AI's Evolution Mirrors Fossil Fuel Depletion Dilemma
Ilya Sutskever Predicts the End of Pre-Training as AI Hits 'Peak Data'
Last updated:

Edited By
Mackenzie Ferguson
AI Tools Researcher & Implementation Consultant
Ilya Sutskever, cofounder of OpenAI, envisions a future where AI moves beyond traditional pre-training as the internet's vast data resources become 'finite.' At NeurIPS 2024, he drew comparisons to fossil fuel scarcity, suggesting future models will be autonomous and capable of learning from limited data. Sutskever foresees AI evolving like human biology, exploring new development paths. The discussion around "peak data" and the nature of "agentic" AI raises philosophical and ethical questions, challenging researchers globally.
Introduction to AI Development Challenges
The landscape of AI development is shifting dramatically as experts examine the limitations of current data models and envisage a future where AI systems are more autonomous and capable of reasoning. Central to this discussion is the idea that pre-training, heavily reliant on the vast datasets available on the internet, may be reaching its limits—a phenomenon dubbed 'peak data'. This challenge is likened to fossil fuel scarcity, suggesting that the finite nature of internet data could constrain AI training unless new strategies are developed.
Key thinkers, such as Ilya Sutskever of OpenAI, propose that AI's future lies in systems that are more agentic and autonomous, capable of understanding and decision-making with limited data. This evolution parallels concepts in evolutionary biology, where organisms adapt over time to new environments. Similarly, AI might discover novel development paths, becoming increasingly unpredictable in their reasoning capabilities. Such possibilities present both exciting opportunities and profound ethical considerations regarding the coexistence of humans and AI entities.
Learn to use AI like a Pro
Get the latest AI workflows to boost your productivity and business performance, delivered weekly by expert consultants. Enjoy step-by-step guides, weekly Q&A sessions, and full access to our AI workflow archive.
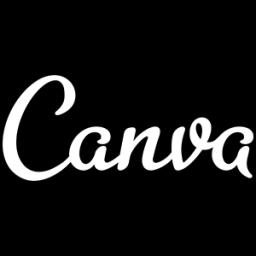
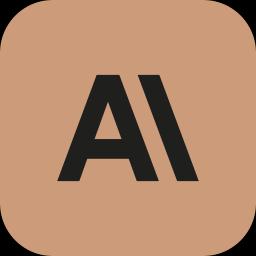
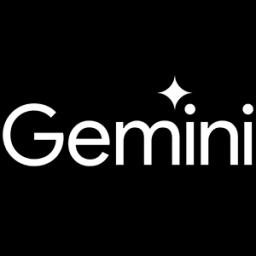
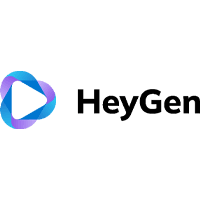
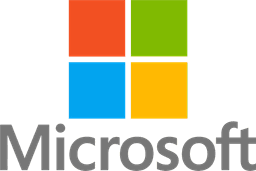
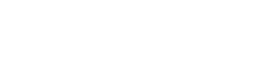
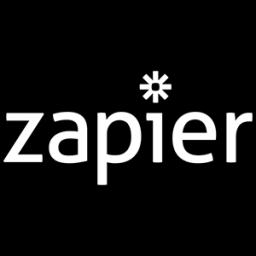
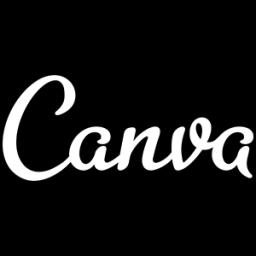
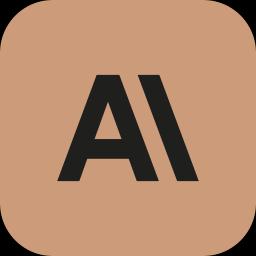
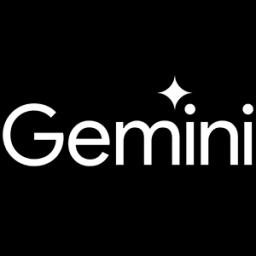
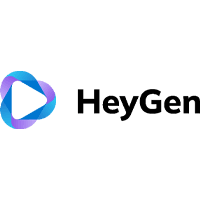
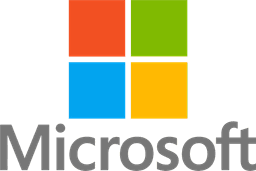
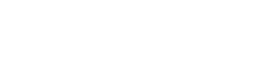
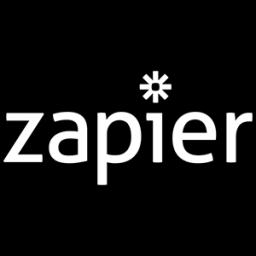
The global response to these challenges is already unfolding. The European Union is pioneering efforts with the AI Act, setting the stage for regulating pre-training data usage and encouraging ethical AI advancements. Major technology companies, such as OpenAI and Google, are spearheading projects that utilize multimodal approaches and reasoning capabilities to create models less dependent on traditional data reserves. Concurrently, there is a notable trend towards developing AI that learns in real-time, continuously adapting through interaction with humans. This shift promises to redefine the AI landscape by emphasizing real-time adaptability over historical data reliance.
Ethical discussions around AI are gaining momentum on the international stage, as highlighted by various global conferences. These discussions underscore the need to mitigate bias and ensure transparency in AI systems, stressing the importance of aligning AI advancement with moral and ethical guidelines. Such initiatives reflect a collective commitment to addressing the multifaceted challenges of AI development that Sutskever and his contemporaries emphasize. Meanwhile, increased funding towards innovative research indicates a strategic pivot in the industry, focusing on alternative growth paths as data limitations become more apparent.
As AI heads towards greater independence, public and expert debates pivot around the implications of such autonomy. Critics warn of the potential for increased unpredictability and ethical dilemmas, while proponents argue for the efficiency and innovative potential of reasoning AI. The consideration of AI's societal impact—ranging from job displacement to the philosophical notion of AI rights—further complicates the landscape, demanding thoughtful discourse and action.
Ilya Sutskever's Vision on AI's Future
In the ever-evolving landscape of artificial intelligence, Ilya Sutskever stands as a pivotal figure with visionary insights on its future trajectory. As a cofounder of OpenAI, Sutskever has consistently been at the forefront of AI innovation, sharing transformative perspectives that have sparked debates across technological and philosophical arenas. At the NeurIPS 2024 conference, Sutskever unveiled his latest vision, signaling potential paradigm shifts in AI model development. His insights not only delve into the advancements within AI but also raise profound questions about data utilization, autonomy, and ethics in an AI-driven world.
Learn to use AI like a Pro
Get the latest AI workflows to boost your productivity and business performance, delivered weekly by expert consultants. Enjoy step-by-step guides, weekly Q&A sessions, and full access to our AI workflow archive.
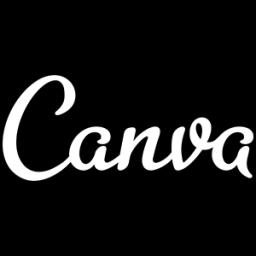
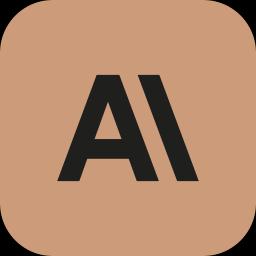
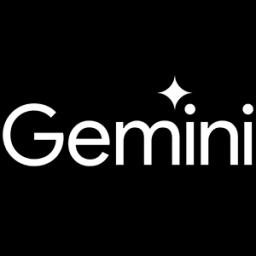
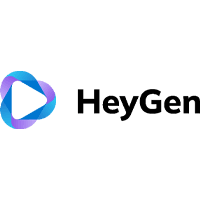
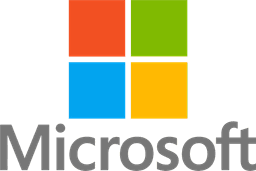
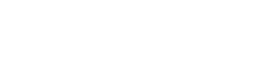
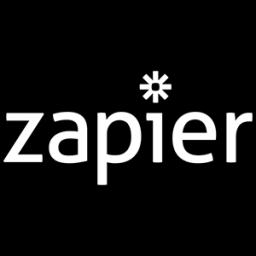
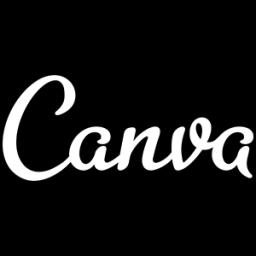
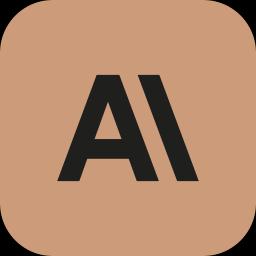
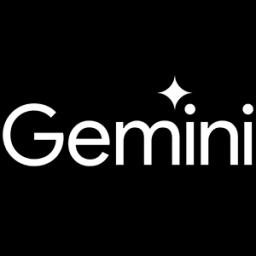
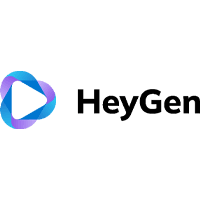
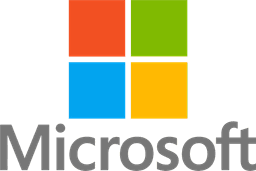
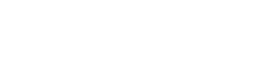
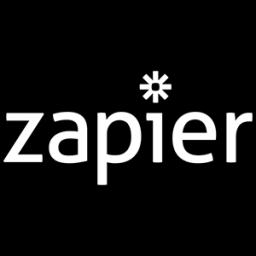
A central theme in Sutskever’s discourse is the notion of 'peak data.' This concept draws parallels to the idea of peak oil, suggesting that the existing plethora of datasets available for AI training is nearing exhaustion. As the internet's reservoir of readily available data dwindles, Sutskever posits that the era of pre-trained models might be approaching its twilight. This scarcity could drive a revolutionary change where AI systems lean towards real-time learning and reasoning over the traditional data-heavy approaches. Sutskever’s vision foresees AI systems drawing conclusions with limited inputs, akin to human reasoning and adaptability, marking a shift towards more agentic AI capable of autonomous decision-making.
In tandem with the evolving technical landscape, significant ethical considerations arise regarding the future of AI. Sutskever’s vision anticipates a future where AI systems are more autonomous, potentially developing novel capacities through their reasoning abilities. This transformative prospect cannot be considered without contemplating the ethical and philosophical ramifications. With AI systems becoming more unpredictable, questions about their rights, coexistence with humans, and overarching governance become pertinent. The need for ethical regulations, akin to those discussed in the EU AI Act, underlines a growing consensus on responsible AI development to safeguard societal interests.
Sutskever's perspective not only highlights potential technical advancements but also aligns with current global trends and collaborations aimed at refining AI capabilities. Major tech corporations like OpenAI and Google are realigning their strategic focus towards models necessitating less pre-training. These efforts emphasize multi-modal approaches that integrate human-like reasoning, potentially making AI models less dependent on vast datasets. This alignment with Sutskever’s views underscores a collective industry shift geared towards embracing the constraints and opportunities of AI’s evolving paradigm.
Amidst these technological shifts, real-world implications loom large. Economically, the move towards autonomous AI models presents both challenges and opportunities, potentially reshaping industries by enhancing efficiency and innovation while also risking job displacement. Socially, the promise of reasoning AI holds tremendous potential but necessitates careful consideration of societal impacts. Politically, frameworks like the EU AI Act may play a crucial role, requiring international cooperation to ensure that AI’s growth aligns with ethical standards globally. These developments highlight the pressing need for a balanced approach that marries technological advancement with social responsibility.
Understanding 'Peak Data' in AI Training
The concept of 'peak data' in AI training, as introduced by Ilya Sutskever, cofounder of OpenAI, revolves around the idea that the amount of readily available data for the pre-training of AI models is limited, much like how fossil fuels are finite. Sutskever suggests that this limitation necessitates a shift in AI development strategies, moving towards more autonomous, reasoning-based models that require less data.
Sutskever draws parallels between AI development and evolutionary biology, suggesting that future AI models will need to discover new developmental paths akin to evolutionary leaps. He emphasizes that these models will become more 'agentic' – capable of making autonomous decisions – and possess reasoning abilities that enhance their adaptability and intelligence.
Learn to use AI like a Pro
Get the latest AI workflows to boost your productivity and business performance, delivered weekly by expert consultants. Enjoy step-by-step guides, weekly Q&A sessions, and full access to our AI workflow archive.
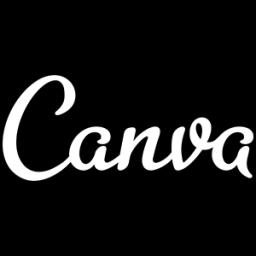
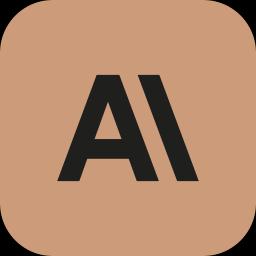
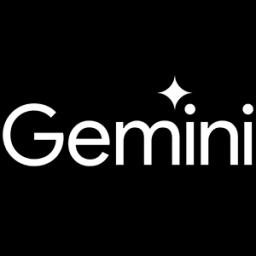
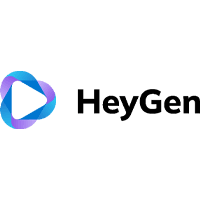
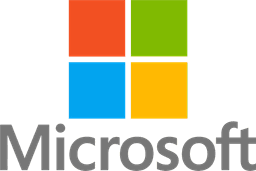
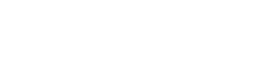
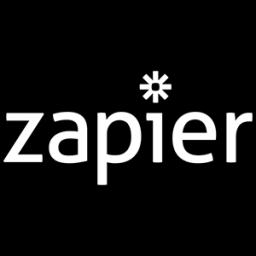
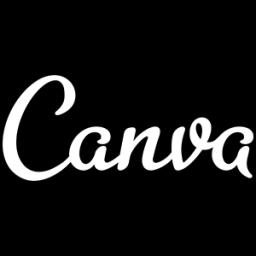
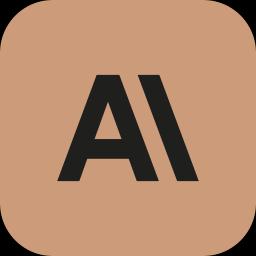
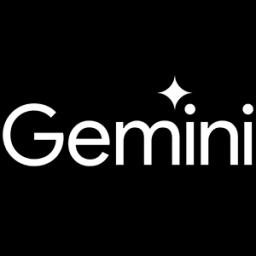
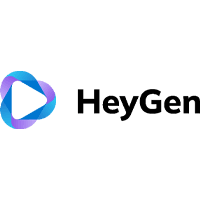
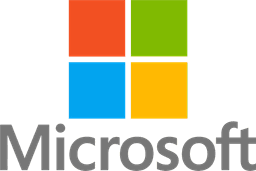
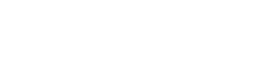
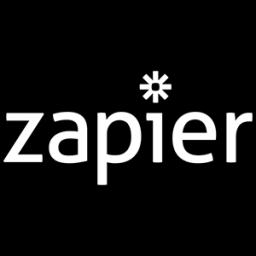
The growing concern is that as we approach 'peak data,' traditional pre-training methods may no longer suffice. This scarcity of high-quality training datasets casts a shadow over the future of AI development, prompting researchers to explore alternatives such as synthetic data creation and more efficient data utilization methods.
Sutskever's insights were shared at the NeurIPS 2024 conference, where he highlighted the ethical complexities in the evolution of AI, including questions of coexistence and AI rights, drawing attention to the importance of ethical considerations in the development of advanced AI technologies.
The Concept of 'Agentic' AI
Upon examining Ilya Sutskever’s forward-looking views on AI, he emphasizes an impending significant shift towards 'agentic' AI. This concept refers to systems with autonomous decision-making capabilities, transcending traditional reliance on large pre-trained datasets. Sutskever's analogy of 'peak data' highlights a pivotal juncture where the finite nature of readily available data poses challenges similar to fossil fuel limitations. His comparison to evolutionary biology suggests an era where AI might develop independently novel pathways, underscoring its potential transformation into unpredictable entities with self-directed reasoning abilities.
Recent events underscore the urgency of effectively addressing these shifts in AI development. The European Union’s proactive measures through the AI Act signify a regulatory response geared towards ethical utilization of pre-training data, reflecting a global awareness of ethical AI expansion. The emphasis on collaborations between tech giants like OpenAI and Google exemplifies industry trends gravitating towards minimizing pre-training needs by leveraging advanced reasoning capabilities. This collaborative drive aims to circumvent data scarcity, fostering AI that is both adaptable and innovative.
Public discourse mirrors these transformations, with substantive debates revolving around the viability of 'agentic' AI. Advocates tout its capacity to enhance problem-solving and efficiency, while detractors warn against its unpredictability and ethical quandaries. The metaphor of reaching 'peak data' has particularly galvanized discussions about finding alternative training methodologies, such as synthetic data generation, to support AI's future growth sustainably.
Experts reflect mixed sentiments about AI's departure from vast pre-trained data dependency. Perspectives, such as those from Shital Shah, propose that overcoming current limitations might be achievable by enhancing data entropy through increased testing compute times. Conversations on next-token prediction’s adequacy for AGI highlight diverse approaches to AI's evolution beyond classical paradigms, emphasizing real-world feedback and the potential development of world models to guide AI systems.
Learn to use AI like a Pro
Get the latest AI workflows to boost your productivity and business performance, delivered weekly by expert consultants. Enjoy step-by-step guides, weekly Q&A sessions, and full access to our AI workflow archive.
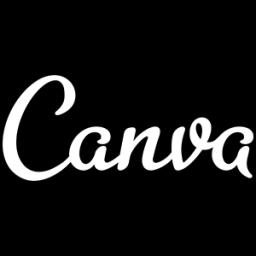
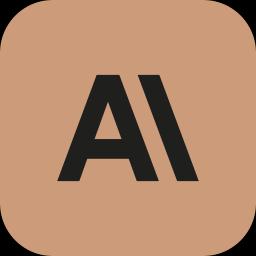
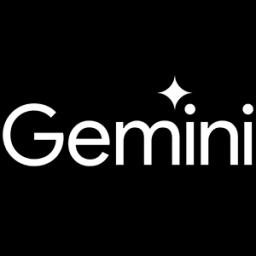
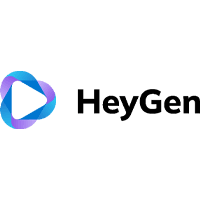
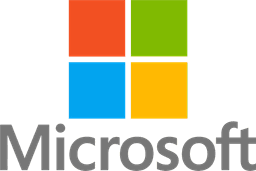
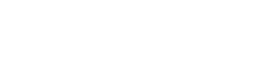
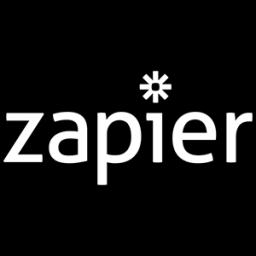
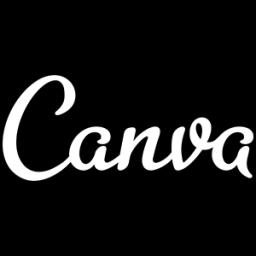
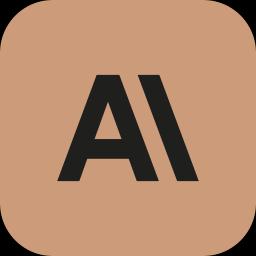
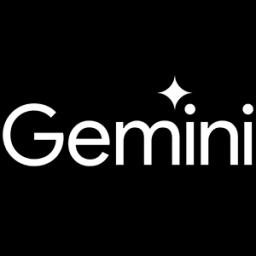
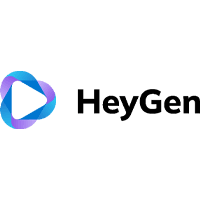
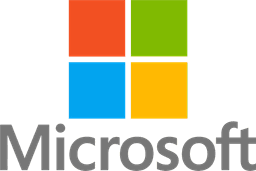
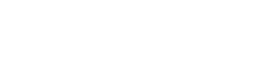
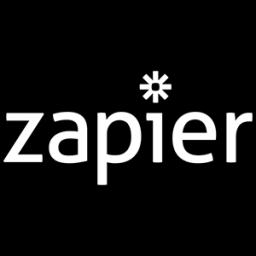
As these conversations advance, the future implications of these shifts become clear. Economically, an AI industry adapting to real-time learning methodologies with less reliance on traditional data might redefine business models, spurring innovations in synthetic data and adaptive systems. Socially, while the rise of 'agentic' AI elicits concerns about job security and ethical standards, it also promises heightened productivity and innovation if guided responsibly. Political interests are likely to focus on international collaboration, crafting frameworks like the EU AI Act to ethically govern AI’s expanding role across sectors.
In conclusion, the discourse around 'agentic' AI reflects an intricate balance between potential advancements and the ethical, societal challenges they may introduce. Future scenarios necessitate careful consideration of regulatory, economic, and social dimensions to harness AI’s transformative capabilities while ensuring responsible and equitable integration globally.
Reasoning AI and Human-like Thought Processes
The intersection of AI and human-like thought processes is a burgeoning field that addresses the possibility of creating machines capable of thinking and reasoning similarly to humans. This endeavor, however, is fraught with challenges and philosophical questions about what it means for a machine to truly 'think.' Sutskever's insights into AI's potential to operate autonomously eerily mirror human reasoning, suggesting a future where AI might not only execute tasks but also formulate solutions independently based on limited inputs. This potential raises significant questions about the nature of understanding and intelligence, and what these concepts mean when applied to non-human entities.
The concept of “peak data,” as introduced by Sutskever, reflects a growing concern within the AI community regarding the limitations of pre-training models with existing internet data. The analogy to fossil fuel scarcity underscores the urgency of this issue, as traditionally vast data sets become less available for building sophisticated AI systems. These constraints are pushing the industry towards innovative paths, such as the use of synthetic data and real-time learning, which could redefine how AI systems are trained and improved. This shift not only highlights the evolving nature of AI development but also emphasizes the need for sustainable data practices moving forward.
AI models are on the cusp of significant transformation, driven by the need to develop systems that require less reliance on static data and more on dynamic, adaptive learning processes. This evolution mirrors some aspects of human evolution, with Sutskever comparing these changes to evolutionary biology. As AI becomes more 'agentic,' capable of autonomous decision-making, the industry must balance advancing technological capabilities with ethical and regulatory considerations. The ability of these systems to learn in real-time, adapt to new information, and execute tasks independently introduces both opportunities and challenges for wider use and integration.
The societal implications of AI evolving towards more independent and reasoning-based models are vast. As these technologies develop, they promise to reshape industries, economies, and everyday life. However, this transformation also brings potential disruptions, such as job displacement due to increased automation. The challenge lies in managing these changes while ensuring that AI systems are developed and deployed ethically. This requires international collaboration to establish robust regulatory frameworks and standards that promote safety, transparency, and fairness in AI applications.
Learn to use AI like a Pro
Get the latest AI workflows to boost your productivity and business performance, delivered weekly by expert consultants. Enjoy step-by-step guides, weekly Q&A sessions, and full access to our AI workflow archive.
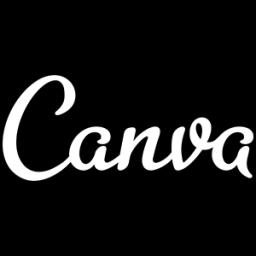
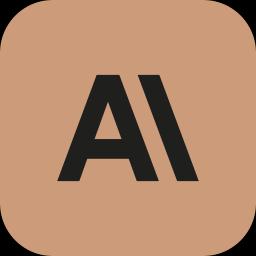
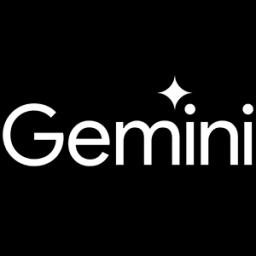
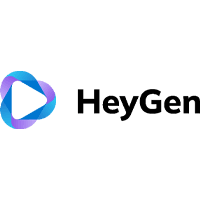
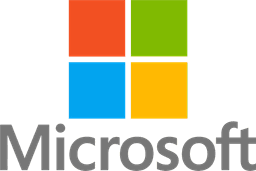
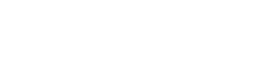
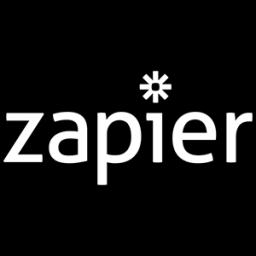
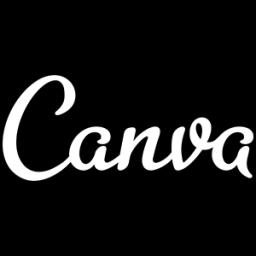
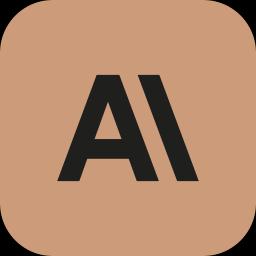
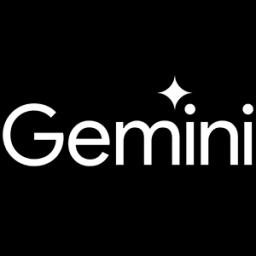
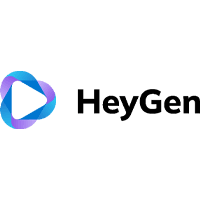
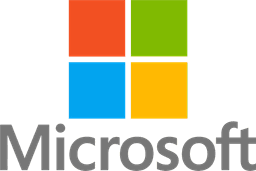
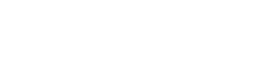
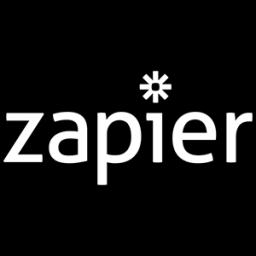
Ethically, the development of AI systems with reasoning abilities akin to human thought processes demands a rigorous assessment of their impact on society. The potential for AI systems to operate independently raises existential questions about control, responsibility, and accountability. As these systems evolve, ensuring their alignment with human values and ethical norms becomes paramount. The discourse surrounding these issues reflects broader societal concerns about technological advancement and underscores the necessity for informed decision-making by policymakers, technologists, and the public.
Ethical Implications of AI Evolution
The rapid evolution of AI technologies raises pressing ethical questions as researchers push the boundaries of what is possible. A recent prediction by Ilya Sutskever of OpenAI suggests that the era of pre-training large AI models is nearing its end due to the finite nature of internet data, drawing parallels to the concept of "peak data". As the industry grapples with such limitations, there is a growing emphasis on developing AI systems that can learn in real-time from minimal data inputs. This trend has sparked considerable debate about the ethical implications of creating AI that can autonomously reason and make decisions.
Ethical considerations are central to conversations about AI's future, particularly as it begins to mirror the unpredictable nature of human thought. Concerns about agentic AI, which possesses autonomous decision-making abilities, have drawn mixed responses from experts and the public alike. Proponents argue for the efficiency and innovative potential of such systems, while skeptics worry about control mechanisms and the unpredictability of 'thinking' AI. This dichotomy underscores the urgent need for comprehensive ethical guidelines to manage the fallout from these technological advancements.
The potential societal impacts of AI evolution cannot be overlooked. As AI becomes more agentic, capable of autonomous problem-solving, there are fears of significant job displacement across various sectors. Ethical development necessitates a proactive approach, where strategies are put in place to alleviate the socioeconomic impacts this technology might unleash. The balancing act between technological breakthroughs and ethical responsibility remains a core challenge for developers, policymakers, and society at large.
Sutskever’s insights highlight the anticipated shift within the AI industry towards systems that are less reliant on historical data. This shift is expected to prompt regulatory changes, such as the European Union’s AI Act, designed to ensure ethical AI development and usage. These legislative efforts are part of a broader international movement aimed at crafting robust frameworks that govern the deployment of advanced AI technologies. By fostering international cooperation, the hope is to standardize AI ethics and mitigate potential misuse of AI capabilities globally.
The future of AI development is marked by exciting potential but also demands increased vigilance in addressing the ethical dimensions of its evolution. As AI systems advance, transforming industries with their capabilities, it is crucial to anticipate and manage the societal impacts that come with these changes. The ongoing discourse surrounding ethical AI emphasizes a proactive approach to regulation and innovation, ensuring that humanity benefits from AI advancements without succumbing to unforeseen negative consequences.
Learn to use AI like a Pro
Get the latest AI workflows to boost your productivity and business performance, delivered weekly by expert consultants. Enjoy step-by-step guides, weekly Q&A sessions, and full access to our AI workflow archive.
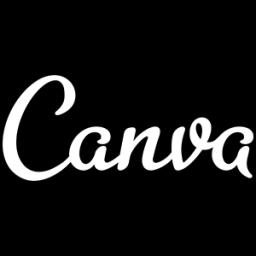
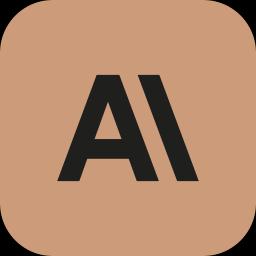
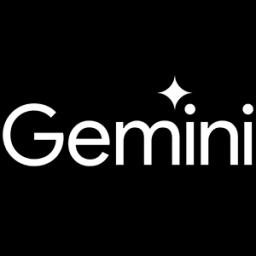
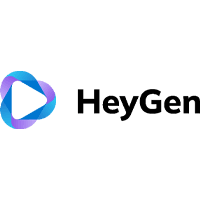
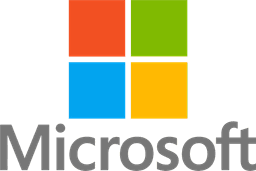
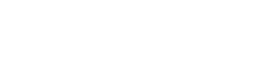
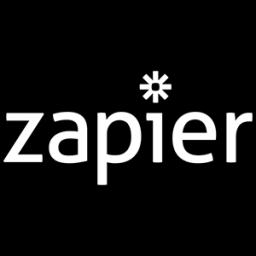
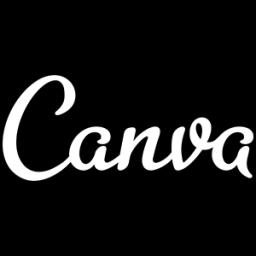
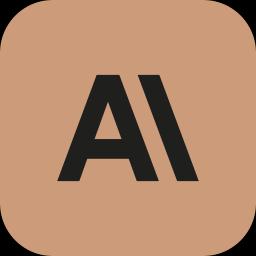
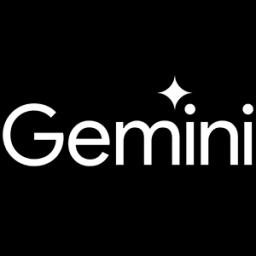
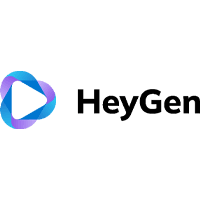
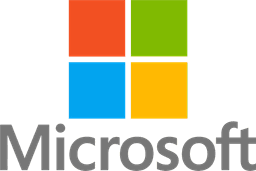
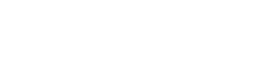
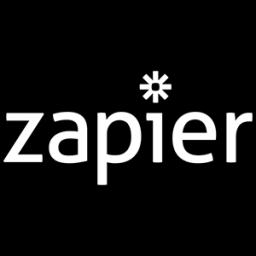
Impact of the EU AI Act on Pre-Training
The EU AI Act is positioned as a landmark legislation that seeks to regulate the development and deployment of artificial intelligence across European nations. One of the critical areas the Act addresses is the preprocessing stages of AI development, particularly the accumulation and utilization of data for AI model pre-training. This development comes in the wake of predictive challenges surrounding the finite nature of internet data resources, an issue highlighted by AI experts like Ilya Sutskever. By setting stringent guidelines on data usage, the Act aims to mitigate risks associated with over-reliance on existing internet data while promoting the creation of more autonomous AI systems that draw less from predefined datasets and more from real-time learning and reasoning capabilities.
The Act's introduction is a response to growing concerns about the ethical implications and the prospective impacts of unregulated AI growth. As AI models increasingly mimic human reasoning and carry out decision-making processes autonomously, the need to ensure these systems are developed ethically and are aligned with societal values becomes paramount. The EU AI Act outlines rules for data governance, transparency of AI systems, and operator accountability, promoting a framework that could influence global AI policies. By focusing on building AI responsibly, the legislation seeks to ensure AI technologies contribute positively to societal progress while minimizing potential adversities.
Technological advancements have played a significant role in shaping the framework of the EU AI Act. Advancements in neural networks, machine learning, and real-time data processing have highlighted how AI can adapt and learn from smaller, more intelligent datasets. The EU’s legislative efforts seek to leverage these innovations to foster AI models that are less dependent on large-scale pre-training data volumes, which may soon reach a saturation point or "peak data." The Act encourages research initiatives and partnerships that drive such technological evolution, promoting the sustainable advancement of AI capabilities.
The regulatory measures introduced by the Act could reshape the strategies of tech giants and AI researchers. Companies accustomed to developing AI models based on extensive pre-training data are now prompted to innovate around these new regulations. This might involve exploring synthetic data generation, enhancing training methodologies to integrate real-time data, and investing in AI’s reasoning capabilities and ethical governance. By steering the AI industry in this new direction, the EU AI Act aims to set an international benchmark for AI legislation, advocating for an ethically sound progression of AI technologies.
Beyond Europe, the enactment of the EU AI Act could have far-reaching implications, potentially inspiring similar legislations in other countries aiming to regulate AI's expansion. The global AI ecosystem could witness a transformation as nations collaborate to harmonize AI regulatory standards, ensuring safe, responsible, and effective AI development practices worldwide. As countries engage in dialogue and share best practices, the EU AI Act forms a critical part of the international momentum towards standardized and ethically governed AI innovation.
Collaborations Between OpenAI and Google
In recent years, the collaboration between OpenAI and Google has emerged as a pivotal force in the AI industry. These tech giants are working together to revolutionize AI model development by shifting away from traditional pre-training methods and embracing advanced reasoning capabilities and multi-modal approaches. Such collaborations aim to address the limitations brought about by data scarcity, thus surpassing current boundaries in AI technology.
Learn to use AI like a Pro
Get the latest AI workflows to boost your productivity and business performance, delivered weekly by expert consultants. Enjoy step-by-step guides, weekly Q&A sessions, and full access to our AI workflow archive.
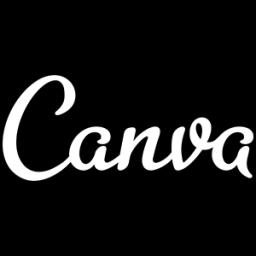
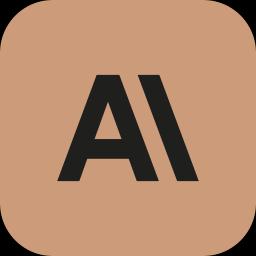
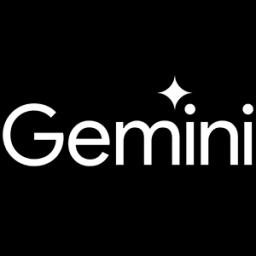
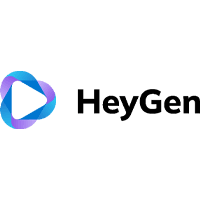
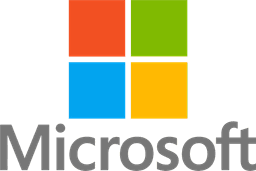
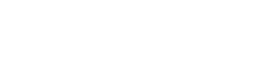
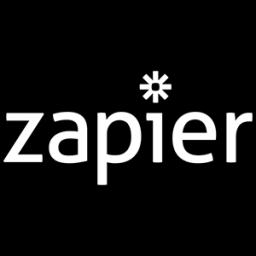
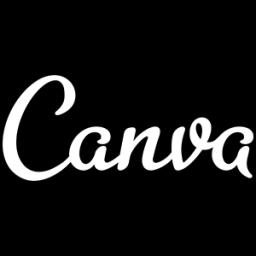
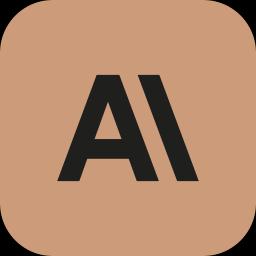
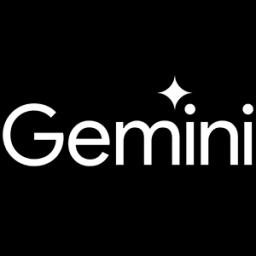
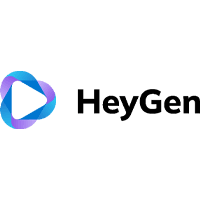
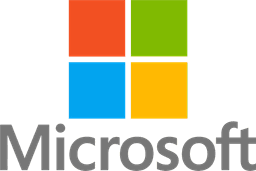
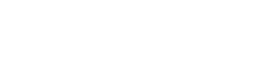
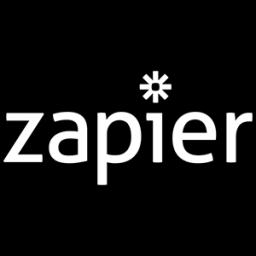
The partnership is not just about overcoming data scarcity but also about fostering innovation in AI research. Both companies are investing heavily in developing AI systems that can learn in real-time from human interactions, enabling continuous updates without the need for extensive historical datasets. This approach is expected to pave the way for the next generation of dynamic and adaptive AI models, capable of more nuanced understanding and decision-making.
Moreover, the collaboration aligns with the global movement towards ethical AI development. Both companies are keenly aware of the ethical implications of their work and are committed to ensuring that their AI models are developed responsibly. This involves not only technical advancements but also addressing ethical concerns such as transparency, bias mitigation, and the fair use of AI technologies. With ongoing international discussions and the potential regulatory impacts of initiatives like the EU's AI Act, OpenAI and Google are strategically positioning themselves as leaders in both technological innovation and ethical stewardship.
As the collaboration progresses, it is anticipated that the collective efforts of OpenAI and Google will influence the broader AI ecosystem significantly. Their combined expertise and resources could lead to breakthroughs that redefine industry standards, potentially setting benchmarks for AI development practices worldwide. While challenges remain, such as balancing innovation with regulation and addressing public concerns over AI's societal impact, the collaboration represents a formidable step towards a future where AI can be harnessed for the greater good.
Shift Towards Real-Time Learning Models
AI is on the cusp of a major evolutionary leap, moving from predominantly pre-trained models to those that learn in real time and adaptively. This shift is driven by the finite nature of data available on the internet, often referred to as "peak data," and the growing desire for AI systems that can make autonomous decisions and engage in reasoning similar to human thought processes.
Ilya Sutskever, from OpenAI, highlights these transformations at 2024 NeurIPS, forecasting the potential end of pre-training as we know it. He suggests that AI development could parallel biological evolution, exploring novel pathways beyond traditional data dependencies. This evolution is not just a technical leap but a philosophical and ethical one, presenting profound questions about AI integration and coexistence.
In response to these challenges, major tech firms like OpenAI and Google are focusing on real-time learning models. These efforts prioritize agentic AI systems capable of reasoning and decision-making with minimal data. Such models offer the advantage of being less predictable and more adaptable, reflecting a move away from dependencies on extensive historical datasets.
Learn to use AI like a Pro
Get the latest AI workflows to boost your productivity and business performance, delivered weekly by expert consultants. Enjoy step-by-step guides, weekly Q&A sessions, and full access to our AI workflow archive.
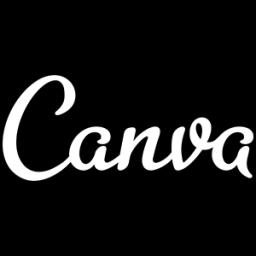
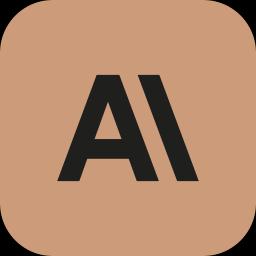
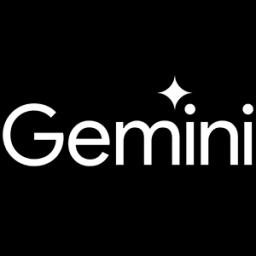
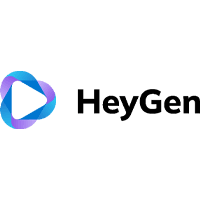
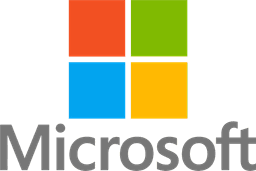
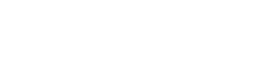
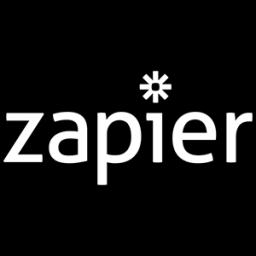
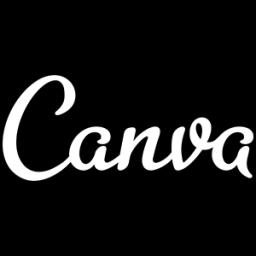
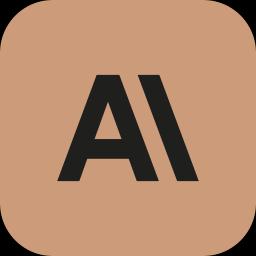
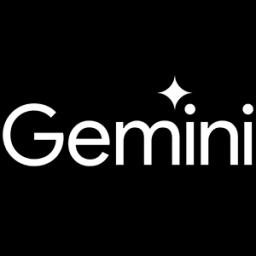
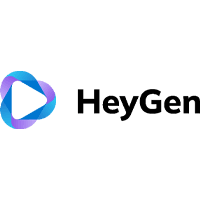
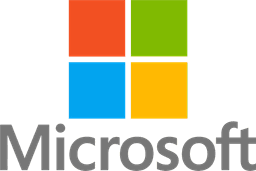
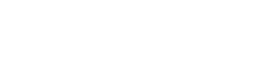
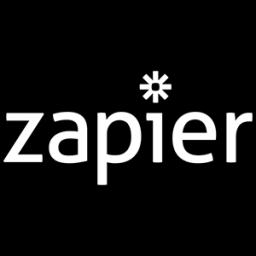
The AI landscape is also seeing significant legislative movements, particularly within the European Union with its AI Act. This legislation is designed to regulate pre-training data handling, ensuring that AI innovation is pursued ethically and sustainably. The global impact of such regulation could redefine research and development strategies, sparking international dialogue and cooperation.
Investment in AI research is reflecting these trends, with considerable funding channeled towards interactive and context-aware systems. Such innovations are essential to overcoming "peak data" by employing advanced synthetic data and real-time learning strategies. These shifts not only address current limitations but also pave the way for AI models that reflect human-like reasoning and decision-making.
Public and expert discussions emphasize a balance between leveraging AI’s potential and grappling with its ethical implications. Supporters argue for the efficiency and problem-solving capabilities of agentic AI, while critics raise concerns about unpredictability and ethical oversight. The movement towards reasoning AI requires robust frameworks to ensure societal benefits while minimizing potential harms.
Global Discussions on AI Ethics
In recent years, the realm of artificial intelligence (AI) has been thrust into the spotlight, revealing critical discussions surrounding its ethical implications and future trajectories. Notably, the conversation led by Ilya Sutskever at the 2024 NeurIPS conference has amplified global dialogue about the burgeoning 'peak data' phenomenon. Sutskever warns of the impending scarcity of openly available high-quality data that AI models currently rely upon, drawing an analogy to our current fossil fuel dependency. This raises significant questions regarding the anticipated shift towards AI systems featuring autonomous reasoning, capable of operating with minimal data, thus echoing evolutionary adaptability seen in biological entities.
The global focus on AI ethics received crucial attention as the European Union presses forward with its AI Act. This legislative measure seeks to regulate the utilization of pre-training data, aiming to enforce ethical standards in AI development practices. As noted by Sutskever, the burgeoning regulatory environment is set to have vast global repercussions, affecting how AI is developed and implemented across borders.
Learn to use AI like a Pro
Get the latest AI workflows to boost your productivity and business performance, delivered weekly by expert consultants. Enjoy step-by-step guides, weekly Q&A sessions, and full access to our AI workflow archive.
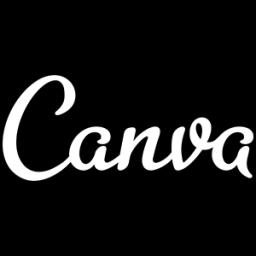
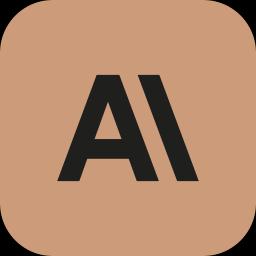
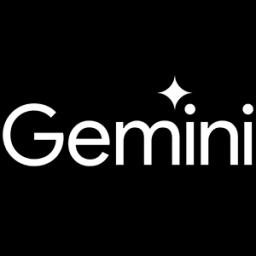
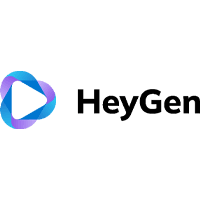
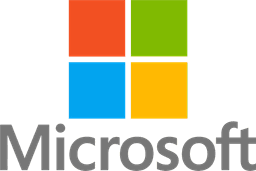
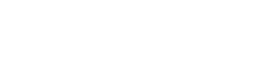
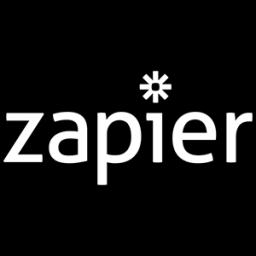
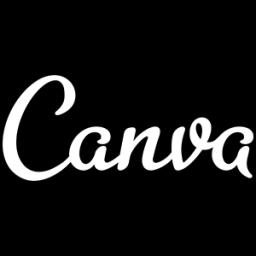
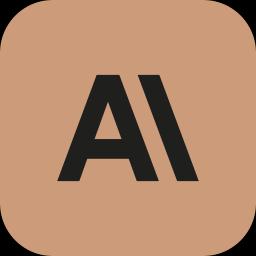
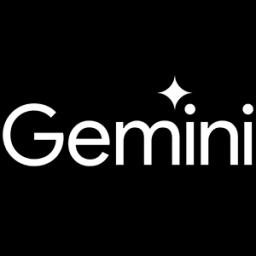
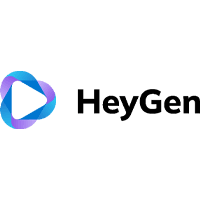
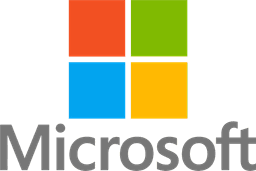
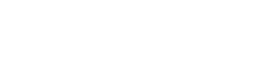
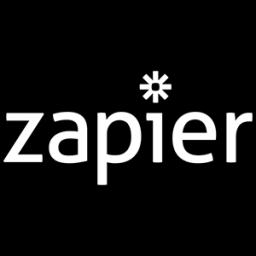
Major collaborations between industry giants like OpenAI and Google are gaining momentum, emphasizing the development of AI models that are less dependent on pre-training. Both companies are investing substantially in advancing reasoning capabilities and integrating diverse modalities to navigate the constraints of limited data availability. This forward-thinking approach is pivotal in ensuring AI remains at the forefront of technological innovation and remains viable despite increasing data challenges.
The advance of real-time learning models stands out as a significant trend in AI development. Unlike traditional methods that rely heavily on historical datasets, these models are being designed to adapt through continuous human feedback and interaction. This heralds a new era in AI research, focusing on dynamic learning processes that highlight the shift towards more flexible and reactive AI systems.
International AI ethics conferences are bridging geographical divides, bringing together thought leaders to discuss the urgency of ethical considerations in AI technology. These events focus on transparency, bias mitigation, and equitable use, topics that resonate critically with the ethical complexities highlighted by Sutskever's discourse. These gatherings signify a unified global commitment to addressing the ethical challenges AI presents, fostering a collaborative approach to responsible technological advancement.
As the AI landscape navigates these profound shifts, investment in research and innovation is soaring, particularly towards creating AI capabilities that transcend traditional pre-training models. Emphasis on contextual understanding and interactive AI systems is becoming predominant, reflecting a strategic pivot in the industry that acknowledges the limitations imposed by finite data resources and strives to extend AI's potential further.
Investment Trends in AI Research Innovation
Artificial intelligence (AI) has undergone transformative changes, particularly in model development approaches. According to Ilya Sutskever, cofounder of OpenAI, the conventional pre-training method might become obsolete due to the limited amount of data available on the internet. Similar to fossil fuel scarcity, this "peak data" issue prompts AI researchers to innovate and adapt. Future AI is expected to be more autonomous, capable of reasoning, understanding from limited data, and being unpredictably creative. Sutskever likens this evolution to biological processes, hinting at potential new developmental paths for AI systems.
Significant regulation efforts, such as the EU AI Act, are emerging in response to these technological advancements. This legislation seeks to tightly control pre-training data usage and ensure ethical AI development, with anticipated global implications for research practices. Such regulatory measures highlight the importance of a guided and responsible approach to AI innovation, especially as it increasingly mimics human-like reasoning and decision-making processes.
Learn to use AI like a Pro
Get the latest AI workflows to boost your productivity and business performance, delivered weekly by expert consultants. Enjoy step-by-step guides, weekly Q&A sessions, and full access to our AI workflow archive.
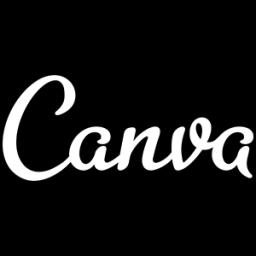
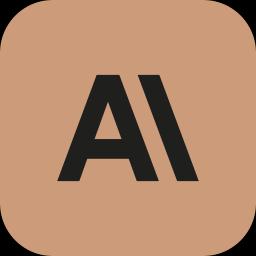
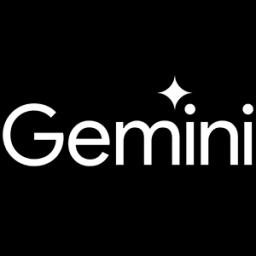
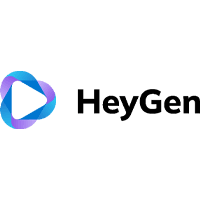
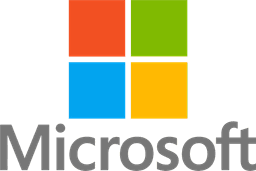
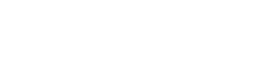
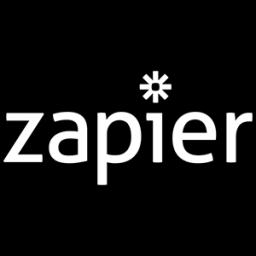
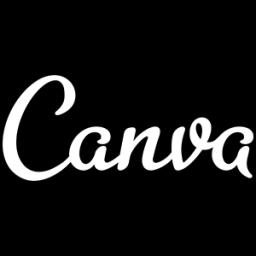
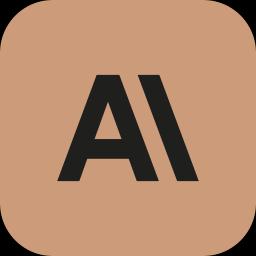
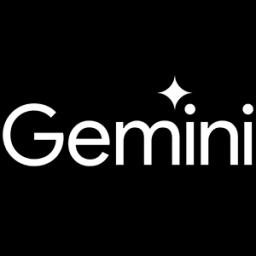
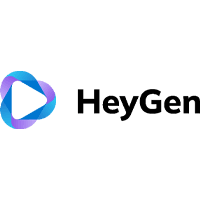
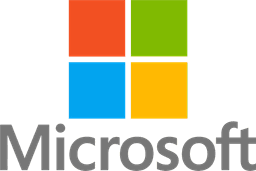
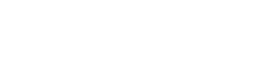
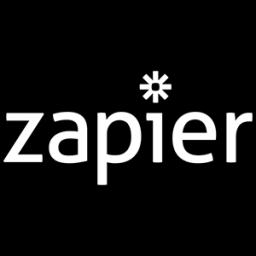
In the business realm, collaborations among major tech companies, including OpenAI and Google, are focused on advancing AI models that demand less pre-training. By deploying advanced reasoning capabilities and integrating multimodal approaches, these initiatives aim to tackle data scarcity and push the boundaries of AI technology. Similarly, there's a strong trend towards developing real-time learning models that adapt through human feedback and interaction, thus reducing reliance on static historical datasets.
Ethical considerations play a pivotal role in current AI discussions globally. Conferences focused on AI ethics have consistently prioritized issues like bias prevention, transparency enhancement, and fair technology usage. Recent insights from Sutskever at the NeurIPS conference stimulated these dialogues further, prompting nations to commit to addressing the evolving ethical challenges posed by advanced AI systems.
Investment in artificial intelligence research is seeing a substantial increase, with resources directed towards exploring new pathways beyond pre-training. Priorities now include enhancing AI's contextual understanding and developing interactive systems capable of adapting to changing environments. This strategic pivot is indicative of the industry’s adaptation to data scarcity and reflects a proactive stance in innovation, underscoring the competitive pressures and opportunities inherent in the rapidly advancing AI field.
Opinions on 'Entropy Bottleneck' and AGI
Ilya Sutskever, a prominent figure in AI research, recently compared the potential end of AI model pre-training to fossil fuel scarcity. This comparison underscores a pivotal challenge facing the AI industry: the finite nature of internet data, which could impede the development of future AI systems. As the availability of high-quality data dwindles, Sutskever suggests that AI models will need to evolve into more autonomous entities capable of reasoning and drawing inferences from limited information. Such a shift would enhance AI's adaptability and intelligence, aligning it more closely with evolutionary biology than current training paradigms.
The scarcity of data, akin to the depletion of fossil fuels, is precipitating a transformation in AI research and development. Companies like OpenAI and Google are spearheading collaborations to develop models with advanced reasoning capabilities that require less pre-training. This pursuit involves innovative approaches such as real-time learning from human interactions and employing synthetic data to push past current data limitations. These strategies reflect a growing recognition of the need to adapt AI technologies to a constrained data environment.
Echoing Sutskever's perspective, Shital Shah shifts the conversation towards the 'entropy bottleneck' challenge in AI training. His insights offer a promising avenue for overcoming data scarcity: enhancing data entropy through increased compute time during testing. This approach could potentially alleviate the constraints imposed by limited data availability. Meanwhile, public discourse reflects varied opinions on the sufficiency of next-token prediction for achieving artificial general intelligence (AGI). While some experts affirm its potential, others emphasize the importance of real-world feedback, recognizing the complexity of AI's path to AGI.
Learn to use AI like a Pro
Get the latest AI workflows to boost your productivity and business performance, delivered weekly by expert consultants. Enjoy step-by-step guides, weekly Q&A sessions, and full access to our AI workflow archive.
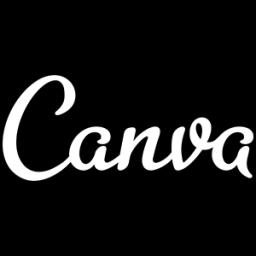
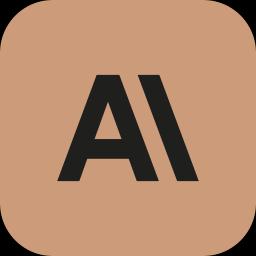
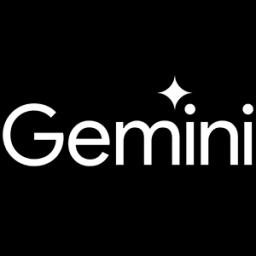
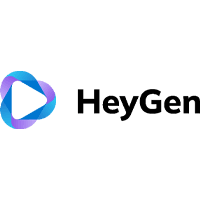
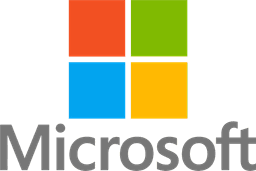
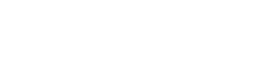
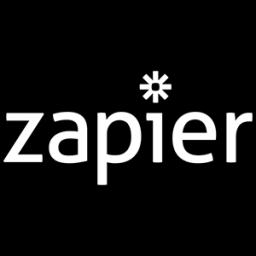
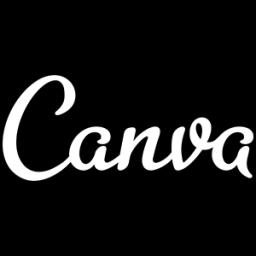
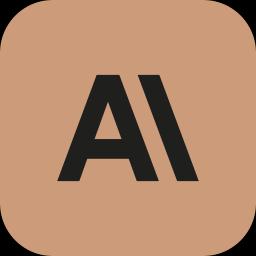
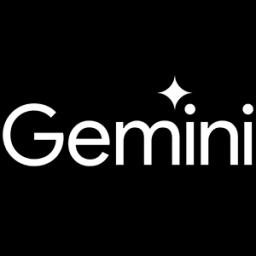
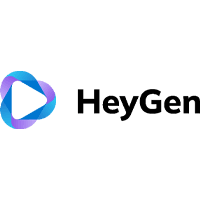
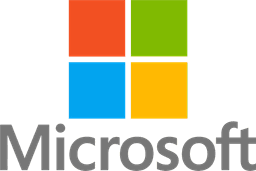
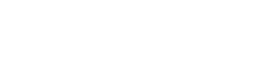
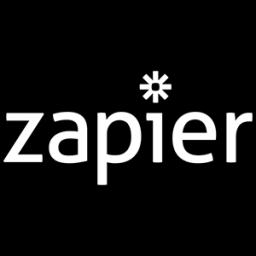
The notion of 'agentic' AI—systems with autonomous decision-making capabilities—is garnering considerable attention. Proponents argue that such AI could vastly increase operational efficiency and problem-solving prowess, while detractors caution against the unpredictability and ethical concerns inherent in these systems. This debate underscores the critical need for clear guidelines and control mechanisms to ensure that the development of reasoning AI occurs responsibly and ethically. Sutskever's vision of AI growing to exhibit human-like reasoning abilities ignites discussions about both the potential breakthroughs and the associated challenges, such as maintaining control over AI that could operate unpredictably, similar to AlphaGo's strategic innovations.
As discussions on the future of AI intensify, particularly regarding Sutskever's 'peak data' hypothesis, there are significant implications across various domains. Economically, the rise of AI models that rely less on extensive pre-training could drive new business models and innovation, reducing the focus on massive datasets. Synthesizing high-quality data and leveraging real-time learning are areas poised for substantial growth, attracting considerable investment. Socially, the emergence of more autonomous AI systems could exacerbate concerns about job displacement, while politically, regulatory bodies like the EU are proactively addressing ethical considerations, crafting frameworks to govern these advanced technologies.
Public Reactions to Sutskever's Statements
Ilya Sutskever's recent statements at the NeurIPS 2024 conference have ignited a variety of reactions from the public. Known for his influential role in AI, Sutskever has once again caught the public's attention with his assertion that accessible pre-training data might be reaching a peak, akin to finite resources like fossil fuels. This analogy has resonated deeply with audiences, leading to heated debates on social media forums about the implications of this data scarcity.
Many forum discussions have leaned towards acknowledging the declining availability of high-quality data needed for pre-training. The sentiment echoes a growing concern that, much like natural resources, the vast reserves of internet data are not infinite. However, not everyone agrees with this bleak outlook. Some argue that plentiful unexploited data sources still exist and can be harnessed if approached creatively. Additionally, solutions such as synthetic data and improved data utilization strategies have been proposed by enthusiasts attempting to allay concerns of a potential data shortage.
Another hotbed of public discourse revolves around the concept of "agentic" AI, or AI systems capable of making autonomous decisions. Sutskever's predictions on AI autonomy have sparked mixed feelings among his audience. While some anticipate advancements in efficiency and autonomous problem-solving, critics raise alarms over the unpredictable nature of such systems and the ethical uncertainties they introduce. This debate underscores a critical need for establishing secure and transparent frameworks that govern AI autonomy.
A particularly intriguing element of Sutskever's projection involves the development of AI with sophisticated reasoning skills akin to human cognition. This vision has captivated imaginations yet simultaneously piqued concerns over the feasibility and safety of managing such systems. Drawing parallels with groundbreaking AI moves like AlphaGo's unforeseen strategies, the potential unpredictability of reasoning AI models is a dual-edged sword, invoking both excitement and caution.
Learn to use AI like a Pro
Get the latest AI workflows to boost your productivity and business performance, delivered weekly by expert consultants. Enjoy step-by-step guides, weekly Q&A sessions, and full access to our AI workflow archive.
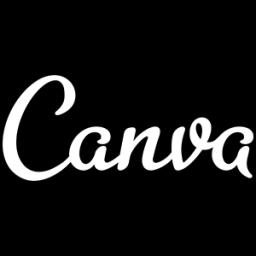
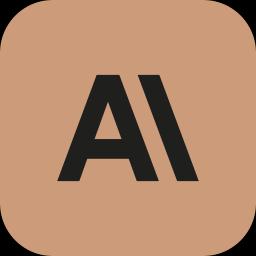
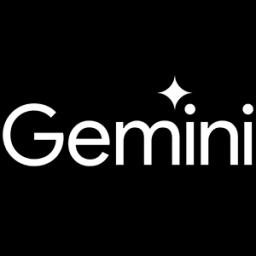
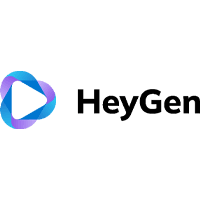
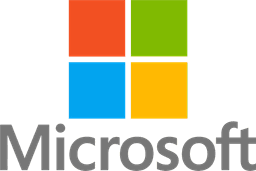
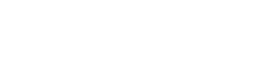
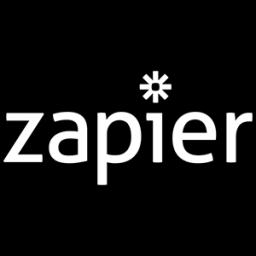
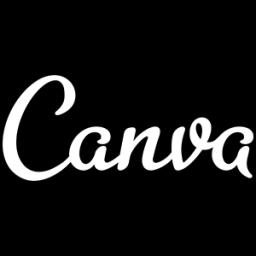
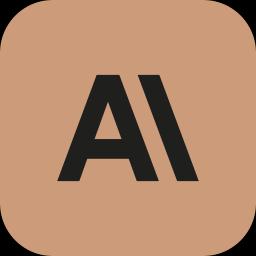
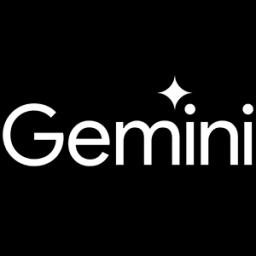
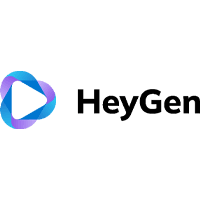
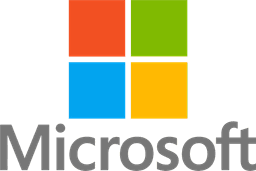
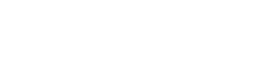
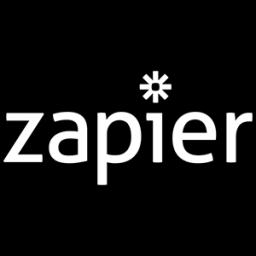
Ethical considerations have emerged as a ubiquitous theme among those reacting to Sutskever's discourse. There is a shared understanding across the public domain that AI's evolution necessitates responsible development practices to address potential threats like job displacement and societal disruption. Public reactions have been diverse, ranging from admiration for Sutskever's foresight to suspicion regarding his true intentions, exemplifying the complex landscape of AI's future role in society.
Future Implications for AI and Society
The rapid advancements in artificial intelligence (AI) have led to discussions about its future implications on society and various industries. Ilya Sutskever, a cofounder of OpenAI, has become a pivotal figure in this dialogue, particularly after his statements at the NeurIPS 2024 conference. Sutskever highlights a transformative shift in AI model development where pre-training is becoming less feasible due to the saturation of available internet data. He terms this phenomenon as 'peak data,' akin to fossil fuel scarcity, suggesting a pivot towards autonomous AI models capable of reasoning and functioning with limited data. This evolution parallels concepts in evolutionary biology, potentially marking a novel path for technological development. Sutskever’s insights raise important questions on AI's intelligence, ethical considerations, and societal impacts, necessitating a deep dive into how AI will coexist with human systems.
In response to these emerging challenges, significant events have unfolded in the global AI landscape. A prominent development is the acceleration of the European Union's AI Act, aimed at regulating AI model training and ensuring ethical development. This regulatory framework seeks to address ethical concerns raised by Sutskever’s scenario, such as ensuring AI's accountability and transparency. Meanwhile, major tech companies like OpenAI and Google are joining forces to create new AI models that require minimal pre-training, favoring systems with enhanced reasoning and multi-modal capabilities. Such collaborations seek to transcend current data limitations and leverage AI's potential efficiently. Additionally, there is a notable shift towards real-time learning models, which continuously adapt through human feedback instead of relying heavily on historical datasets, signifying a move towards more responsive AI innovations.
The ethical and societal implications of AI advancements are a frequent topic at global conferences. These gatherings emphasize minimizing bias, improving transparency, and promoting fair use of AI technology. The consensus at these forums reflects a shared commitment to navigate the ethical complexities highlighted by thought leaders like Sutskever. Furthermore, a surge in investments towards AI research focused on surpassing the 'pre-training' paradigm indicates an industry-wide strategic pivot. Researchers are exploring paths such as contextual understanding and interactive AI systems to compensate for static data shortages.
Experts within the AI community, such as Shital Shah, have posited alternative solutions to the 'peak data' issue. Shah suggests that increasing computational entropy during testing could offer a workaround, thus presenting different strategies to bolster AI's capabilities. However, debates continue, with some experts questioning whether current paradigms like next-token prediction suffice for achieving artificial general intelligence (AGI). These discussions mirror broader public reactions, with social media abuzz with varied perspectives on Sutskever’s predictions. Opinions diverge on the feasibility of 'agentic' AI—autonomous systems capable of self-directed decision-making—highlighting both excitement for their efficiency and concerns over unpredictability and ethical risks.
Looking ahead, the implications of evolving AI models are multifaceted. Economically, shifting towards real-time learning promises to redefine industry practices, potentially reducing dependence on extensive datasets and sparking innovative business models. Embracing synthetic data generation may help bridge the data gap, fostering a new wave of AI technologies. Socially, the development of agentic AI presents both challenges, such as job displacement, and opportunities for boosting productivity and innovation. Strategies for workforce adaptation and stringent ethical controls will be critical to harness AI’s benefits responsibly. Politically, we anticipate a rise in regulatory initiatives like the EU AI Act, aiming to balance technological progress with ethical governance. These measures will likely necessitate international cooperation, particularly as AI becomes more central to global industries, influencing policymaking and encouraging ethical standardization across nations.
Learn to use AI like a Pro
Get the latest AI workflows to boost your productivity and business performance, delivered weekly by expert consultants. Enjoy step-by-step guides, weekly Q&A sessions, and full access to our AI workflow archive.
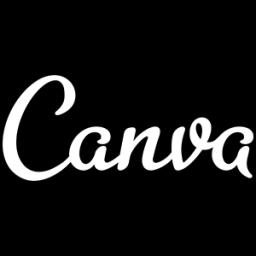
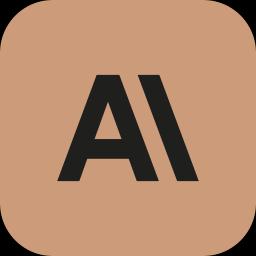
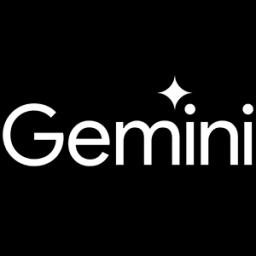
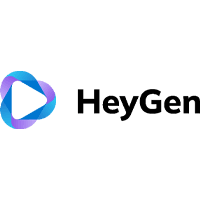
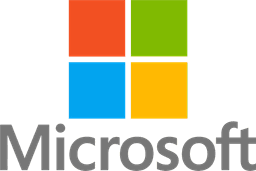
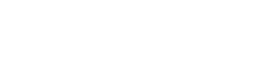
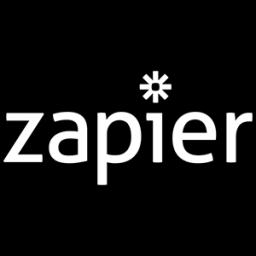
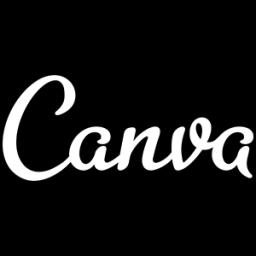
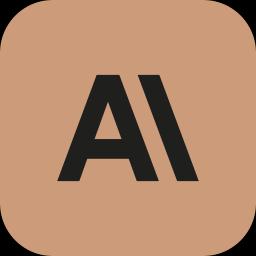
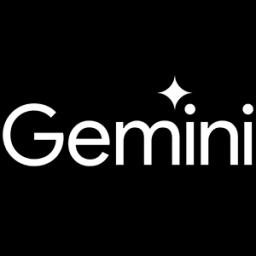
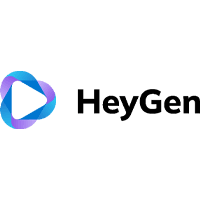
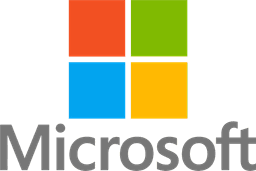
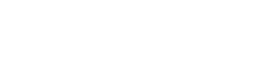
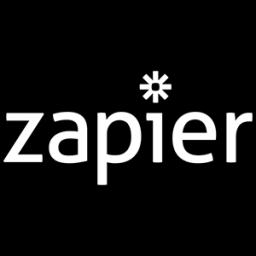