Faster, Smaller, Smarter AI on Your Phone!
Meta Takes AI Mobility to New Heights with Llama Models - Outpacing Google and Apple!
Last updated:

Edited By
Mackenzie Ferguson
AI Tools Researcher & Implementation Consultant
Meta launches compact Llama AI models for mobile, placing powerful AI directly on smartphones and tablets. With quantization techniques, these models offer brilliant performance, minimized memory usage, and enhanced speed. This upends Google's and Apple's cloud-centric strategies, as Meta partners directly with chip makers like Qualcomm to expand AI's reach across Android devices. Welcome a new era of privacy-centric, device-based AI!
Introduction
In recent news, Meta has announced the release of compact versions of its Llama AI models that can function efficiently on smartphones and tablets. This step marks a significant advancement in mobile AI technology as these models are designed to retain high performance while minimizing memory and processing requirements. By employing strategies like quantization, Meta's Llama models offer comprehensive AI functions on personal devices, taking AI capabilities a step further from traditional cloud-based systems.
Meta's approach effectively overcomes typical platform restrictions imposed by tech giants like Google and Apple by collaborating directly with hardware manufacturers such as Qualcomm and MediaTek. This collaboration allows Meta to expand its AI functionalities across a variety of Android devices. This strategy underscores a pivotal shift from relying on central servers for computation, promoting localized data processing, which ensures privacy by handling data directly on the user's device.
Learn to use AI like a Pro
Get the latest AI workflows to boost your productivity and business performance, delivered weekly by expert consultants. Enjoy step-by-step guides, weekly Q&A sessions, and full access to our AI workflow archive.
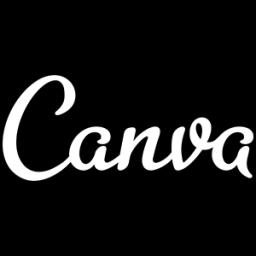
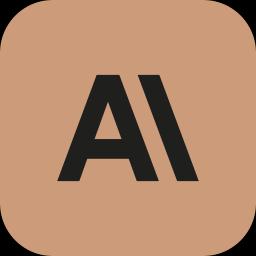
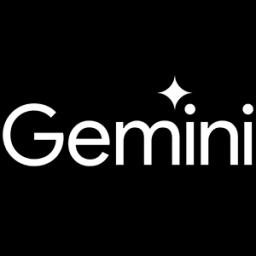
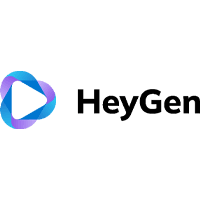
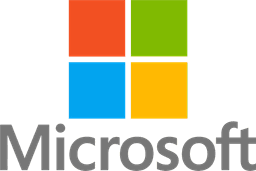
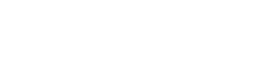
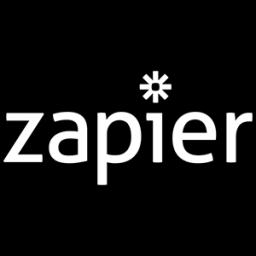
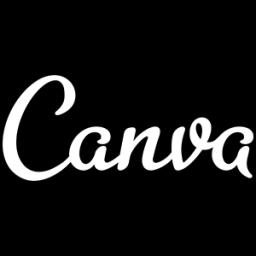
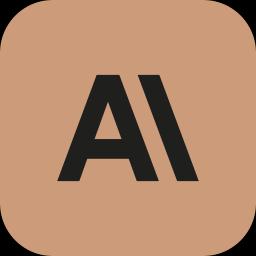
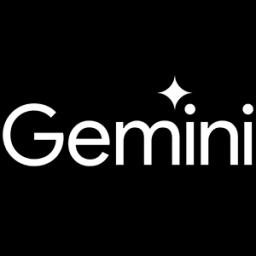
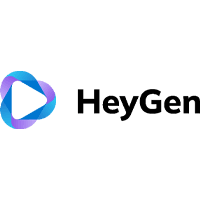
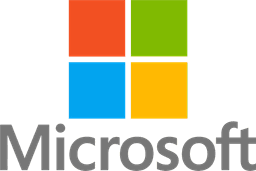
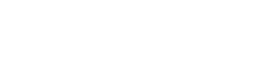
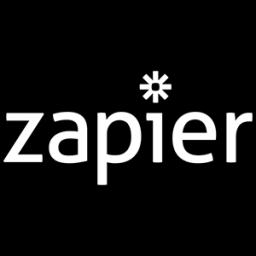
The technical innovations introduced by Meta are noteworthy, including methods like Quantization-Aware Training with LoRA adaptors (QLoRA) and SpinQuant. These allow the models to be reduced in size, yet maintain equivalent performance to larger, cloud-based AI systems. The Llama 3.2 1B and 3B models demonstrate this by being significantly smaller—56% less in size—while using 41% less memory without sacrificing their ability to process efficiently up to 8,000 characters. Such efficiencies are crucial for the practical application of AI on mobile platforms.
Meta's open-source strategy sets it apart from competitors, offering developers more flexibility in application development. This approach is seen as revolutionary compared to Google and Apple's more integrated and closed systems. By making its models openly available, Meta encourages innovation and collaboration across the technology landscape, potentially democratizing the mobile AI sector.
While having AI operate on personal devices provides benefits like enhanced privacy and the ability to harness real-time data processing capabilities, there are notable challenges. The biggest trade-off lies in limited computational power and increased energy consumption that comes with the territory of mobile technology. Experts see Meta’s mobile device AI models as groundbreaking but caution that careful management of these limitations is essential for effective use.
Public and expert reactions to Meta's endeavor have been mixed. There is palpable excitement over the potential for broader AI access and innovation, but concerns remain about security and ethical implications, especially regarding the open-source nature of these models and the potential for misuse. Meta's vast data resources might give it a competitive edge, yet this also raises questions about transparency and control in AI development.
Learn to use AI like a Pro
Get the latest AI workflows to boost your productivity and business performance, delivered weekly by expert consultants. Enjoy step-by-step guides, weekly Q&A sessions, and full access to our AI workflow archive.
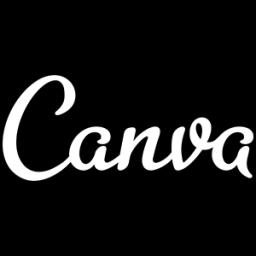
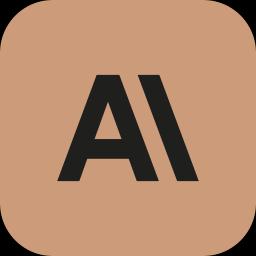
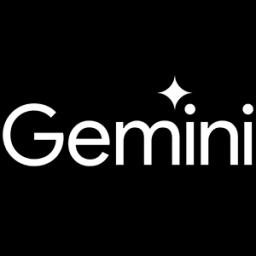
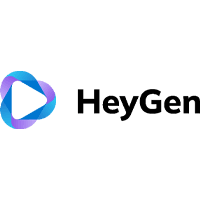
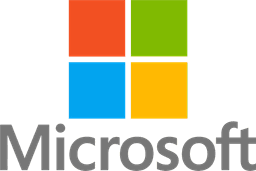
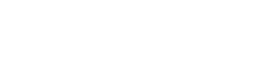
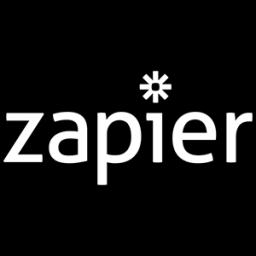
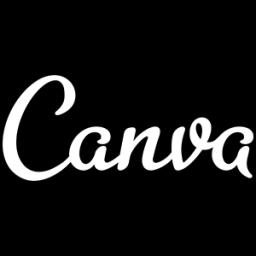
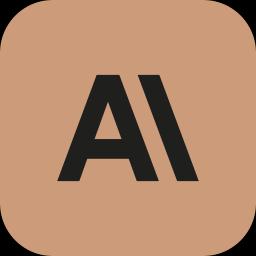
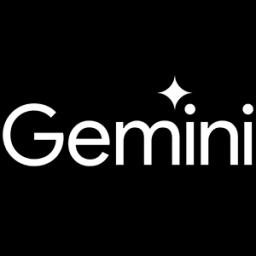
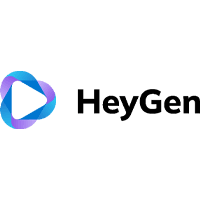
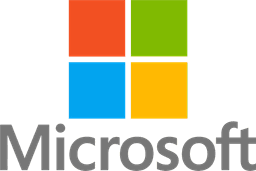
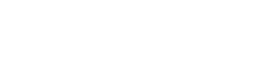
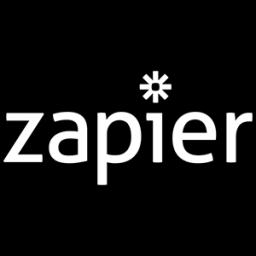
Looking towards the future, Meta's strategy could reshape the AI ecosystem, potentially instigating more competitive practices in mobile computing while pushing the industry closer to personal device-centered AI solutions. This evolution might also prompt regulatory changes to navigate the challenges of democratized AI use, steering global technology policies towards more balanced practices that consider security, privacy, and innovation.
Technical Advancements Enabling AI on Mobile
Meta's introduction of smaller, more efficient Llama AI models marks a significant advancement in the field of mobile AI technology. With the application of quantization and Quantization-Aware Training with LoRA adaptors (QLoRA) and SpinQuant, these models achieve a reduced size while retaining high performance. This breakthrough enables AI functionalities on personal devices, facilitating faster processing and enhanced privacy by handling sensitive data locally, without the need for extensive computational resources from cloud-based systems.
By reducing the size of their Llama models by 56% and cutting memory usage by 41%, Meta ensures that these models provide near-equivalent performance to their larger, cloud-based counterparts. The efficiency of these models allows them to handle up to 8,000 characters, making them suitable for a wide array of mobile applications. This strategic move not only differentiates Meta from competitors like Google and Apple, who lean towards integrated cloud ecosystems, but also democratizes AI usage by making it accessible directly on consumer devices.
Meta's decision to open-source their AI models has potential industry-wide implications. Unlike Google's and Apple's more proprietary AI ecosystems, Meta's strategy of collaborating with chip manufacturers such as Qualcomm and MediaTek provides developers the freedom to innovate without being tied to the update cycles of iOS or Android platforms. This open approach could spur a new wave of creativity in mobile AI development, but it also underscores the need for responsible usage, as the accessibility of AI models carries inherent risks of misuse.
Running AI applications locally on mobile devices presents both significant benefits and challenges. The most prominent advantage is the enhanced privacy that comes with processing data directly on users' phones, which limits the amount of sensitive information shared across networks. Conversely, this shift demands more from the device's processing power, potentially affecting battery life and performance. Developers need to balance these trade-offs against the benefits of localized data processing.
Meta's initiative to enhance AI capabilities on personal devices could redefine the mobile computing landscape. As Meta asserts itself as a key player in the tech industry by challenging traditional platform dominators, it could encourage further advancements in AI applications on consumer devices. This transition reflects a broader trend towards personal computing, with implications for data privacy, security, and innovation, much like the transformative role of platforms like TensorFlow and PyTorch in earlier AI development cycles.
Learn to use AI like a Pro
Get the latest AI workflows to boost your productivity and business performance, delivered weekly by expert consultants. Enjoy step-by-step guides, weekly Q&A sessions, and full access to our AI workflow archive.
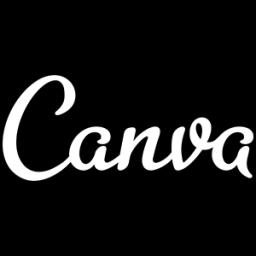
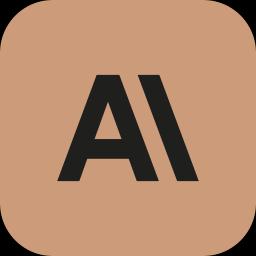
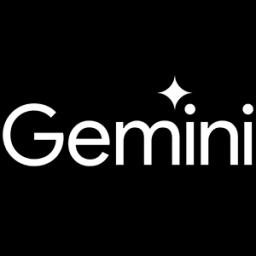
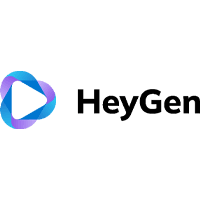
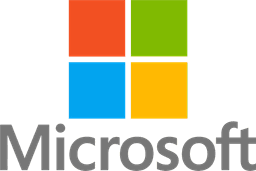
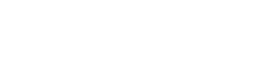
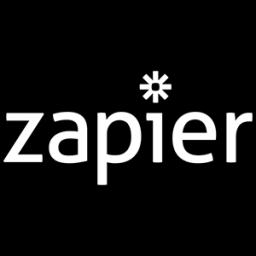
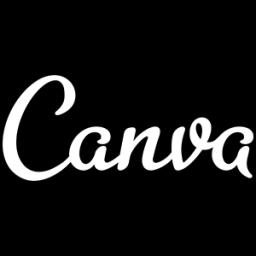
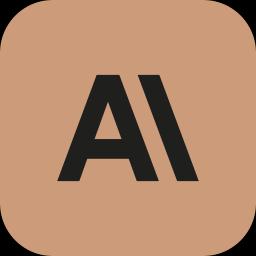
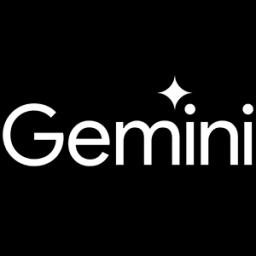
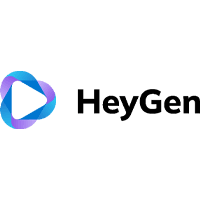
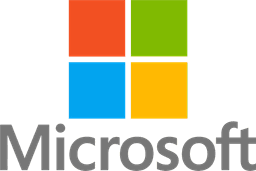
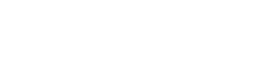
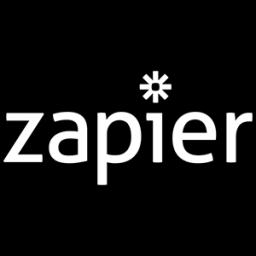
Comparison with Cloud-Based AI Models
Cloud-based AI models have set a high standard in providing robust and scalable artificial intelligence applications, but they typically require a stable internet connection and contribute to data privacy concerns. Comparing this with Meta's approach of operationalizing AI models directly on personal devices offers a contrasting perspective. This on-device model processing significantly minimizes latency and improves user privacy since sensitive data can be processed without transmission over the internet.
From a technical standpoint, the newer, compact versions of Meta's Llama AI models are designed to perform optimally on smartphones and tablets. Techniques like quantization, which involves compressing the AI model to reduce its size while maintaining performance, empower these models to operate efficiently on devices with limited resources. This is a significant leap in AI technology, facilitating functions that previously required the computational power of large cloud infrastructures.
Moreover, integrating AI on-device through these compact models reduces dependency on cloud-based systems, which traditionally face bottlenecks due to network speeds and server capacities. This transition to on-device AI models presents numerous advantages, including lower operational costs and enhanced offline capabilities, thereby democratizing access to AI technologies.
Notably, by working directly with chip manufacturers such as Qualcomm and MediaTek, Meta bypasses conventional platform constraints imposed by tech giants like Google or Apple. This collaboration could signal a shift in the mobile AI landscape, where performance optimization happens at the hardware level, aligning closely with software enhancements. Such strategies could redefine mobile AI computing standards, encouraging more dynamic and innovative implementations across various device types.
Overall, Meta's introduction of smaller, yet equally potent AI models on personal devices embodies a shift towards a more decentralized and integrated approach to AI. It challenges the status quo of cloud dependency, aiming for a future where personal devices are empowered to handle complex AI tasks independently. While there are inherent challenges regarding processing power and energy consumption on mobile platforms, the continued advancement of these models could catalyze a major transformation within the AI ecosystem.
Meta’s Strategic Divergence from Google and Apple
Meta Platforms has taken a bold step in the competitive arena of artificial intelligence by diverging strategically from the approaches traditionally employed by tech giants like Google and Apple. The company's initiative to develop and deploy smaller Llama AI models capable of functioning on mobile devices marks a significant departure from conventional wisdom, which has largely favored cloud-based AI solutions. By compressing their models through advanced quantization techniques such as Quantization-Aware Training with LoRA adaptors (QLoRA) and SpinQuant, Meta has not only maintained the performance integrity of its AI but also vastly reduced the model sizes and memory requirements, consequently boosting processing speeds on personal devices.
Learn to use AI like a Pro
Get the latest AI workflows to boost your productivity and business performance, delivered weekly by expert consultants. Enjoy step-by-step guides, weekly Q&A sessions, and full access to our AI workflow archive.
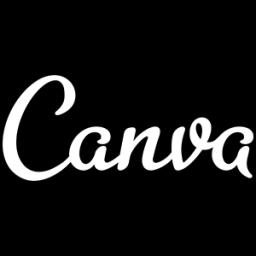
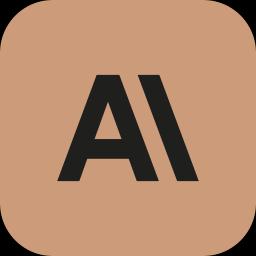
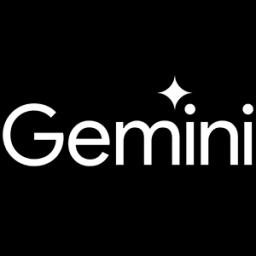
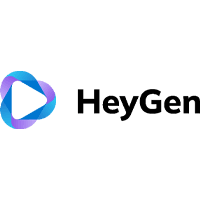
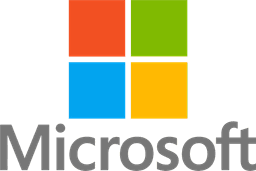
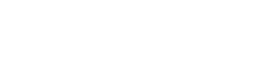
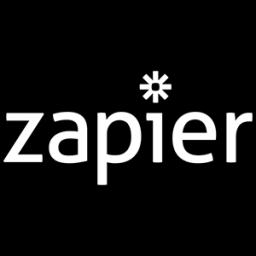
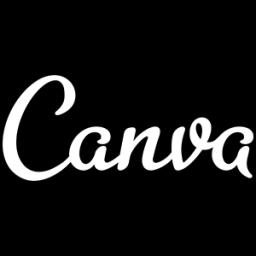
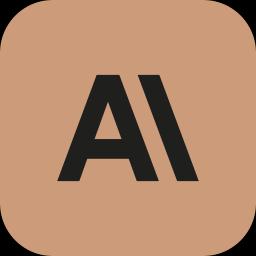
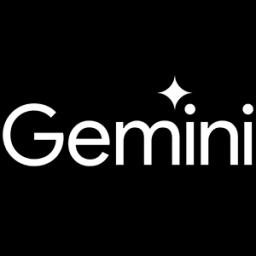
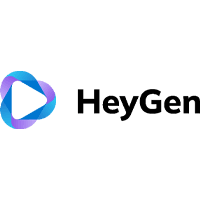
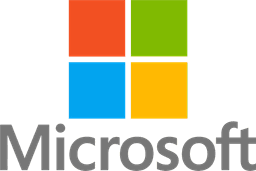
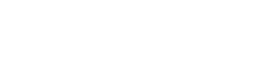
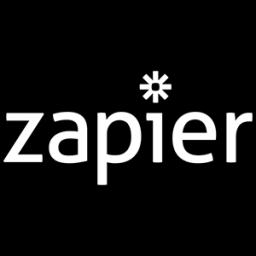
This strategic shift has allowed Meta to circumvent the control exerted by platform gatekeepers like Google and Apple. By collaborating directly with chip manufacturers like Qualcomm and MediaTek, Meta enhances AI capabilities across Android devices without being beholden to the ecosystem restrictions typically imposed by dominant operating systems. This open-source strategy provides developers the freedom to innovate and build applications unhindered by the limitations of closed platforms. Unlike Google and Apple, who maintain tighter controls over their AI developments, Meta's expansive approach democratizes AI technology, enabling widespread participation in its advancement.
Deploying AI models directly onto mobile devices presents distinct benefits and challenges. On one hand, it ensures that sensitive user data is processed directly on the device, thus enhancing privacy. This local processing approach allows for faster response times and potentially more personalized user experiences. However, it also introduces challenges, including the need to optimize for the relatively limited computational power and battery life of mobile devices. Despite these challenges, Meta's move is viewed by experts as a groundbreaking development, as it offers an alternative path to AI integration that balances the benefits of privacy with performance. This is expected to drive further innovations in how AI is accessed and utilized on the go.
The introduction of Meta's Llama models is poised to reshape the AI ecosystem and mobile computing landscape. By reducing reliance on cloud computing, Meta is setting a precedent for personal computing where AI functionalities are more intimately entwined with daily smartphone use. This shift also suggests a potential trend towards decentralized AI systems that can operate independently from massive data centers. Such a transition promotes a sustainable model where AI technologies are more user-centric and privacy-conscious, potentially revolutionizing mobile AI as significantly as past frameworks like TensorFlow and PyTorch have in broader software development contexts.
Public and expert reactions to Meta's initiative have been varied. While there is enthusiasm about the potential for innovation and increased security from handling data locally, concerns persist regarding the ethical implications and security risks of making AI more accessible. Critics worry about biases within AI models and the potential for misuse in the absence of stringent oversight and ethical guidelines. Proponents argue for a balanced approach, advocating for comprehensive safety measures to accompany these advancements, ensuring that the benefits of privacy and accessibility do not come at the cost of security and ethical integrity.
Looking ahead, Meta's strategy could have far-reaching implications across economic, social, and political spheres. Economically, bypassing traditional gatekeepers could lead to a more competitive environment that lowers barriers to entry for new developers and reduces costs for consumers. This could foster greater innovation and expand the availability of personalized AI solutions. Socially, while enhancing privacy through local data processing, there is a crucial need to address data security and bias, ensuring that these open-source models are used responsibly. Politically, the increased accessibility and potential cross-border collaborations could influence regulatory frameworks, prompting the need for new policies to balance innovation with security and privacy concerns. Given Meta's collaboration with major chipmakers, this strategy could also inform international tech relations, particularly among AI-leading nations.
Benefits and Challenges of On-Device AI
In recent years, the advancement of artificial intelligence (AI) has seen significant growth and has begun shifting from being predominantly cloud-based to running directly on personal devices. On-device AI presents both opportunities and challenges. By processing information locally on smartphones or tablets, it offers the potential for enhanced privacy as sensitive data is no longer sent to remote servers. This direct processing reduces latency, leading to quicker responses which are pivotal for applications like real-time translation, augmented reality, and personalized recommendations.
Learn to use AI like a Pro
Get the latest AI workflows to boost your productivity and business performance, delivered weekly by expert consultants. Enjoy step-by-step guides, weekly Q&A sessions, and full access to our AI workflow archive.
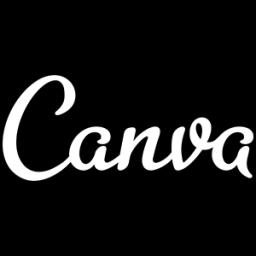
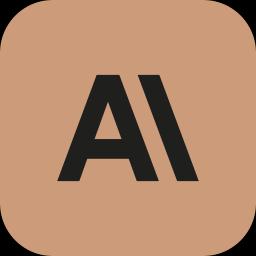
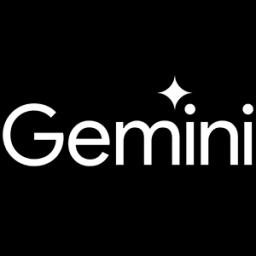
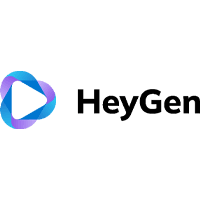
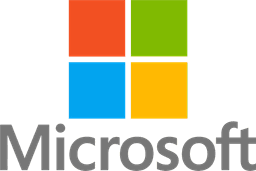
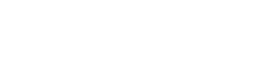
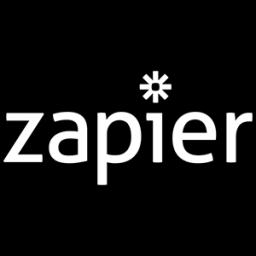
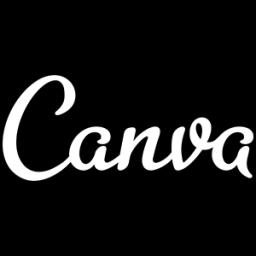
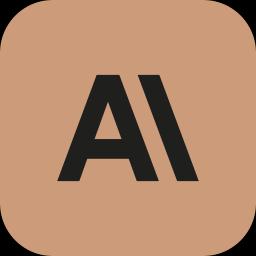
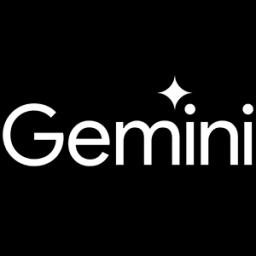
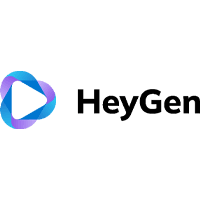
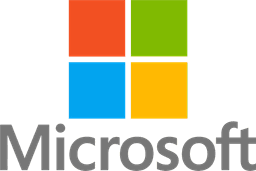
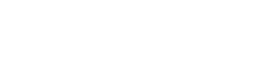
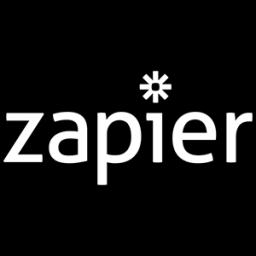
However, the implementation of on-device AI is not without its challenges. Mobile devices are constrained by their limited processing power, battery life, and memory capacity. To overcome these hurdles, companies like Meta have resorted to innovative techniques such as quantization, which shrinks the model size while maintaining its efficacy, enabling AI functionalities that would traditionally require robust server infrastructure. But these efforts, while mitigating some issues, do not entirely bridge the gap with the near-limitless resources of cloud computing.
Moreover, the transition from cloud to device introduces new dimensions to data privacy and security. While on-device AI minimizes external data exposure, it demands rigorous local security measures to protect against breaches. Additionally, as these models become open-sourced, there are concerns about potential misuse. For instance, increased accessibility to robust AI models could accelerate AI-enabled threats like deepfakes or sophisticated phishing attacks. Therefore, ensuring comprehensive safeguards and ethical guidelines in developing and deploying on-device AI is crucial.
The deployment of AI on personal devices also shifts the dynamics in the technology industry. It paves the way for a more decentralized AI ecosystem, contrary to the centralized, cloud-dependent systems dominated by tech giants like Google or Apple. Meta’s collaboration with hardware partners exemplifies an alternative approach that could democratize AI technology by making it more accessible to developers and consumers alike. Nevertheless, this shift could prompt regulatory bodies to rethink current policies to address the unique risks and opportunities posed by on-device AI.
Impact on the AI Ecosystem and Mobile Computing
Meta's recent unveiling of smaller Llama AI models poised to operate seamlessly on smartphones and tablets marks a transformative shift in the AI ecosystem and mobile computing landscape. By crafting AI models that are optimized for mobile platforms through advanced techniques like quantization, Meta enables powerful AI functionalities directly on personal devices. This pivotal move not only circumvents traditional platform control by tech giants like Google and Apple but does so by strategically collaborating with chip manufacturers such as Qualcomm and MediaTek. These partnerships facilitate the distribution of robust AI capabilities across a wide array of Android devices, fostering a decentralization of AI processing which retains the capacity to manage sensitive data more securely and instantly on personal electronics.
The technological innovations behind these AI models significantly reduce their size and memory usage without compromising performance, setting a new benchmark for mobile AI development. Through techniques like Quantization-Aware Training with LoRA adaptors (QLoRA) and SpinQuant, Meta has effectively minimized the demands on device memory and processing power while maintaining functionality akin to larger, cloud-based models. This balance of local processing power and privacy represents an evolution in how AI models can be used to enhance user experiences independently of cloud infrastructure, addressing rising concerns about data privacy and security.
Meta's strategy sets itself apart from competitors by adopting an open-source model, offering developers increased freedom to innovate without the constraints typically associated with closed ecosystems. By aligning with chipmakers and fostering an ecosystem of open development, Meta stands to not only democratize AI technology but also accelerate the global expansion and application of AI across new sectors and industries. However, this approach is not without its challenges, as it raises potential risks around AI misuse, data bias, and management of shared intellectual properties.
Learn to use AI like a Pro
Get the latest AI workflows to boost your productivity and business performance, delivered weekly by expert consultants. Enjoy step-by-step guides, weekly Q&A sessions, and full access to our AI workflow archive.
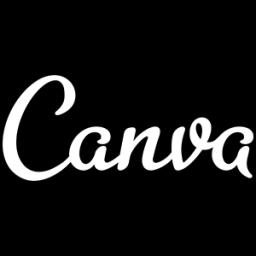
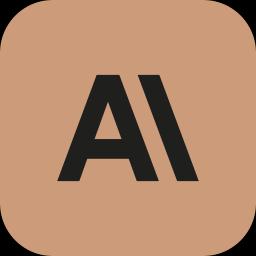
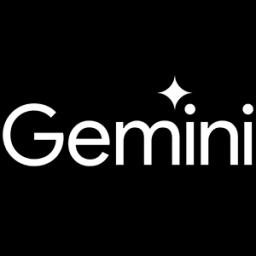
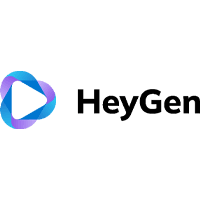
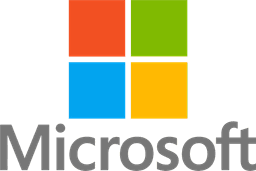
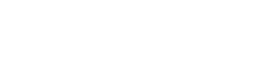
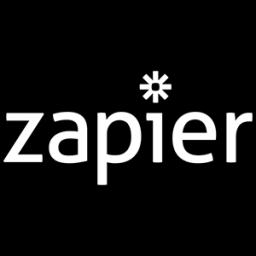
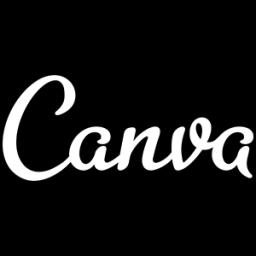
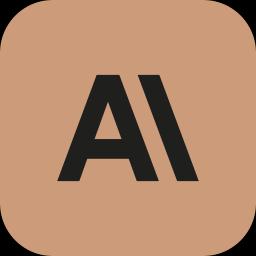
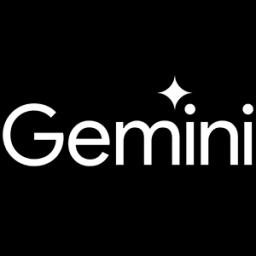
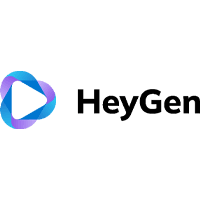
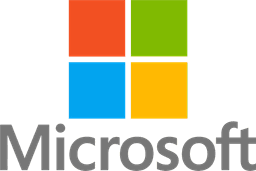
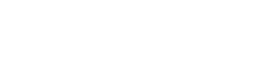
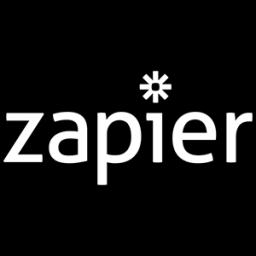
Public and expert response to Meta's approach has been mixed, with enthusiasm for the privacy benefits and innovation potential counterbalanced by concern over the ethical implications and potential for misuse. While many advocate for the need for stringent oversight and safety measures, others are hopeful that the open-source methodology will lead to faster problem identification and resolution within the AI community. The capacity for these smaller AI models to handle significant data tasks locally represents a substantial step forward, but underscores the need for responsible deployment and usage of AI technologies.
Looking ahead, the adoption of Meta's AI models on mobile devices is poised to influence the economic, social, and political fabric of technology adoption and development. Economically, by bypassing established platform gatekeepers, Meta aids in creating a more competitive environment conducive to innovation and lowering costs for end-users. Socially, the local processing of AI contributes to enhanced privacy but necessitates safeguards against data misuse and bias. Politically, the global collaboration with chip producers positions Meta as a pivotal player in tech diplomacy, potentially influencing international policies on AI development, regulation, and cross-border collaboration. As such, Meta's advancements in mobile AI are likely to have lasting ramifications across multiple sectors, reflecting broader trends towards localized, privacy-conscious technology solutions.
Related Industry Developments
In the rapidly evolving AI landscape, there's a significant push towards empowering mobile devices with robust AI capabilities. One of the front-runners in this technological shift is Meta, which has developed smaller, efficient versions of its Llama AI models to function on smartphones and tablets. These models are not just replicas of their larger counterparts, but optimized through advanced compression techniques such as quantization. This allows the AI to perform complex tasks quickly while maintaining user privacy by processing data directly on the device instead of relying on cloud computing.
Meta's strategy stands in contrast to the approaches of tech giants like Google and Apple. While these companies tend to integrate their AI models within their own ecosystems, Meta's decision to open-source its models and collaborate with chip manufacturers like Qualcomm and MediaTek offers a fresh alternative. This partnership ensures that a wider range of Android devices can leverage advanced AI without being gated by platform-specific restrictions. This move not only democratizes AI technology but also fosters innovation by enabling more developers to create AI applications tailored to diverse needs.
Recent developments in AI hardware and software play a pivotal role in this shift towards on-device AI. Qualcomm's introduction of the Snapdragon 8 Elite chip is a prime example, designed to handle sophisticated generative AI tasks more efficiently. Similarly, both Apple and Google are advancing their AI functionalities by enhancing user experiences on personal devices. Apple, for instance, is integrating AI to boost mobile commerce and user interaction, while Google's Gemini assistant aims for more intuitive conversational capabilities across Android and Pixel devices.
From an expert viewpoint, Meta's mobile AI models are groundbreaking. They utilize quantization techniques like QLoRA and SpinQuant to maintain accuracy while minimizing resource use, capitalizing on the instant processing and enhanced privacy that on-device processing provides. However, experts also highlight challenges such as the limited computational power and higher energy demands of mobile devices compared to their cloud-based counterparts. Despite these hurdles, the potential reduction in developer dependence on Android or iOS updates is seen as a valuable upside.
Learn to use AI like a Pro
Get the latest AI workflows to boost your productivity and business performance, delivered weekly by expert consultants. Enjoy step-by-step guides, weekly Q&A sessions, and full access to our AI workflow archive.
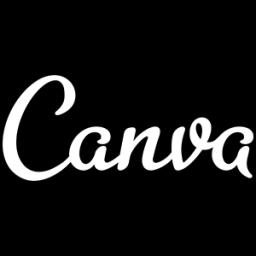
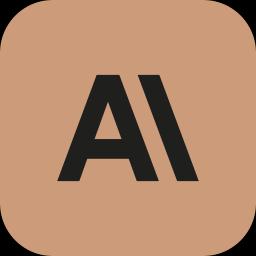
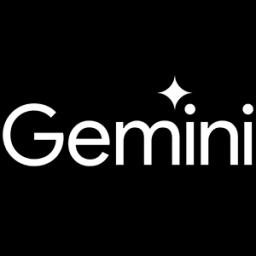
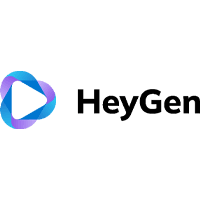
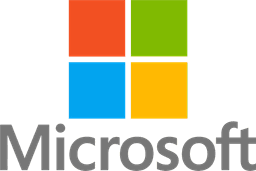
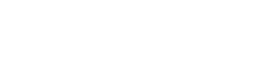
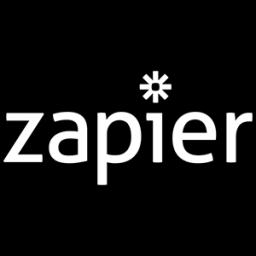
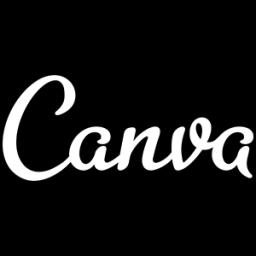
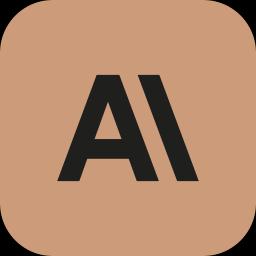
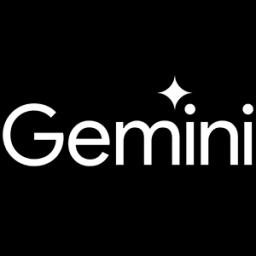
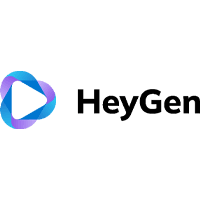
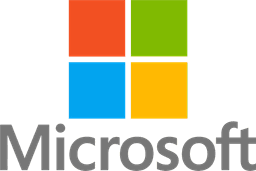
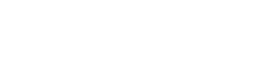
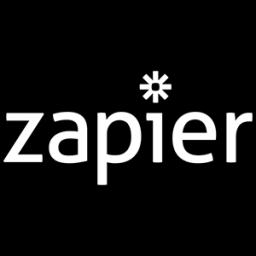
Public opinion on Meta's open-source initiative is divided yet cautiously hopeful. While concerns about data security, potential bias, and misuse linger, the transparency and collaborative potential of open-source models have ignited a wave of optimism. This could lead to more rigorous scrutiny and faster identification of issues, promoting a culture of innovation alongside safety. Consequently, there's a growing consensus that the benefits of open-source AI, such as increased accessibility and inclusivity in development, might outweigh the risks if implemented responsibly.
Looking forward, the ramifications of Meta's AI strategy could be extensive. Economically, by bypassing traditional platform control, Meta encourages a competitive market space, fostering lower costs and accessibility for developers and consumers alike. Socially, these advancements promise improved privacy and personalization but warrant strong data security measures to safeguard against misuse. Politically, this decentralized approach may compel new regulatory frameworks to oversee ethical AI growth, while aligning global tech policies to reconcile innovation with essential security measures.
Expert Opinions on Meta's AI Models
Meta's recent advancements in AI technology have sparked a significant wave of interest and analysis from industry experts. Their Llama AI models, designed to operate effectively on mobile devices, have been lauded for their technical sophistication. By employing quantization techniques such as Quantization-Aware Training with LoRA adaptors (QLoRA) and SpinQuant, these models achieve a smaller size and reduced memory usage, without compromising on accuracy or speed. This allows for quicker data processing and improved privacy, as sensitive information can be managed locally rather than through cloud servers.
While the benefits of deploying AI on personal devices are clear, experts also highlight the accompanying challenges. Mobile platforms inherently possess limited processing power and can lead to higher energy consumption, potentially impacting user experience. Despite these hurdles, Meta's strategy to open-source its models, contrasting with Google and Apple's more closed ecosystems, is seen as a creative nudge towards innovative applications development. By collaborating with major chip manufacturers like Qualcomm and MediaTek, Meta not only bypasses traditional mobile platform constraints but also empowers developers with greater autonomy.
However, with increased accessibility comes the risk of AI misuse. Analysts express concerns over possible scenarios where such technologies might be employed for phishing or creating spam. Meta's immense repository of user data could potentially offer them a competitive advantage, yet it also necessitates a discussion on privacy and ethical data use.
Public Reactions and Concerns
Following Meta's announcement of its new Llama AI models for mobile devices, the public response has been varied. On the one hand, there are rising concerns about the potential misuse of these models. With AI processing now possible on personal devices, there is a fear of increased privacy risks and the possibility of AI being used for malicious purposes such as phishing or spam. The open-source nature of the project also raises questions about the lack of transparency in training data which could lead to biased outcomes.
Learn to use AI like a Pro
Get the latest AI workflows to boost your productivity and business performance, delivered weekly by expert consultants. Enjoy step-by-step guides, weekly Q&A sessions, and full access to our AI workflow archive.
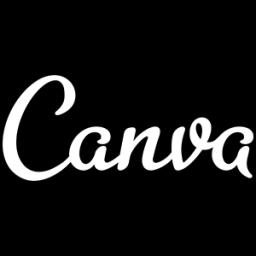
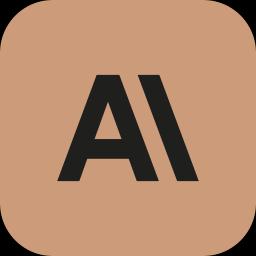
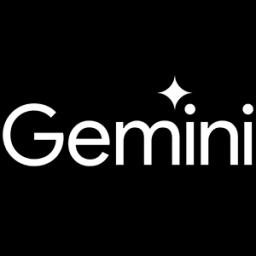
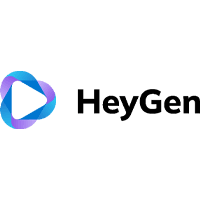
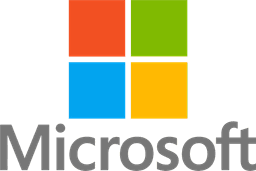
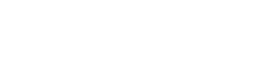
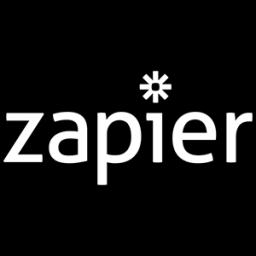
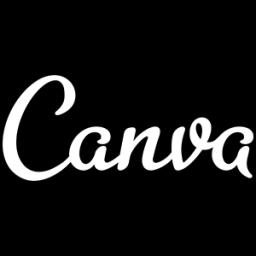
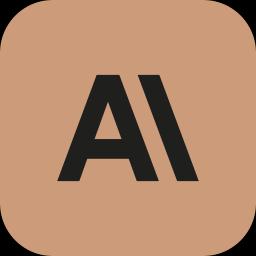
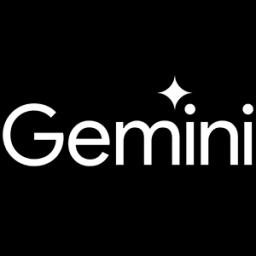
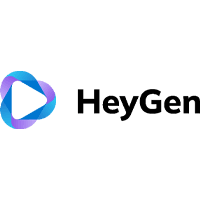
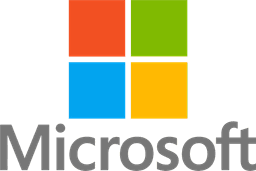
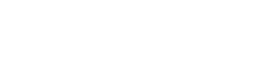
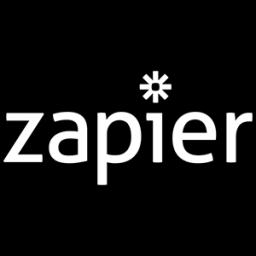
Conversely, there is notable excitement about the democratization of AI technology.Meta's decision to open-source its models is viewed positively by those who see it as a way to foster innovation and inclusive development in the AI space. This move allows greater participation from developers around the world, thereby accelerating advancements and enabling a broader array of applications. Some see this as an opportunity to identify and address potential issues early on due to increased scrutiny.
This dichotomy in public opinion reflects a cautious optimism. Many are calling for Meta to implement robust safety measures to mitigate the risks associated with wider user access to AI technology. The success of these models hinges on Meta's ability to balance innovation with responsible usage, assuring users of the privacy and security of their data while tapping into the technology's full potential.
Future Implications of Meta's Strategy
Meta's strategy to miniaturize and deploy Llama AI models on mobile devices signifies a turning point in AI applications, breaking free from the traditional limitations imposed by cloud-based systems. These innovations in quantization and model compression not only enable these powerful AI tools to reside on smartphones and tablets, but also preserve their effectiveness while enhancing speed and reducing memory usage. This technological leap forward positions Meta as a leader in embedding AI capabilities directly into personal devices, a move that presents significant opportunities and challenges.
In stark contrast to the conventional approaches of tech giants like Google and Apple, Meta's method of open-sourcing its AI models and forming alliances with hardware manufacturers revolutionizes the development landscape. This partnership with companies like Qualcomm allows for a broader integration of Llama models across various devices, thus democratizing AI accessibility. The collaborative strategy may dismantle existing technological silos, inviting an explosion of innovation and diversifying AI's application landscape.
The shift from cloud-computing to local processing on mobile devices heralds a new era of user privacy and data stewardship. By confining AI functions to personal devices, Meta's approach answers growing concerns about data privacy, ensuring that sensitive information remains on the device rather than being transferred to external servers. However, this shift is not without its trade-offs; developers must navigate the constraints of mobile processing power and scrutinize the energy demands of these advanced applications.
Meta's groundbreaking AI strategy could reshape the future political and economic terrain by challenging the dominance of current digital juggernauts and reducing entry barriers for developers. Economically, this democratization of AI resources has the potential to invigorate competitive markets, paving the way for innovative, personalized AI solutions at more competitive prices. Politically, it could necessitate the genesis of new regulations to manage the influx of AI applications and ensure ethical deployment of these technologies.
Learn to use AI like a Pro
Get the latest AI workflows to boost your productivity and business performance, delivered weekly by expert consultants. Enjoy step-by-step guides, weekly Q&A sessions, and full access to our AI workflow archive.
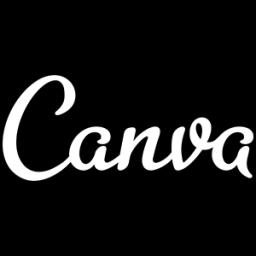
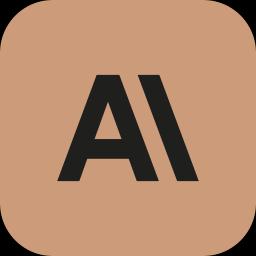
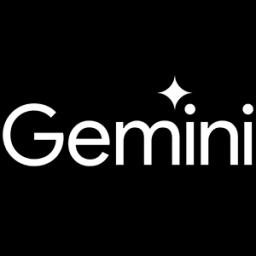
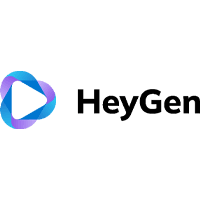
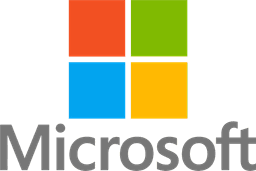
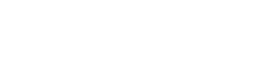
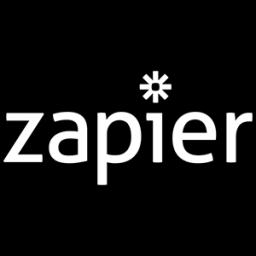
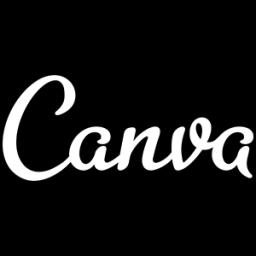
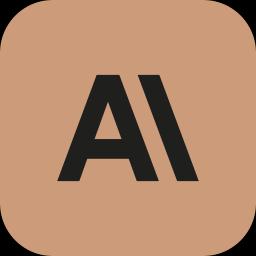
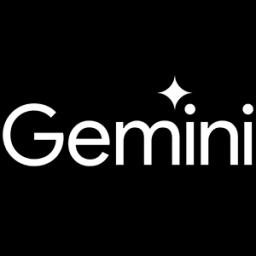
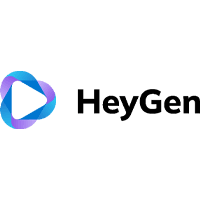
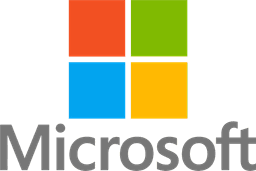
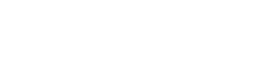
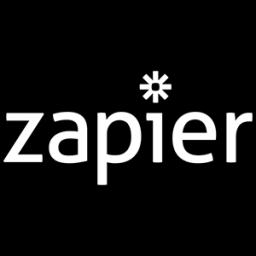
Despite the technical marvel that Meta's AI models represent, public perception remains a crucial factor in their future impact. While there is enthusiasm around the possibilities that such open-source models offer, there is also apprehension regarding privacy and the potential misuse of AI technologies. Addressing these concerns while fostering innovation will be critical for Meta as it navigates the complex interplay of technology and society, making safety and transparency pivotal components of its future endeavors.