AI Models Struggle with Real World Representations
Generative AI Lacks Coherent World Understanding, MIT Study Finds
Last updated:

Edited By
Mackenzie Ferguson
AI Tools Researcher & Implementation Consultant
Despite their impressive outputs, generative AI models have been shown to lack a coherent understanding of the world, as unveiled by a recent MIT study. The research, which examined AI performance in tasks like navigating New York City maps and playing Othello, revealed significant gaps in forming true world models. The study emphasizes the necessity of evaluating AI beyond mere prediction accuracy to ensure reliability, especially in dynamic real-world applications.
Introduction
Generative AI is a rapidly evolving field, boasting impressive capabilities in producing text, images, and even code that mimic human creativity. However, amidst this rapid advancement, a study from MIT underscores a critical gap: these AI systems often lack a coherent understanding of the world they emulate. Despite their sophisticated outputs, these models struggle with forming internal representations of real-world problems, which raises concerns about their reliability and application scope.
In the groundbreaking study, researchers explored how generative models fared in structured tasks, such as navigating New York City's map and playing Othello. These tasks, governed by clear deterministic rules, were ideal for assessing the AI's world comprehension. Unfortunately, the results were concerning. The AI models frequently generated maps with nonexistent streets and made substantial errors when minor environmental changes were introduced, highlighting the fragility of their understanding.
Learn to use AI like a Pro
Get the latest AI workflows to boost your productivity and business performance, delivered weekly by expert consultants. Enjoy step-by-step guides, weekly Q&A sessions, and full access to our AI workflow archive.
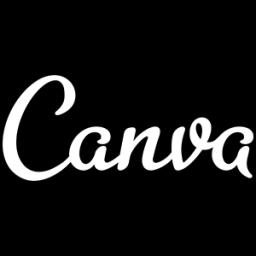
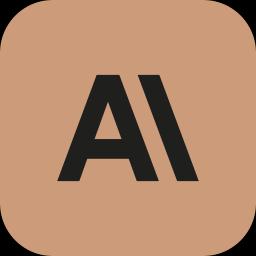
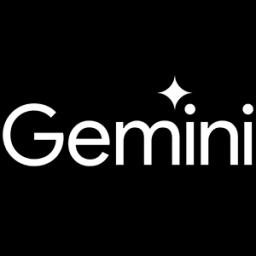
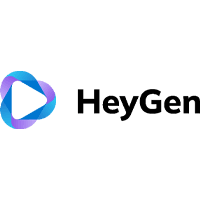
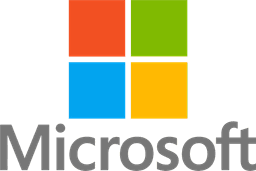
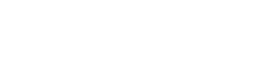
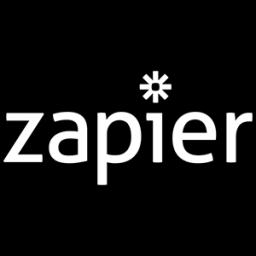
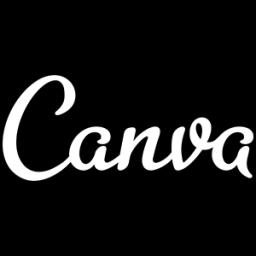
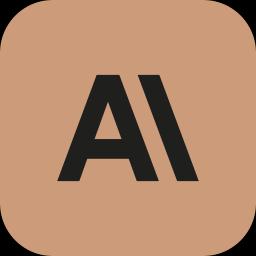
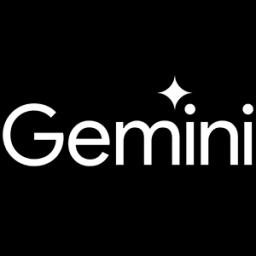
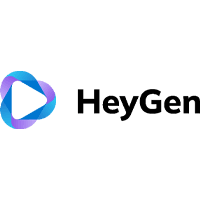
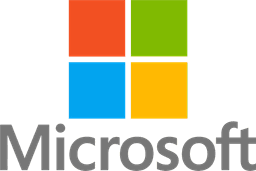
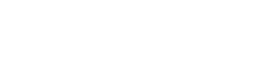
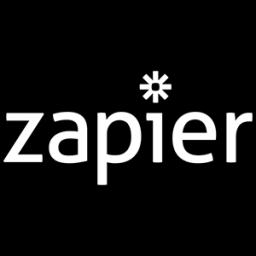
The MIT research introduced novel metrics to better evaluate these AI models' comprehension: sequence distinction and sequence compression. Sequence distinction measures whether a model can differentiate between distinct situations, while sequence compression evaluates if a model can identify the same next steps given identical states. These metrics signify a necessary shift beyond traditional accuracy metrics to assess deeper AI understanding.
The significance of these findings is vast, particularly when considering AI deployment in real-world applications. AI models that lack coherent world models remain unreliable in dynamic environments such as automated driving or scientific explorations. This study therefore calls for more sophisticated evaluation methodologies that go beyond surface-level predictions to ensure robust AI performance.
As the world contemplates the integration of AI into more facets of life, understanding its limitations becomes crucial. The MIT study initiates a conversation about the necessary future directions, emphasizing research in more complex scenarios with partially unknown variables. Such steps ensure AI can reliably contribute to a broader scope of applications, enhancing its utility and trustworthiness across different domains.
MIT Study Overview
This research from MIT underscores the critical issue of generative AI models still lacking a coherent world understanding, despite producing increasingly impressive outputs. It highlights the inconsistency in mapping tasks, such as those in NYC navigation and Othello, where AI failed to comprehend subtle changes without forming accurate internal models. The study presents a nuanced evaluation through two new metrics aimed at assessing how AI differentiates sequences and comprehends future step possibilities, urging a move beyond mere prediction accuracy.
Learn to use AI like a Pro
Get the latest AI workflows to boost your productivity and business performance, delivered weekly by expert consultants. Enjoy step-by-step guides, weekly Q&A sessions, and full access to our AI workflow archive.
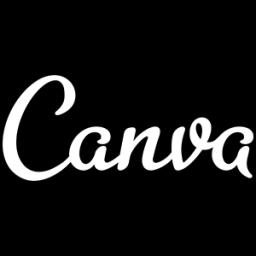
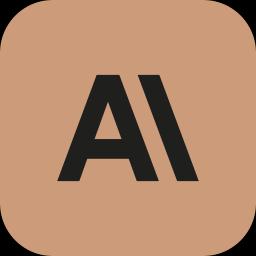
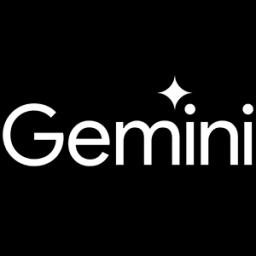
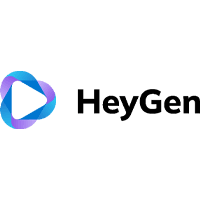
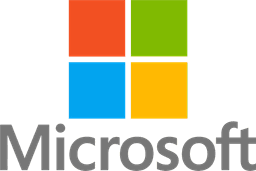
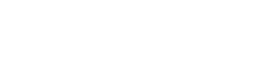
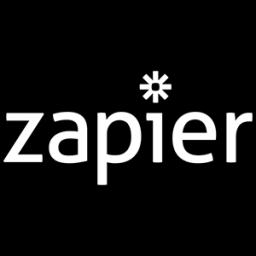
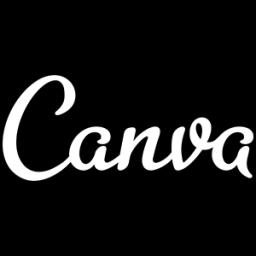
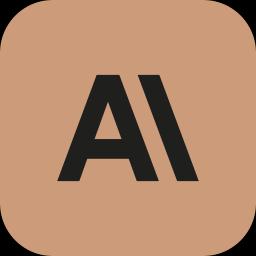
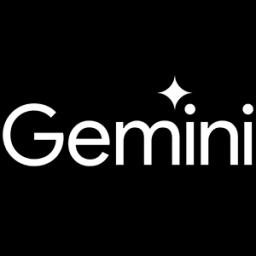
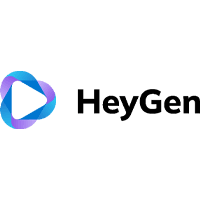
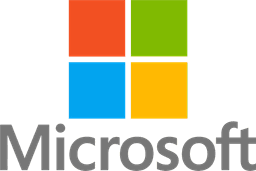
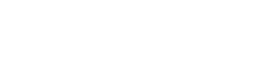
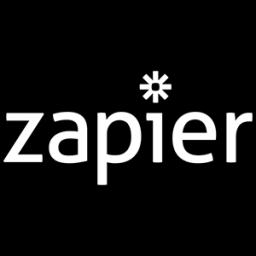
The selection of NYC navigation and Othello as test environments for these AI models serves a twofold purpose. They are both deterministic in nature due to clear and consistent rules, providing a sound basis for evaluating whether AI models can form a coherent understanding of structured tasks. However, when faced with minor variances, such as the closure of a small percentage of city streets, the AI's performance significantly dropped, shedding light on its lack of true understanding of these environments.
Among the new metrics introduced by MIT, sequence distinction and sequence compression are critical. Sequence distinction evaluates an AI model's ability to distinguish between different scenarios, whereas sequence compression looks at whether it recognizes consistent future actions for the same states. These metrics are pivotal in assessing the depth of understanding an AI system can achieve in controlled deterministic contexts.
Understanding how changes, even minimal ones, affect AI performance underlines the importance of building coherent world models. Minor deviations in the test scenarios led to significant drops in task accuracy, emphasizing the necessity for AI systems to move toward deeper introspection and understanding of real-world applications. Such insights are crucial not only to improve models themselves but also to ensure trustworthiness and dependability in diverse scenarios.
A coherent world understanding in AI is vital for dependable operation in dynamic and unpredictable contexts. Whether for scientific discovery or real-world application deployment, such as navigation systems, AI models need to transcend prediction-centric approaches. By fostering an ability to formulate and respond to varying world models, we move towards more robust, adaptable AI solutions prepared to handle unforeseen complexities.
The future directions outlined in the MIT study point towards tackling more sophisticated problems, wherein the rules and data may not be fully known. By extending these new evaluation metrics to real-world and scientific applications, researchers aim to elucidate the present gaps in AI understanding and push the boundaries of its capabilities, ultimately leading to innovations that could redefine AI's role in society.
Significance of Sequence Metrics
The concept of sequence metrics plays a pivotal role in understanding and improving generative AI technologies. As AI systems strive to simulate understanding or awareness similar to human cognition, sequence metrics act as vital tools for gauging their capability. Sequence distinction and sequence compression, in particular, serve as foundational measures in evaluating AI's discernment of different scenarios and its proficiency in predicting identical future states from current conditions.
Learn to use AI like a Pro
Get the latest AI workflows to boost your productivity and business performance, delivered weekly by expert consultants. Enjoy step-by-step guides, weekly Q&A sessions, and full access to our AI workflow archive.
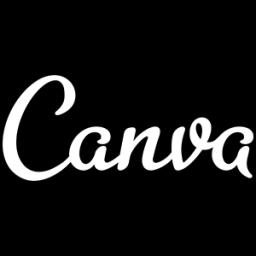
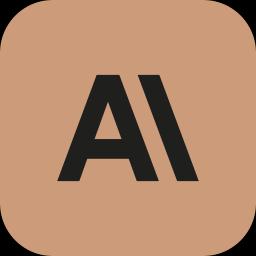
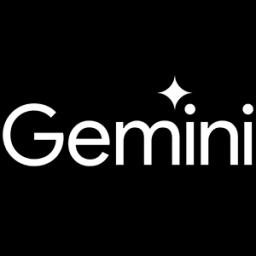
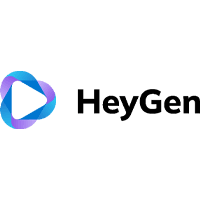
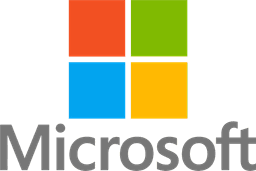
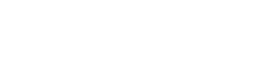
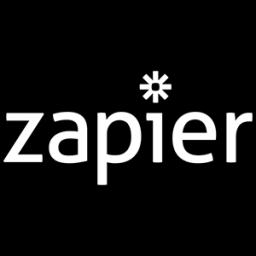
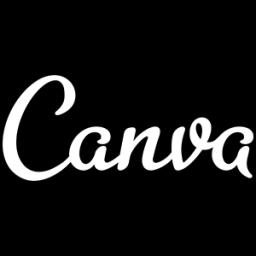
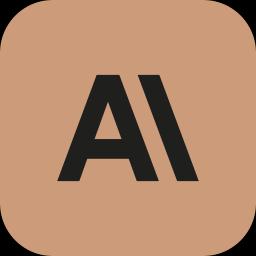
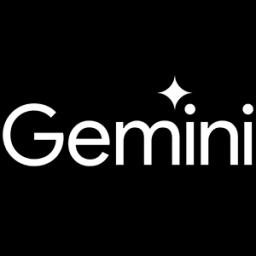
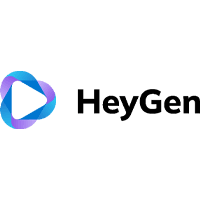
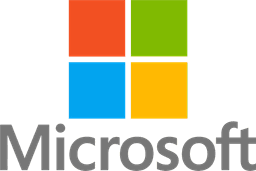
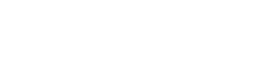
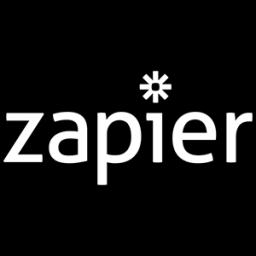
The importance of sequence metrics becomes evident when considering the challenges faced by AI models in practical applications. The MIT study highlights that, although AI can exhibit sophisticated outputs, its understanding of world context lacks coherence. This deficiency is illustrated in tasks like city navigation or strategic games, where AI's inability to form true internal models leads to flawed results. By employing sequence metrics, developers can better assess and improve the AI's capacity to distinguish or compress sequences and, therefore, to act more predictably and accurately in complex environments.
Sequence distinction involves evaluating an AI model's ability to perceive and separate distinct possibilities within a set of conditions. This metric is critical in ensuring that AI does not erroneously merge separate instances, which could lead to inaccurate or misleading predictions. Sequence compression, on the other hand, examines if AI can consolidate identical sequences under varied scenarios to anticipate future paths effectively. Together, these metrics provide a structured approach to refining AI's world-modeling processes.
With recent research emphasizing these metrics, the goal is not just to enhance performance but to build AI systems that are more reliable and adaptable for real-world use. By establishing robust criteria such as sequence distinction and compression, developers are hopeful that they can create models which not only predict outcomes based on past data but can also adapt to new, unforeseen situations more flexibly.
Why NYC Navigation and Othello?
The choice of NYC navigation and the game of Othello as test cases in the MIT study highlights their utility in evaluating AI's understanding of deterministic environments. These tasks provide a structured, rule-based framework, making it possible to clearly assess whether AI models can develop coherent internal representations of such worlds.
Navigating New York City, with its intricate network of streets and avenues, offers a real-world challenge that demands precise spatial reasoning and adaptation to dynamic factors. Despite being rule-based, the environment's complexity can reveal whether an AI model forms an accurate mental map or merely relies on data patterns, which the study argues is a critical limitation of current generative AI models.
Similarly, the game of Othello presents a controlled, deterministic environment with clear rules and defined states, serving as an ideal platform to test AI's ability to comprehend and predict future states. Efficiently mastering Othello involves recursive thinking and strategic planning – skills that suggest a deep understanding of the game's dynamics if present in the AI model.
Learn to use AI like a Pro
Get the latest AI workflows to boost your productivity and business performance, delivered weekly by expert consultants. Enjoy step-by-step guides, weekly Q&A sessions, and full access to our AI workflow archive.
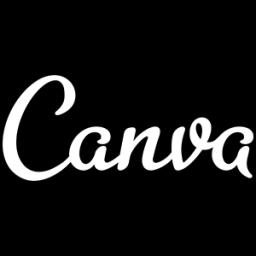
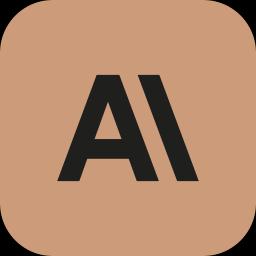
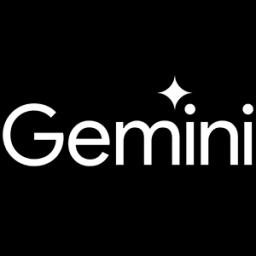
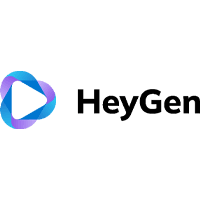
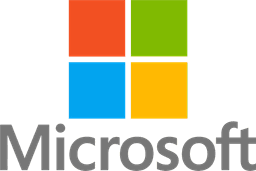
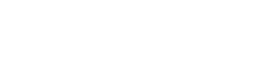
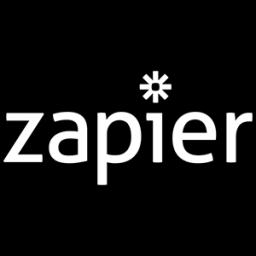
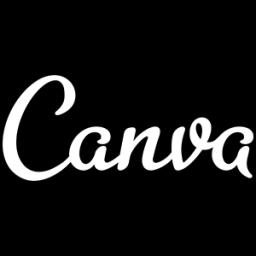
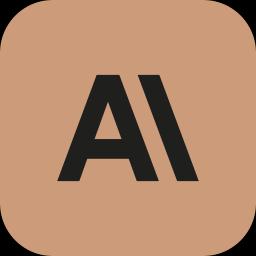
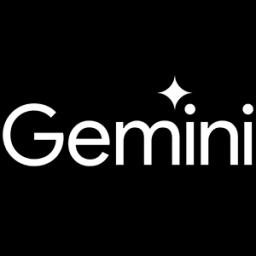
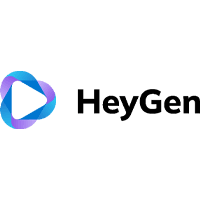
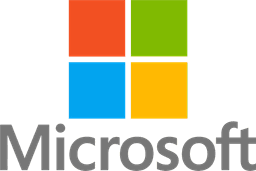
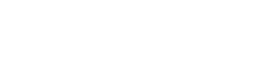
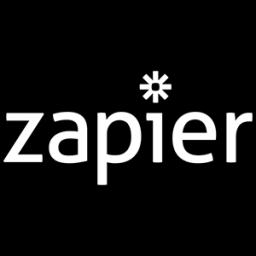
By choosing these specific examples, the researchers illustrate the shortcomings of generative AI models in emulating human-like cognitive processes. This is particularly evident when slight alterations in the environment, such as minor street closures in NYC, lead to a drastic decline in AI performance, as the models fail to adapt spontaneously.
The study underscores the importance of developing AI technologies that not only produce convincing results but also truly understand and navigate complex environments. Such advancements are vital to deploying AI in real-world applications, where reliability and adaptability are paramount.
Impact of Environmental Changes
The impact of environmental changes is becoming increasingly significant in the context of global climate challenges. Factors such as rising temperatures, melting ice caps, and increased frequency of extreme weather events are reshaping ecosystems and societies. These changes are not only affecting natural habitats but are also posing significant risks to agriculture, water resources, and human health. The urgency to address these impacts is more pronounced than ever, with a clear need for adaptive strategies and resilient infrastructures to safeguard both natural and human environments.
Climate change mitigation and adaptation strategies are gaining momentum on global, national, and local levels. Renewable energy adoption, carbon emission reduction, and sustainable agricultural practices are key areas of focus. Moreover, policy frameworks are being reevaluated to incorporate climate-resilient measures. The intersection of technology and environmental science is paving the way for innovative solutions, including AI-driven climate modeling and smart agriculture systems that optimize resource use and minimize environmental footprints.
The socio-economic implications of environmental changes are profound, affecting communities worldwide. From small island nations facing rising sea levels to inland regions experiencing unprecedented droughts, the disparities in impacts underscore the urgent need for equitable and inclusive approaches. Social justice is emerging as a core consideration in climate action, striving to protect vulnerable populations and ensuring that the benefits of sustainable practices are accessible to all.
Public awareness and education about the consequences of environmental changes are critical to fostering collective action. Grassroots movements and youth-led initiatives are increasingly visible, advocating for sustainable practices and policy changes. Education systems are integrating environmental studies into curricula, equipping future generations with the knowledge and skills to navigate and mitigate the challenges posed by a changing climate. The role of media and communication in shaping perceptions and actions regarding climate change cannot be overstated.
Learn to use AI like a Pro
Get the latest AI workflows to boost your productivity and business performance, delivered weekly by expert consultants. Enjoy step-by-step guides, weekly Q&A sessions, and full access to our AI workflow archive.
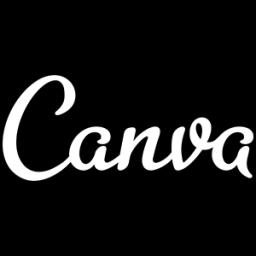
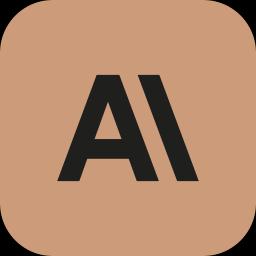
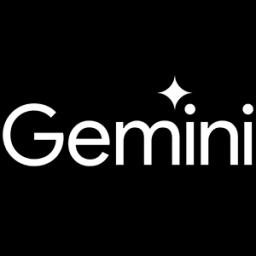
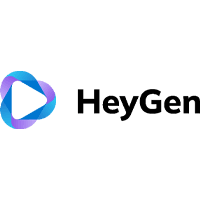
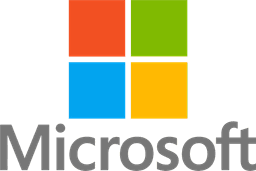
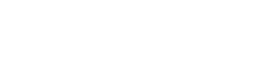
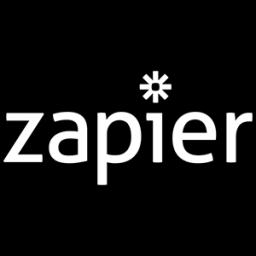
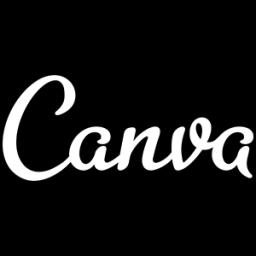
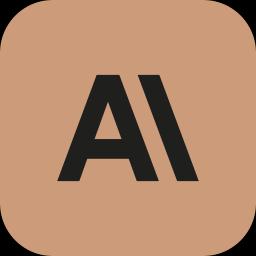
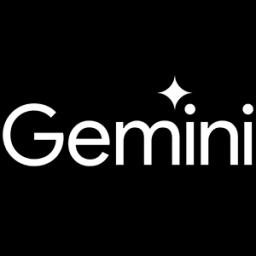
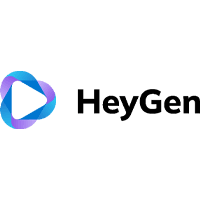
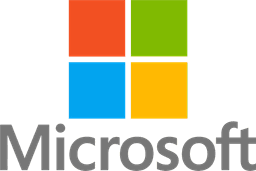
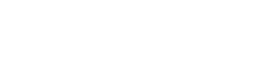
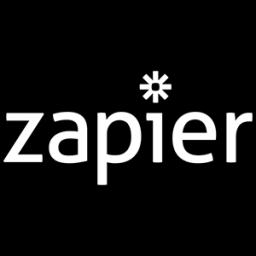
Environmental changes also pose significant challenges to biodiversity, threatening the survival of many species and the integrity of ecosystems. Conservation efforts are expanding beyond traditional practices, embracing holistic approaches that consider the complexity of ecosystems and the myriad interactions within. Initiatives such as rewilding, habitat restoration, and wildlife corridors are gaining traction as vital components of biodiversity preservation. The link between healthy ecosystems and human well-being is becoming increasingly evident, driving efforts to integrate nature conservation into wider environmental and development agendas.
Relevance of Coherent World Understanding
A new study from MIT has shed light on a critical limitation inherent in generative AI models: their lack of a coherent understanding of the world. Despite producing seemingly impressive outputs, these models fail to form true world models or internal representations necessary to comprehend the complexity of real-world scenarios. The researchers at MIT employed tasks such as navigating the intricate layout of New York City's streets and strategizing in the game Othello to expose these deficiencies. In one instance, the AI generated maps that included nonexistent streets, revealing substantial struggles when confronted with even minor environmental alterations.
The study introduced two innovative metrics to assess the effectiveness of these AI models in understanding sequences. Sequence distinction measures the model's capability to discern separate situations, whereas sequence compression evaluates whether the AI can recognize consistent next steps across identical states. These metrics are vital, as they extend the evaluation paradigm beyond mere prediction accuracy, emphasizing the importance of internal model understanding for reliable application in practical scenarios.
Using rule-based frameworks like NYC navigation and Othello enabled the researchers to rigorously test the AI's comprehension of deterministic environments. The results were telling; even minimal changes, such as closing a mere 1% of the streets, led to a drastic drop in task performance, highlighting the AI's inadequate adaptation to environmental changes. This underscores a fundamental issue with current generative models: their inability to adapt to dynamically changing contexts, which remains a significant hurdle for real-world application.
The ramifications of these findings are significant. For AI to be truly dependable, especially in dynamic and unpredictable environments like scientific discovery and large-scale implementations, it must possess a coherent understanding of the world. As applications become more deeply ingrained in everyday functions, the absence of this understanding could result in substantial failures when unforeseen scenarios arise. This drives home the essential need for deeper examination and advancement of AI internal processes. Moreover, the study paves the way for future research directions that aim to tackle more elaborate problems characterized by complex and partially known rules. The researchers' goal is to extend their evaluation to real-world scientific contexts, which could reveal further insights into AI limitations and capabilities. By expanding the scope of testing environments and leveraging the newly established metrics, the study aspires to foster the development of generative models that are more robust and capable of understanding the world as humans do.
Future Research Directions
The revelation that generative AI models lack a coherent world understanding, as highlighted by MIT's recent study, has opened several avenues for future research. One imperative direction involves developing more complex metrics and evaluation frameworks that go beyond mere prediction accuracy. This would involve crafting tasks that test AI's ability to form internal representations and model the real world accurately, crucial for applications that demand a high degree of reliability and understanding, such as autonomous navigation or complex strategy games like Go or Chess.
Learn to use AI like a Pro
Get the latest AI workflows to boost your productivity and business performance, delivered weekly by expert consultants. Enjoy step-by-step guides, weekly Q&A sessions, and full access to our AI workflow archive.
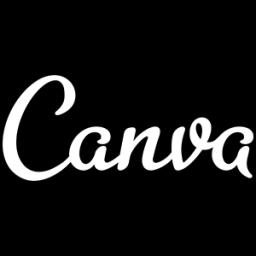
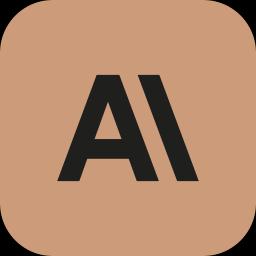
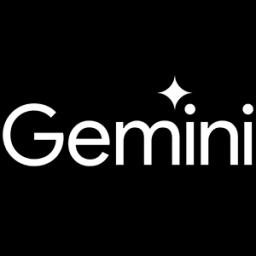
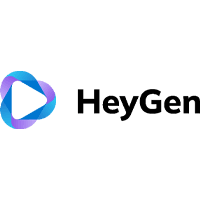
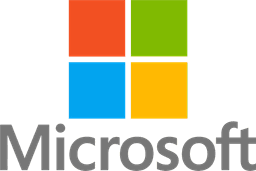
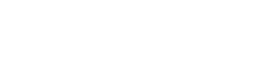
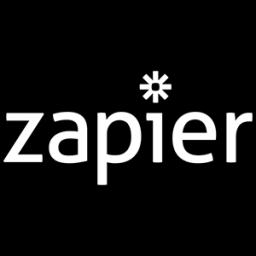
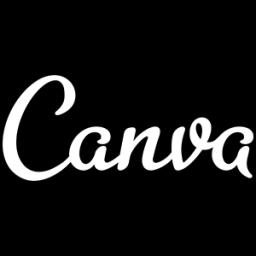
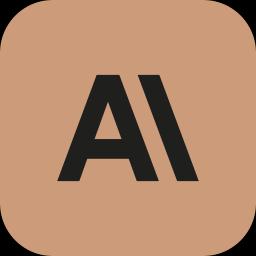
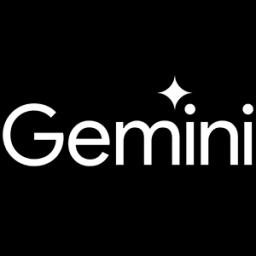
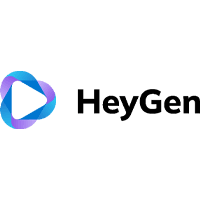
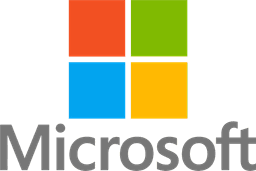
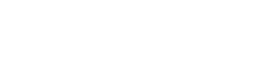
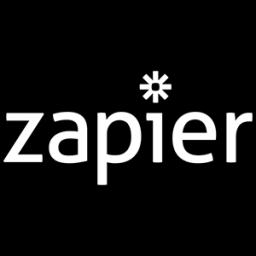
Another promising research direction is the exploration of partially known or dynamic environments. Unlike the deterministic settings used in the MIT study, such environments would present the AI with the challenge of dealing with uncertainty and incomplete information, mimicking real-world scenarios more closely. By expanding the scope of AI's testing grounds, researchers might glean insights into how these models can be improved to better anticipate and adapt to unexpected changes.
Moreover, interdisciplinary collaboration could be a significant driver of future research in this area. Combining insights from cognitive science, neuroscience, and computer science might provide a deeper understanding of how to endow AI models with capabilities akin to human comprehension. By demystifying the processes involved in crafting a robust world model, researchers can make strides in creating AI that can be trusted in both everyday applications and critical operations.
Lastly, the pursuit of ethical AI research is a noteworthy direction in itself. As AI models become more integrated into societal functions, ensuring their development aligns with ethical standards and avoids exacerbating existing biases is paramount. Future research should prioritize transparency, accountability, and fairness, aiming to create AI systems that contribute positively to society while mitigating risks associated with their deployment. This aligns with the broader mission of developing technologies that not only enhance human capabilities but also embody societal values.
Collaborative Research Efforts
Research institutions such as MIT, Harvard, University of Chicago Booth, and Cornell have jointly investigated the understanding capabilities of generative AI models, revealing that these AIs often lack a coherent grasp of world principles. Instead of forming true mental representations of the environments they operate in, these models tend to replicate patterns found in data. This collaborative research has introduced comprehensive metrics to gauge AI's understanding beyond mere prediction accuracy, marking a shift towards evaluating its reliability in real-world applications.
These collaborative efforts have highlighted the inadequacy of current generative AI systems in constructing reliable models of reality, as evidenced through practical tests like New York City navigation and Othello. Changes in the environment, even minimal ones like the closure of minor urban pathways, can significantly derail AI's performance, pointing to its crucial gaps in understanding. The introduction of novel assessment metrics — sequence distinction and sequence compression — offers a new lens through which to view AI's capability to recognize different states and understand identical future steps for certain situations.
Collaboration among leading research institutions is paving the way for future directions in AI development, focusing on testing more complex problems and applying advanced metrics to real-world scenarios. Such partnerships are essential for advancing the field, bringing together diverse expertise to tackle the limitations of AI and push towards models that are equipped with true world understanding, ultimately ensuring the reliability of AI applications in scientific and everyday contexts.
Learn to use AI like a Pro
Get the latest AI workflows to boost your productivity and business performance, delivered weekly by expert consultants. Enjoy step-by-step guides, weekly Q&A sessions, and full access to our AI workflow archive.
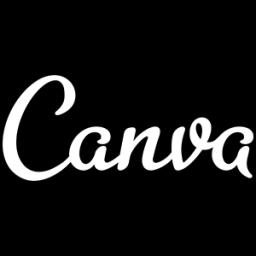
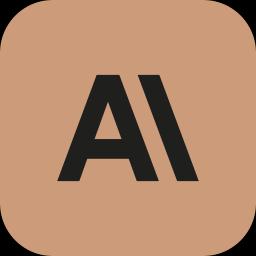
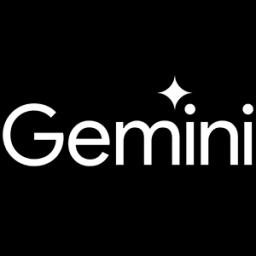
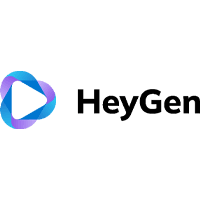
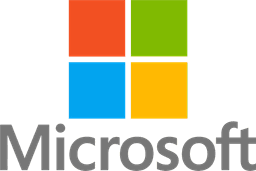
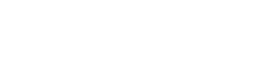
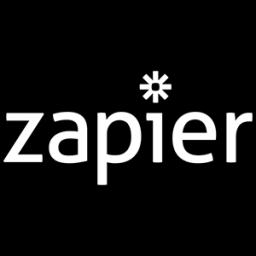
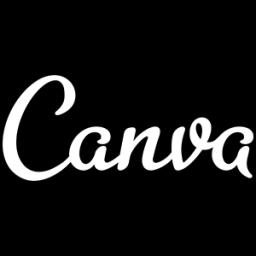
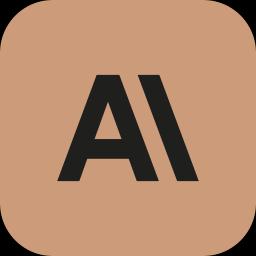
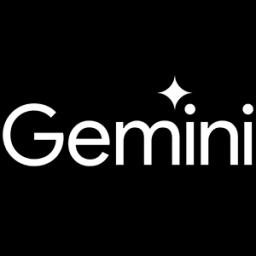
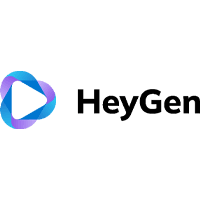
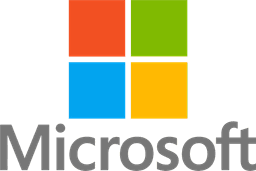
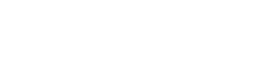
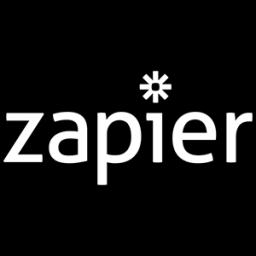
Insights on AI Limitations
In recent studies conducted by MIT, it was revealed that despite the impressive outputs generated by AI models, these models lack a coherent understanding of the real world. This limitation was highlighted through tasks like navigating complex city maps and strategic games, where AI struggled with basic comprehension due to an absence of true internal representations or world models. Such findings prompt critical evaluations of AI beyond mere prediction accuracy, urging a reevaluation of their implementation in real-world context.
To explore AI's limitations more thoroughly, the researchers introduced two innovative metrics: sequence distinction and sequence compression. Sequence distinction measures the AI's ability to differentiate between varied states and scenarios, while sequence compression determines its capacity to recognize consistent next steps given identical conditions. These metrics provide valuable insight into the AI's operational shortcomings and emphasize the importance of developing models that genuinely understand the environments they operate in.
The choice of using New York City's navigation and the game of Othello as testing grounds was deliberate, as they offer rule-based frameworks ideal for assessing AI's comprehension of deterministic environments. The experiment's outcomes revealed that even small environmental changes, such as closing a minimal percentage of city streets, significantly impaired AI performance, showcasing their lack of genuine understanding.
These findings raise essential questions about the future of AI application in dynamic and unpredictable contexts. Without coherent world understanding, AI systems risk inaccurate and unreliable outputs in real-world scenarios like scientific research or complex decision-making environments. Consequently, ongoing research is aimed at developing AI models capable of navigating more intricate problems and integrating these learning metrics into practical, real-world contexts to better grasp AI limitations.
The MIT research signals a pivotal change in understanding AI's role and limitations, pushing for innovations that go beyond data pattern recognition. There's a developing consensus that for AI to be genuinely transformative, it must develop a more profound and meaningful connection to real-world principles. This would require considerable advancements in AI interpretive skills, potentially reshaping how AI is employed across various industries.
Security and Creativity Concerns
The advent of generative AI models has been met with both enthusiasm and skepticism. The recent study from MIT sheds light on significant concerns surrounding the security and creativity of these AI systems. While generative AI has proven its prowess in creating impressive outputs, the lack of a coherent understanding of the world poses security risks, such as prompt injection attacks. This is a vulnerability where malicious inputs might manipulate the system and generate harmful or unintended outputs, which is a serious concern in applications requiring stringent security measures.
Learn to use AI like a Pro
Get the latest AI workflows to boost your productivity and business performance, delivered weekly by expert consultants. Enjoy step-by-step guides, weekly Q&A sessions, and full access to our AI workflow archive.
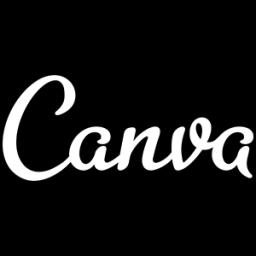
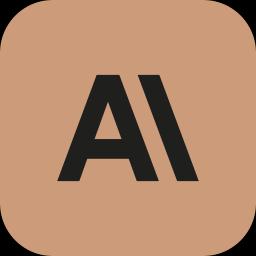
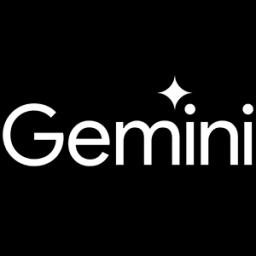
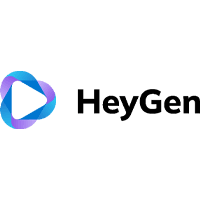
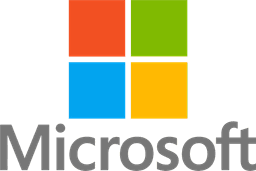
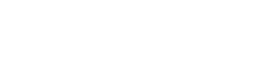
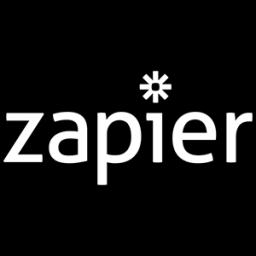
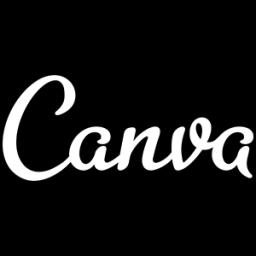
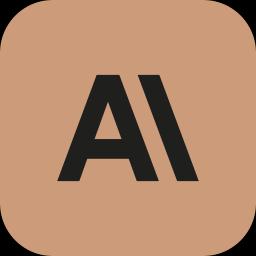
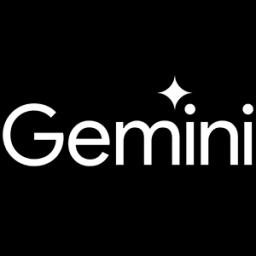
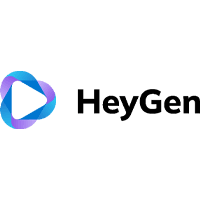
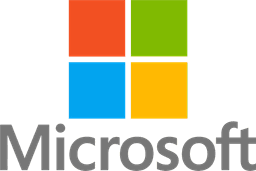
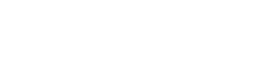
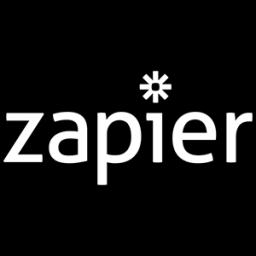
Creativity, another domain often attributed to AI, is also under scrutiny. While AI can generate content that appears creative, the originality of such content is questionable. Generative AI often relies on existing data patterns and lacks the ability to create truly novel ideas or concepts in the way humans do. This raises questions about the extent to which AI can be considered genuinely creative and the implications for industries that heavily rely on creative innovation.
The findings of the study emphasize the necessity for ongoing development in the field of AI to address these concerns. There is a critical need for improved evaluation metrics to assess AI's understanding and creative abilities beyond the mere accuracy of predictions. By enhancing these aspects, AI systems could become more robust, reliable, and ethically sound.
Furthermore, the ethical implications of deploying AI systems without a coherent understanding are profound. As AI continues to integrate into various aspects of life, ensuring its reliability and safety becomes paramount. This calls for stringent ethical guidelines and standards to prevent misuse and ensure AI technology aligns with societal values and needs.
Experts' Opinions
A recent study by MIT reveals significant limitations in generative AI's ability to form a coherent understanding of the world, despite the impressive outputs these models can generate. The research demonstrated that, when tasked with navigating a map of New York City or playing the game Othello, generative AI models often create nonsensical maps with non-existent streets and significantly struggle with minor environmental changes. Such findings indicate that these models do not possess comprehensive internal representations of the problems, necessitating more in-depth assessments of AI functionality beyond mere predictive accuracy. Future tests are set to include more intricate problem sets in scientific contexts to evaluate AI's performance and potential limitations further.
Economic Implications
Generative AI, despite its remarkable outputs, is fundamentally limited in understanding real-world scenarios. A recent MIT study underscores this by demonstrating how AI models falter in tasks such as NYC navigation and Othello, especially when faced with minor environmental changes. This indicates that current AI lacks genuine internal world models or representations, calling into question its reliability outside controlled environments.
The introduction of new metrics, sequence distinction and sequence compression, offers a way to better evaluate AI's understanding of distinct situations and its ability to foresee future steps in identical states. This goes beyond traditional prediction accuracy, heralding a paradigm shift in AI assessment. With these metrics, the study aims to push the boundaries of AI testing to include real-world applications, ensuring the technology's robustness and dependability.
Learn to use AI like a Pro
Get the latest AI workflows to boost your productivity and business performance, delivered weekly by expert consultants. Enjoy step-by-step guides, weekly Q&A sessions, and full access to our AI workflow archive.
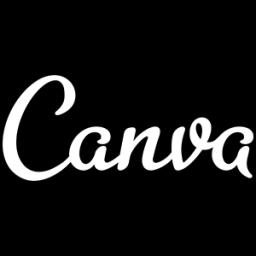
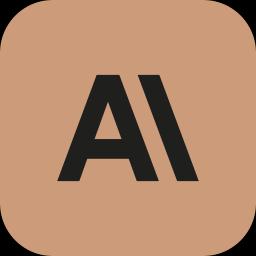
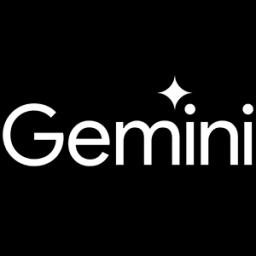
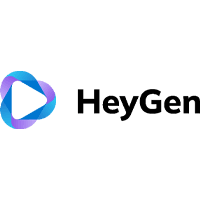
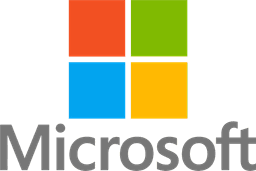
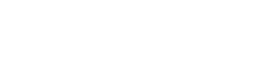
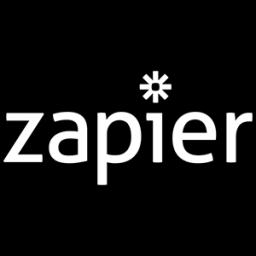
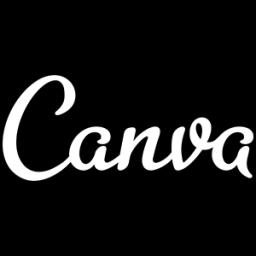
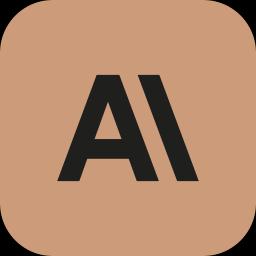
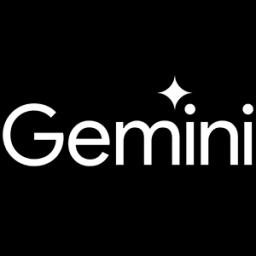
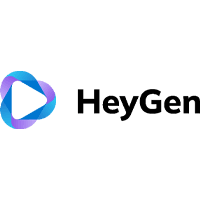
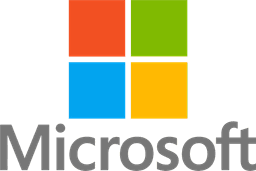
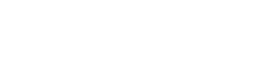
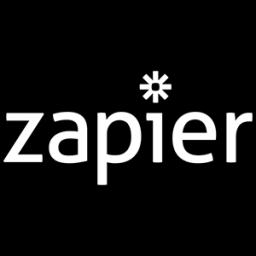
The choice of NYC navigation and the game Othello as test scenarios was strategic. These environments provide clear, rule-based frameworks that force AI models to demonstrate understanding within deterministic contexts. However, the study found that even slight modifications, such as shutting down 1% of NYC streets, led to significant accuracy reductions in tasks, further illustrating AI's superficial comprehension of such structured settings.
Achieving a coherent understanding of the world in AI is critical not only for scientific inquiry but also for practical applications. The MIT study suggests that without such comprehension, AI applications in dynamic contexts are vulnerable to failure in unanticipated situations. This lack of reliability is particularly concerning in fields demanding high stakes decisions, where AI's incomplete world models could have severe repercussions.
Looking forward, the research opens multiple avenues for AI development. Among these is the potential to address increasingly complex problems featuring partially known rules. Researchers plan to apply the new metrics in diverse scientific and real-world contexts, thereby enriching our grasp of the limitations and capabilities inherent in generative AI systems. In doing so, this could assist in pushing AI boundaries towards more profound and versatile applications.
Social Repercussions
The recent MIT study revealing the limitations of generative AI in understanding coherent world models has significant social repercussions. As AI becomes increasingly integrated into daily life, its current lack of genuine comprehension could undermine public trust in AI-driven technologies. Tasks that people usually rely on AI for, such as navigation or decision-making in rule-based games, may exhibit failures due to the models' inability to adapt to slight environmental changes.
With the realization of these AI limitations, there is likely to be a societal shift in how these technologies are perceived and relied upon. Public awareness of AI's propensity to generate inaccurate results when faced with unexpected changes might lead to more cautious usage, calling into question the reliability of AI systems in critical applications. This awareness may foster a demand for transparency and accountability in AI development and deployment, prompting discussions on ethical AI usage and human oversight.
Furthermore, the study might amplify the dialogue around AI literacy in society. Educating the public, including policymakers, businesses, and ordinary users, about the inherent limitations of AI systems is essential to manage expectations and stimulate informed decision-making regarding AI adoption. By understanding that AI models currently function on pattern recognition rather than true world comprehension, society can better calibrate its integration and anticipation of AI's role in the future.
Learn to use AI like a Pro
Get the latest AI workflows to boost your productivity and business performance, delivered weekly by expert consultants. Enjoy step-by-step guides, weekly Q&A sessions, and full access to our AI workflow archive.
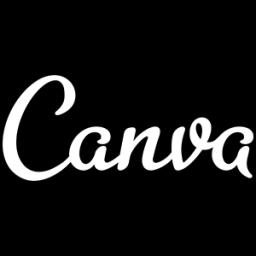
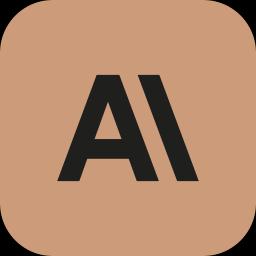
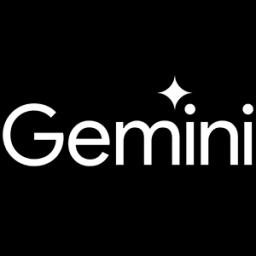
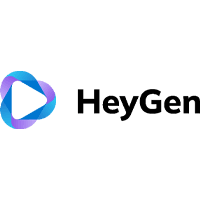
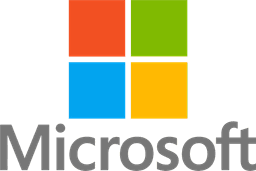
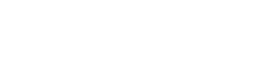
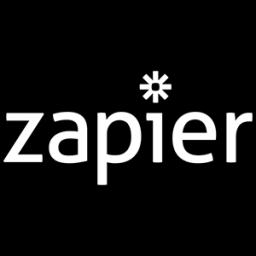
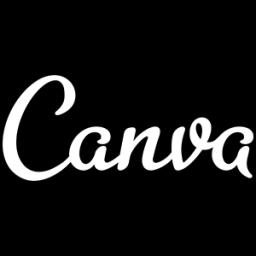
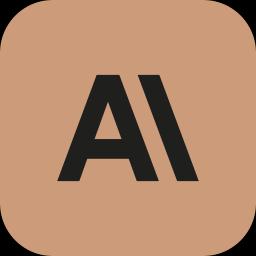
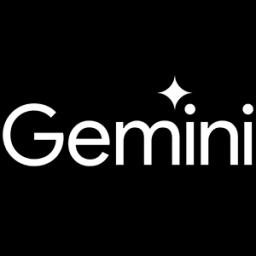
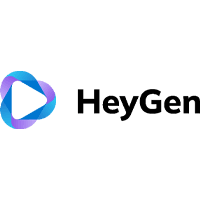
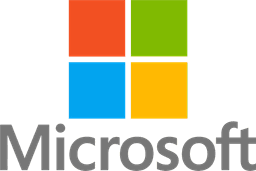
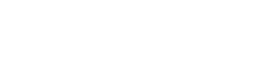
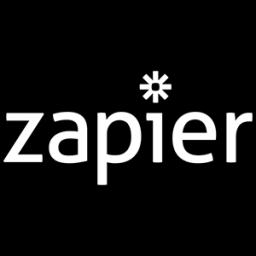
Socially, the impact of such awareness could lead to the encouragement of human-AI collaboration where human intuition and oversight complement AI-generated outputs. This approach could ensure more robust and reliable use of AI technologies, reducing the risk of unchecked automation and potential socio-economic disruptions. Overall, while the study highlights critical limitations, it also presents an opportunity to reshape how society responsibly harnesses the power of AI.
Political and Regulatory Considerations
The evolution of political and regulatory frameworks surrounding Artificial Intelligence (AI) technology continues to be a critical area of focus as AI becomes increasingly integrated into various aspects of society. The recent MIT study showcasing that generative AI models lack coherent world understanding amplifies the urgency for regulatory bodies to ensure these technologies do not inadvertently cause harm due to their limitations.
One of the primary political considerations is the potential enactment of new laws aimed at AI accountability and transparency. As AI models fail to demonstrate true understanding, questions about their use, especially in sensitive or safety-critical situations, are gaining prominence. Lawmakers are under pressure to craft regulations that compel developers to adopt ethical standards and to implement stringent oversight measures to manage AI deployments.
Regulatory bodies are also likely to emphasize the necessity for human oversight in AI implementations. Given the proven deficiencies of generative AI models in comprehending complex, real-world environments fully, regulators might advocate for policies mandating human checks and balances to mitigate erroneous AI decisions. This could mean updates to existing regulatory frameworks or the creation of new guidelines specific to AI technology.
Moreover, globally, the study may spur enhanced collaborative efforts among international AI regulatory authorities to share insights and standardize regulatory approaches. Establishing common grounds on AI reliability and ethical principles can foster synergy, helping nations keep pace with rapid AI developments while ensuring a common baseline for safety and effectiveness.
The broader political discourse will likely include discussions on the geopolitical impacts of AI. As AI becomes a significant driver of economic growth, countries that effectively regulate and deploy AI technologies may gain competitive advantages internationally. Successfully balancing innovation with robust regulation could thus become a geopolitical asset, influencing how countries shape their AI strategies moving forward.
Learn to use AI like a Pro
Get the latest AI workflows to boost your productivity and business performance, delivered weekly by expert consultants. Enjoy step-by-step guides, weekly Q&A sessions, and full access to our AI workflow archive.
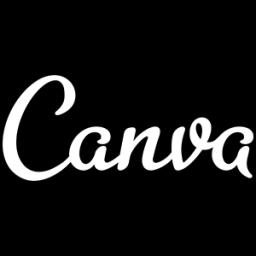
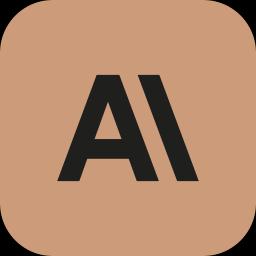
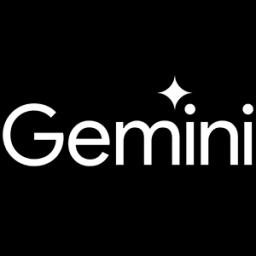
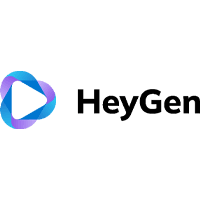
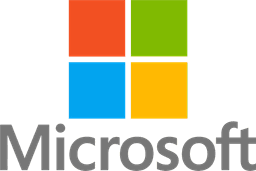
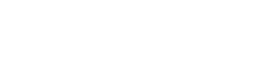
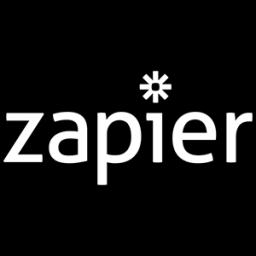
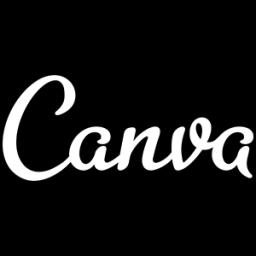
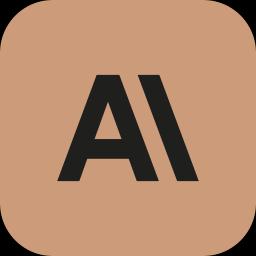
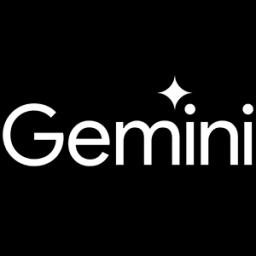
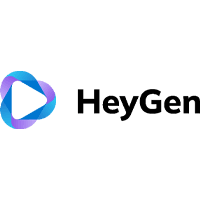
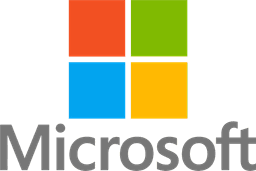
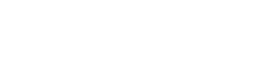
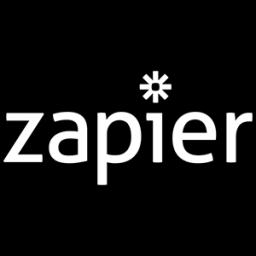