AI Just Got Smol-ler!
Hugging Face Debuts SmolLM2: AI at Your Fingertips with Revolutionary Edge Models!
Last updated:

Edited By
Mackenzie Ferguson
AI Tools Researcher & Implementation Consultant
Hugging Face has unleashed SmolLM2, a series of compact yet powerful language models that are set to reshape AI on your smartphone. Designed to thrive on devices with limited computational power, SmolLM2 offers sizes of 135M, 360M, and 1.7B parameters, surpassing even Meta's Llama 1B in various benchmarks. This game-changer empowers AI deployment on edge devices, reducing reliance on the cloud and cutting environmental impacts, while also democratizing AI access.
Introduction to SmolLM2
SmolLM2 is an exciting innovation by Hugging Face, introducing a cutting-edge series of compact language models tailored for use on devices with limited computational power, such as smartphones. These models are presented in three sizes—135M, 360M, and 1.7B parameters—ensuring they are both efficient and effective for personal device usage. Remarkably, the largest variant with 1.7 billion parameters is capable of outperforming more sizeable models like Meta’s Llama 1B on several performance benchmarks.
The launch of SmolLM2 marks a pivotal moment in AI technology, especially its application on edge devices. With these models, Hugging Face targets reducing the dependency on extensive cloud computing, thus offering a more environmentally friendly and cost-effective alternative. This move is crucial as it addresses major concerns such as computational demand, cost inefficiencies, and privacy, particularly by allowing AI to operate locally on devices where data privacy remains a primary concern.
Learn to use AI like a Pro
Get the latest AI workflows to boost your productivity and business performance, delivered weekly by expert consultants. Enjoy step-by-step guides, weekly Q&A sessions, and full access to our AI workflow archive.
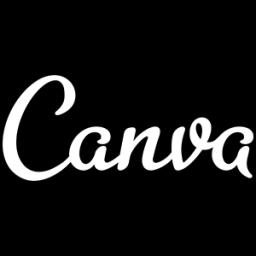
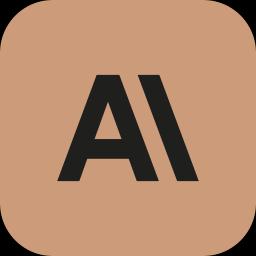
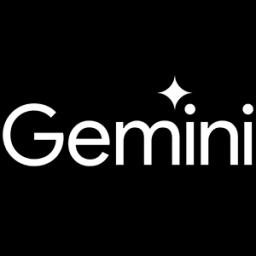
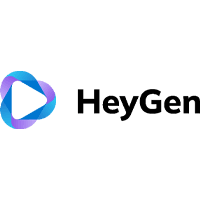
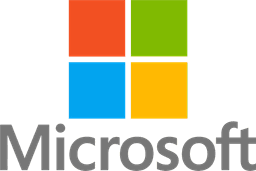
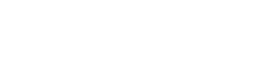
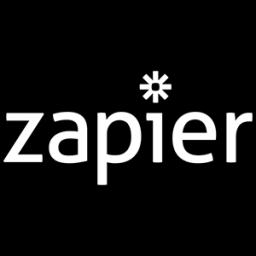
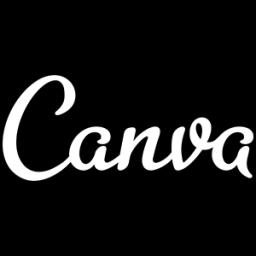
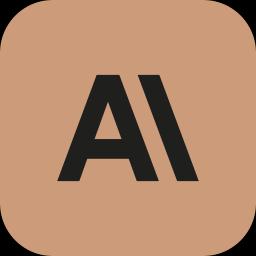
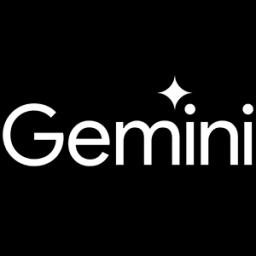
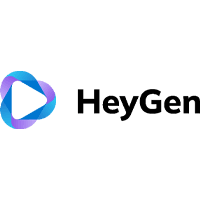
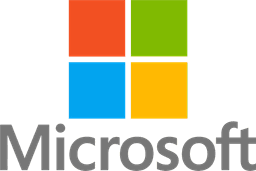
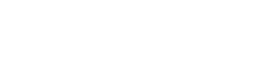
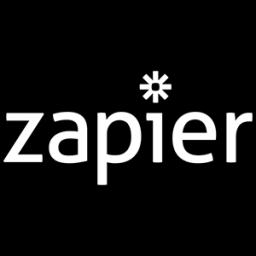
SmolLM2 models unlock vast potential for a multitude of applications. They are particularly suitable for tasks needing text rewriting, summarization, and interaction with various functionalities, making them a valuable asset for mobile apps, IoT devices, and privacy-sensitive industries. These models demonstrate Hugging Face’s commitment to evolving AI capabilities while prioritizing both privacy and user autonomy.
Despite its innovation, SmolLM2 does have its set of limitations. Predominantly focused on the English language, these models might occasionally struggle with factual accuracy and logical consistency, as indicated by Hugging Face itself. Nonetheless, their potential for enhancement through continuous training and development offers a promising future for broader language support and improved accuracy.
Unique Features and Advantages of SmolLM2
Hugging Face's SmolLM2 series presents a unique blend of efficiency and power, redefining the capacity for AI performance on edge devices such as smartphones. These models are distinctively compact, featuring 135M, 360M, and 1.7B parameters, yet they deliver performance that rivals larger counterparts without the need for robust computational infrastructure. This makes SmolLM2 particularly suitable for environments where resources are limited, allowing for effective on-device utilization.
One of the standout advantages of SmolLM2 is its potential to democratize AI access by circumventing the heavy reliance on cloud-based computing. This shift not only reduces operational costs but also significantly enhances privacy, as it limits data transfer to external servers. Such attributes are critical for applications where data sovereignty is a priority, offering practical solutions for mobile and IoT devices across industries focused on privacy compliance.
Learn to use AI like a Pro
Get the latest AI workflows to boost your productivity and business performance, delivered weekly by expert consultants. Enjoy step-by-step guides, weekly Q&A sessions, and full access to our AI workflow archive.
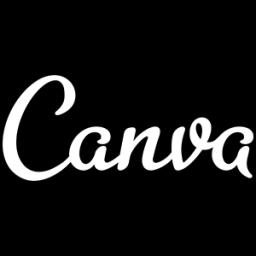
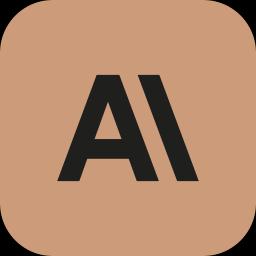
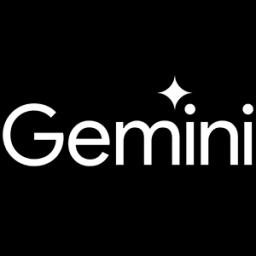
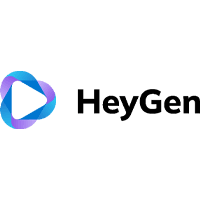
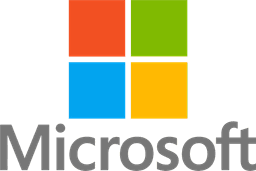
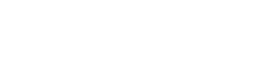
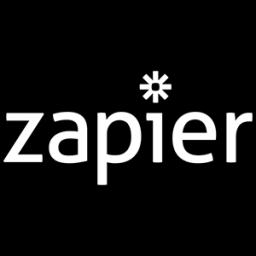
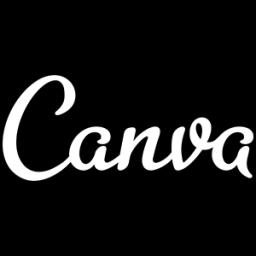
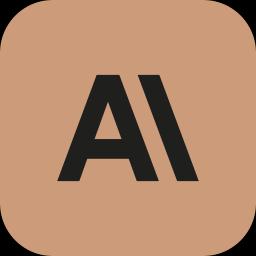
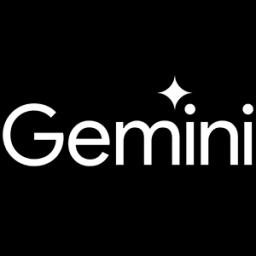
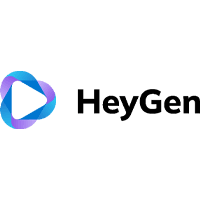
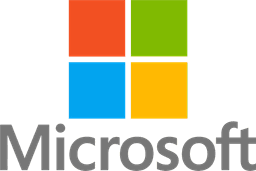
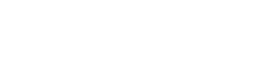
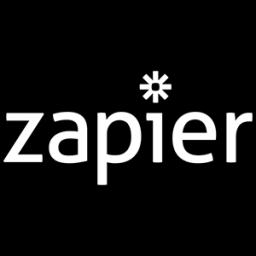
The innovative architecture of SmolLM2 delivers on multiple fronts—efficiency, accessibility, and environmental impact. These models highlight a transition towards more sustainable AI practices by reducing energy consumption typically associated with larger models. This aligns with global efforts to curb the environmental footprint of AI, making SmolLM2 a forward-thinking choice for developers and businesses aiming to foster eco-friendly technology.
Notably, the high performance of the SmolLM2 series, particularly the 1.7B model, matches or exceeds that of larger models like Meta's Llama 1B in various benchmarks. This is achieved through its advanced training on diverse datasets comprising 11 trillion tokens, empowering it with robust instruction-following, reasoning, and mathematical capabilities. Its design philosophy emphasizes the importance of balance between model size and performance, making it a pivotal tool in the era of compact AI solutions.
Importance of SmolLM2's Introduction
The launch of SmolLM2 by Hugging Face marks a significant shift in AI technology, particularly in terms of accessibility and efficiency. The importance of SmolLM2's introduction cannot be overstated, as it offers a powerful alternative to traditional large models that require substantial computing resources. By enabling advanced capabilities on devices as compact as smartphones, SmolLM2 makes AI more accessible to a broader audience. This democratization of AI is crucial in breaking down the barriers that have historically limited access to cutting-edge technology, especially in regions with limited infrastructure.
SmolLM2 represents a response to the growing demand for AI solutions that operate efficiently on the edge, bypassing the need for extensive cloud computing. By supporting advanced AI tasks directly on mobile devices, these models not only reduce latency and operational costs but also enhance data privacy, a significant concern in today's digital landscape. The introduction of these models aligns with the pressing need to address environmental impact by reducing the computational power required to run AI applications, thus promoting more sustainable practices in technology.
The introduction of SmolLM2 also signals a broader industry trend towards developing compact, efficient AI models. This move is set to influence numerous sectors by lowering the entry cost for AI technology deployment, thereby encouraging innovation and widening participation among smaller companies and startups. In addition to economic benefits, the ability of SmolLM2 to maintain performance without sacrificing privacy could lead to more widespread acceptance and utilization of AI in sensitive and regulated industries, such as healthcare and finance.
Ultimately, the debut of SmolLM2 by Hugging Face is a pivotal development in the ongoing evolution of AI technology. It not only highlights advancements in creating models that are both powerful and resource-efficient but also emphasizes the critical role of AI in shaping future technological landscapes. As SmolLM2 and similar models gain traction, they pave the way for more inclusive and privacy-friendly AI innovations, which are essential for holistic growth and adaptation in the AI field.
Learn to use AI like a Pro
Get the latest AI workflows to boost your productivity and business performance, delivered weekly by expert consultants. Enjoy step-by-step guides, weekly Q&A sessions, and full access to our AI workflow archive.
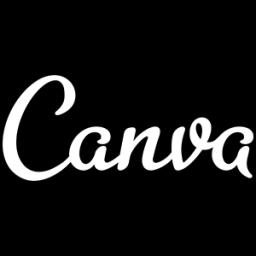
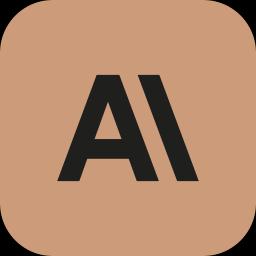
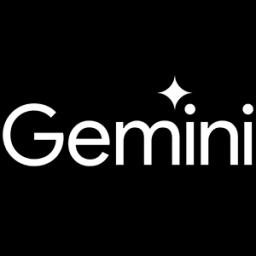
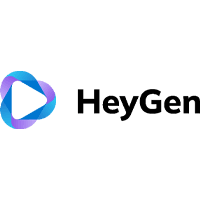
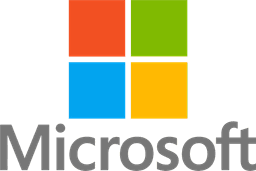
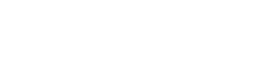
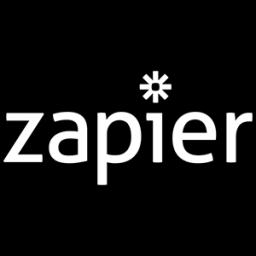
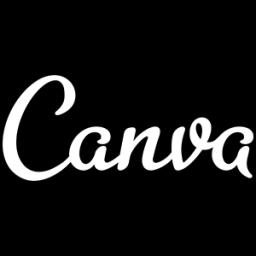
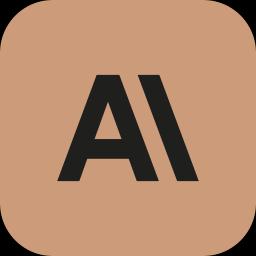
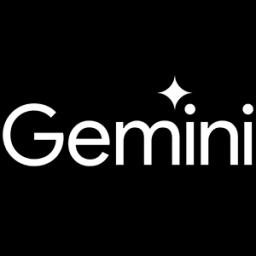
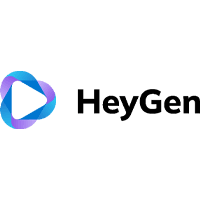
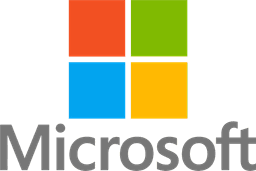
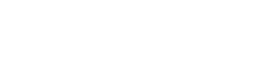
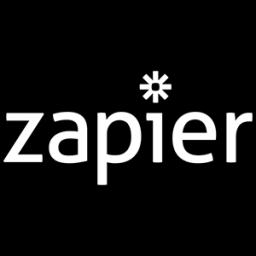
Applications Supported by SmolLM2
The SmolLM2 models, recently introduced by Hugging Face, have been instrumental in supporting a variety of applications across different platforms. Due to their compact and efficient nature, these models are particularly well-suited for tasks that can be processed directly on edge devices such as smartphones and IoT devices, without the need for heavy cloud infrastructure.
One of the primary applications powered by SmolLM2 is text processing, which includes functionalities such as text rewriting and summarization. These tasks are essential for a wide range of mobile applications that require real-time processing and quick turnaround.
Another area where SmolLM2 has proven beneficial is in function calling capabilities, allowing applications to integrate AI-driven features seamlessly. This can be particularly useful in contexts where real-time data processing is crucial, such as in smart home devices or wearable technology.
Furthermore, the resource-efficient nature of SmolLM2 models supports privacy-centric applications, where data processing occurs locally, significantly reducing the risk of data breaches and unauthorized access. This feature is especially important for industries that handle sensitive information, like healthcare and finance.
Besides these, edge applications such as real-time language translation and virtual assistants are also tapping into SmolLM2's potential, offering services that are not just responsive but also protect users' privacy by minimizing cloud data transfers. Across these applications, SmolLM2 stands out as a tool that combines efficiency with cutting-edge AI performance.
Limitations of SmolLM2 Models
One of the notable limitations of SmolLM2 models is their predominant focus on the English language. Despite their efficiency, these models may offer limited performance when applied to other languages, which could restrict their applicability in multilingual contexts. This limitation highlights a broader challenge in the field of language models: the need to cater to an increasingly diverse global audience while maintaining linguistic accuracy and nuance.
Learn to use AI like a Pro
Get the latest AI workflows to boost your productivity and business performance, delivered weekly by expert consultants. Enjoy step-by-step guides, weekly Q&A sessions, and full access to our AI workflow archive.
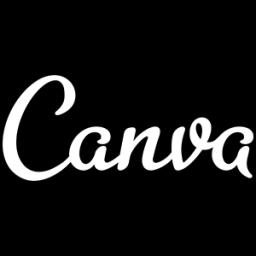
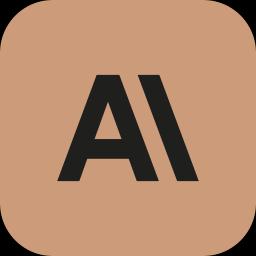
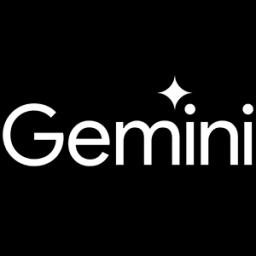
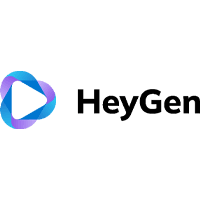
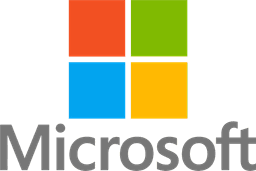
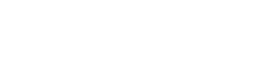
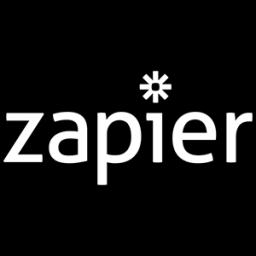
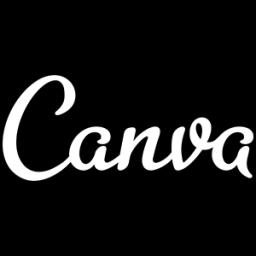
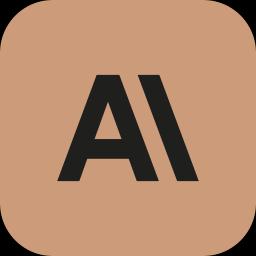
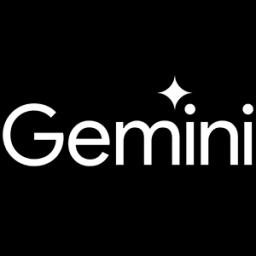
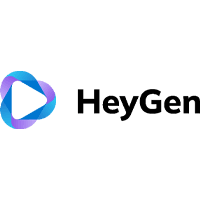
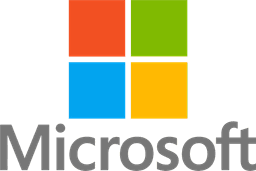
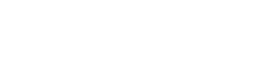
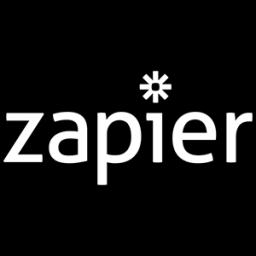
Moreover, although SmolLM2 models exhibit impressive performance for their size, they are not infallible. Users and experts have pointed out occasional issues with factual accuracy and logical consistency in the outcomes generated by these models. This can pose challenges in applications where precision and reliability of information are critical, such as in educational tools or automated customer support systems.
In addition, while SmolLM2 models are designed to operate efficiently on devices with limited computational capacity, this very feature presents its own set of limitations. The reduction in model size, though beneficial for edge computing, may also lead to a compromise in the depth and breadth of understanding when dealing with complex tasks or questions that require extensive knowledge or reasoning capabilities.
The emphasis on making SmolLM2 models resource-efficient and suitable for on-device use addresses environmental concerns, yet it also underscores a trade-off in computational capacity. This trade-off might be reflected in lower-tier model configurations, which, although compact and efficient, might not be able to perform at the same level as larger cloud-based models in extremely demanding scenarios.
Finally, the effort to democratize AI through compact language models such as SmolLM2 comes with the challenge of ensuring these models continue to improve in handling diverse applications without increasing reliance on large computational infrastructures. This includes overcoming existing language and logic constraints and possibly extending the model's capabilities to include more languages and improved contextual comprehension.
Comparison with Other Models
The AI industry is currently experiencing a profound transformation with the rise of compact models like Hugging Face's SmolLM2 series. These models highlight the technological leap towards edge computing, making substantial AI power available on devices with limited processing capabilities, such as smartphones. SmolLM2's various sizes, specifically the most significant 1.7B parameters iteration, provide a pragmatic approach to deploying AI without the over-reliance on cloud infrastructures. This shift not only promotes cost-efficiency and reduces environmental impact but also aligns with the growing consumer demand for more private, secure AI solutions. Compare this with other established models, and SmolLM2 stands out for its efficiency in high-demand tasks such as instruction following, mathematics, and sophisticated reasoning, marking it as a viable competitor against larger models like Meta's Llama 1B.
Although Meta's Llama 1B has set benchmarks in AI for its robust capabilities, SmolLM2 outperforms it in specific evaluations, indicating a paradigm shift in AI deployment strategies. SmolLM2's efficiency without significant computational resources means broader accessibility for developers and businesses, especially those targeting mobile and edge applications. This advantage becomes clearer when explored alongside Mistral AI's Ministraux models, which also focus on edge optimization with their sizable 3B and 8B parameter models.
Learn to use AI like a Pro
Get the latest AI workflows to boost your productivity and business performance, delivered weekly by expert consultants. Enjoy step-by-step guides, weekly Q&A sessions, and full access to our AI workflow archive.
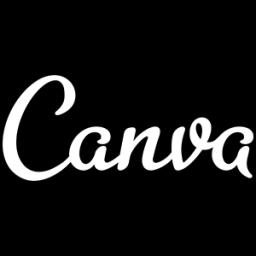
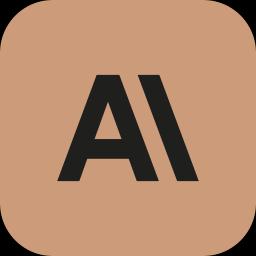
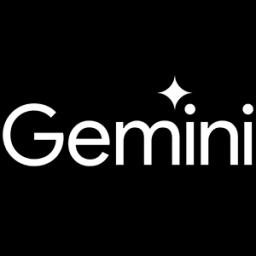
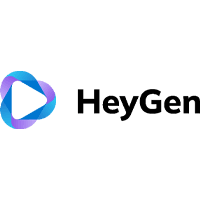
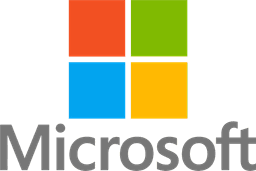
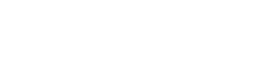
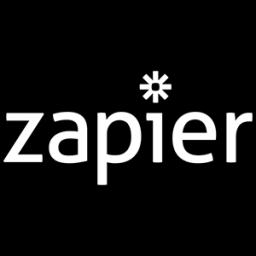
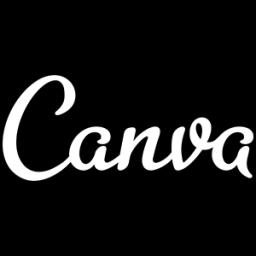
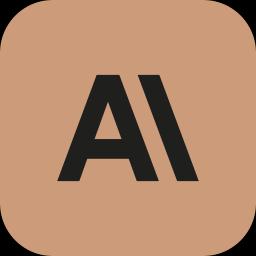
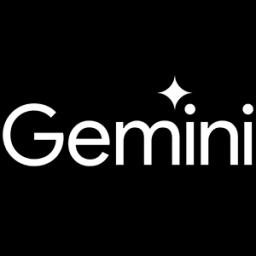
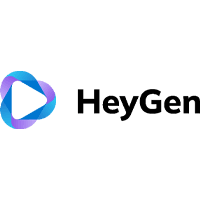
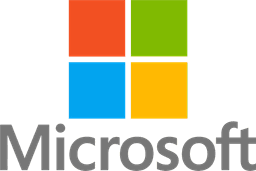
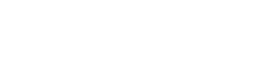
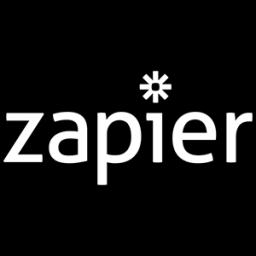
Other significant players emphasize the movement towards resource-efficient AI solutions, such as the partnership between DigitalOcean and Hugging Face, aimed at democratizing AI through easier model deployment. Such collaborations underscore a shared industry goal to make AI more inclusive and practical for smaller entities that might lack extensive computational resources.
Despite SmolLM2's advancements, it faces competition from models like Mistral AI's Ministraux. The latter, with its larger parameters, pushes the envelope in delivering high-performance computing power to edge devices, emphasizing minimal latency and enhanced privacy. In this battle for compact yet robust AI, SmolLM2 and Ministraux both drive a narrative towards empowering less resource-intensive devices without sacrificing capability.
The trend towards efficient AI models is reflective of broader industry and societal changes. As environmental awareness heightens, the push for smaller, efficient models becomes more prominent. This movement is a response to the substantial carbon footprint of traditional, large-scale AI models that demand significant energy consumption. Therefore, SmolLM2 not only serves technological interests but also the planet's environmental welfare by emphasizing resource efficiency.
Public and expert reception of SmolLM2 points to a growing trust in Hugging Face's technology, particularly due to its impressive performance compared to traditional large models. Experts like Vignesh Kumar acknowledge the model's exceptional capabilities tailored for specific tasks, which although primarily in English, still sets a precedent for future multicentric linguistic developments within compact models. Public feedback further recognizes its potential to democratize AI, especially in areas lacking robust computing infrastructure.
The performance and accessibility of SmolLM2 herald a future where AI is more integrated across various facets of daily life. With the promise of reduced costs and increased privacy protections, such models are paving the way for novel applications, spanning from healthcare to education and beyond. Nevertheless, addressing language constraints and striving for factual precision remain crucial areas for improvement, ensuring the benefits of SmolLM2 are maximized for global AI use.
Environmental and Economic Impact
The recent introduction of Hugging Face's SmolLM2 represents a significant progress in AI model architecture, particularly for its environmental and economic implications. As technology continues to advance, the demand for computational efficiency becomes even more critical. Traditional large language models generally require substantial cloud computing resources, contributing to high energy consumption and significant carbon footprints. SmolLM2, with its compact design, offers a much-needed alternative that can operate directly on edge devices like smartphones. This shift reduces reliance on centralized cloud infrastructure, leading to lower energy use and environmental impact, marking a pivotal move towards more sustainable AI solutions.
Learn to use AI like a Pro
Get the latest AI workflows to boost your productivity and business performance, delivered weekly by expert consultants. Enjoy step-by-step guides, weekly Q&A sessions, and full access to our AI workflow archive.
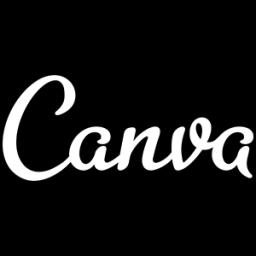
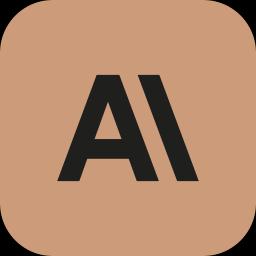
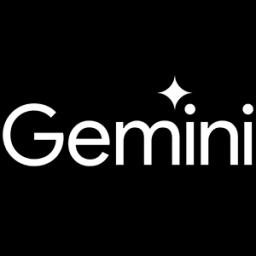
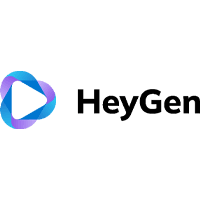
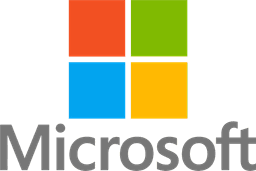
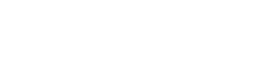
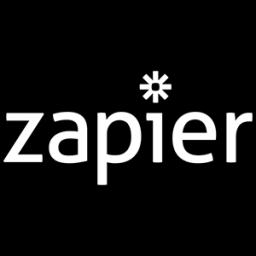
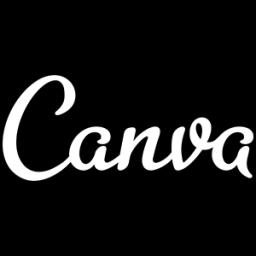
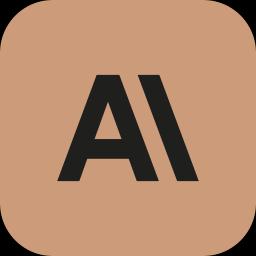
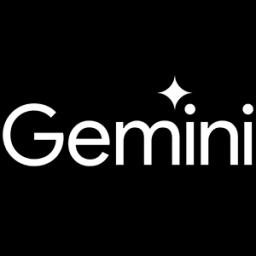
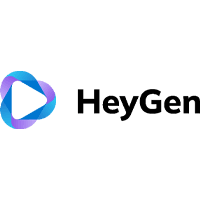
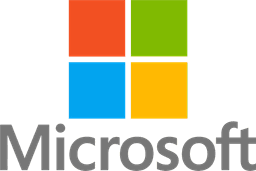
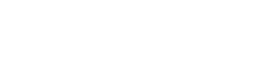
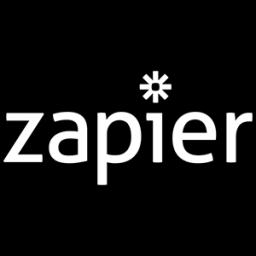
Economically, the deployment of lightweight models like SmolLM2 can lead to notable cost reductions for businesses investing in AI. Since these models require less computational power and cloud infrastructure, organizations, particularly startups and small businesses, can dramatically cut down their operational expenses. This cost-effectiveness not only democratizes access to AI technology but also stimulates innovation by enabling companies with limited resources to leverage powerful AI capabilities. Such democratization has the potential to level the playing field, fostering a more inclusive technological landscape where smaller entities can compete alongside tech giants.
The environmental benefits of SmolLM2 are complemented by its potential economic impact. By minimizing the need for cloud-based processing, these models significantly lower operational costs, making advanced AI applications accessible to a wider range of users and businesses. This kind of technological democratization can drive innovation and competition in the market, as smaller companies can now afford to implement AI-driven solutions without exorbitant expenses. In turn, this can foster a diverse ecosystem of applications that benefit various sectors, from healthcare to finance, ultimately contributing to economic growth and development.
SmolLM2's emphasis on edge computing also aligns with ongoing efforts to enhance data privacy and security. By processing information directly on devices, these models minimize data transfers to external servers, addressing widespread concerns over data protection and sovereignty. As data security becomes increasingly paramount, the ability to keep processing local could enhance trust in AI technologies, encouraging broader adoption across industries sensitive to privacy issues. This could have profound implications for sectors such as healthcare and finance, where data privacy is crucial.
With its reduced environmental impact and economic advantages, SmolLM2 is poised to influence policy and regulatory decisions in the AI domain. As governments and organizations become more conscious of the carbon footprint associated with technology, models like SmolLM2 could set standards for sustainable AI practices. Additionally, by contributing to the broader accessibility of AI tools, these models could shape international policy discussions, encouraging collaborations that prioritize environmental and economic sustainability in AI advancements globally.
Public and Expert Reactions
Hugging Face's release of SmolLM2 has sparked considerable interest among both the public and experts, largely due to its compact size and significant performance capabilities. One particularly commendable aspect is SmolLM2's ability to function effectively on devices with limited computational power, such as smartphones. This groundbreaking capability aligns with the growing demand for efficient AI solutions that do not over-rely on cloud computing resources, mitigating concerns related to latency, costs, and data privacy.
Experts have praised SmolLM2, pointing out that these models, especially the one with 1.7 billion parameters, outclass larger models such as Meta's Llama 1B in instruction-following, knowledge retention, reasoning, and even mathematical computations. Vignesh Kumar, an expert, noted the success of SmolLM2 could be attributed to its complex training on an extensive dataset of 11 trillion tokens. He predicts that the industry will witness more compact models like SmolLM2, which, when fine-tuned, excel in specific tasks. This could potentially lead to the integration of these models into multi-agent systems, enhancing their applicability across diverse scenarios.
Learn to use AI like a Pro
Get the latest AI workflows to boost your productivity and business performance, delivered weekly by expert consultants. Enjoy step-by-step guides, weekly Q&A sessions, and full access to our AI workflow archive.
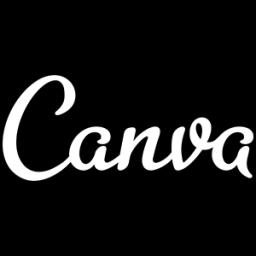
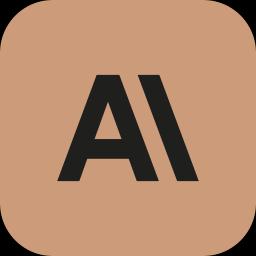
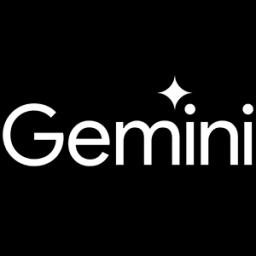
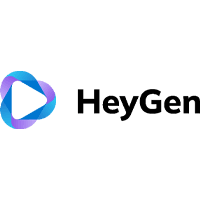
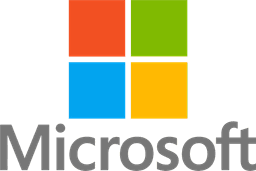
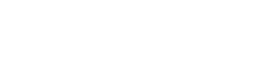
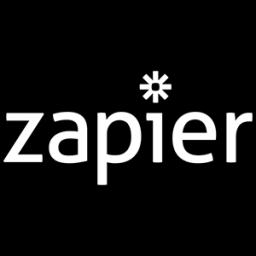
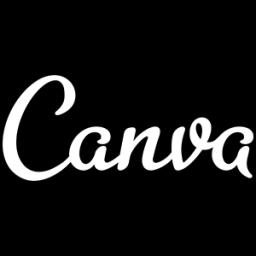
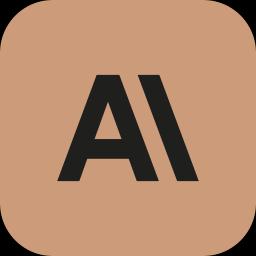
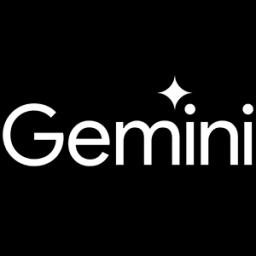
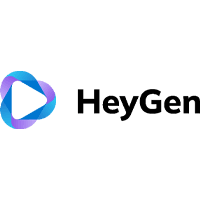
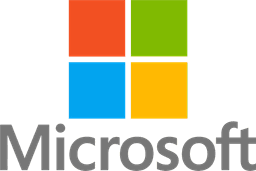
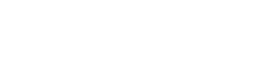
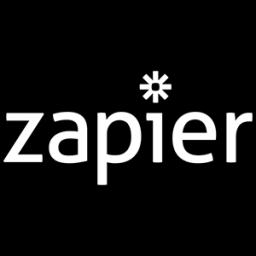
On the public front, reactions on platforms like social media and forums reflect a positive reception. Users were impressed by SmolLM2's performance, especially on benchmarks where it surpasses its larger counterparts despite its smaller size. The efficiency provided by SmolLM2 is viewed as a significant advantage, as it addresses several inherent issues in traditional large models, such as privacy concerns, latency, and the high costs linked to cloud dependencies.
However, there are mixed reactions regarding some limitations inherent to SmolLM2 models. Some users have expressed concerns about the models' language limitations, since they are primarily English-focused. Furthermore, worries about occasional factual inaccuracies in outputs persist. Despite these criticisms, many remain optimistic about the broader implications and transformative potential of these models to democratize AI access, especially in resource-scarce environments. The Apache 2.0 license is highlighted as a catalyst for broader adoption by facilitating experimentation and collaboration among developers and researchers.
Future Implications and Societal Impact
The launch of Hugging Face's SmolLM2, a line of compact language models, represents a significant advancement in the AI industry, particularly in addressing edge AI demands. The models are specifically designed for use on devices with limited computational power, such as smartphones, allowing for high-performance language processing without depending heavily on cloud computing resources. This development not only reduces operational costs but also ensures better privacy management as more data can be processed locally, enhancing user trust in AI technologies.
The societal impact of SmolLM2 and similar models extends beyond mere technological advancement. Economically, by offering AI solutions that require less infrastructure investment, these models can help level the playing field for smaller startups and businesses, democratizing access to deep learning technologies which were previously confined to larger corporations with substantial resources. This shift could lead to a surge in innovation, with more players having the capability to contribute to advancements in various sectors, including healthcare, finance, and education.
In terms of social implications, the ability of SmolLM2 to perform effectively on local devices means greater emphasis on user privacy and data sovereignty. As more data can be processed on personal devices, the risks associated with transmitting sensitive information to cloud servers are significantly reduced. This could lead to broader acceptance and trust in AI technologies, especially in areas where data sensitivity is paramount, such as in medical diagnostics or financial transactions.
Politically, the growing trend towards smaller, efficient AI models may push governments to foster supportive regulatory environments that prioritize sustainability and data privacy. As AI becomes an integral part of various industries, future policy frameworks might need to accommodate these technological shifts, ensuring that privacy concerns and environmental impacts are adequately addressed. Moreover, nations eager to be at the forefront of AI technology might see this as an opportunity to enhance international collaborations while promoting home-grown AI capabilities.
Learn to use AI like a Pro
Get the latest AI workflows to boost your productivity and business performance, delivered weekly by expert consultants. Enjoy step-by-step guides, weekly Q&A sessions, and full access to our AI workflow archive.
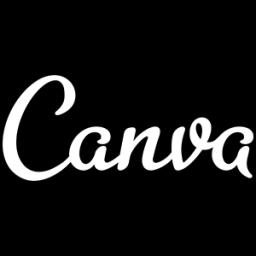
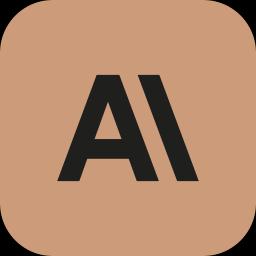
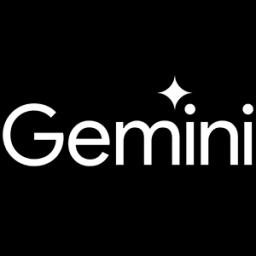
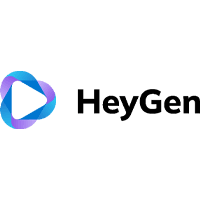
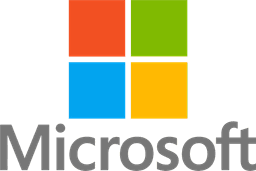
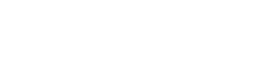
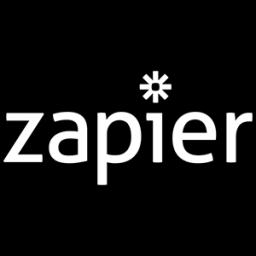
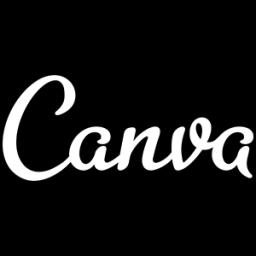
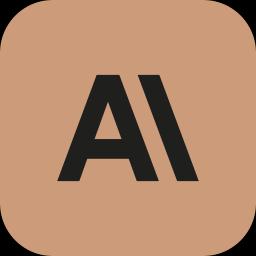
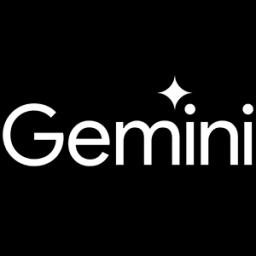
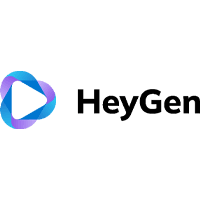
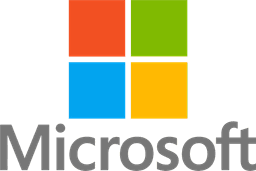
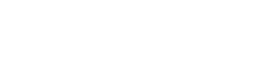
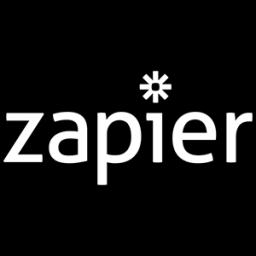
Future implications of models like SmolLM2 also include potential geopolitical shifts. As accessibility to AI tools increases, countries around the world might recalibrate their competitive strategies, focusing on developing domestic AI expertise that aligns with sustainable and efficient technology usage. This could influence the global AI landscape, with collaborative efforts potentially leading to shared standards and practices that promote responsible AI development.