intelligence supports immediate data correction and management, which is essential for sustaining the performance and reliability of AI systems.
The role of real-time processing and edge computing in handling visual data is further underscored by platforms like Volt Active Data. This platform exemplifies how real-time data ingestion and processing can integrate seamlessly with existing computer vision systems to enable contextual and informed decision-making. By providing a robust infrastructure that supports both edge and hybrid-cloud environments, such platforms facilitate efficient data handling and reduce potential bottlenecks in data processing pipelines.The RTInsights article details how these systems enable immediate interventions and streamline operations, ultimately enhancing productivity and safety.
Volt Active Data's Solutions for Industrial AI
Volt Active Data has carved out a pivotal role in the realm of industrial AI by addressing the crucial need for high-quality visual data. This platform offers robust solutions for real-time data ingestion, processing, and response. By integrating seamlessly with computer vision pipelines, Volt Active Data enables contextual decision-making, crucial for industrial settings where every millisecond counts. Ensuring the prompt and accurate processing of visual data not only enhances the operational efficiency but also significantly contributes to safety by mitigating risks associated with delayed or incorrect data interpretations. The necessity of such solutions is underscored by experts like Salvatore Salamone, who highlight the limitations of AI models when faced with poor data quality, thereby advocating for enhanced hardware and software solutions along with smarter labeling practices to boost industrial AI's effectiveness. For a deeper insight into these challenges and solutions, particularly why visual data quality is paramount for industrial AI's success, you can explore more in this RTInsights article.
Expert Insights on Visual Data Quality
In the rapidly evolving domain of Industrial AI, the quality of visual data plays a pivotal role in determining the effectiveness and reliability of AI systems. According to a discussion on RTInsights, poor visual data quality is a critical barrier that can lead to numerous setbacks such as model drift, inaccurate predictions, and increased safety risks. These challenges emphasize the necessity for robust data quality measures to maintain trust and functionality in AI applications.
Learn to use AI like a Pro
Get the latest AI workflows to boost your productivity and business performance, delivered weekly by expert consultants. Enjoy step-by-step guides, weekly Q&A sessions, and full access to our AI workflow archive.
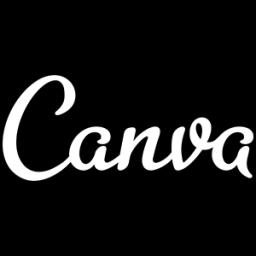
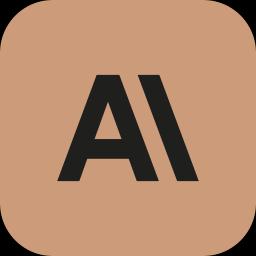
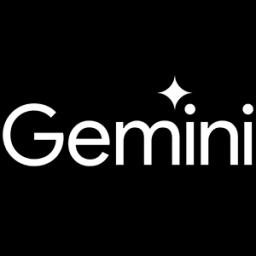
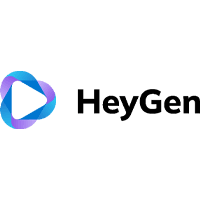
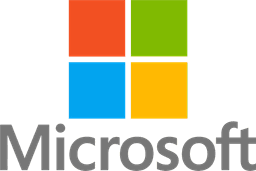
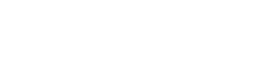
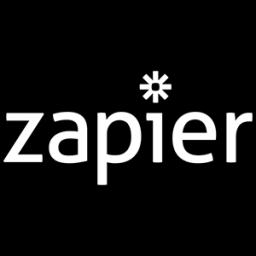
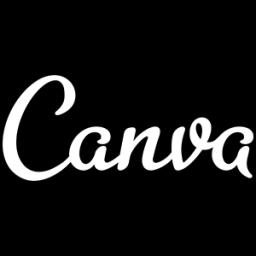
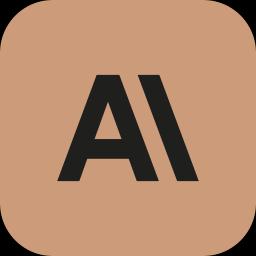
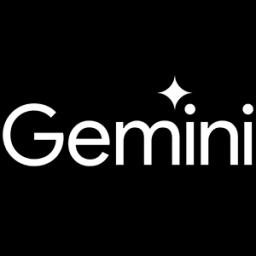
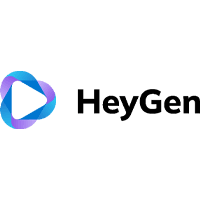
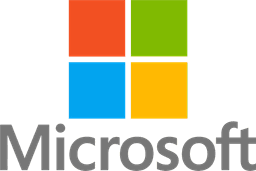
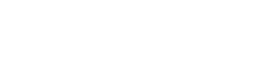
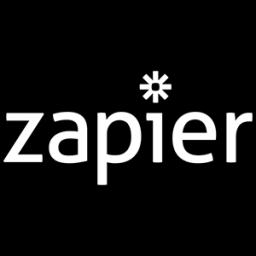
Expert Salvatore Salamone of RTInsights highlights that even sophisticated AI models struggle with subpar visual data inputs, emphasizing the need for improvements in both hardware and software systems to enhance data capture and processing accuracy (RTInsights). Common issues, such as poor lighting and misaligned cameras, can severely hamper the data quality, resulting in wasted resources and potential safety hazards.
Strategies to improve visual data quality include hardware and software upgrades, smarter data labeling, and the integration of edge intelligence, allowing real-time data processing and localized decision-making. As pointed out in the RTInsights article, tools like Volt Active Data provide platforms that enhance real-time data capabilities by integrating seamlessly with existing AI and computer vision infrastructure. This approach not only bolsters the quality of data but also facilitates timely and contextually aware decisions in dynamic industrial environments.
Public Reaction and Discussion
The public reaction to the pivotal role that high-quality visual data plays in industrial AI systems appears muted, according to the available context. While the article emphasizes the significance of excellent visual data and suggests solutions such as hardware and software upgrades, along with the adoption of edge intelligence, there seems to be a lack of direct engagement or response from the general public. Discussions on platforms like Reddit touch upon the potential of AI in enhancing visual and model-based testing for quality control, yet these do not directly grapple with the specific challenges highlighted in the article. This could indicate a gap in awareness or an underestimation of the challenges posed by poor visual data quality in industrial AI [1](https://www.rtinsights.com/why-industrial-ai-fails-without-quality-visual-data/).
Moreover, expert comments and insights from the industry recognize the critical nature of visual data quality, but the general public conversation has not quite caught on to these professional circles' discussions. People might be broadly interested in AI's capabilities, especially its promise for efficiency and productivity. Still, there is limited evidence of a widespread understanding of the nuances related to visual data issues. As a result, this lack of public discourse could delay widespread acceptance and the necessary urgency in addressing these visual data quality challenges at larger scales [1](https://www.rtinsights.com/why-industrial-ai-fails-without-quality-visual-data/).
Salvatore Salamone from RTInsights pinpoints the exact issues caused by low-grade visual data, such as wasted resources and safety risks due to poor lighting and camera misalignment—yet these remain mostly within the confines of industry discussions. Similarly, while Upasna Doshi of Akaike emphasizes frameworks and AI-powered tools to combat poor data quality, these topics haven't stirred much public commentary nor extensive debate. This suggests that while experts are gearing up for heightened quality demands, the conversation has yet to trickle down to laypersons or prompt significant public policy responses [1](https://www.rtinsights.com/why-industrial-ai-fails-without-quality-visual-data/).
Economic Implications of Industrial AI
The economic implications of implementing Industrial AI are profoundly shaping modern industries, primarily through increased investment in technology and subsequent innovation. Companies aiming to harness the power of Industrial AI are compelled to invest in high-resolution cameras, advanced sensors, cutting-edge data processing software, and robust data infrastructure like edge computing and real-time data pipelines. This surge in investment significantly boosts innovation within fields related to AI, such as computer vision, machine learning, and data analytics. The competitive edge gained by companies through precise quality control, predictive maintenance, and streamlined processes translates to considerable cost savings and higher productivity, establishing a growth trajectory for businesses vested in providing high-quality visual data and related technologies. More insights into these advancements can be found in the discussion on industrial AI's dependency on quality visual data [here](https://www.rtinsights.com/why-industrial-ai-fails-without-quality-visual-data/).
Learn to use AI like a Pro
Get the latest AI workflows to boost your productivity and business performance, delivered weekly by expert consultants. Enjoy step-by-step guides, weekly Q&A sessions, and full access to our AI workflow archive.
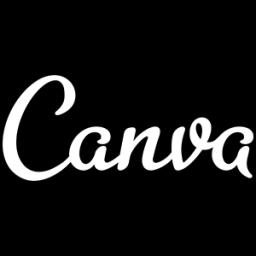
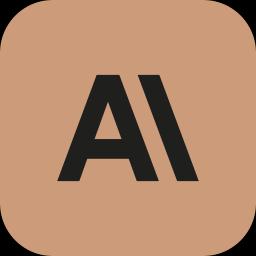
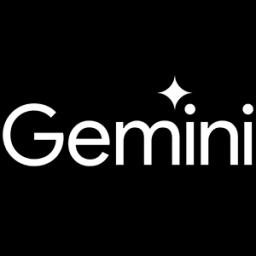
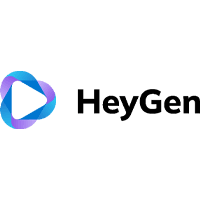
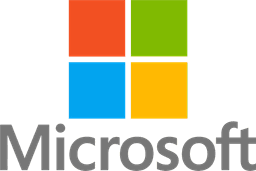
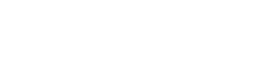
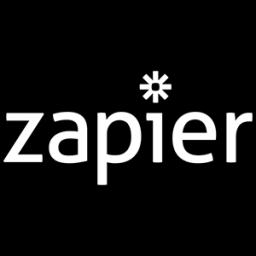
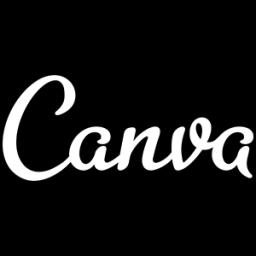
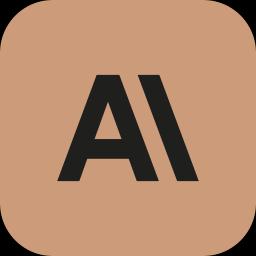
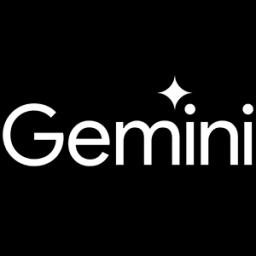
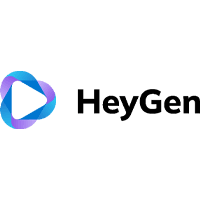
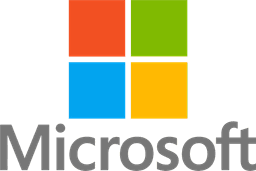
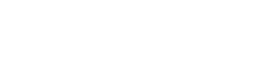
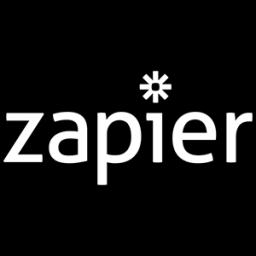
However, the economic landscape is not without challenges. As AI-driven systems enhance operational efficiency, there is a potential risk for job displacement, especially in roles heavily reliant on manual inspection and repetitive tasks. While AI technologies advance, there is an urgent need to address the shifting job market dynamics through proactive reskilling and workforce development initiatives. Such initiatives are vital to prepare the workforce for new roles created by AI technologies, mitigating social disruption and ensuring inclusive economic growth. This perspective underscores the importance of adapting to an evolving job market fueled by Industrial AI, as highlighted by the insights shared [here](https://www.rtinsights.com/why-industrial-ai-fails-without-quality-visual-data/).
Social Impact and Safety Considerations
In the evolving landscape of industrial AI, the significance of visual data extends beyond technological efficiency to encompass profound social and safety considerations. High-quality visual data is not only a catalyst for improved operational outcomes but also a cornerstone for enhancing workplace safety and societal welfare. According to an insightful article from RTInsights, poor-quality visual data can lead to increased safety risks, among other issues such as model drift and inaccurate predictions. By upgrading both hardware and software, industries can significantly mitigate these risks, ultimately fostering a safer working environment ().
Furthermore, the integration of advanced visual data systems in industrial settings is not merely about catching up with technological advancements but rather about safeguarding human-centric outcomes. Real-time processing capabilities, as discussed by Volt Active Data, play a crucial role in identifying and responding to potential hazards swiftly, thus underscoring the essentiality of high-quality visual data in maintaining and enhancing safety standards. These technological improvements not only prevent accidents but also bolster employee confidence and societal trust in AI-driven industrial solutions ().
On a broader scale, the social impact of high-quality visual data in AI systems includes improved product reliability and ethical manufacturing practices. With enhanced data accuracy, companies can ensure superior product quality, thereby strengthening brand reputation and consumer trust. Equally important is the potential for mitigating inherent biases in AI models through comprehensive and unbiased data collection strategies, which are crucial in avoiding the perpetuation of social inequalities. As Upasna Doshi from Akaike indicates, the financial and reputational risks of poor data quality necessitate robust data quality frameworks to prevent biases and ensure ethical AI usage ().
Ethical Concerns and Bias in AI Systems
Artificial intelligence (AI) systems have become increasingly embedded in various aspects of our daily lives, offering unprecedented capabilities in data processing and decision-making. However, these systems are not without their ethical challenges, particularly concerning bias and fairness. Algorithmic bias in AI systems can occur due to skewed data inputs, which may not represent diverse populations adequately. This can lead to discriminatory outcomes, particularly in critical areas such as hiring, lending, and law enforcement. Transparency in AI algorithms is crucial to ensure that biases are identified and addressed, thereby promoting equity and fairness in AI applications.
Industrial AI systems, despite their potential for enhancing efficiency and productivity, can also reflect significant ethical concerns when it comes to data quality. The effectiveness of these systems is heavily dependent on high-quality visual data, as noted by Salvatore Salamone. Poor visual data quality can introduce bias, degrade AI model performance, and compromise safety, as poorly aligned cameras or inadequate lighting conditions skew input data, resulting in errors and unsafe decisions (). To mitigate such risks, investments in better hardware and smarter data labeling are essential.
Learn to use AI like a Pro
Get the latest AI workflows to boost your productivity and business performance, delivered weekly by expert consultants. Enjoy step-by-step guides, weekly Q&A sessions, and full access to our AI workflow archive.
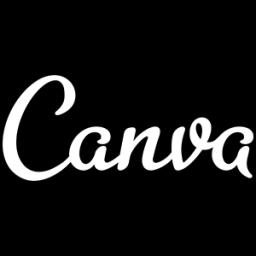
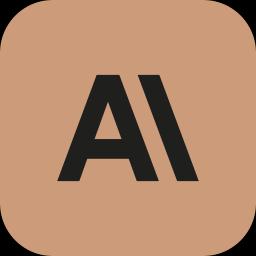
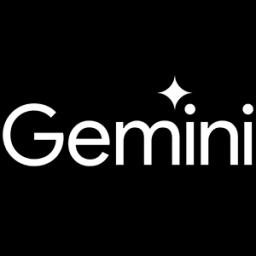
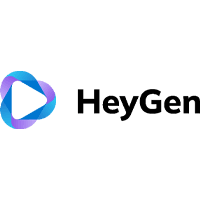
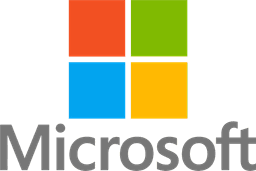
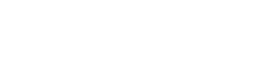
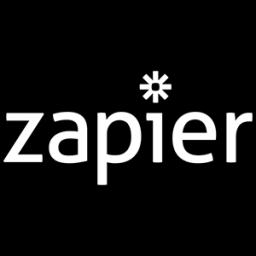
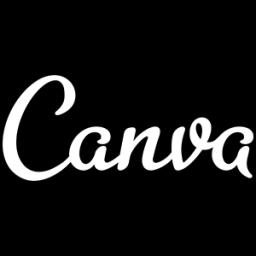
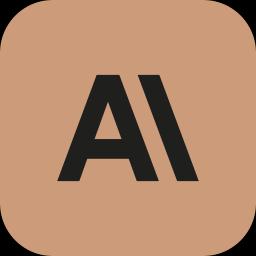
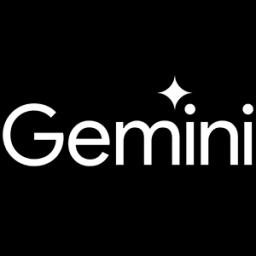
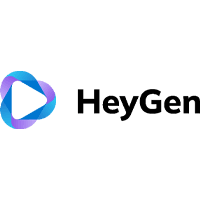
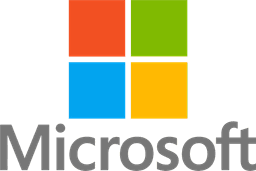
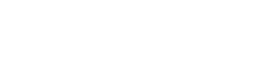
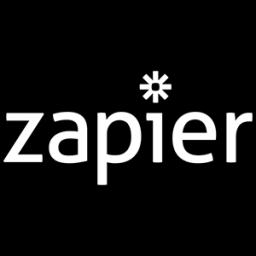
Moreover, bias in AI systems can have severe implications beyond operational inefficiencies. As Upasana Doshi points out, inadequate data quality and resulting biases can lead to model drift and erode trust in AI’s decision-making processes (). Ensuring fairness and addressing these ethical challenges require a robust data quality management framework and the implementation of comprehensive auditing processes to continually assess and correct biases.
Public trust in AI systems hinges on these ethical dimensions being adequately addressed. Transparency and clear communication about how AI models work and their decision-making processes are vital. This not only involves informing the public about the strengths and limitations of AI but also ensuring informed consent where personal or sensitive data is used. The development of regulatory frameworks that emphasize data privacy, bias prevention, and accountability is essential to guide the ethical use of AI technologies and secure societal acceptance.
Regulatory and Political Impacts of AI
The integration of artificial intelligence (AI) into various sectors is substantially influenced by regulatory and political frameworks. As AI technologies develop rapidly, regulators are confronted with the challenge of crafting policies that effectively address the unique risks and opportunities these technologies present. Issues such as data privacy, security, and ethical considerations have moved to the forefront of legislative agendas. Ensuring robust regulatory standards is imperative for fostering innovation while safeguarding public interest [1](https://www.rtinsights.com/why-industrial-ai-fails-without-quality-visual-data/).
Moreover, the political landscape plays a pivotal role in shaping the trajectory of AI development and deployment. Governments worldwide are investing in AI research and infrastructure, reflecting its strategic importance in attaining technological leadership. Political discourse increasingly focuses on balancing the benefits of AI, such as enhanced efficiency and economic growth, with the socio-economic challenges it may pose, including job displacement and ethical use of AI systems [1](https://www.rtinsights.com/why-industrial-ai-fails-without-quality-visual-data/).
International collaboration on AI standards is becoming increasingly essential. With AI applications transcending national boundaries, harmonized regulations could facilitate seamless integration and operation of AI technologies across global markets. However, geopolitical competition may complicate these efforts, as nations strive to safeguard their interests while collaborating on establishing universal guidelines [1](https://www.rtinsights.com/why-industrial-ai-fails-without-quality-visual-data/).
The impact of politics on AI is not purely regulatory. Public perception and trust in AI systems are significantly influenced by political narratives and media portrayals. Transparency in AI operations and accountability mechanisms are crucial for building this trust. Furthermore, political initiatives that emphasize inclusivity and address concerns such as algorithmic bias can play a critical role in ensuring equitable AI deployment, fostering public confidence in these technologies [1](https://www.rtinsights.com/why-industrial-ai-fails-without-quality-visual-data/).
Learn to use AI like a Pro
Get the latest AI workflows to boost your productivity and business performance, delivered weekly by expert consultants. Enjoy step-by-step guides, weekly Q&A sessions, and full access to our AI workflow archive.
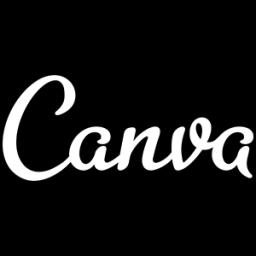
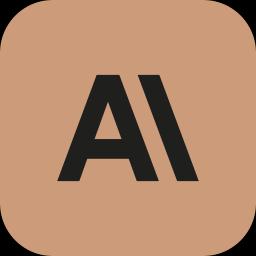
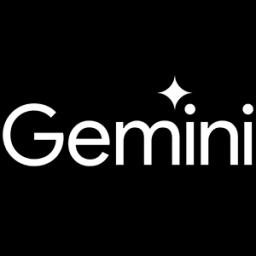
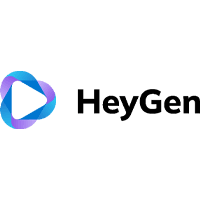
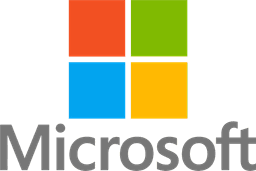
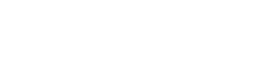
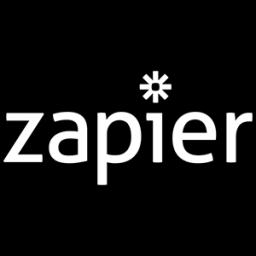
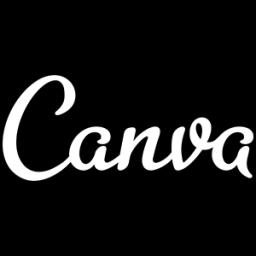
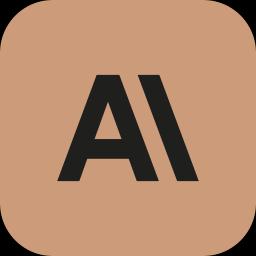
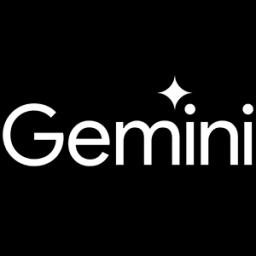
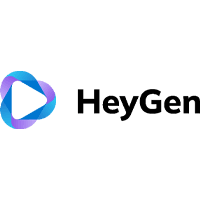
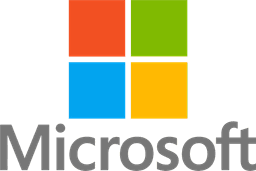
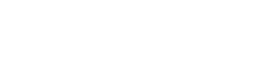
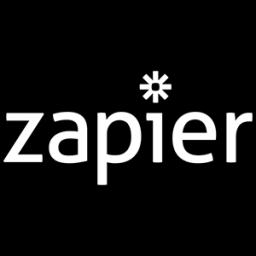
Ultimately, the regulatory and political impacts of AI underscore a crucial turning point for technological governance. As AI becomes increasingly integrated into essential industries like healthcare, finance, and transportation, a proactive approach towards inclusive, transparent, and accountable AI governance is necessary. Such measures will not only bolster public trust but also ensure that AI technologies contribute positively to societal advancement and economic prosperity [1](https://www.rtinsights.com/why-industrial-ai-fails-without-quality-visual-data/).
Conclusion and Future Considerations
In conclusion, the pursuit of high-quality visual data is pivotal for the advancement and effectiveness of industrial AI systems. This necessity stems from the realization that the value and accuracy of AI outputs are directly tied to the quality of input data. As noted by experts such as Salvatore Salamone, even the most sophisticated AI models are only as effective as the data they process. This underscores the critical importance of continuous improvements in visual data fidelity, encompassing hardware and software upgrades, as well as innovative data processing techniques.
Looking forward, future considerations must prioritize building infrastructure and ecosystems that support high-quality visual data management. This involves significant investments in edge computing technologies that enable real-time data processing, ensuring swift and contextual decision-making in dynamic industrial environments. Companies like Volt Active Data exemplify the integration of these capabilities by offering platforms that efficiently manage real-time data and enhance AI-driven processes. The trajectory towards robust industrial AI systems will likely involve more collaborative efforts across sectors to continually refine the quality and applicability of visual data technologies.
Moreover, a proactive approach to addressing potential future challenges is essential. As more industries integrate AI technologies, ensuring ethical considerations in their deployment will be vital. Addressing data bias, ensuring transparency in AI operations, and fostering public trust and understanding are necessary steps in achieving sustainable growth and acceptance of AI systems. Additionally, as highlighted in the broader discussion of data readiness by experts such as Upasna Doshi, financial and reputational considerations driven by data quality concerns must be addressed through comprehensive frameworks and AI-powered tools. Investing in such strategies will be crucial for mitigating risks associated with poor data practices and enhancing AI model reliability and trustworthiness.