We've used up all the data...now what?
AI Hits 'Peak Data,' But Google DeepMind Has a Clever Plan to Keep Progress Rolling!
Last updated:

Edited By
Mackenzie Ferguson
AI Tools Researcher & Implementation Consultant
AI's rapid progress is hitting a snag known as 'peak data,' as researchers grapple with the exhaustion of high-quality internet training data. Enter Google DeepMind with an innovative 'test-time compute' solution, which slices complex queries into smaller, manageable pieces, helping AI continue to churn out high-quality outcomes. Discover how these high-quality outputs could serve as fresh training data, sparking a cycle of self-improvement and keeping AI advancement on track!
Introduction to Peak Data
The development of artificial intelligence (AI) has reached what some are calling a "peak data" scenario, where readily available, high-quality training data from the internet has been largely exhausted. Researchers argue that this scarcity poses a significant challenge to further progress, potentially slowing down the remarkable pace at which AI models have evolved in recent years.
The Challenges of Peak Data in AI
Artificial Intelligence (AI) is on a transformative journey, driving innovation and redefining efficiency across sectors. However, as AI systems grow more sophisticated, they encounter new challenges. One pressing issue in the AI landscape is the concept of 'peak data,' a scenario where AI models have already leveraged most of the available high-quality training data from the internet. This bottleneck threatens to decelerate the rapid pace at which AI advancements have been made, necessitating innovative solutions to circumvent data scarcity.
Learn to use AI like a Pro
Get the latest AI workflows to boost your productivity and business performance, delivered weekly by expert consultants. Enjoy step-by-step guides, weekly Q&A sessions, and full access to our AI workflow archive.
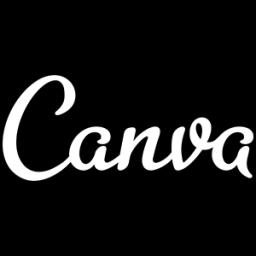
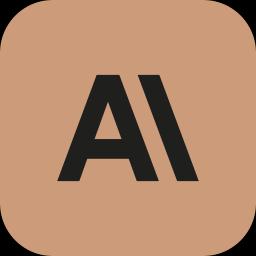
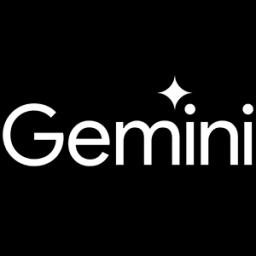
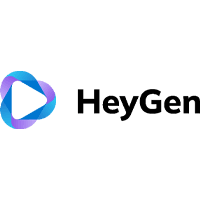
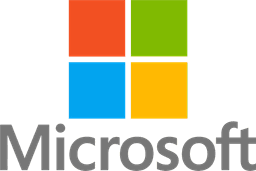
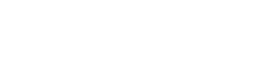
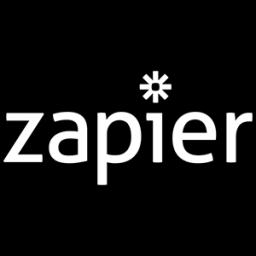
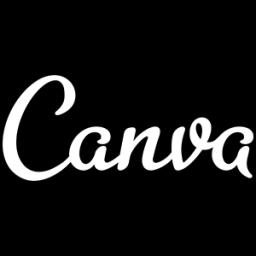
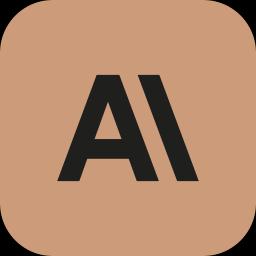
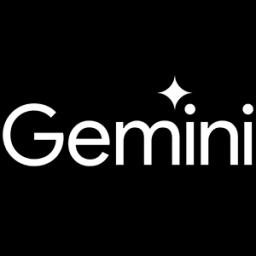
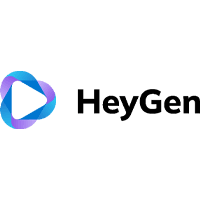
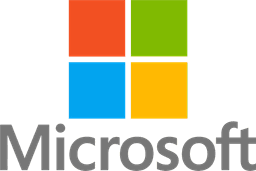
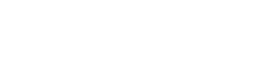
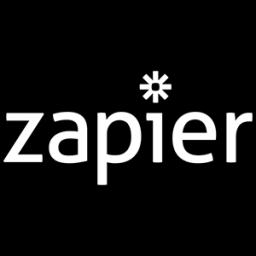
Exploring Test-Time Compute Solutions
The concept of 'peak data' presents a significant turning point in the AI landscape, as it signifies the exhaustion of readily available high-quality training data from the internet. This situation poses a potential roadblock for the continued rapid development of AI technologies, necessitating innovative solutions to overcome data scarcity challenges. To address this emerging issue, researchers, particularly at Google DeepMind, are investigating the potential of 'test-time compute' to enable AI models to self-improve despite data constraints.
Test-time compute offers a promising avenue to mitigate the peak data challenge by enabling AI models to process complex queries through a step-by-step reasoning approach. This method involves breaking down intricate questions into smaller, more manageable prompts, allowing AI systems to generate high-quality outputs. These outputs can then serve as new training data, fostering a self-improving loop where the models fine-tune their abilities over time. This innovative approach, while still under investigation, has garnered attention and optimism from industry leaders such as Microsoft CEO Satya Nadella, who views it as a potent enhancement capability for AI models.
However, the implementation of test-time compute is not without its challenges. The approach's success largely hinges on its ability to generalize beyond straightforward tasks, such as basic mathematical problems, to more open-ended queries where answers may not be easily verifiable. There is a degree of skepticism regarding its applicability to tasks that require creative or less deterministic responses, which presents a hurdle that researchers must address through rigorous testing and refinement. Additionally, competitive dynamics within the AI sector highlight the importance of developing robust, scalable solutions to maintain an edge over rival companies exploring similar technologies.
Industry leaders and experts are acutely aware of the implications of reaching peak data, voicing concerns over the potential slowdown of AI advancements and the increased competition for scarce data resources. DeepSeek's reported use of outputs from OpenAI's model to train its own systems underscores the competitive pressures and ethical considerations at play in today's AI research ecosystem. Ensuring fair data usage and attribution will be critical in navigating this competitive landscape.
Learn to use AI like a Pro
Get the latest AI workflows to boost your productivity and business performance, delivered weekly by expert consultants. Enjoy step-by-step guides, weekly Q&A sessions, and full access to our AI workflow archive.
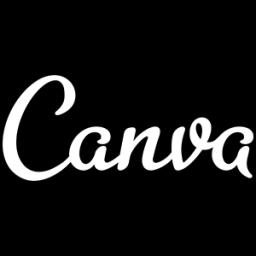
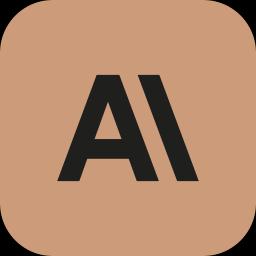
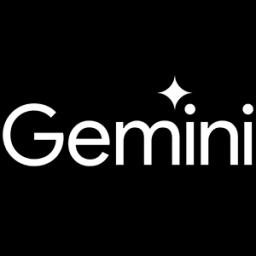
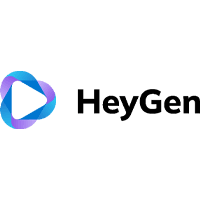
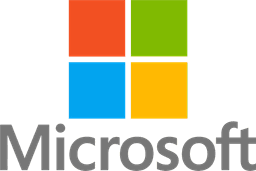
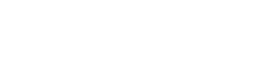
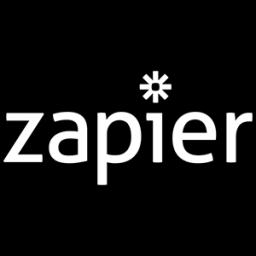
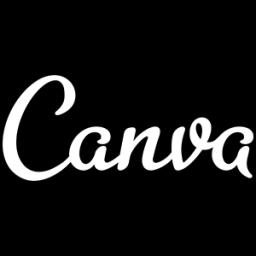
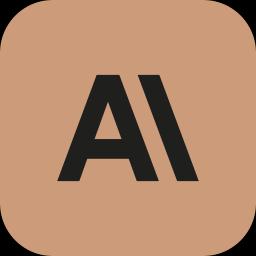
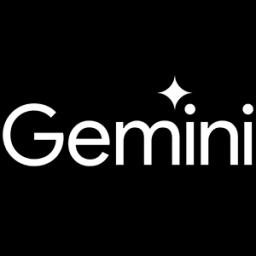
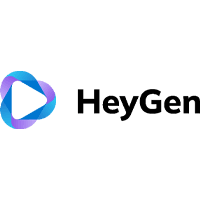
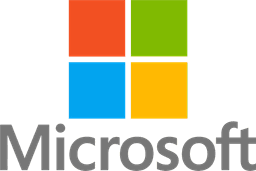
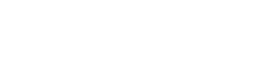
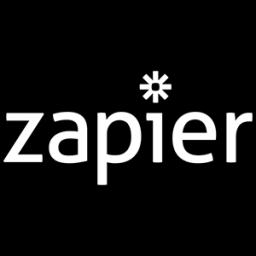
Nevertheless, test-time compute holds considerable promise for various sectors impacted by AI technologies. By enabling machines to learn efficiently and continuously even with limited datasets, this method could pave the way for more sustainable and adaptive AI systems. Its success would not only bolster the capabilities of existing AI systems but also drive forward alternative training methodologies that reduce dependency on massive pre-training datasets. This evolution in AI training paradigms could lead to the development of more energy-efficient and data-conscious AI models, aligning with broader technological, social, and economic trends.
Industry Leaders' Perspectives on Data Limitations
The concept of 'peak data' has been a predominant discussion among industry leaders, highlighting a pivotal point where artificial intelligence (AI) development is hindered by a scarcity of high-quality training data. This limitation could potentially decelerate the rapid pace of AI advancements as current models have exhausted readily accessible data from the internet. Leading voices in the field, including OpenAI’s co-founder Ilya Sutskever, have acknowledged this challenge, emphasizing a pressing need for innovative solutions that address this bottleneck.
In response to these data restrictions, researchers, particularly at Google DeepMind, are exploring innovative techniques such as 'test-time compute'. This approach involves deconstructing complex queries into smaller, manageable prompts, allowing AI models to iteratively reason through problems in a step-by-step fashion. As these models generate higher-quality outputs, these can, in turn, serve as fresh training data, fostering a self-sustaining cycle of improvement.
The exploration of test-time compute has also received endorsements from significant industry figures. Satya Nadella, CEO of Microsoft, has publicly expressed optimism, perceiving it as a potential new 'scaling law' for AI capabilities. This perspective suggests a promising shift in how AI models might be trained and improved without relying solely on the expansive volume of pre-existing datasets.
Despite such optimism, the practicality of test-time compute is still under examination, particularly regarding its applicability to tasks that lack clear, verifiable answers, such as essay writing. The industry thus stands at a crossroads, where the generalizability of these new methods will dictate their long-term effectiveness in overcoming the peak data challenge.
Meanwhile, the competitive landscape of AI development is highlighted by companies like DeepSeek, a Chinese AI laboratory, which reportedly utilized outputs from OpenAI’s models for their training processes. This revelation not only underscores the intense competition prevailing in the industry but also raises ethical questions about content usage and data sharing in AI training contexts.
Learn to use AI like a Pro
Get the latest AI workflows to boost your productivity and business performance, delivered weekly by expert consultants. Enjoy step-by-step guides, weekly Q&A sessions, and full access to our AI workflow archive.
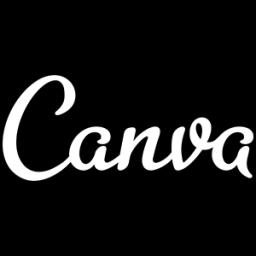
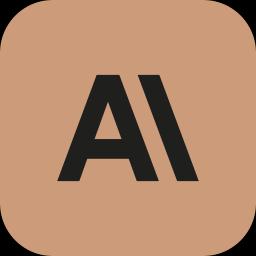
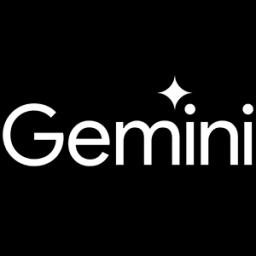
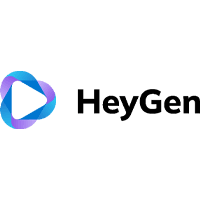
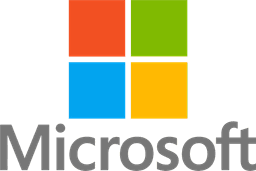
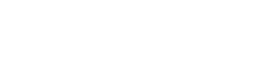
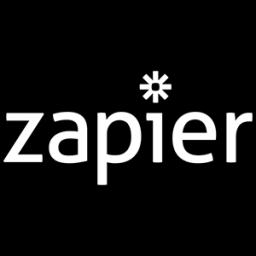
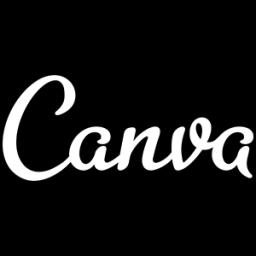
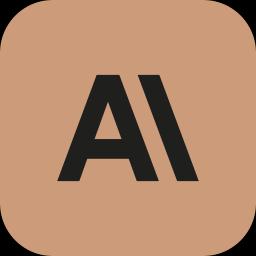
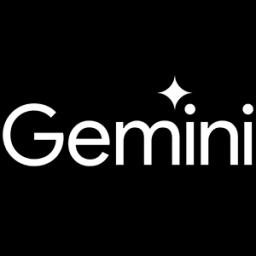
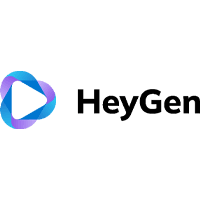
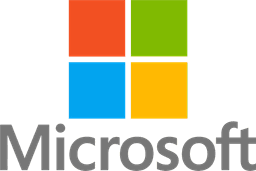
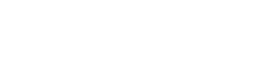
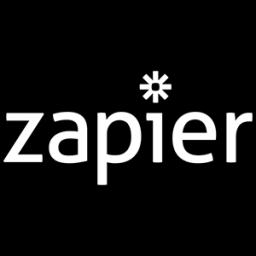
Overall, industry leaders are actively seeking solutions to the peak data challenge, balancing optimism for innovative computational approaches with a cautious evaluation of their limitations and societal implications. The road ahead includes navigating data scarcity while fostering technological advancements in a competitive and ethically sensitive environment.
Competitive Dynamics in AI Development
The race to dominate artificial intelligence development has intensified, driven largely by challenges like the 'peak data' phenomenon. As AI models reach the limits of available high-quality training data, the industry faces the formidable task of maintaining its rapid advancement trajectory. A significant player in addressing these constraints is Google DeepMind. They have pioneered 'test-time compute,' a novel approach that allows AI systems to break down complex queries into smaller, more manageable prompts and solve problems incrementally. This process not only enhances AI reasoning capabilities but also supplies new, high-quality data for continuous training, facilitating a self-improving loop.
Elaborating on the solution of 'test-time compute,' Google DeepMind and its peers, such as OpenAI, are exploring this method to tackle the data scarcity issue. By allowing AI models to perform additional calculations during inference, this technique generates better outputs, which can serve as fresh training data. This approach has gained endorsement from industry influencers like Microsoft CEO Satya Nadella, who views it as a potential 'scaling law' that could significantly boost AI capacities. Yet, the challenge remains in extending this approach beyond tasks with straightforward solutions—like mathematical problems—to those with less clear-cut answers.
The competition in AI development is not limited to addressing technical constraints but also revolves around strategic maneuvers among leading tech entities. The actions of companies such as DeepSeek, which reportedly used AI-generated outputs from rivals like OpenAI for training, highlight a fiercely competitive environment. This dynamic raises essential questions about ethics and intellectual property, especially as firms seek to leverage AI's evolving capabilities to their advantage. Public discourse around these practices continues to reflect both skepticism and optimism towards these competitive dynamics.
Industry experts and researchers acknowledge the urgent need to develop new AI training methodologies that do not rely on the traditional data-heavy paradigms. The concept of 'peak data' underscores a critical juncture in AI evolution, pressing researchers to devise innovative solutions to sustain progress. In this context, 'test-time compute' emerges as a promising strategy, though it faces scrutiny regarding its generalizability and practical deployment. Its success could redefine AI model development, especially if it adapts effectively to varied tasks beyond its current scope.
With the backdrop of 'peak data', the future of AI development is poised at an inflection point marked by both challenges and opportunities. Economically, the potential slowdown in AI progression could reshape investment patterns and spur the emergence of niche markets dedicated to synthetic data and novel training techniques. Socially, as the AI narrative shifts, debates around data privacy and ethical AI use gain prominence, reflecting public consciousness and policy discussions. The geopolitical landscape also sees increased tension as nations vie for AI supremacy, considering data as a strategic asset. Technologically, the urgency to innovate drives a focus on alternative methodologies and resource-efficient AI models, hinting at a future where AI can perform optimally with constrained datasets.
Learn to use AI like a Pro
Get the latest AI workflows to boost your productivity and business performance, delivered weekly by expert consultants. Enjoy step-by-step guides, weekly Q&A sessions, and full access to our AI workflow archive.
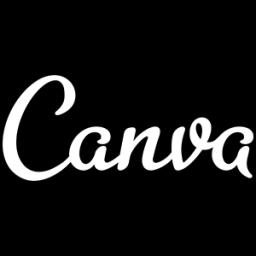
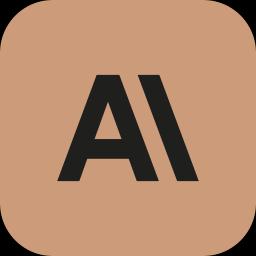
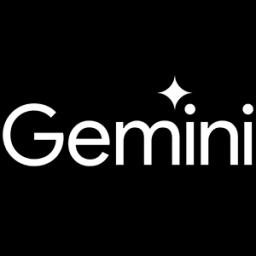
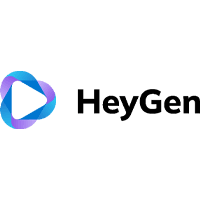
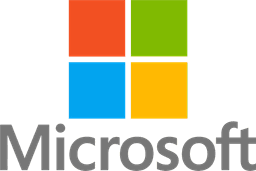
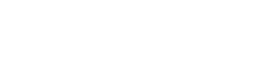
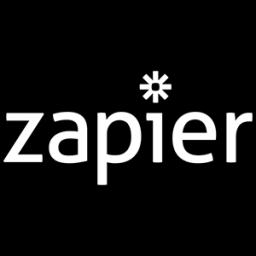
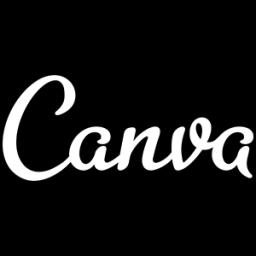
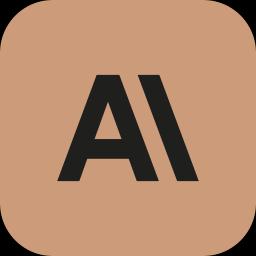
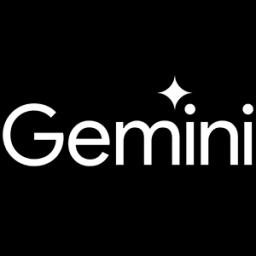
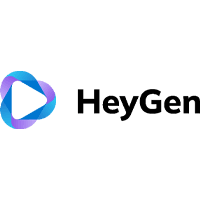
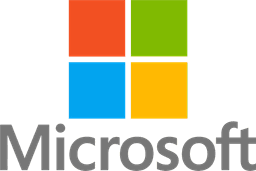
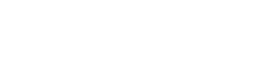
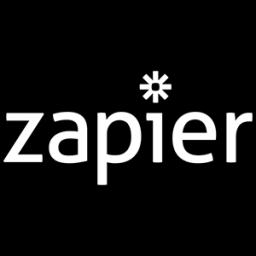
Public Reactions and Ethical Considerations
The introduction of the concept of 'peak data' has stirred significant public discourse and scrutiny across various sectors. Recognizing the finite quantity of easily accessible, high-quality training data has led to diverse reactions. Many draw parallels between this scarcity and the depletion of natural resources like fossil fuels, highlighting a growing awareness of the limitations intrinsic to current AI development methodologies. Some sectors of the public remain optimistic, suggesting that untapped resources and innovative methods, such as synthetic data creation, may offer a viable path forward in addressing these limitations.
On the ethical front, the discussions have grown more intense, particularly in light of competitive practices within the AI industry. For instance, concerns have been raised about the use of AI outputs from one company, such as OpenAI, as training data by competitors like DeepSeek. This raises critical questions about intellectual property rights and the need for proper attribution and licensing in AI model development. These discussions are not just limited to the ethical use of data, but also to the transparency and fairness of AI advancements, which remain crucial considerations as we navigate this technological frontier.
The concept of 'test-time compute' has emerged as a promising solution in the face of peak data limitations, touted by significant figures in the industry including Satya Nadella, the Microsoft CEO. While this approach, which allows AI models to perform complex problem-solving through incremental steps, holds substantial potential, public opinion is divided. There remains skepticism about its applicability to tasks that require abstract reasoning or creativity, as these do not have easily verifiable outputs. Therefore, while some industry leaders remain optimistic about its capacity to sustain AI growth, broader acceptance will depend on demonstrable successes across a range of applications.
As these debates continue, the integration of ethical considerations into AI's advancement becomes increasingly imperative. The possibility of utilizing outputs from one AI system to train another draws attention to the necessity for clear ethical guidelines and potentially new regulatory frameworks. These must address not just the technical and operational aspects, but also the competitive dynamics and innovation-driven culture within the AI sector. The balance between fostering innovation and ensuring responsible, ethical AI development is a nuanced challenge that will undoubtedly shape future discourse and policy-making.
Future Implications of Peak Data and Test-Time Compute
The concept of peak data presents a formidable challenge to the AI industry, signaling a saturation point where the abundance of high-quality training data, once freely available on the internet, has now diminished significantly. This challenge threatens to slow the relentless pace of AI advancements, as AI models heavily depend on vast datasets to enhance their learning and output capabilities. As the pool of available data becomes shallow, researchers and developers are compelled to explore innovative training methods to sustain progress.
One promising solution on the horizon is the strategic use of 'test-time compute,' a method gaining traction notably within Google DeepMind's research circles. Test-time compute involves deconstructing complex queries into smaller, more digestible prompts. This granular approach allows AI models to process information in a step-by-step manner, enhancing their ability to reason through challenges meticulously. By breaking down tasks into simpler parts, AI can generate higher-quality outputs. These outputs do not just solve immediate queries but also provide fresh training data, ushering in a potential self-improvement loop that could invigorate AI development even in data-scarce environments.
Learn to use AI like a Pro
Get the latest AI workflows to boost your productivity and business performance, delivered weekly by expert consultants. Enjoy step-by-step guides, weekly Q&A sessions, and full access to our AI workflow archive.
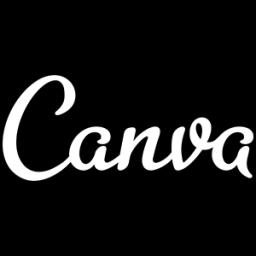
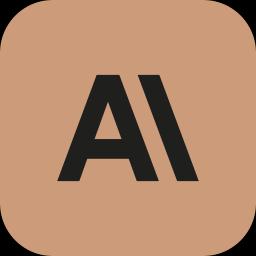
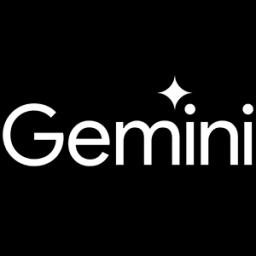
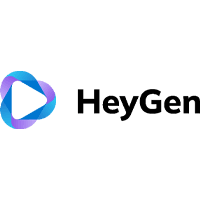
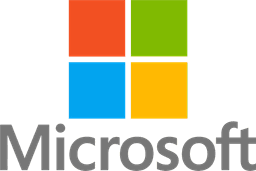
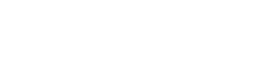
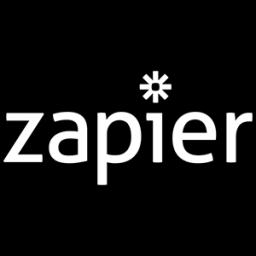
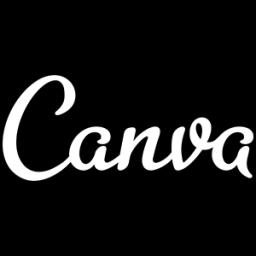
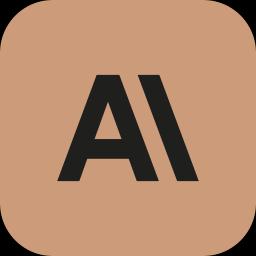
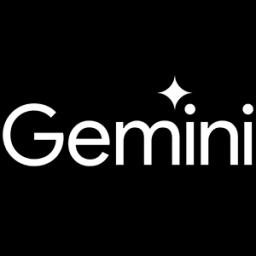
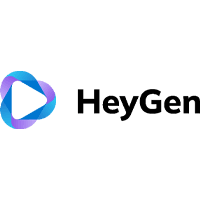
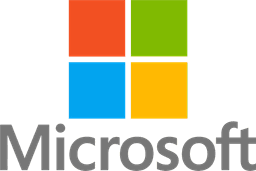
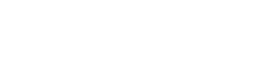
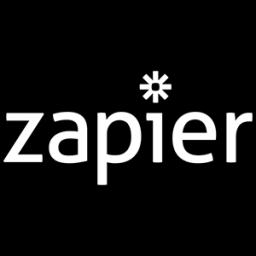
However, test-time compute is not without its limitations and challenges. Its effectiveness is largely contingent on the nature of tasks it is employed upon. For tasks with clear, objective answers, such as mathematical computations, test-time compute has demonstrated significant promise. Yet, for subjective or complex problems without definitive answers, like creative writing or nuanced decision-making, the applicability of test-time compute remains uncertain. This uncertainty underscores the necessity for further research to expand its generalizability and effectiveness across diverse AI applications.
The industry is actively engaged in addressing these challenges, with major players such as OpenAI and Microsoft expressing optimism in test-time compute's potential. OpenAI has already incorporated this method in its model, dubbed as 'o1', aiming to improve performance through multi-step reasoning without the sole reliance on massive pre-training datasets. Similarly, Microsoft views test-time compute as another 'scaling law', a revolutionary metric in AI advancement, capable of enhancing model capabilities through iterative sample testing during inference. Nevertheless, the competitive spirit of the AI industry also brings to light ethical and attribution debates, particularly as new players like DeepSeek leverage outputs from more established models for training purposes.
Looking forward, the ripple effects of the peak data challenge and the adoption of test-time compute are poised to extend across economic, social, political, and technological spectrums. Economically, a prolonged slowdown in AI advancement might affect market growth and investment trajectories, with companies potentially facing increased costs for acquiring or generating high-quality data. Socially, peak data could decelerate AI-driven changes, affording societies more time for adaptation, while highlighting the importance of data privacy and ownership. Politically, countries might intensify their pursuit of AI dominance, leading to debates on data protectionism and the ethics of AI-generated content. Technologically, expect an accelerated push towards developing AI systems that perform efficiently within the constraints of limited data, pioneering research into alternative training methods and crafting AI architectures that prioritize energy sustainability.
Conclusion: Navigating the AI Data Landscape
As the landscape of AI continues to evolve, the concept of 'peak data' emerges as a critical juncture. This term, describing the saturation point of available high-quality training data from online sources, signifies a potential slowdown in the previously rapid advancements of artificial intelligence models. The depletion of these readily available data reserves challenges the industry to innovate beyond traditional massive data acquisition strategies, prompting a significant shift in focus towards optimizing the quality of data and the efficiency of AI training methodologies.
In response to these challenges, the novel approach of 'test-time compute' emerges as a promising solution. Pioneered by researchers at institutions like Google DeepMind, this method involves deconstructing complex queries into simpler, manageable components. This allows AI systems to process and solve problems incrementally, enhancing their reasoning capabilities and generating higher-quality outputs. These outputs, in turn, serve as new, rich datasets, fostering an iterative cycle of self-improvement that could mitigate the data scarcity issue.
Industry leaders acknowledge the dual-edged nature of this innovation. Prominent figures such as OpenAI's Ilya Sutskever and Microsoft's Satya Nadella highlight both the urgency of addressing peak data limitations and the potential of test-time compute to redefine AI scaling. However, concerns remain about the generalizability of these techniques, particularly for tasks lacking clear, verifiable outcomes. This underscores a fundamental need for further research and experimentation to fully harness the capabilities of test-time compute beyond its current applications.
Learn to use AI like a Pro
Get the latest AI workflows to boost your productivity and business performance, delivered weekly by expert consultants. Enjoy step-by-step guides, weekly Q&A sessions, and full access to our AI workflow archive.
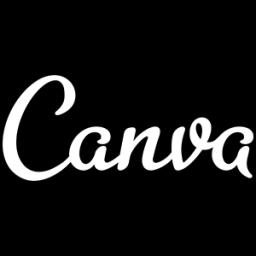
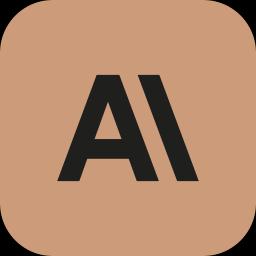
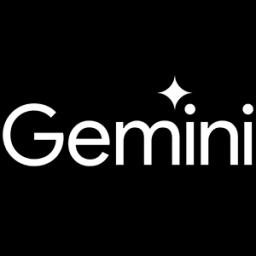
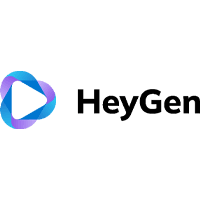
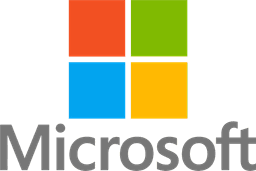
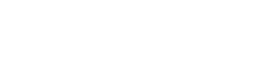
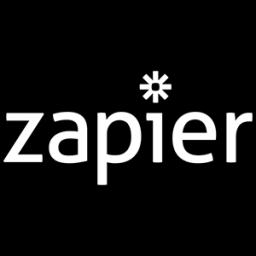
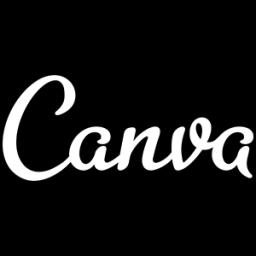
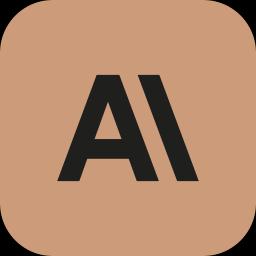
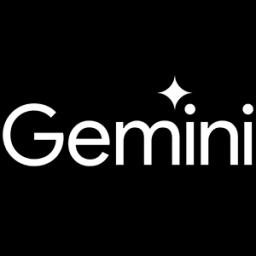
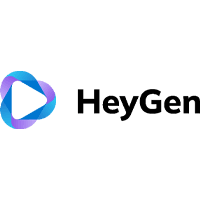
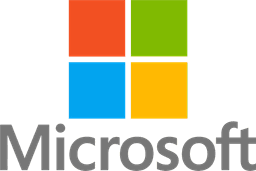
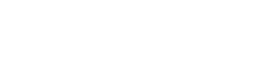
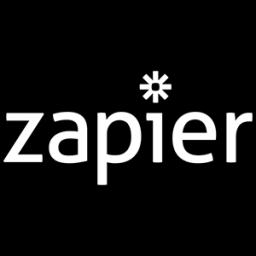
Public sentiment reflects a mixture of awareness and anticipation. There is an increasing recognition of data scarcity, akin to discussions on resource depletion in other industries. While some express cautious optimism about alternative data sources or synthetic data generation, skepticism persists regarding the broader applicability of test-time compute. This diversity of opinions aligns with the ongoing debates about AI's ethical considerations, data rights, and the competitive dynamics within the technological domain.
Looking forward, the implications of navigating this new AI data landscape are profound. Economically, the scarcity of high-quality data may alter investment patterns and drive up development costs, while simultaneously opening new markets for synthetic data solutions. Socially, this could slow the pace of AI-driven change, allowing societies more time to adapt. Politically, data protectionism and regulatory frameworks are anticipated to evolve, addressing the ethical and competitive challenges posed by AI's data needs. Technologically, there is likely to be an acceleration in research focused on enhancing AI's ability to learn from limited data, heralding a future of more sustainable and efficient machine learning models.