AI's Future 2025: Big Leaps Ahead
AI in 2025: More Power, Bigger Challenges, Say Experts at Anthropic
Last updated:

Edited By
Mackenzie Ferguson
AI Tools Researcher & Implementation Consultant
Anthropic co-founder Jack Clark predicts dramatic advancements in AI by 2025. With innovations like OpenAI's o3 modeling 'thinking out loud', there's promise of greater adaptability in AI systems. However, these advancements bring cost concerns, highlighted by Anthropic delaying its Opus 3.5 model. Dive into the balance of innovation and cost, and what the future holds for AI development.
Introduction: The Dawn of a New Era in AI
The rapid advancements in artificial intelligence (AI) mark the beginning of an exciting new era, reshaping how we perceive and interact with technology. As 2025 approaches, the AI sector is expected to witness monumental breakthroughs that could redefine boundaries in numerous fields. This introduction aims to outline the anticipated changes and contexts for these transformations, delving into how they may influence various sectors including economics, social interaction, and regulatory landscapes.
Artificial intelligence has continually evolved, with significant milestones being achieved in shorter timeframes than previously expected. The pace of AI development is not only swift but is also integrating deeper into economic and social fabrics, impacting how businesses operate and individuals interact daily. As such, there is an impending shift expected around 2025, driven by both technological advancement and practical implementations in the AI sector.
Learn to use AI like a Pro
Get the latest AI workflows to boost your productivity and business performance, delivered weekly by expert consultants. Enjoy step-by-step guides, weekly Q&A sessions, and full access to our AI workflow archive.
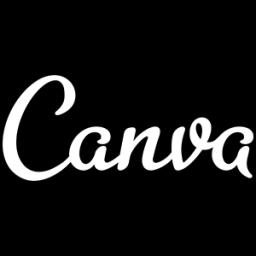
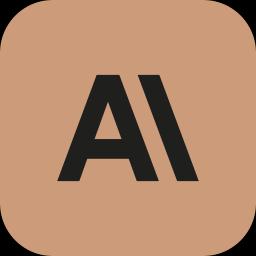
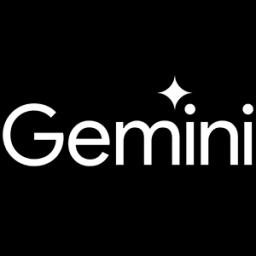
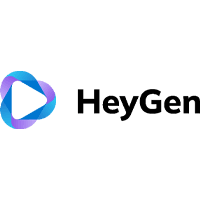
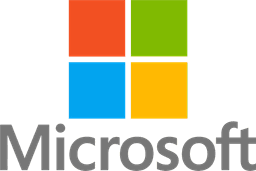
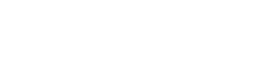
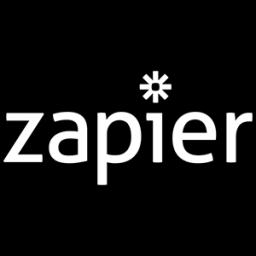
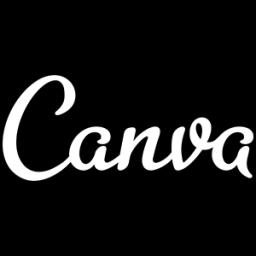
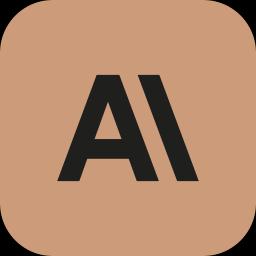
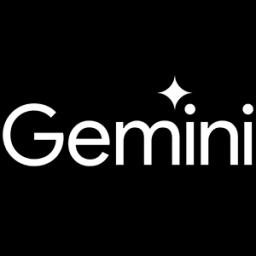
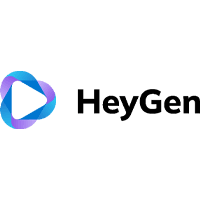
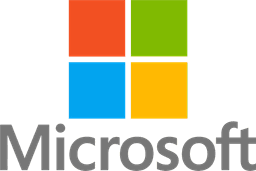
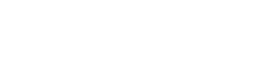
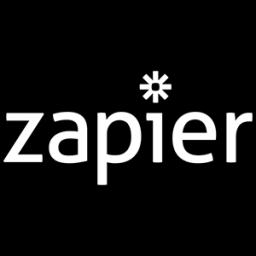
Jack Clark, co-founder of Anthropic, predicts a dramatic escalation in AI capabilities by 2025. This foresight isn't without basis; current projects such as OpenAI's new model, which emphasizes 'thinking out loud', showcase the field's ongoing advancements. These innovations signify not merely incremental changes but a fundamental shift in AI's functional and economic paradigms.
One noteworthy discussion point in modern AI advancement is the 'test-time compute scaling'. This innovative approach allows AI systems to dynamically allocate resources based on the complexity of tasks at hand, presenting both opportunities and challenges, particularly in cost predictability. Anthropic's delay of their Opus 3.5 model indicates just how these cost factors are influencing critical decisions in AI developments.
As AI continues to grow, the industry must grapple with the financial implications of enhanced computational power requirements. This financial bottleneck has prompted tech firms to rethink strategies around cost management and operational efficiency, with implications extending to market leadership and technological competition. The introduction of cost-effective AI chips like Google's TPU v5e and NVIDIA's H200 exemplifies efforts to counteract these financial strains, illustrating a proactive approach to evolving technological needs.
Moreover, these shifts bring about pressing regulatory and ethical considerations. The European Union's passage of the AI Act exemplifies the growing concern over AI's role in society and introduces new regulations to ensure responsible AI deployment. Such legislative measures may profoundly impact how AI technology is scaled and utilized across different sectors.
Learn to use AI like a Pro
Get the latest AI workflows to boost your productivity and business performance, delivered weekly by expert consultants. Enjoy step-by-step guides, weekly Q&A sessions, and full access to our AI workflow archive.
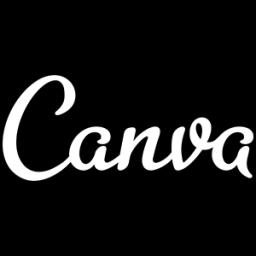
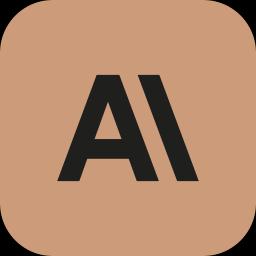
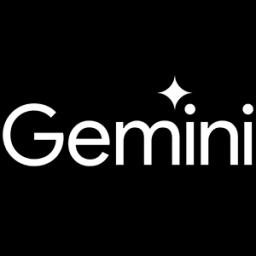
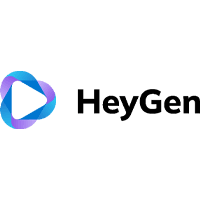
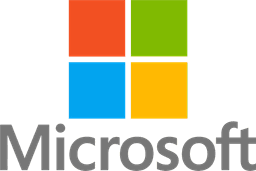
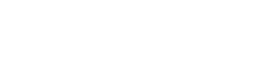
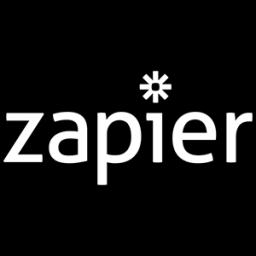
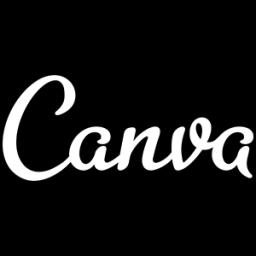
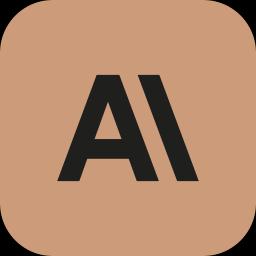
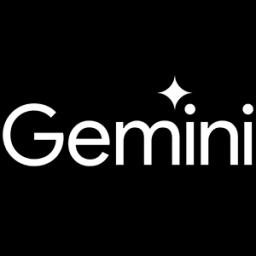
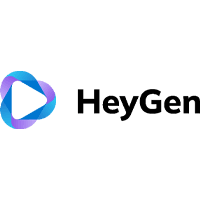
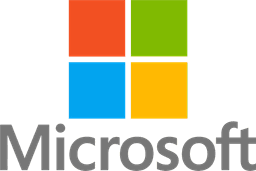
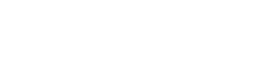
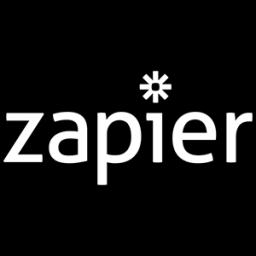
Looking ahead, the future of AI seems poised for transformative shifts not only in technological capabilities but also in societal impacts. The move towards more energy-efficient and specialized AI systems will likely redefine interactions with AI, encouraging the development of collaborative AI agents capable of offering advanced solutions to today's problems. Amid these changes, the balance between leveraging AI's capabilities and maintaining ethical governance will be paramount.
Anthropic's Vision for 2025: Bold Predictions and Innovations
In today's rapidly evolving landscape of artificial intelligence, Anthropic, a cutting-edge AI research company, envisions groundbreaking advancements and innovations by the year 2025. As detailed in a recent article, Jack Clark, co-founder of Anthropic, predicts that the progress in AI will be considerably more dramatic, with innovations such as the "thinking out loud" model, 'o3', and advanced compute scaling methodologies. These advancements promise to redefine computational efficiency and application scope across various domains, paving the path for potentially unprecedented capabilities in AI systems.
According to Jack Clark of Anthropic, the thinking out loud model, known as 'o3', exemplifies the future of AI modules which dynamically adjust resource allocations based on the complexity of tasks. Different from traditional fixed-resource models, the o3 model employs reinforcement learning alongside runtime computational flexibility, optimizing itself by using additional computing power adaptively. This innovative approach enables AI models to scale computationally in real-time, a shift from static resource assignments, allowing them to handle complex tasks more efficiently and effectively.
A notable aspect of the advancements predicted by Anthropic involves 'test-time compute scaling', a concept allowing for dynamic resource allocation in AI systems. This breakthrough proposes a model where computational investment correlates with task complexity, enhancing the efficiency and potential capabilities of AI. However, this also brings forth challenges in scalability related to costs. For instance, the operational costs of implementing the o3 model significantly exceed those of earlier iterations and competitors, complicating budget predictions and posing financial hurdles for widespread implementation.
Anthropic's exploration of new AI frontiers, such as the Opus 3.5 model, reflects both potential and hurdles in today's technological environment. Despite significant performance enhancements, the Opus 3.5 project witnessed postponements due to prohibitive costs, highlighting the economic reality complicating AI innovation. Anthropic's decision to delay this model reflects a cautious approach towards managing resource-intensive processes in AI development, ensuring economic viability amidst rapidly expanding compute demands.
Parallel developments in the AI field offer additional context to Anthropic's vision. Google's introduction of its TPU v5e chip, for example, aims to mitigate the growing costs of AI computations by offering a more cost-effective training and inference solution, demonstrating industry-wide recognition of the need for efficient resource management in AI. Similarly, NVIDIA's release of the H200 GPU, with its enhanced performance capabilities, underscores the broader trend of developing hardware solutions to meet the growing demands of AI scaling.
Learn to use AI like a Pro
Get the latest AI workflows to boost your productivity and business performance, delivered weekly by expert consultants. Enjoy step-by-step guides, weekly Q&A sessions, and full access to our AI workflow archive.
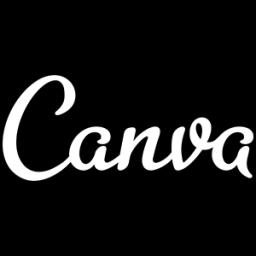
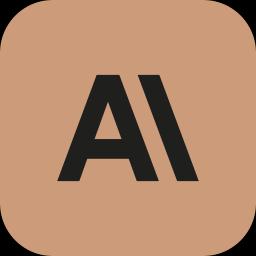
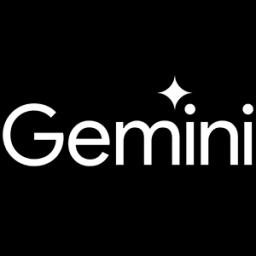
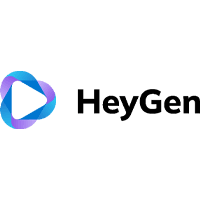
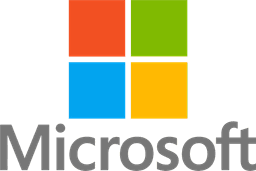
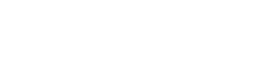
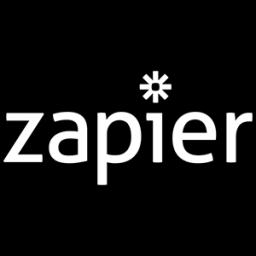
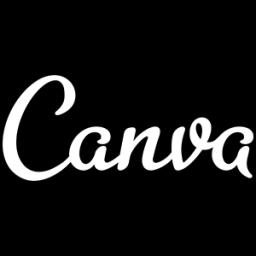
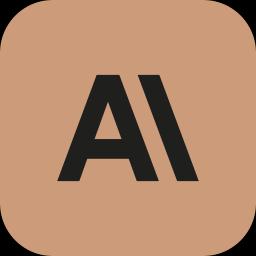
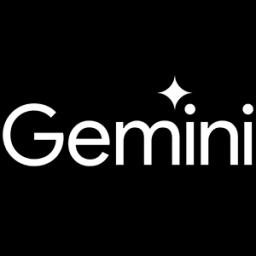
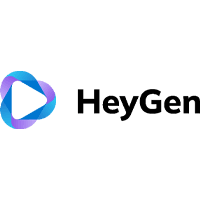
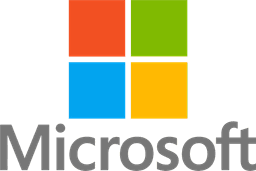
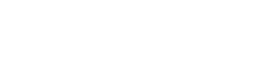
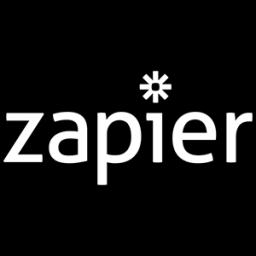
Amidst the technological advancements, the industry also observes varying expert opinions on the implications of AI development pace and computational demands. IBM experts stress the significance of compute scaling costs, urging organizations to understand these expenses deeply. Stanford academics predict potential shifts towards collaborative AI systems and the potential costs involved, while Pew Research Center assessments weigh both potential benefits and societal risks of AI expansion. This diverse expert landscape offers valuable insights into managing the economic, ethical, and operational impacts of AI advancements.
Understanding OpenAI's o3 Model: "Thinking Out Loud"
The implementation of OpenAI's o3 model, dubbed "Thinking Out Loud," marks a significant advancement in artificial intelligence (AI) technology. This model is designed to utilize reinforcement learning mechanisms and leverage additional computing power during runtime operations. By facilitating dynamic resource allocation based on task complexity, the o3 model adapts its resource usage, unlike traditional AI models that stick to predefined computational resources. This adaptability not only improves processing efficiency but also reflects a considerable leap towards more sophisticated AI behaviors and functionalities. Such capabilities set the stage for future AI systems that require innovative resource management strategies.
Test-Time Compute Scaling: A New Approach to AI Resource Allocation
Artificial intelligence's rapid progress is not just reshaping industries but also altering the landscape of computational resource allocation. Test-time compute scaling emerges as a pivotal innovation, allowing AI models to dynamically adjust their resource consumption based on the complexity of tasks. This flexibility can significantly optimize performance but introduces new challenges in predicting and managing operational costs.
The concept of test-time compute scaling involves using reinforcement learning and additional compute during AI operations. This enables models like OpenAI's o3 to 'think out loud,' meaning they can adjust their processing power as needed during specific tasks. Unlike traditional models which are pre-set with fixed resources, this approach ensures more efficient resource utilization, adapting to the diverse demands of various tasks.
However, the adaptability of test-time compute scaling does not come without cost implications. As highlighted by the Anthropic co-founder, this approach requires substantial computational power, exceeding previous models and making budgeting more complex. The advanced version of o3, for instance, uses 170 times more computing power than its basic version, presenting challenges in cost forecasting and financial planning for AI-driven projects.
Despite these challenges, the potential benefits of this approach in AI are immense. It could lead to more personalized and effective AI interactions, fulfilling intricate computational needs on demand. However, it also signifies a shift towards necessitating even greater investments in cloud infrastructure and hardware innovation to support such adaptable AI systems effectively.
Learn to use AI like a Pro
Get the latest AI workflows to boost your productivity and business performance, delivered weekly by expert consultants. Enjoy step-by-step guides, weekly Q&A sessions, and full access to our AI workflow archive.
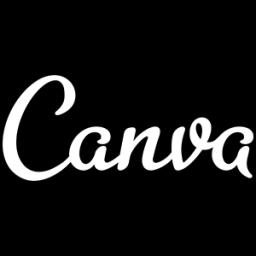
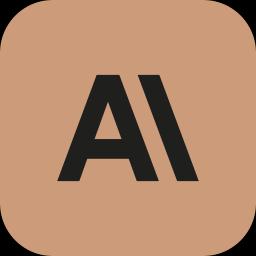
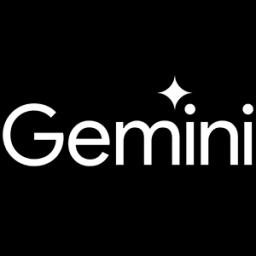
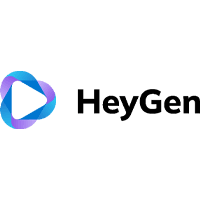
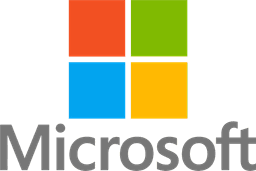
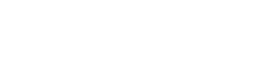
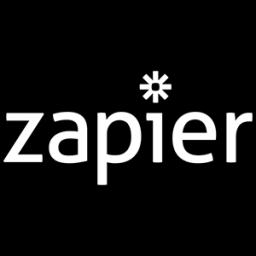
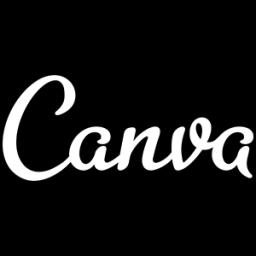
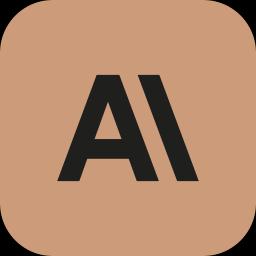
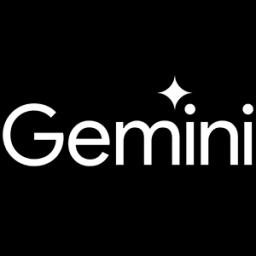
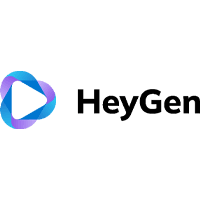
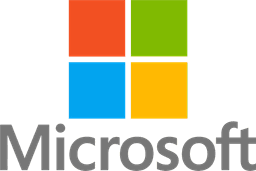
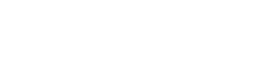
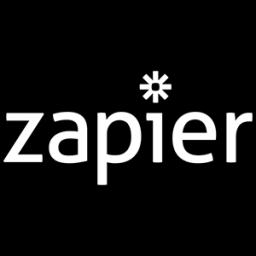
As the industry grapples with these advancements, stakeholders must consider both the technological benefits and the economic implications of scaling AI compute capacity. Future AI systems will not only need to be powerful but also cost-efficient, balancing the need for advanced capability with practical economic considerations. This balance will become crucial as AI becomes more integrated into everyday applications, influencing competitive strategies of major tech companies.
The Cost of Progress: Economic Implications of Increased Computing Power
In the rapidly evolving landscape of artificial intelligence (AI), the economic implications of increased computing power are becoming a focal point for industry leaders and researchers alike. As AI systems become more complex, the computational resources required to power these advancements exponentially increase, leading to significant financial considerations. The recent predictions for AI progress in 2025, as discussed by Anthropic's Jack Clark, underscore the urgency for stakeholders to re-evaluate cost structures and investment strategies. The introduction of dynamic resource allocation methods, such as 'test-time compute scaling,' adds a layer of complexity to budgeting, as it challenges traditional fixed-resource frameworks.
A critical aspect of this discussion is the groundbreaking capabilities demonstrated by models like OpenAI's o3, which introduces a 'thinking out loud' methodology. This approach allows for a more fluid and adaptable use of computational resources, thereby optimizing performance based on task complexity. However, the downside of this flexibility is the substantial increase in costs associated with running these models. Estimates indicate that the o3 model's advanced version requires 170 times more computing power than its basic iteration, elevating cost considerations to a strategic level for AI developers.
Anthropic's cautious approach, as evidenced by their Opus 3.5 model delay, reflects the broader industry's struggle to balance technological advancements with economic sustainability. The high operating costs, despite the potential performance gains, have prompted Anthropic to reassess their release timelines and competitive strategies. Interestingly, while Opus 3.5 has contributed to advancements like Sonnet 3.5, Anthropic has yet to challenge the market dominance of OpenAI's and Google's latest AI innovations.
This increased computational demand is reshaping the AI development landscape, potentially leading to market consolidation where only the largest players can afford the expense. Smaller companies might struggle to keep up, resulting in reduced diversity and innovation across the industry. To address these challenges, tech companies are investing in more cost-effective AI hardware solutions, as seen with Google's TPU v5e and NVIDIA's H200 GPU. These investments aim to curb the escalating costs of AI training and inference, making advanced AI more accessible.
Beyond the economic factors, the advancements in AI computing have far-reaching implications across social, political, and technological domains. AI's growing capabilities could lead to more personalized and sophisticated digital experiences, raising concerns about privacy and data security. Additionally, the potential for increased AI-generated misinformation and scams needs addressing. On a regulatory front, there will likely be intensified calls for comprehensive AI regulations, similar to the EU's AI Act, to ensure responsible AI deployment.
Learn to use AI like a Pro
Get the latest AI workflows to boost your productivity and business performance, delivered weekly by expert consultants. Enjoy step-by-step guides, weekly Q&A sessions, and full access to our AI workflow archive.
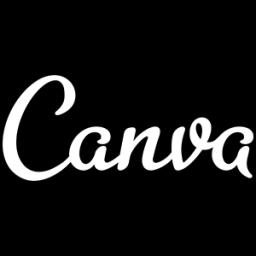
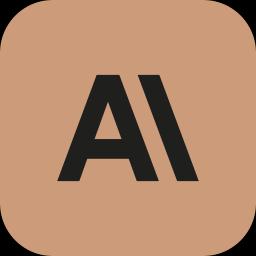
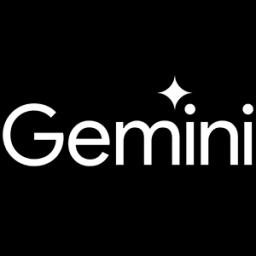
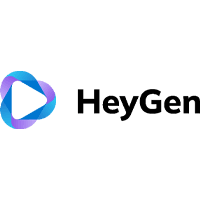
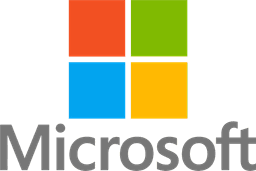
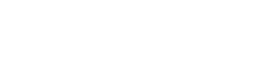
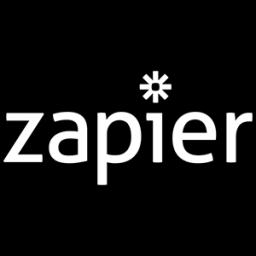
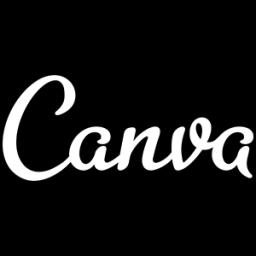
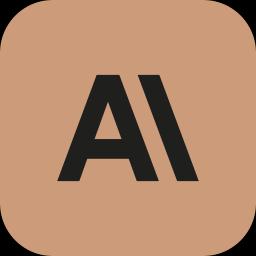
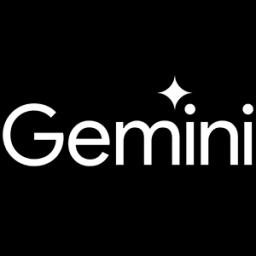
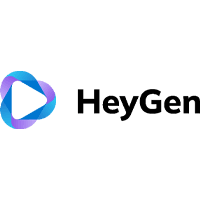
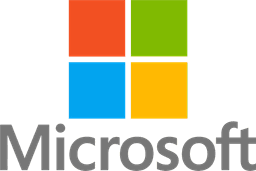
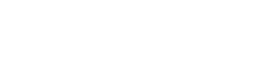
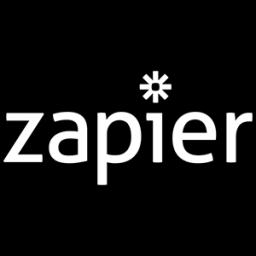
Technological advancements are also on the horizon, with a shift towards collaborative AI systems envisioned. These would utilize multiple specialized agents to achieve greater intelligence and efficiency, albeit at potentially higher costs. Quantum computing represents another exciting frontier, as IBM's recent breakthroughs suggest alternative pathways for AI scaling. As these developments unfold, the race to create more energy-efficient AI hardware becomes crucial to sustaining long-term growth while balancing the economic implications of enhanced computing power.
Delays and Decisions: Anthropic's Opus 3.5 Model
In the rapidly evolving landscape of artificial intelligence, Anthropic's Opus 3.5 model stands as a testament to both groundbreaking innovation and the complex decision-making processes involved in AI development. In recent discussions, Anthropic co-founder Jack Clark highlighted the significant progress anticipated in the field by 2025, underscoring the transformative potential of advanced AI systems like OpenAI's o3 model. The o3 model is particularly noted for its 'thinking out loud' capabilities, which utilize reinforcement learning to dynamically adjust computing resources in real-time, a departure from traditional models with static resource allocation. This capability, known as 'test-time compute scaling,' represents a pivotal shift in how computing power is harnessed and optimized during AI operations.
Although these advancements promise enhanced AI proficiency, they also bring forth significant cost challenges. The computational power required by more advanced AI systems, such as the o3 version, which demands 170 times more computing resources than its predecessors, poses substantial budgetary and cost prediction difficulties. These escalating costs have led to strategic delays in the rollout of Anthropic's Opus 3.5 model, despite its integration contributing to the advancement of models like Sonnet 3.5.
Observing these trends, it's clear that the future of AI development heavily leans on innovative computing solutions that can balance performance with affordability. The introduction of specialized chips, like Google's TPU v5e and NVIDIA's H200 GPU, reflects the industry's response to the burgeoning demand for efficient AI scaling. These technological innovations are critical in addressing the cost-related pressures faced by AI developers. Meanwhile, efforts by companies like Meta to develop in-house AI hardware, such as the MTIA chip, showcase the strategic moves tech giants are making to mitigate dependency on external suppliers and enhance operational efficiency.
The implications of such developments are multifaceted, spanning economic, social, and political realms. Economically, the rising costs of AI development could lead to industry consolidation, with major tech entities having the upper hand due to expansive resources. Socially, the potential for sophisticated AI models to disrupt job markets and foster new industries—like AI ethics and prompt engineering—indicates a shifting workforce landscape. Politically, technological advancements necessitate robust regulatory frameworks to ensure ethical usage, as illustrated by initiatives like the EU's AI Act.
Looking forward, the trajectory for AI technology continues to advance towards more collaborative and complex systems. Experts from Stanford predict a future where AI agents work in tandem, requiring nuanced and potentially costly computing frameworks. Additionally, the exploration of quantum computing for AI tasks poses opportunities for significant breakthroughs, potentially reshaping the current paradigms of AI capacities. Therefore, as Anthropic and other AI pioneers navigate these challenges, the decisions made today will undoubtedly influence the AI landscape of tomorrow.
Learn to use AI like a Pro
Get the latest AI workflows to boost your productivity and business performance, delivered weekly by expert consultants. Enjoy step-by-step guides, weekly Q&A sessions, and full access to our AI workflow archive.
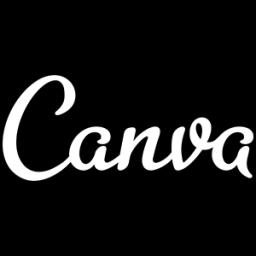
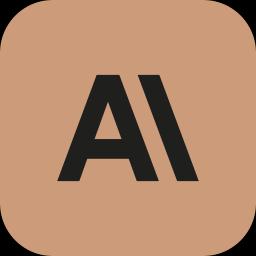
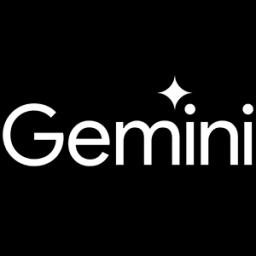
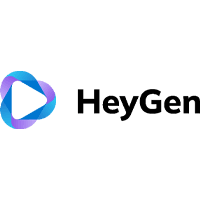
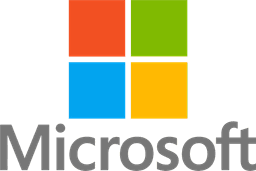
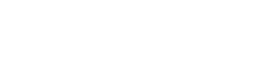
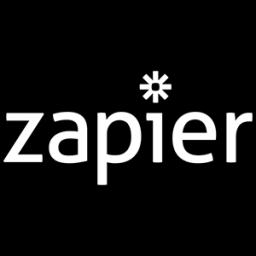
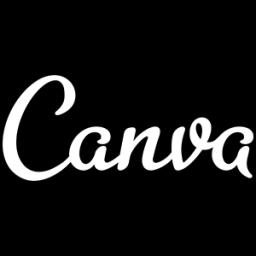
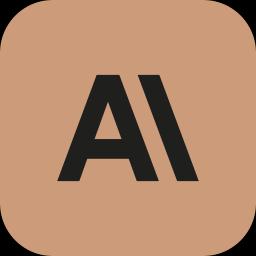
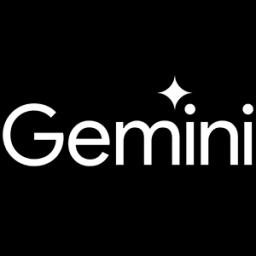
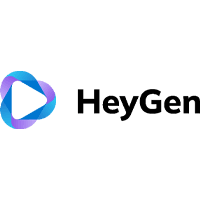
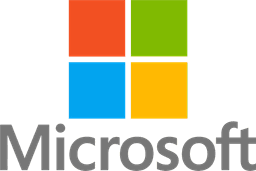
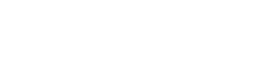
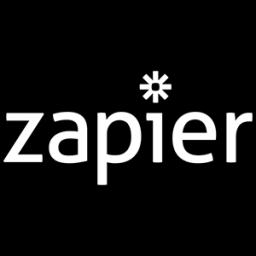
Expert Insights: The Future of AI and Compute Scaling
Artificial Intelligence (AI) stands at the brink of a transformative shift as it heads into the year 2025. Spearheading this evolution, tech luminary and Anthropic’s co-founder, Jack Clark, anticipates advancements that could dwarf past progress. This profound change is not just about speed or volume of information, but about fundamentally redefining how AI 'thinks' and allocates its computational resources dynamically to harness the future’s full potential. At the heart of this paradigm is OpenAI's o3 model, whose novel capability to 'think out loud' marks a departure from traditional models by adjusting its resource usage in real-time according to task complexity.
A major stride in AI development is the method of 'test-time compute scaling', which is revolutionizing the landscape by enabling AI systems to allocate computational resources dynamically, based on the complexities of tasks at hand. This innovation seeks to optimize performance while addressing the ever-looming challenge of cost efficiency in computational scaling. However, the surge in computational demand, reflected by the advanced o3 version's 170-fold increase in power requirement over its basic counterpart, amplifies the unpredictability in cost projections. Traditional budgeting methods may falter in the face of such fluid computational needs, often pushing developers like Anthropic to reconsider the financial viability of subsequent model releases like Opus 3.5 indefinitely. This unpredictability in scaling costs highlights a new frontier where economic considerations are as transformative as technological innovations themselves.
Regulatory Challenges and Developments in AI Technology
The rapidly evolving field of AI technology faces significant regulatory challenges as governments and organizations strive to keep pace with advancements. The EU's AI Act, recently passed, exemplifies regulatory attempts to set boundaries and standards for AI deployment. This legislative initiative aims to ensure AI's ethical use while fostering innovation, but it also presents compliance challenges for tech companies. Moreover, regulatory bodies must address the balance between encouraging AI development and protecting public interests.
One of the major challenges in regulating AI technology is the unpredictable pace of advancements. Innovations such as OpenAI's o3 model, with its 'thinking out loud' capabilities, highlight the complexities of dynamic compute scaling. This presents both opportunities and regulatory hurdles, as traditional oversight mechanisms may struggle to adapt swiftly enough. Additionally, cost implications due to increased computing power strain budgets, further complicating regulatory policies intended to oversee equitable technology distribution.
Developments like IBM's exploration of quantum computing for AI tasks offer new avenues for growth but also require rethinking regulatory approaches. Quantum computing's potential to revolutionize AI paradigms could outpace current legal frameworks, necessitating swift adaptation and international cooperation on standard-setting. Overall, the interconnected challenges of regulatory adaptation and technological advancement require a collaborative approach among governments, industry leaders, and researchers to develop comprehensive policies that address ethical, economic, and social implications of AI technologies.
The Road Ahead: Economic, Social, and Political Implications of AI Advancements
Artificial Intelligence (AI) advancements are setting the stage for transformative changes across economic, social, and political arenas. With predictions of notable progress in AI by 2025, driven by leaders like Jack Clark from Anthropic, there are both challenges and opportunities on the horizon. As AI models like OpenAI's o3 demonstrate 'thinking out loud' capabilities, the focus shifts to understanding how these methodologies will reshape industries and societies.
Learn to use AI like a Pro
Get the latest AI workflows to boost your productivity and business performance, delivered weekly by expert consultants. Enjoy step-by-step guides, weekly Q&A sessions, and full access to our AI workflow archive.
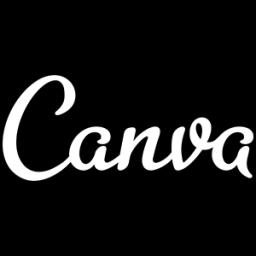
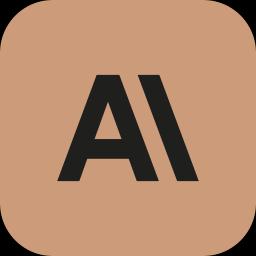
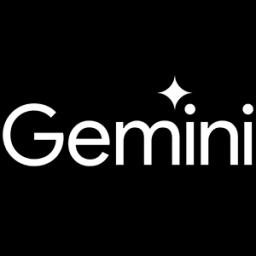
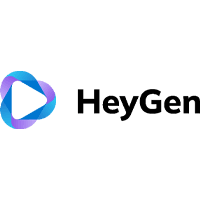
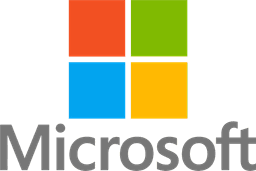
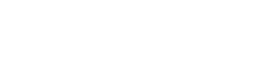
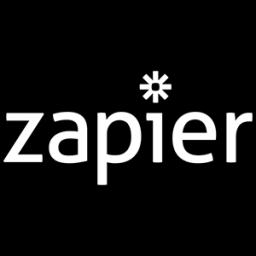
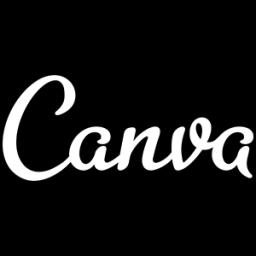
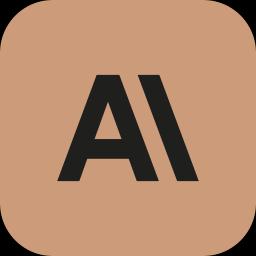
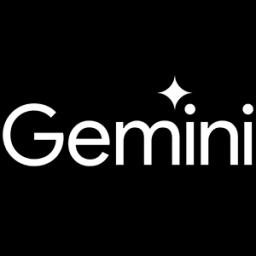
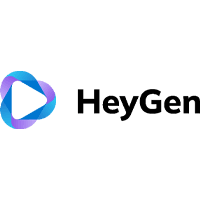
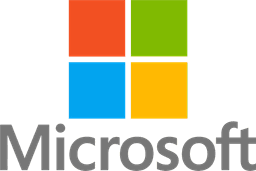
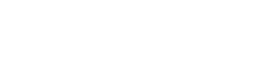
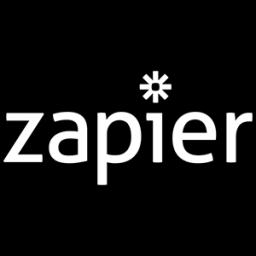
In the realm of economics, the increasing cost of AI development and operation is unavoidable. Dynamic compute scaling, a new frontier in AI technology, offers enhanced adaptability by allowing models to utilize resources more efficiently. This capability makes cost prediction challenging, as exemplified by Anthropic's decision to delay its Opus 3.5 model despite advancements due to high operational costs. The financial landscape will need to adapt, potentially leading to market consolidation with only the tech giants capable of supporting such costly ventures.
Socially, AI's evolution prompts both promising advancements and serious concerns. Enhanced AI systems promise sophisticated and personalized digital experiences, yet they also pose the risk of facilitating misinformation and scams. As AI becomes more pervasive, privacy and data security will be paramount concerns, necessitating robust frameworks to safeguard personal information.
Politically, the ramifications of AI growth may alter global power dynamics and regulatory landscapes. Regions may follow in the EU's footsteps with comprehensive legislation to control AI deployment, impacting how technology firms operate worldwide. Discussions around ethical AI development are likely to intensify, influencing both public discourse and policy-making.
Technologically, the future of AI leans towards collaboration between multiple specialized AI agents, offering a more holistic approach to problem-solving. Innovation in hardware, especially with energy-efficient designs and quantum computing, underlines the potential for revolutionary shifts in AI implementation. As these technologies mature, they will define the future of AI and its place in our world.
Technological Breakthroughs and Future Prospects in AI
The rapid advancements in artificial intelligence (AI) continue to reshape the technological landscape. With AI systems becoming more sophisticated, experts anticipate profound changes by 2025, driven by new methodologies and computing enhancements. Anthropic's co-founder Jack Clark predicts that AI progress in 2025 will be even more remarkable than previously expected.
One such breakthrough is seen in OpenAI's o3 model, which showcases 'thinking out loud' capabilities through reinforcement learning and dynamic resource allocation. Unlike traditional AI models restricted by fixed resource constraints, the o3 model adapts computing power based on task demands. This innovative approach, known as 'test-time compute scaling,' presents both opportunities and challenges, particularly in cost management and prediction.
Learn to use AI like a Pro
Get the latest AI workflows to boost your productivity and business performance, delivered weekly by expert consultants. Enjoy step-by-step guides, weekly Q&A sessions, and full access to our AI workflow archive.
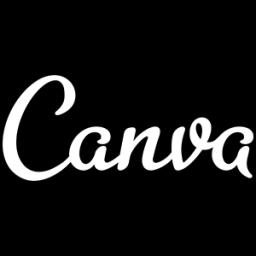
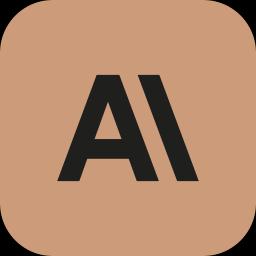
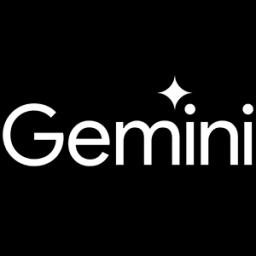
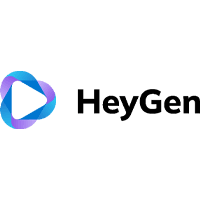
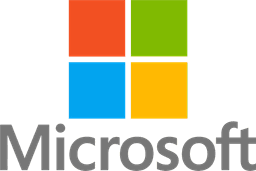
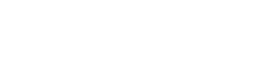
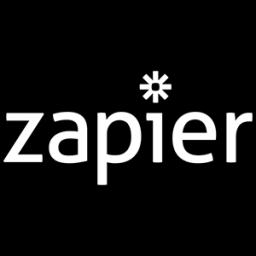
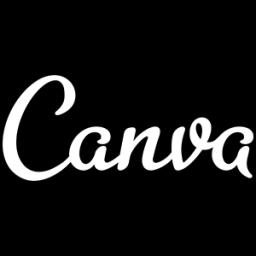
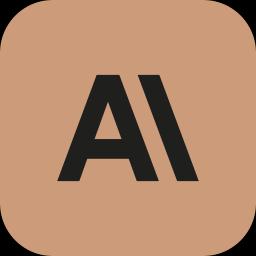
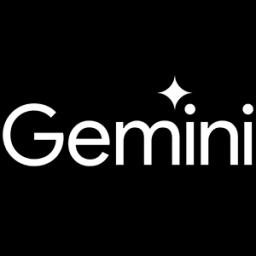
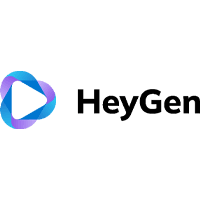
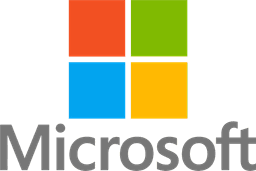
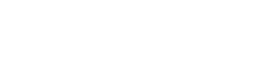
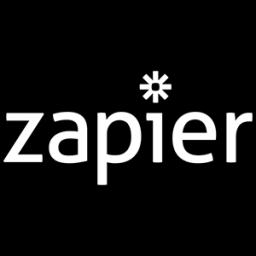
However, the growing need for increased computing power brings with it economic and managerial hurdles. The operating costs for advanced models like o3, which require significantly more resources than their predecessors or competing models like GPT-4o, are formidable, leading to delays in deployments such as Anthropic's Opus 3.5. This contrast highlights the balance between technological advancements and cost-effective scaling strategies.
In response to these challenges, major tech companies are pursuing various hardware innovations aimed at reducing costs and enhancing computing efficiency. Google's launch of its TPU v5e, NVIDIA's introduction of the H200 GPU, and Meta's development of the MTIA chip exemplify efforts to produce more efficient and cost-effective AI chips. These developments are pivotal in addressing the ever-increasing needs of AI power without proportionately escalating costs.
Looking forward, the implications of such rapid AI advancement are vast and multifaceted. Economically, we may see a consolidation in the market where only the largest tech entities can support the financial demands of cutting-edge AI systems. Socially, the evolution of AI suggests both enhanced digital interactions and increased challenges such as privacy concerns and the spread of misinformation. Policymakers and regulators will also face more pressure to address these issues, balancing innovation with ethical considerations as AI technology continues to evolve towards more advanced, possibly quantum computing models.