When AI Meets Healthcare
AI-Powered Blood Tests: Transforming Disease Detection
Last updated:

Edited By
Mackenzie Ferguson
AI Tools Researcher & Implementation Consultant
AI-driven technologies are revolutionizing blood tests, enabling early detection of diseases like ovarian cancer and pneumonia. By utilizing nanotube sensors and microbial DNA databases, these methods surpass traditional techniques, promising faster, more accurate diagnoses.
Introduction to AI in Disease Detection
Artificial Intelligence (AI) continues to revolutionize the field of disease detection, particularly through advancements in blood test methodologies. These innovations, driven by AI, aim to enhance early disease detection, which is crucial in improving patient outcomes. Technologies utilizing AI-powered nanotube sensors and the analysis of microbial DNA databases represent a significant leap in identifying subtle patterns in blood. Such methods offer potential for faster and earlier disease detection compared to traditional approaches.
In the realm of ovarian cancer detection, AI has shown promise by using nanotube sensors that emit fluorescent light upon interacting with blood molecules. This fluorescence can then be analyzed by AI algorithms to recognize unique light patterns, potentially detecting ovarian cancer years before symptoms arise. Similarly, AI's application in pneumonia diagnosis involves analyzing microbial DNA in blood samples, allowing for rapid pathogen identification and targeted antibiotic treatments. These advancements hold the promise of not only earlier detection but also more personalized and effective treatment planning, ultimately improving healthcare outcomes.
Learn to use AI like a Pro
Get the latest AI workflows to boost your productivity and business performance, delivered weekly by expert consultants. Enjoy step-by-step guides, weekly Q&A sessions, and full access to our AI workflow archive.
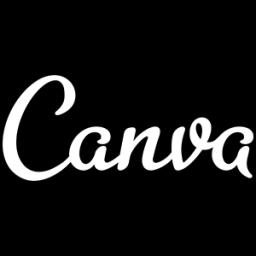
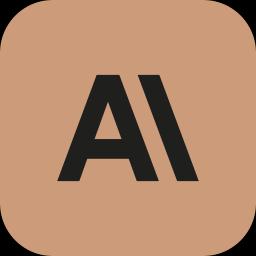
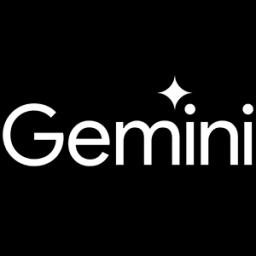
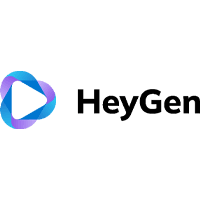
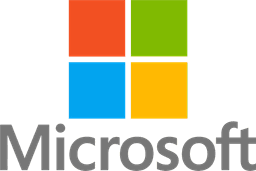
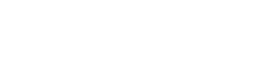
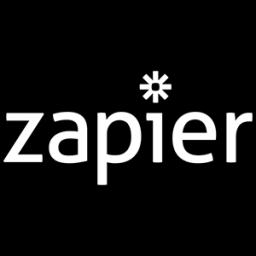
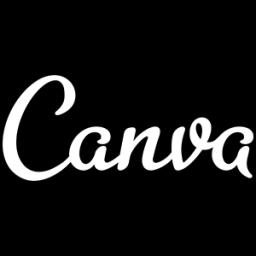
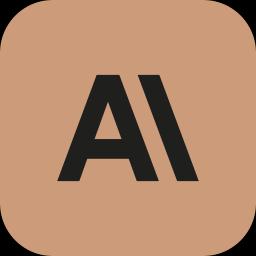
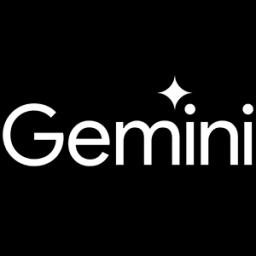
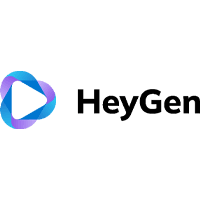
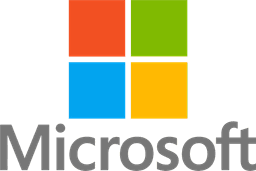
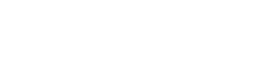
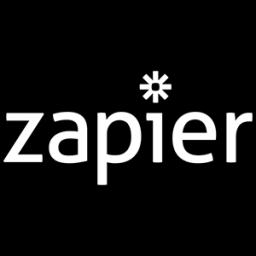
Despite the promise of AI in disease detection, several challenges persist. Key issues include limited data availability for training AI algorithms, especially concerning rare diseases, and the lack of data sharing among researchers and institutions. Another significant challenge is understanding the complex connections between biomarkers and diseases, which can hinder the development of robust AI models. These factors present barriers to fully realizing the potential of AI-powered blood tests in routine clinical settings.
The accuracy and accessibility of AI-powered blood tests continue to be a subject of inquiry and evolving research. While techniques like the nanotube method for ovarian cancer show potential, more comprehensive studies are essential to ascertain precise accuracy rates. Furthermore, the availability of these tests often remains limited to research phases or specific clinical settings. However, some tests, such as those offered by companies like Karius for pneumonia, are becoming more available, leading to discussions on cost comparison with traditional methods. AI-powered tests promise to be significantly cheaper, potentially reducing costs from thousands of dollars to the price of a single test. Despite these opportunities, ethical considerations around the use of AI, including data privacy, algorithmic bias, and equitable access, are paramount in guiding the future implementation of these technologies in healthcare.
Ovarian Cancer Detection with AI
AI technology is bringing a revolution in the field of disease detection and prevention, particularly in the realm of ovarian cancer detection. Traditional methods relied heavily on symptomatic analysis and invasive testing, often leading to late detection when the disease had already progressed significantly. Today, with AI, we have the potential to identify this deadly disease much earlier.
The approach involves using highly sensitive nanotube sensors that interact with the molecules present in the blood. These nanotubes emit specific patterns of fluorescent light, which vary depending on the molecular composition of the sample. AI algorithms have been developed to interpret these unique light patterns, enabling the detection of risk indicators for ovarian cancer potentially years before any physical symptoms manifest.
Learn to use AI like a Pro
Get the latest AI workflows to boost your productivity and business performance, delivered weekly by expert consultants. Enjoy step-by-step guides, weekly Q&A sessions, and full access to our AI workflow archive.
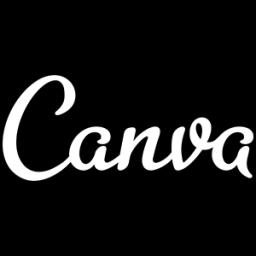
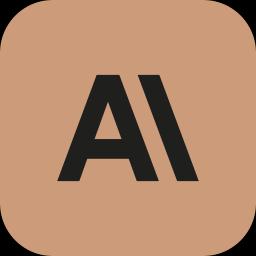
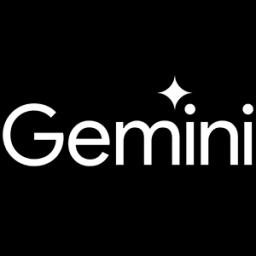
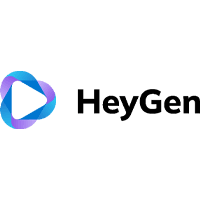
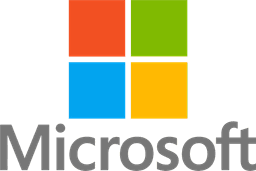
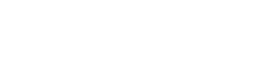
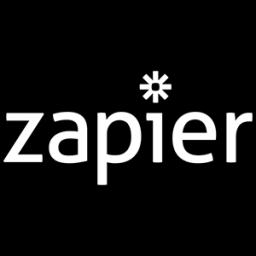
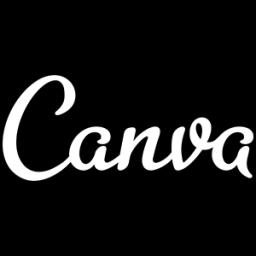
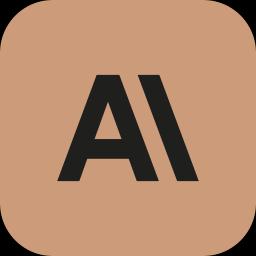
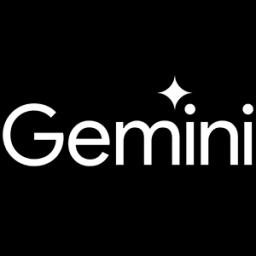
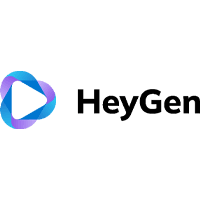
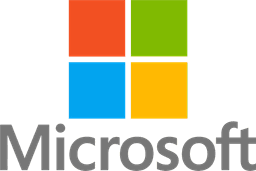
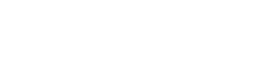
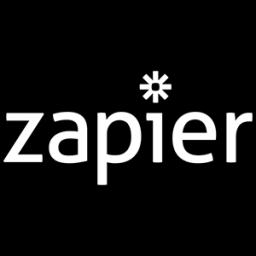
This advancement could be life-changing, as early detection is crucial for improving survival rates and treatment outcomes. If observed early, options for less invasive and more effective treatments increase, giving patients and healthcare providers a significant advantage in combating this disease.
However, challenges remain. Notably, the AI systems require extensive training with large datasets to correctly identify patterns associated with ovarian cancer, a disease that has varied presentations. Data limitations, especially for rare conditions like ovarian cancer, pose a significant barrier. Additionally, without extensive data sharing between research institutions and hospitals, developing universally effective models becomes challenging.
Moreover, ethical considerations such as patient data privacy, algorithmic transparency, and the potential for AI bias need to be addressed. Realizing the benefits of AI in healthcare depends on overcoming these hurdles to ensure equitable access and trustworthy systems.
AI-Enhanced Pneumonia Diagnosis
AI technology is ushering in a new era for pneumonia diagnosis, enabling quicker and more precise identification of pathogens responsible for the infection. Traditional methods of pneumonia diagnosis often involve lengthy processes, including X-rays and blood cultures, which might delay the initiation of targeted treatment. However, AI-powered blood tests are transforming this diagnostic landscape by rapidly analyzing microbial DNA in blood samples. This allows healthcare providers to identify the specific pathogens causing pneumonia much faster, facilitating immediate and appropriate antibiotic therapy which is crucial in combating antibiotic resistance and improving patient outcomes.
One promising development in this field is the Karius test, which is already making strides in clinical settings. By leveraging AI algorithms, this test can pinpoint the pathogen within 24 hours of blood collection, significantly reducing the dependency on traditional diagnostic methods that can take days. This rapid identification not only expedites treatment but also curtails the need for multiple, often costly, diagnostic tests, thereby reducing overall healthcare expenditure on pneumonia.
Learn to use AI like a Pro
Get the latest AI workflows to boost your productivity and business performance, delivered weekly by expert consultants. Enjoy step-by-step guides, weekly Q&A sessions, and full access to our AI workflow archive.
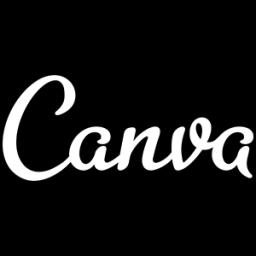
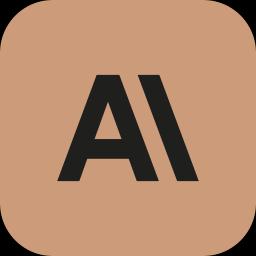
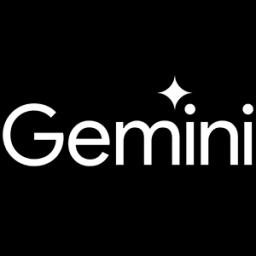
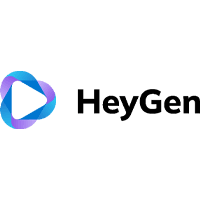
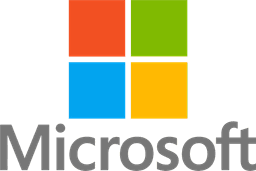
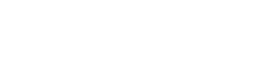
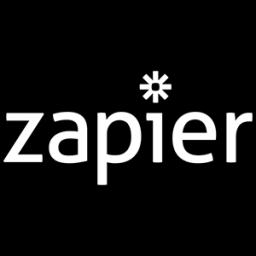
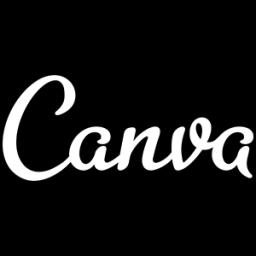
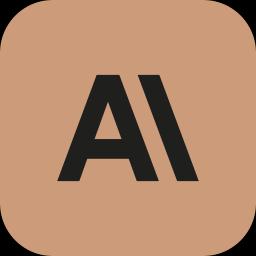
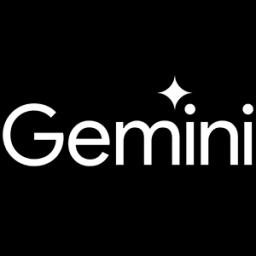
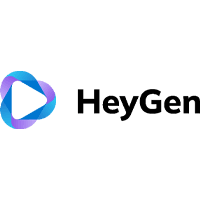
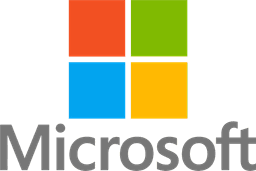
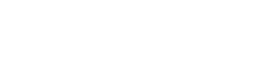
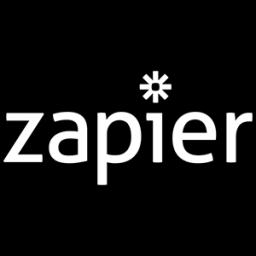
Despite these advancements, several challenges persist in the implementation of AI in pneumonia diagnostics. The effectiveness of AI models largely depends on the quality and quantity of data they are trained on. Limited data access and sharing within the medical research community pose significant hurdles. Moreover, understanding the link between specific microbial patterns and their manifestation as diseases remains an area of ongoing research. Without comprehensive data sets and transparent methodologies, the robustness of AI diagnostic tools might be compromised, potentially affecting their reliability and acceptance in everyday medical practice.
There is a notable enthusiasm in both the medical community and the public regarding the potential of AI-enhanced diagnostics to revolutionize pneumonia treatment. These innovations promise not only to streamline diagnosis but also to ensure that patients receive the most effective treatment sooner, potentially reducing the duration and severity of the illness. However, this optimism is tempered by concerns over data security, privacy, and the inherent "black box" nature of AI technologies that can make it challenging to understand how diagnoses are derived, thereby impacting trust in these technologies.
AI-powered diagnostics for pneumonia, while promising, face several ethical and practical challenges that need to be addressed to maximize their potential benefits. Key among these are the ethical considerations related to patient consent, data bias, and equitable access to these advanced diagnostic technologies. As healthcare systems increasingly adopt AI-driven solutions, it is imperative for regulators and developers to work collaboratively to create frameworks that ensure these tools benefit all segments of the population equitably while safeguarding against potential misuse or misinterpretation of the diagnostic data generated by AI systems.
Challenges in AI-Driven Blood Testing
Artificial Intelligence (AI) is transforming the field of blood testing by enhancing the early detection of diseases such as ovarian cancer and pneumonia. One of the core methods involves the use of nanotube sensors, which can identify subtle patterns in blood samples. These sensors emit fluorescent light when interacting with blood molecules, and AI algorithms are able to recognize these unique light patterns to potentially detect cancer years before symptoms appear. This capability not only promises to accelerate diagnosis but also to significantly improve patient outcomes by facilitating prompt treatment interventions.
Amidst these technological advancements, there are considerable challenges that need addressing to fully realize the potential of AI in blood testing. One significant obstacle is the limited data available for training AI algorithms, particularly for rare diseases, which hampers the accuracy and reliability of these diagnostic tools. Additionally, there's a notable lack of cooperation among research institutions in terms of data sharing, which restricts the development of comprehensive AI models. Understanding the intricate connections between various biomarkers and corresponding diseases is another challenge that researchers are striving to overcome to ensure robust and reliable disease prediction through AI.
The potential benefits of AI-driven blood testing are immense, ranging from reduced healthcare costs due to earlier diagnoses and more targeted treatments to a paradigm shift in disease management that focuses on early intervention and personalized medicine. The advancements in AI technology promise significant improvements in life expectancy and quality of life by facilitating timely and precise disease detection. However, to fully harness the benefits of this technology, issues such as data privacy, informed consent, and equitable access to AI-powered diagnostics must be effectively addressed. The challenge remains to balance technological innovations with ethical considerations to ensure that AI-driven blood testing contributes positively to societal health outcomes.
Learn to use AI like a Pro
Get the latest AI workflows to boost your productivity and business performance, delivered weekly by expert consultants. Enjoy step-by-step guides, weekly Q&A sessions, and full access to our AI workflow archive.
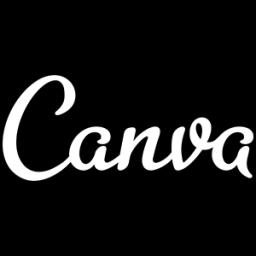
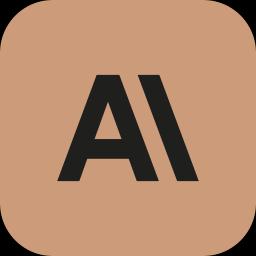
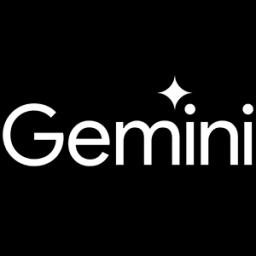
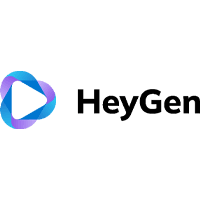
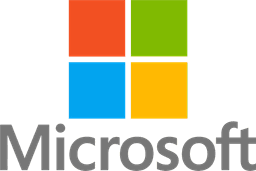
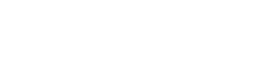
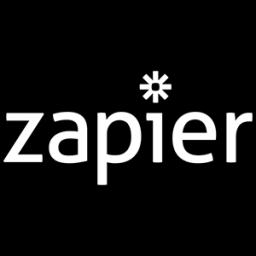
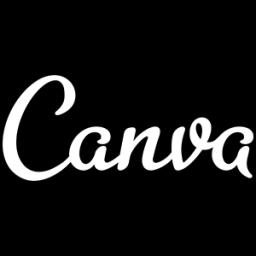
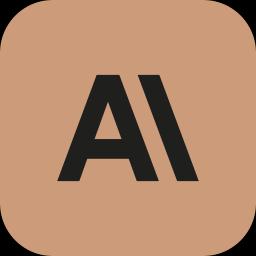
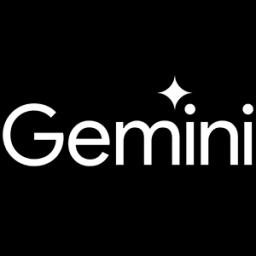
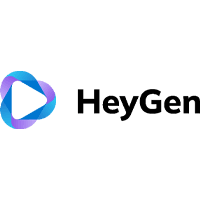
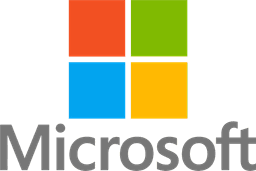
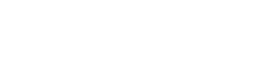
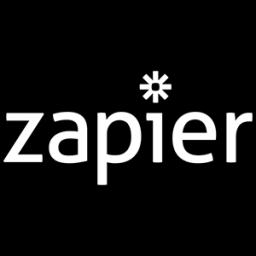
Accuracy and Availability of AI Blood Tests
AI-powered blood tests are revolutionizing healthcare by enhancing the early detection of diseases such as ovarian cancer and pneumonia, contributing to improved patient outcomes. These tests utilize advanced techniques, including nanotube sensors and microbial DNA databases, to recognize subtle patterns in blood samples. Nanotubes interact with blood molecules and emit fluorescent light, which AI algorithms then analyze to identify unique patterns. For instance, these technologies hold the potential to detect ovarian cancer well before symptom onset, providing an edge over traditional methods.
In the realm of pneumonia diagnosis, AI-driven systems analyze microbial DNA in blood samples, cross-referencing large-scale databases to quickly and accurately identify potential pathogens. This allows for rapid and targeted antibiotic treatment, significantly improving the speed and effectiveness compared to conventional diagnostic methods. However, despite these advances, challenges remain. There is a noticeable lack of comprehensive data, particularly for rare diseases, which limits the training of AI algorithms. Additionally, issues surrounding data sharing among institutions and the understanding of biomarker-disease connections remain significant hurdles.
The availability and accuracy of these AI-powered tests are subjects of growing interest and debate. While certain tests like the pneumonia diagnosis by Karius have started to enter clinical settings, most remain in experimental phases. Questions about the cost-effectiveness also arise, as AI tests have the potential to be cheaper, thus offering a sustainable alternative to the much costlier traditional diagnostic procedures. Other diseases could benefit from these AI advancements, which may one day extend to various cancers, infectious diseases, and chronic conditions.
Similarly, ethical considerations about AI in medical diagnostics pose important questions. Concerns regarding data privacy, the potential for algorithmic bias, and equitable access to AI technologies are paramount. Transparency of AI algorithms, often described as 'black boxes,' remains critical to building trust among the public. Moreover, supporting equitable access to AI-driven diagnostics is essential to prevent widening health disparities, ensuring that these technologies benefit a broad spectrum of society.
Public reactions to the introduction of AI-powered blood tests are mixed, with enthusiasm around the promise of earlier disease detection and improved treatment outcomes tempered by concerns over data privacy and the potential for over-reliance on AI systems leading to a de-skilling of clinicians. The economic, social, and ethical implications of widespread AI adoption in diagnostics further underscore the need for comprehensive discussions and regulations to safeguard the interests of all stakeholders.
Cost Implications of AI Testing
The integration of AI into blood testing offers significant opportunities for cost savings across healthcare systems. With traditional diagnostic methods often being time-consuming and costly, AI-driven blood tests present a viable alternative that can lower costs through automation and increased efficiency. The costs associated with conditions like pneumonia, which traditionally involve extensive testing and prolonged hospital stays, can be drastically reduced. The Karius test, for instance, exemplifies how AI can cut down the expenses from tens of thousands to just the price of a single test, facilitating more economical healthcare solutions.
Learn to use AI like a Pro
Get the latest AI workflows to boost your productivity and business performance, delivered weekly by expert consultants. Enjoy step-by-step guides, weekly Q&A sessions, and full access to our AI workflow archive.
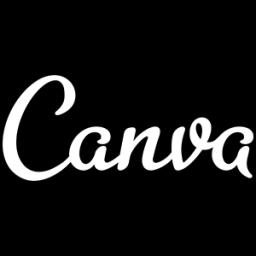
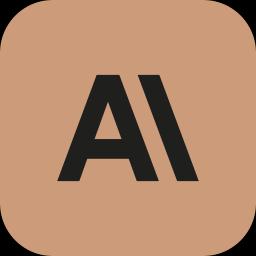
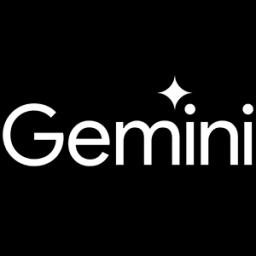
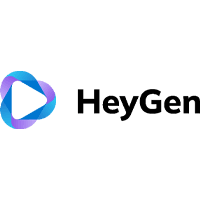
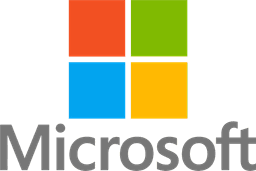
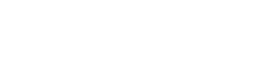
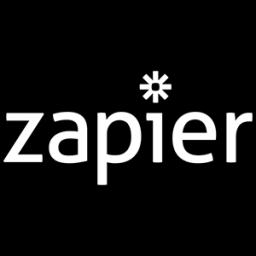
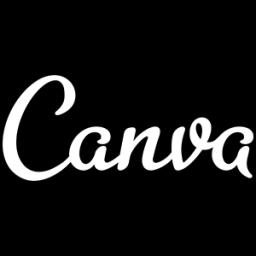
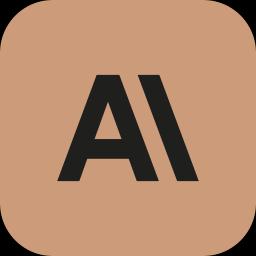
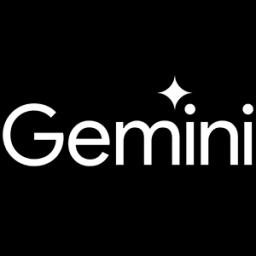
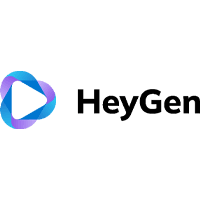
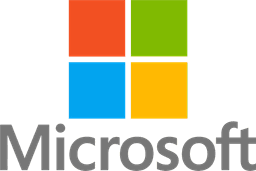
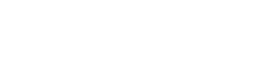
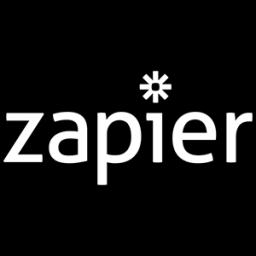
However, the initial investment in technology and infrastructure necessary for AI testing can be substantial. This includes the costs related to developing AI algorithms, procuring advanced laboratory equipment, and hiring skilled personnel to manage and interpret AI outputs. For medical facilities, particularly in low-income areas, these upfront costs can be prohibitive, potentially widening the gap in healthcare access between different demographics and regions.
The long-term financial benefits of AI testing could outweigh the initial development and implementation costs. Earlier and more accurate disease detection can lead to better patient management, reducing the need for costly treatments at advanced disease stages. This shift towards preventive medicine and early intervention is likely to drive down overall healthcare expenditures and improve healthcare quality and outcomes.
Nevertheless, ethical and regulatory challenges might introduce additional costs. Ensuring data privacy, addressing biases in AI algorithms, and maintaining compliance with healthcare regulations are essential but also financially demanding. These requirements necessitate continuous investments in technology updates and staff training, which could impact the affordability and widespread adoption of AI-powered blood tests.
In summary, while AI-powered blood tests hold the promise of reduced costs and improved healthcare efficiency, the associated cost implications are complex. Stakeholders must balance technological investments with ethical and regulatory responsibilities to ensure these innovations are both economically viable and accessible to diverse populations.
Potential Diseases for AI Blood Tests
Artificial intelligence (AI) technology is at the forefront of transforming traditional medical practices, particularly in the realm of blood tests for early disease detection. AI algorithms, processing vast amounts of data from nanotube sensors and microbial DNA databases, are capable of identifying patterns undetectable by conventional methods. These advancements offer a significant leap in diagnosing diseases such as ovarian cancer and pneumonia, not only fostering earlier intervention but also enhancing patient outcomes.
Ovarian cancer, often diagnosed at a late stage, stands to benefit immensely from these AI-enhanced techniques. By employing nanotube sensors that emit specific fluorescent patterns when interacting with cancerous blood molecules, AI systems are paving the way to spot the disease years before physical symptoms manifest themselves. This level of early detection could dramatically decrease mortality rates for ovarian cancer, presenting a promising shift in cancer diagnostics.
Learn to use AI like a Pro
Get the latest AI workflows to boost your productivity and business performance, delivered weekly by expert consultants. Enjoy step-by-step guides, weekly Q&A sessions, and full access to our AI workflow archive.
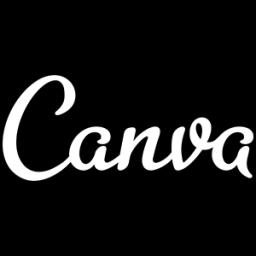
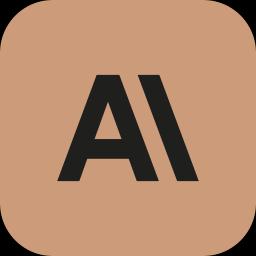
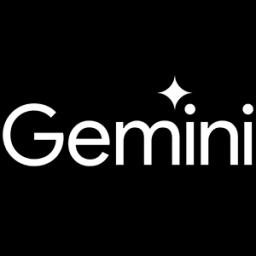
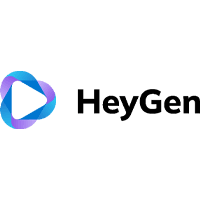
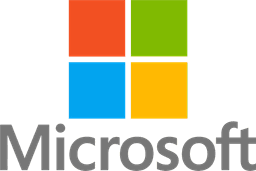
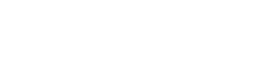
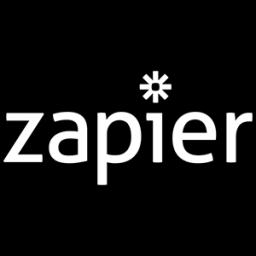
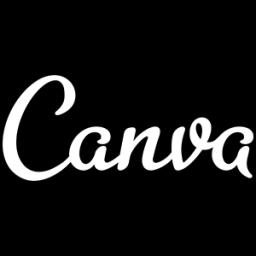
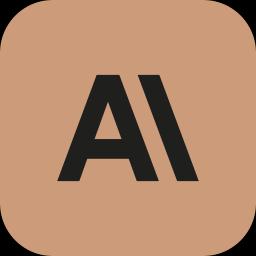
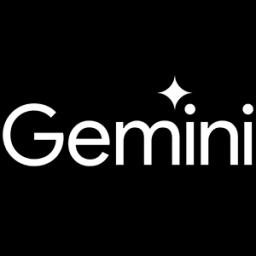
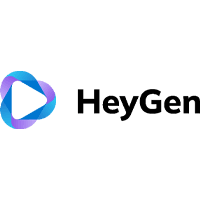
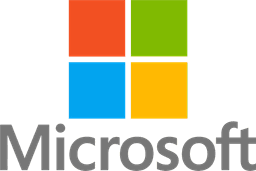
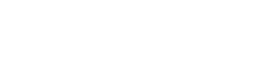
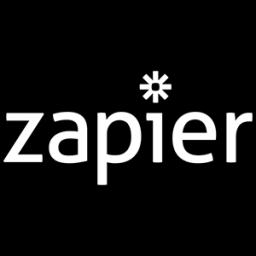
Similarly, in the case of pneumonia, AI's ability to scrutinize microbial DNA from blood samples allows for rapid identification of the causative pathogens. This capability aids in administering timely and precise antibiotic treatments, significantly improving recovery times and reducing hospital stays. Moreover, the cost efficiency introduced by utilizing fewer diagnostic resources further underscores AI's potential to make healthcare more accessible and affordable.
However, significant challenges remain, particularly related to the availability and quality of data used to train AI models. The lack of comprehensive and shared databases limits the effectiveness of AI, especially for rare diseases. Furthermore, bridging the gap between identified biomarkers and their clinical significance requires deeper understanding and collaboration across the medical and research communities.
As some AI-powered tests make their way into clinical settings, questions about their accuracy and cost compared to traditional diagnostics continue to surface. While AI-driven tests like those developed by Karius for pneumonia show promising reductions in diagnosis costs, widespread adoption hinges on further validation and understanding of these technologies. The potential to extend this AI technology to other diseases is immense, promising advancements in various fields of medicine.
Ethical Considerations of AI in Diagnostics
As AI continues to revolutionize the field of medical diagnostics, its application in blood tests is garnering significant attention, effectively changing how diseases are detected and managed. These advancements raise important ethical questions concerning patient privacy, data security, and the potential for algorithmic bias.
One major ethical consideration involves data privacy. Given that AI systems rely on large datasets to train and improve, ensuring the privacy of this medical data becomes paramount. Stringent measures must be implemented to protect sensitive patient information from unauthorized access and breaches.
Another critical issue is algorithmic bias. AI systems tend to reflect the biases present in the data they are trained on. This has the potential to lead to inequitable healthcare outcomes, particularly for underrepresented and vulnerable groups. Thus, it is essential to be vigilant about testing AI systems for bias and ensuring they are trained on diverse datasets.
Learn to use AI like a Pro
Get the latest AI workflows to boost your productivity and business performance, delivered weekly by expert consultants. Enjoy step-by-step guides, weekly Q&A sessions, and full access to our AI workflow archive.
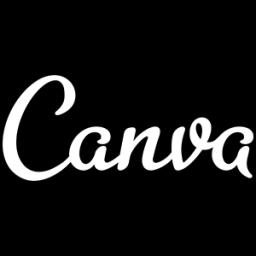
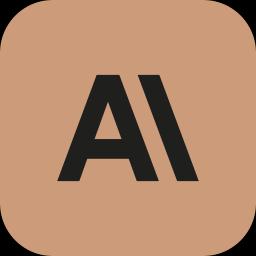
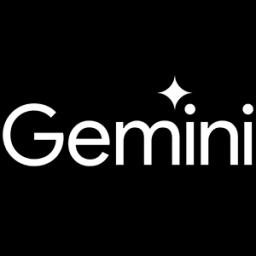
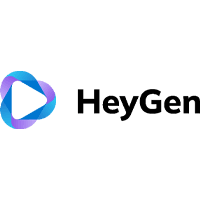
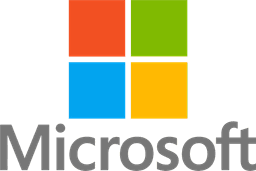
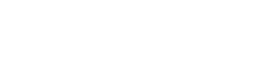
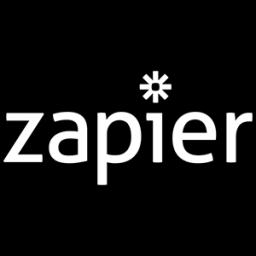
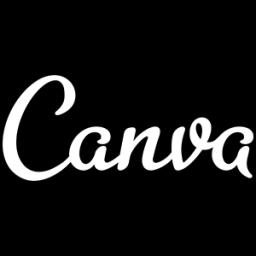
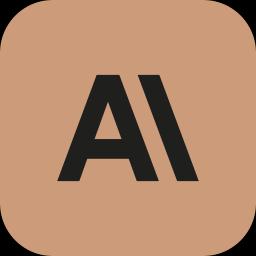
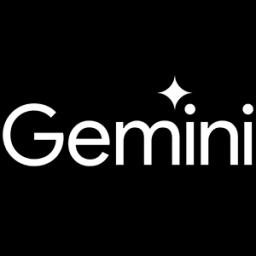
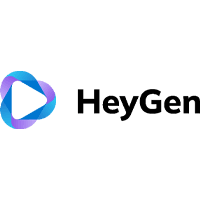
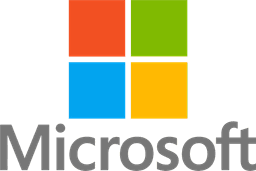
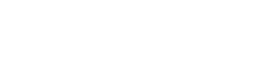
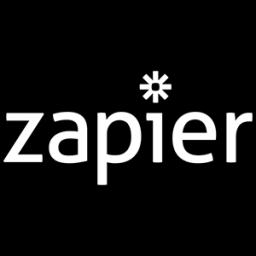
In addition to bias and privacy, there is an ethical concern around the 'black box' nature of AI technologies, where the decision-making process of AI systems remains opaque. This lack of transparency can hinder the trust clinicians and patients place in AI-powered diagnostics, necessitating efforts to make AI algorithms more interpretable.
Equitable access to AI diagnostics is another considerable ethical challenge. While these technologies promise significant advancements in early disease detection, there is a risk that they could exacerbate existing healthcare disparities if they become accessible only to those who can afford them. Policymakers and stakeholders must work towards making these technologies widely available.
Moreover, implementing AI diagnostics raises issues around informed consent. Patients must be adequately informed about how AI technologies operate, including their potential limitations and risks, to make informed decisions about their use.
Finally, the integration of AI in diagnostics poses legal and liability questions. As AI-driven decisions become integral to patient care, establishing clear liability frameworks for potential errors, such as misdiagnoses, will be essential. This will require novel legal approaches to address responsibility and patient rights in the age of AI healthcare technologies.
Key Developments in AI Blood Testing
AI blood testing represents a significant breakthrough in medical diagnostics, offering promising advancements in the early detection of diseases. By leveraging sophisticated technologies such as AI algorithms, nanotube sensors, and expansive DNA databases, researchers are uncovering intricate patterns within blood samples. This innovative approach has the potential to revolutionize how diseases like ovarian cancer and pneumonia are detected and treated, shifting the focus from reactive care to proactive and preventive healthcare strategies.
In the realm of ovarian cancer detection, scientists have successfully harnessed the unique properties of nanotubes. These nanotubes emit fluorescent light when interacting with specific molecules in blood samples. AI algorithms are then used to analyze these light patterns, potentially identifying cancer years before the onset of symptoms. This method not only enhances early detection capabilities but could also significantly improve patient outcomes by enabling earlier interventions.
Learn to use AI like a Pro
Get the latest AI workflows to boost your productivity and business performance, delivered weekly by expert consultants. Enjoy step-by-step guides, weekly Q&A sessions, and full access to our AI workflow archive.
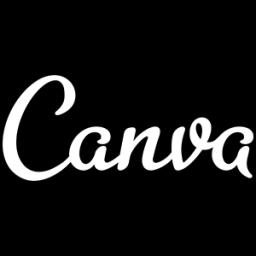
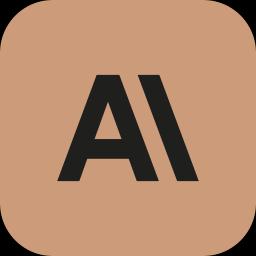
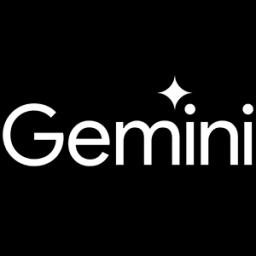
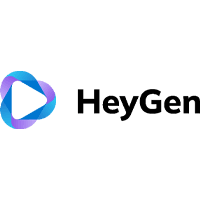
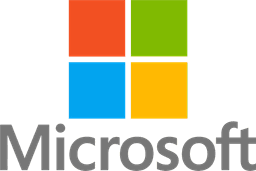
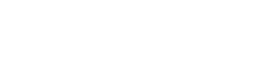
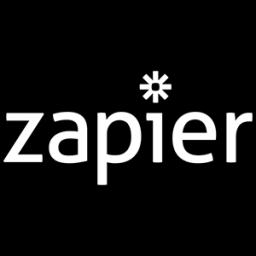
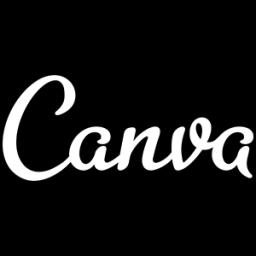
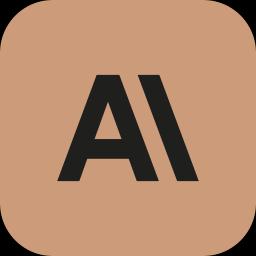
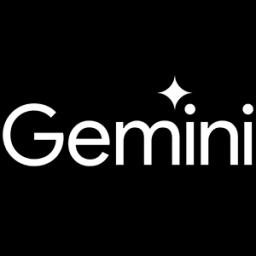
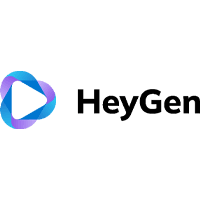
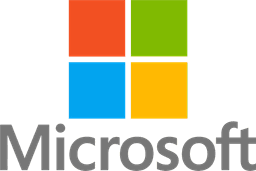
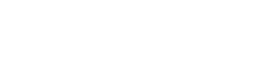
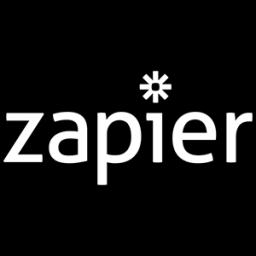
The application of AI in diagnosing pneumonia involves analyzing microbial DNA found in blood samples. By comparing this DNA against extensive databases, AI systems can rapidly identify pathogens, allowing for timely and targeted antibiotic treatments. This represents a considerable improvement over traditional methods, which often require complex and time-consuming testing processes.
Despite these impressive advances, the implementation of AI in blood testing faces significant challenges. One of the primary obstacles is the limited data available for training AI algorithms, particularly in the case of rare diseases. Additionally, the lack of data sharing among various researchers and institutions hinders progress in this field. Understanding the complex connections between various biomarkers and diseases remains a critical hurdle that researchers must overcome.
The accuracy of AI-powered blood tests remains a concern, as it varies depending on the disease in question. For instance, while AI methodologies for ovarian cancer detection show promise, further validation is needed to establish definitive accuracy rates. Furthermore, although AI-powered tests for diseases like pneumonia are becoming more accessible in clinical settings, many remain in experimental stages.
Economic implications of AI blood testing suggest potential cost reductions in healthcare, as these methods typically involve fewer tests and faster diagnostic processes. Socially, the technology offers hope for improved life expectancy and quality of life through earlier disease detection. However, there are concerns about health disparities if access to these tests is limited by cost or geography.
From a medical perspective, AI blood testing marks a paradigm shift towards more personalized medicine, encouraging early interventions rather than traditional treatment approaches. However, it also raises ethical and legal questions, including issues of data privacy and the potential biases in AI algorithms. Ensuring equitable access and addressing potential discrimination based on genetic information remain integral to the successful integration of AI-driven diagnostics into mainstream healthcare.
Future implications of AI in blood testing extend beyond healthcare improvements. Politically, the use of AI in medical diagnostics will likely spur policy discussions regarding genetic privacy and data regulation. Moreover, it highlights the need for international cooperation in developing equitable health technologies and addressing potential disparities exposed by these advancements.
Learn to use AI like a Pro
Get the latest AI workflows to boost your productivity and business performance, delivered weekly by expert consultants. Enjoy step-by-step guides, weekly Q&A sessions, and full access to our AI workflow archive.
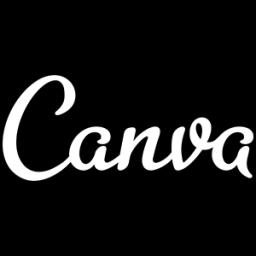
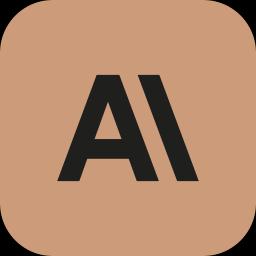
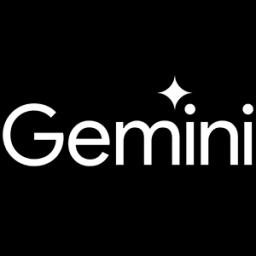
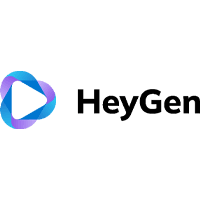
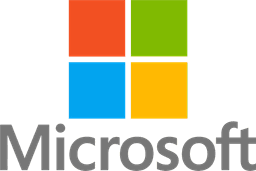
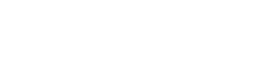
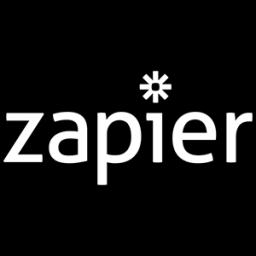
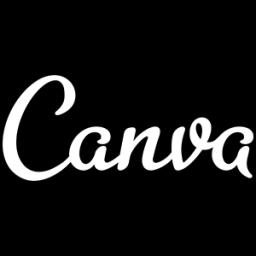
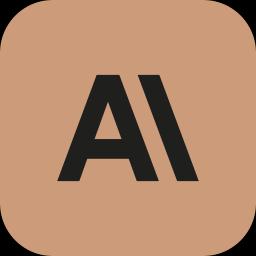
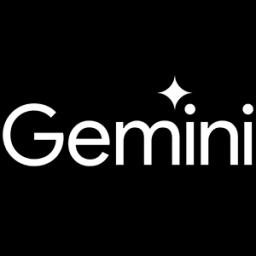
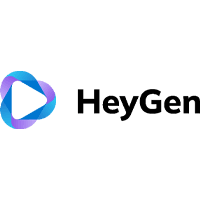
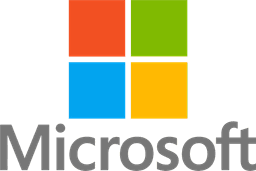
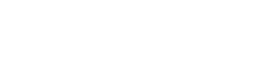
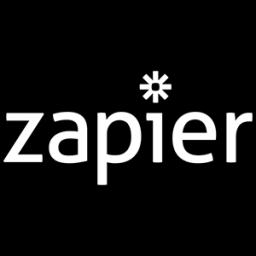
Expert Opinions on AI Blood Tests
The advent of AI-powered blood tests marks a significant shift in the landscape of disease detection and diagnosis, offering the potential for earlier identification of conditions that traditionally go unnoticed until they have progressed to more advanced stages. Techniques such as nanotube sensors and databases of microbial DNA expand the possibilities for detecting cancers and infections far earlier than was previously feasible. In ovarian cancer detection, for example, the ability of nanotubes to emit unique fluorescent patterns upon interacting with specific blood molecules provides a promising avenue for recognizing cancer long before symptoms manifest, perhaps even years in advance.
In the realm of pneumonia diagnosis, AI's analytical prowess comes into play by comparing microbial DNA from blood samples against extensive databases, facilitating the swifter identification of pathogens and allowing for more targeted antibiotic treatments. Such innovations portend a future where patients receive not only faster but more precise medical interventions, which could substantially improve outcomes and healthcare efficiency.
Despite these promising advancements, several hurdles must be overcome before AI blood tests can fulfill their potential. A primary challenge involves the scarcity of data necessary for training accurate AI algorithms, especially concerning rare disease profiles. Moreover, the prevalent lack of data-sharing practices among hospitals and research institutions hampers the widespread applicability and enhancement of AI technologies.
Furthermore, the intricate relationship between biomarkers observed through these AI methods and their respective diseases remains an enigma that continues to puzzle researchers. This ‘black box’ aspect of AI engenders skepticism and necessitates further studies to demystify the underlying processes that AI leverages to draw conclusions.
Access to these AI advancements also poses an ethical dilemma. While the potential for significantly reduced costs and fewer diagnostic procedures is appealing, there are valid concerns regarding cost-related access disparities and the security of data used in AI computations. Moreover, the potential for AI to exacerbate existing biases—given the current limitations in data diversity—raises questions about equitable healthcare distribution.
Public sentiment towards AI-powered blood diagnostics is a blend of optimism and apprehension. Positively, there's a surge of hope for earlier and less intrusive diagnosis of diseases like ovarian cancer and pneumonia, alongside a broad appreciation for the increased speed and cost-effectiveness AI brings. On the flip side, concerns about AI's accuracy in rare conditions, its potential cost barriers, and the ethical implications of using AI in everyday clinical settings remain prevalent.
Learn to use AI like a Pro
Get the latest AI workflows to boost your productivity and business performance, delivered weekly by expert consultants. Enjoy step-by-step guides, weekly Q&A sessions, and full access to our AI workflow archive.
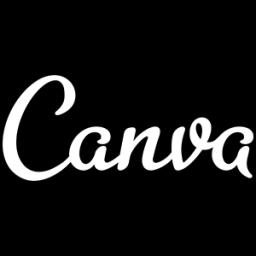
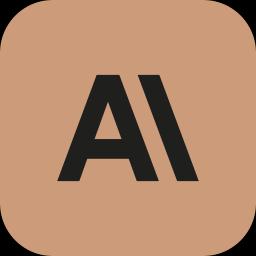
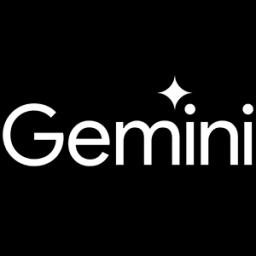
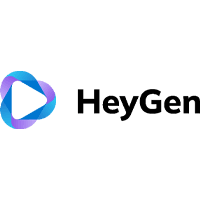
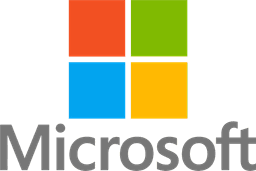
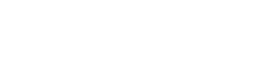
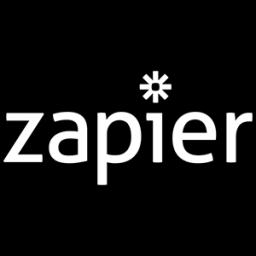
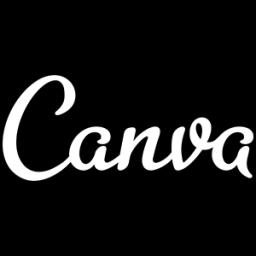
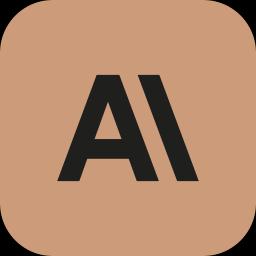
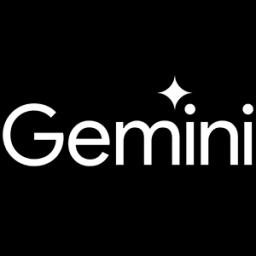
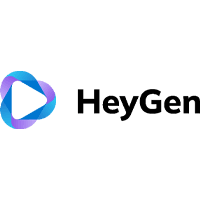
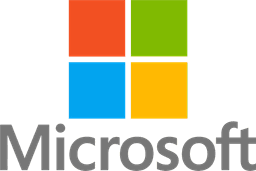
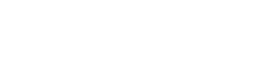
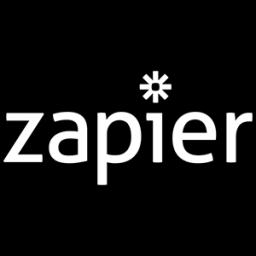
Regardless of these challenges, the implication of AI in blood tests signals a transformative future for healthcare. Economically, healthcare costs might decrease considerably as treatments become more targeted and preventive, albeit with a potential job market shift from traditional diagnostics to AI-focused roles. Socially, life expectancy and quality of life could see improvements, though the risk of over-diagnosis and health anxiety cannot be disregarded.
Moreover, as AI blood tests edge toward real-time monitoring and possibly predictive epidemiological capabilities, they herald significant strides in precision medicine and patient care. Nonetheless, this burgeoning technological field must tread carefully regarding ethical and legal aspects, navigating liability, genetic privacy, and ensuring equitable treatment access amidst its expansionary trail.
Public Reactions to AI Diagnostics
The advent of AI-powered blood tests has generated significant public interest, with individuals expressing a spectrum of reactions spanning from optimism to cautious skepticism. On one hand, many see AI as a promising tool for earlier detection of diseases like ovarian cancer and pneumonia, potentially leading to improved treatment outcomes and lowered healthcare costs. There is considerable enthusiasm particularly for its capacity to render diagnoses with greater speed and accuracy compared to traditional methods, and for its potential to alleviate healthcare inequalities especially in underserved populations.
Conversely, the public has articulated several reservations about these AI diagnostic systems. Concerns abound regarding the accuracy of these tests, particularly in the context of rare diseases where data may be scarce. There are fears about the economic barriers that may prevent equitable access to such technologies, with high costs potentially fostering disparities. Furthermore, issues of data privacy and security loom large, with patients worried about how their sensitive health data might be handled and protected.
The opacity or so-called 'black box' nature of AI algorithms contributes to unease, as the inner workings and decision-making processes of these systems remain largely inscrutable to their users. This lack of transparency raises questions about trust, particularly among healthcare professionals who may fear de-skilling due to an over-reliance on machine-generated interpretations. Moreover, ethical considerations, including informed consent, potential bias within algorithms, and liability in the event of errors or misdiagnoses, are central to public discourse.
Future implications considered by the public include not only the economic and medical benefits of AI blood tests but also social and ethical ramifications. There is anticipation of a shift towards preventive care and personalized medicine, driven by improved diagnostics. At the same time, there's concern over increased health anxiety due to the affordability and accessibility of frequent testing. Alongside this, potential political and legal controversies could arise concerning genetic privacy, international competitiveness in AI healthcare technologies, and the need for robust regulatory frameworks to ensure equitable access and prevent discrimination.
Learn to use AI like a Pro
Get the latest AI workflows to boost your productivity and business performance, delivered weekly by expert consultants. Enjoy step-by-step guides, weekly Q&A sessions, and full access to our AI workflow archive.
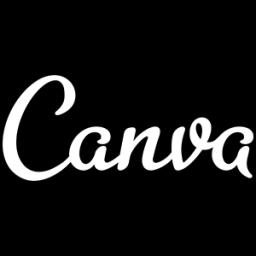
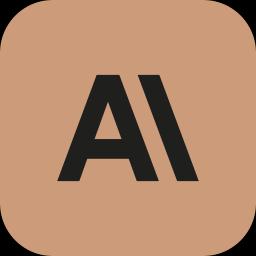
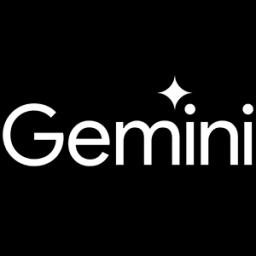
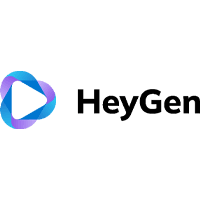
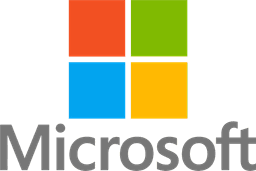
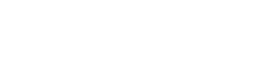
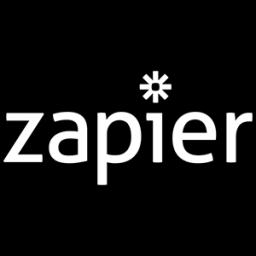
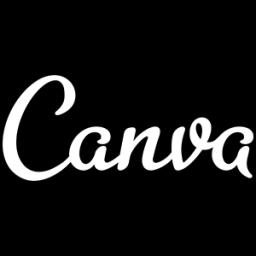
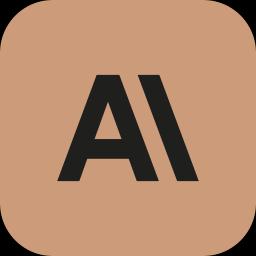
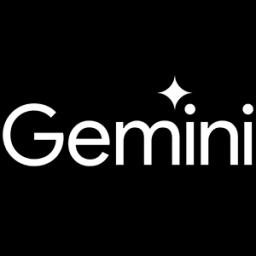
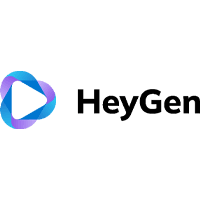
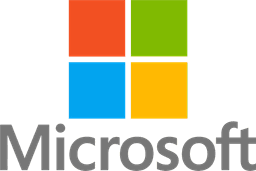
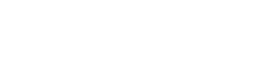
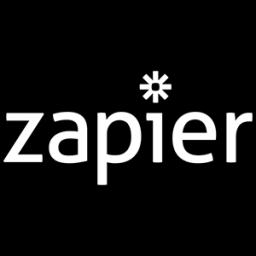
In summary, while AI-driven blood tests for disease detection promise a transformative impact on healthcare, their deployment raises intricate concerns that must be navigated carefully to win public trust and acceptance. Balancing technological advancements with ethical considerations, transparency, and equitable accessibility will be critical as these innovations continue to evolve.
Future Implications of AI in Healthcare
The advent of AI-powered blood tests represents a significant leap in healthcare diagnostics, potentially transforming the industry. AI techniques have already shown promising results in detecting diseases such as ovarian cancer and pneumonia more quickly and accurately than traditional methods. Using nanotube sensors and AI algorithms, medical professionals can identify disease markers from blood tests that were previously undetectable. These technological advances promise to improve patient outcomes by facilitating earlier diagnosis and more personalized treatment plans.
Nanotube sensors, which emit fluorescent light upon interacting with blood molecules, together with AI algorithms capable of recognizing unique patterns, represent a breakthrough in ovarian cancer detection. These AI systems demonstrate the potential to identify cancer many years before symptoms appear, offering hope for significantly reducing mortality rates. Similarly, in pneumonia diagnoses, AI's ability to analyze microbial DNA from blood samples enables quick pathogen identification and more targeted antibiotic treatments, enhancing the standard of care.
Despite their promise, AI-powered blood tests face several key challenges. One significant hurdle is the limited data available for training AI algorithms, especially concerning rare diseases. This challenge is compounded by the insufficient data sharing among researchers and institutions, which hampers collaborative efforts and stifles advancements. Additionally, there is still much to learn about how biomarkers correlate with specific diseases, necessitating further research and development in this field.
The accuracy of these AI-driven diagnostic tools is under scrutiny, with ongoing research aiming to define precise accuracy rates. For instance, while groundbreaking, the use of nanotubes for early ovarian cancer detection requires further validation. As most of these innovations are still in research phases, public accessibility is limited. However, companies like Karius are pioneering clinical applications, with some AI tests for conditions like pneumonia already in use, showing the potential for cost reductions and efficiency gains.
Though most AI-powered blood tests remain restricted to research environments, their potential reach is expansive. Besides ovarian cancer and pneumonia, this technology offers prospects for a wider range of diseases, from various cancers to chronic conditions and infectious diseases. Ethical considerations, particularly around data privacy, bias in AI algorithms, and equitable access, remain at the forefront of discussions. Addressing these challenges is critical for the broader acceptance and integration of AI in healthcare diagnostics.
Learn to use AI like a Pro
Get the latest AI workflows to boost your productivity and business performance, delivered weekly by expert consultants. Enjoy step-by-step guides, weekly Q&A sessions, and full access to our AI workflow archive.
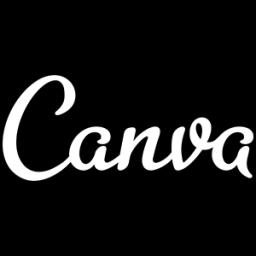
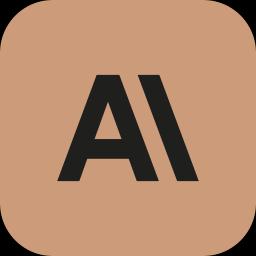
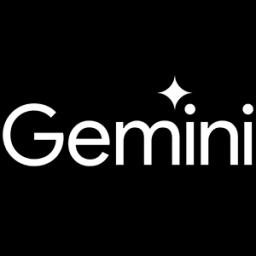
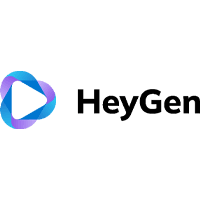
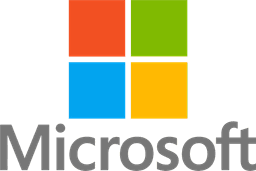
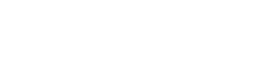
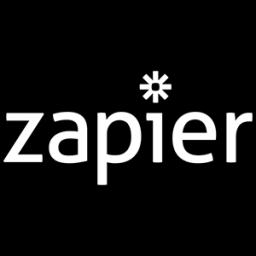
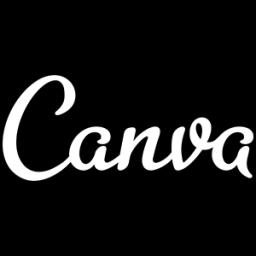
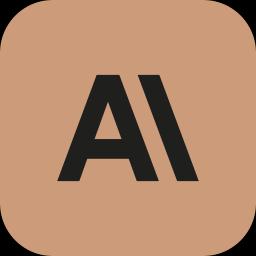
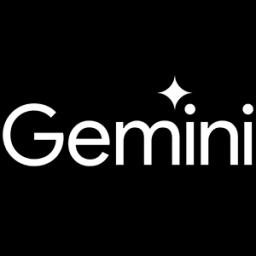
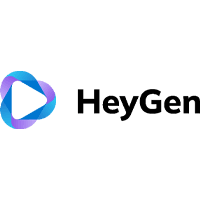
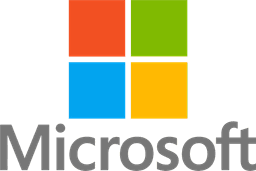
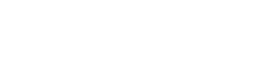
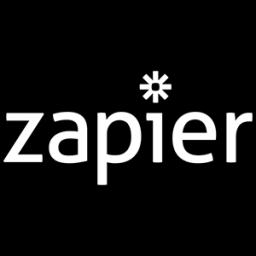