Revolutionizing AI's Internal Logic
Meta's Next Big Leap: Thought Preference Optimization for AI
Last updated:

Edited By
Mackenzie Ferguson
AI Tools Researcher & Implementation Consultant
Researchers at Meta, in collaboration with UC Berkeley and NYU, are pioneering a new method called 'Thought Preference Optimization' (TPO) to enhance generative AI models. This groundbreaking approach focuses on refining AI's internal thought processes, allowing it to 'show its work' and improve the coherence and quality of its responses. TPO addresses the lack of logical reasoning in internet-sourced training data by synthetically generating reasoning within AI, promising significant advancements in AI's thinking capabilities across various fields.
Introduction to Thought Preference Optimization (TPO)
The rapid advancement of artificial intelligence (AI) technologies has triggered a relentless pursuit of innovation to enhance AI's capabilities. An exciting development in the field is Thought Preference Optimization (TPO), a method spearheaded by researchers from Meta in collaboration with UC Berkeley and NYU. This approach aims to refine the internal reasoning processes of generative AI, significantly enhancing the quality and coherence of its outputs.
TPO is unique in that it encourages AI to not only focus on generating the correct answer but also to optimize the reasoning that leads to that answer. This concept of "showing work" mirrors educational practices, where students are instructed to painstakingly document their problem-solving steps. In AI, this eventually leads to more transparent and accountable machine-generated insights.
Learn to use AI like a Pro
Get the latest AI workflows to boost your productivity and business performance, delivered weekly by expert consultants. Enjoy step-by-step guides, weekly Q&A sessions, and full access to our AI workflow archive.
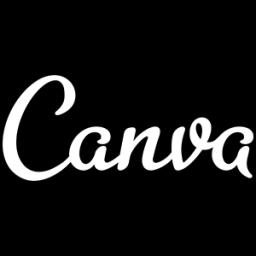
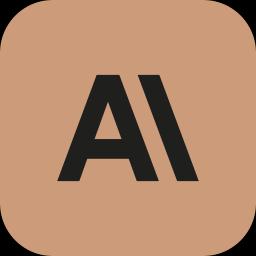
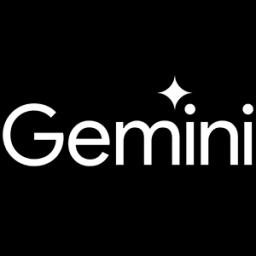
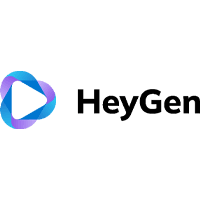
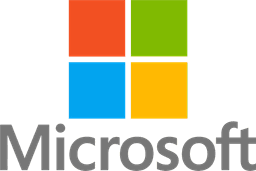
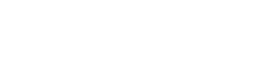
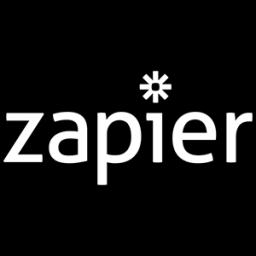
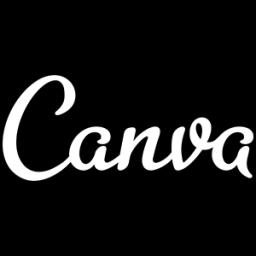
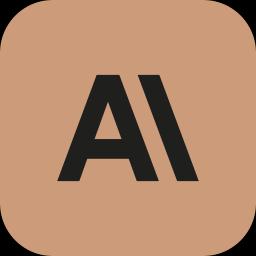
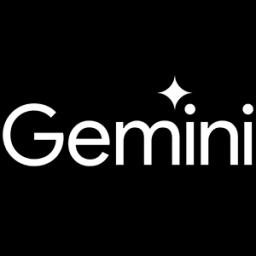
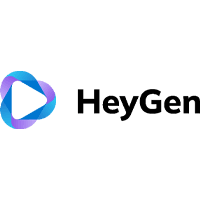
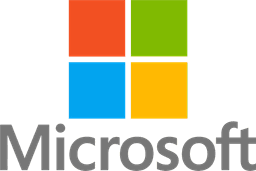
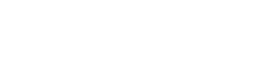
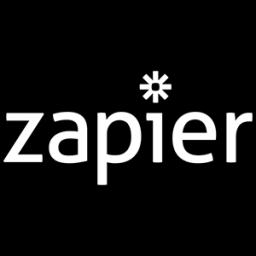
The current landscape of AI development faces challenges due to inconsistencies in the logical reasoning present in internet data that train these models. By synthetically generating reasoning within AI systems, TPO addresses these gaps, potentially revolutionizing the domain by cultivating AIs with strengthened cognitive threads across diverse applications.
Collaborative Development by Meta and Universities
Meta, in collaboration with renowned institutions like UC Berkeley and NYU, has embarked on a groundbreaking journey in the field of generative AI through the development of Thought Preference Optimization (TPO). This innovative method aims to enhance the AI's internal reasoning capabilities, a step forward in making AI responses more coherent and contextually relevant. By addressing the lack of explicit logical reasoning in current AI training data, TPO encourages models to exhibit a structured approach to problem-solving akin to traditional educational techniques of 'showing work.' This collaboration not only strengthens the research outputs but also integrates diverse academic perspectives in refining AI capabilities.
The partnership between Meta and leading universities like UC Berkeley and NYU is a notable example of how academia and industry can work together to push the boundaries of AI technology. TPO represents a synthesis of advanced AI research and educational methodologies, aiming to transform how AI models interpret and respond to complex queries. By focusing on refining the internal thought processes of AI, this collaboration seeks to develop models that deliver more accurate and intuitive responses across various domains, including logic-oriented and creative tasks. Such alliances highlight the potential of collaborative efforts in accelerating technological advancements and addressing the multifaceted challenges of AI development.
The Significance of 'Showing Work' in AI Processing
The ability of AI models to process information and provide logical, coherent responses has been a focal point in advancing AI technologies. Meta's new Thought Preference Optimization (TPO) technique represents a significant leap forward by enabling AI systems to refine their internal reasoning processes. TPO is analogous to the educational strategy of asking students to "show their work" during problem-solving, thereby enhancing understanding and accountability. This detailed internal check allows AI to generate responses that aren't just correct, but also logically sound and transparent, marking a shift towards more sophisticated AI-generated outputs.
Learn to use AI like a Pro
Get the latest AI workflows to boost your productivity and business performance, delivered weekly by expert consultants. Enjoy step-by-step guides, weekly Q&A sessions, and full access to our AI workflow archive.
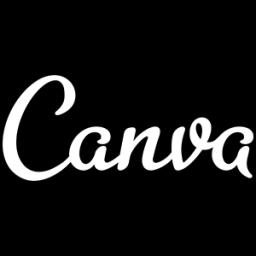
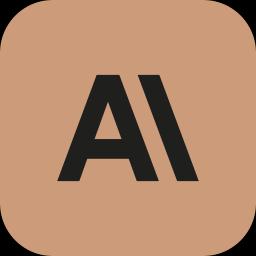
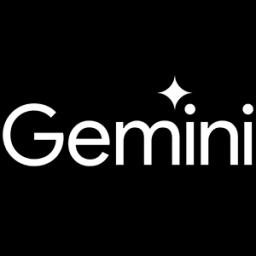
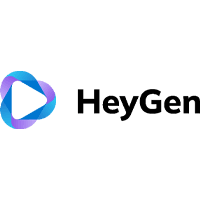
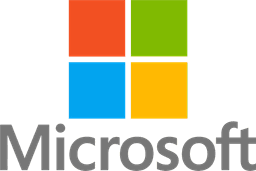
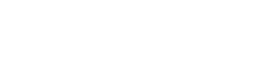
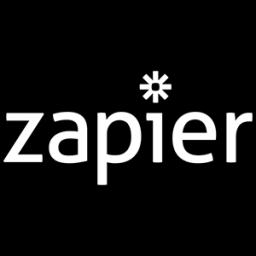
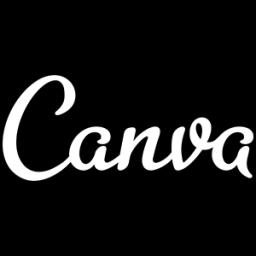
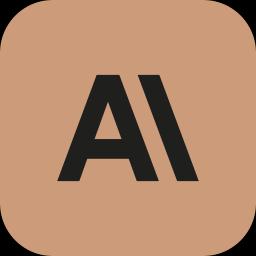
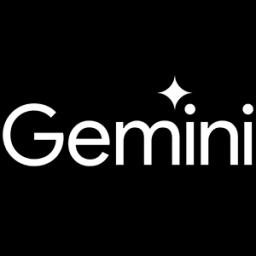
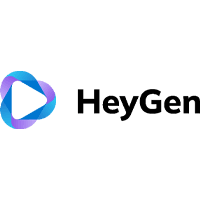
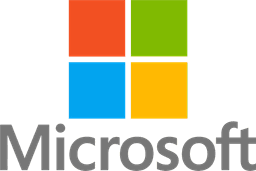
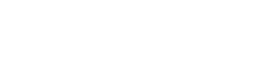
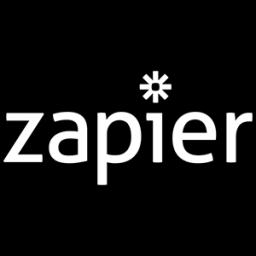
A unique element of TPO is its focus on optimizing internal reasoning before an AI provides its answers. This process ensures that the results are not just outputs of pattern recognition but are underpinned by simulated cognitive processes. These advancements are critical in handling the challenges posed by the vast amounts of internet data, which often lack explicit logical structures. By generating this reasoning internally, TPO has the potential to bridge gaps in logical coherence within AI, leading to more reliable and context-aware outcomes across various applications.
In practice, the implementation of TPO is set to transform how AI interacts with or supplements human tasks. For example, in fields such as customer service or marketing, AI systems capable of demonstrating logical reasoning can lead to more effective and tailored interactions. However, integrating these advanced thought processes into AI models does not come without challenges. The increased computational demand and potential inefficiency in processing time are hurdles that researchers are actively working to overcome, striving to strike a balance between complexity and practicality.
The broader implications of Thought Preference Optimization extend across multiple sectors. Economically, it promises to enhance productivity and reduce costs in industries that rely heavily on AI, although it could also disrupt labor markets by replacing roles traditionally fulfilled by human cognition. Socially, by embedding human-like reasoning in AI, TPO can contribute significantly to educational tools, preparing future generations for an AI-integrated world. Politically, the implications of AI's enhanced decision-making capabilities through TPO could revolutionize public sector operations, albeit with pressing ethical considerations regarding transparency and accountability.
Despite the optimism surrounding TPO, skepticism persists. Critics argue that without domain-specific adjustments, the benefits of TPO might be uneven across different fields of application. There are also concerns about whether AI’s new abilities amount to genuine thinking or merely sophisticated mimicry of human thought patterns. Moreover, as AI becomes more intertwined with social and economic systems, the debate over its impact on jobs, ethics, and human autonomy continues to grow. As TPO and similar technologies evolve, it will be crucial to address these concerns while harnessing the potential benefits of advanced AI reasoning.
Challenges with Current AI Training Data
The development and implementation of Thought Preference Optimization (TPO) presents several challenges linked to existing AI training data. One of the most significant issues is the lack of inherent logical reasoning within the data sourced from the internet. This deficiency complicates the process of training AI models to develop sound and coherent logical reasoning skills. Existing data often reflect a surface-level understanding or pattern-based outputs, which fall short in scenarios that require complex problem-solving abilities or deep cognitive processing.
Moreover, the reliance on vast quantities of unstructured internet data introduces biases and inaccuracies that are difficult to filter out during the training process. These limitations are exacerbated when models, including those being developed under TPO, attempt to simulate human-like thought processes, where the expectation is to not just provide answers but also demonstrate the reasoning behind them. This 'show your work' approach necessitates data that is logically rich and contextually meaningful, which is currently scarce.
Learn to use AI like a Pro
Get the latest AI workflows to boost your productivity and business performance, delivered weekly by expert consultants. Enjoy step-by-step guides, weekly Q&A sessions, and full access to our AI workflow archive.
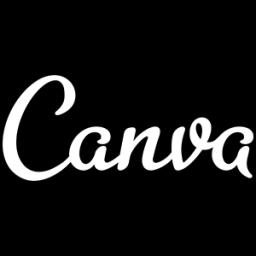
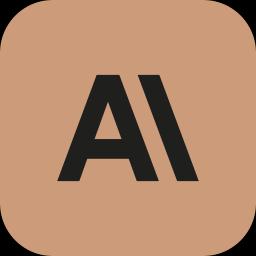
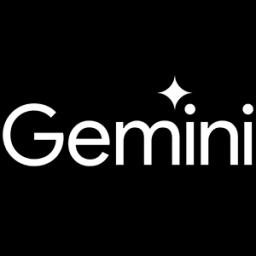
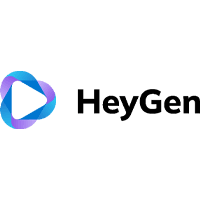
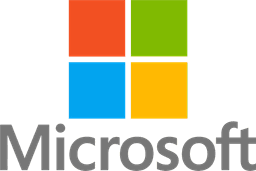
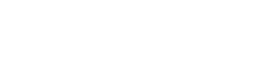
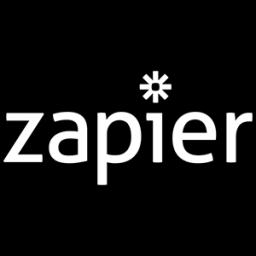
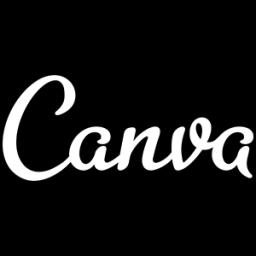
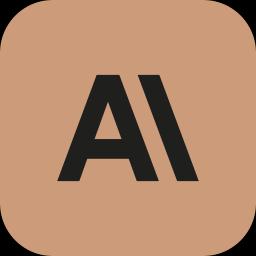
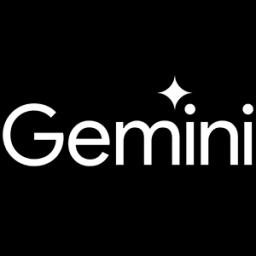
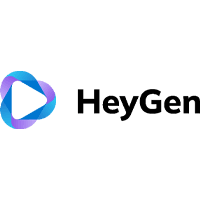
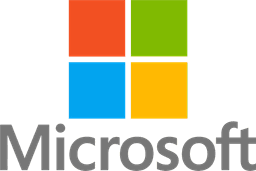
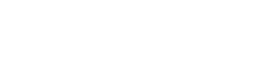
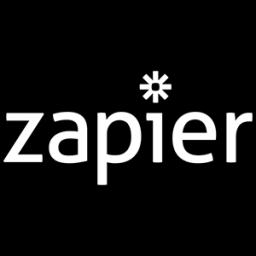
Another challenge lies in the computational demands associated with implementing sophisticated reasoning processes in AI. While the goal of TPO is to enhance the logical reasoning and coherence of AI outputs, this often requires more computational resources and time. The increased complexity may lead to inefficiencies when the AI systems try to handle quick-response tasks, detracting from their applicability in real-time or time-sensitive applications.
As AI practitioners strive to refine these models, overcoming these data-related challenges is paramount. There is a continuous need for advanced techniques to generate or synthesize reasoning-focused datasets that enhance AI's internal decision-making processes. Additionally, balancing the sophistication of TPO with practical application demands remains a key concern, aiming to ensure AI models not only think more like humans but also do so efficiently and effectively across diverse domains.
Innovative TPO Applications in AI
Thought Preference Optimization (TPO), developed by Meta in collaboration with UC Berkeley and NYU, marks a transformative step in enhancing AI's cognitive processes. By focusing on the refinement of AI's internal reasoning, TPO empowers AI to exhibit a more robust chain-of-thought reasoning, akin to the educational requirement of 'showing your work.' This approach is designed to mitigate one of the major limitations of current AI models: the lack of explicit logical reasoning in training data sourced from the internet.
This innovation not only optimizes AI outputs but also has far-reaching implications across multiple domains such as customer service, knowledge management, and beyond. The fundamental uniqueness of TPO lies in its ability to train AI to optimize its reasoning processes internally before delivering responses, thus ensuring greater accountability, coherence, and quality.
As the AI landscape rapidly evolves, TPO is paving the way for generative models that are not only capable of producing sophisticated responses but are also equipped with a consciousness of thought that allows them to adapt and reason across various tasks and challenges. The introduction of TPO thus represents a paradigm shift, promising a future where AI can engage more deeply with complex problems and deliver solutions that are more aligned with human-like thinking.
Comparing TPO with Other AI Reasoning Enhancements
In recent years, the field of AI development has seen various approaches aimed at enhancing the reasoning capabilities of language models. One such method, Thought Preference Optimization (TPO), developed by Meta in collaboration with UC Berkeley and NYU, is gaining attention for its innovative means of improving AI thought patterns. This method stands out because it not only focuses on the outputs generated by the AI, but also the internal reasoning processes that precede these outputs. Unlike traditional methods that often rely heavily on vast amounts of data to train models, TPO encourages AI to 'show its work,' mirroring an educational strategy where students must demonstrate the steps taken to reach a conclusion. This approach aims to fill the gap in current AI training data, which often lacks explicit logical reasoning, by synthetically generating these thought processes within the AI itself.
Learn to use AI like a Pro
Get the latest AI workflows to boost your productivity and business performance, delivered weekly by expert consultants. Enjoy step-by-step guides, weekly Q&A sessions, and full access to our AI workflow archive.
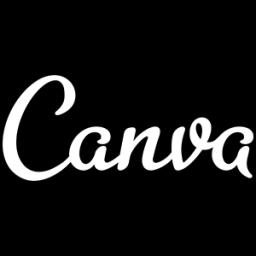
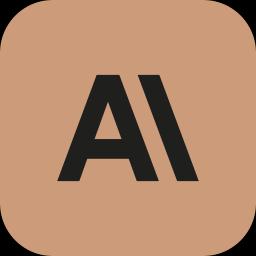
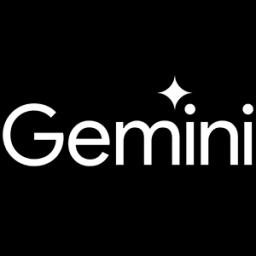
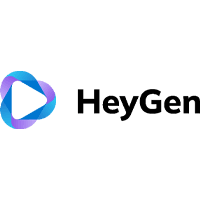
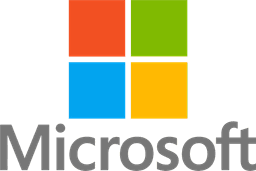
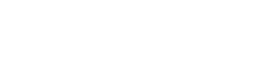
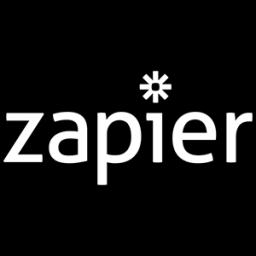
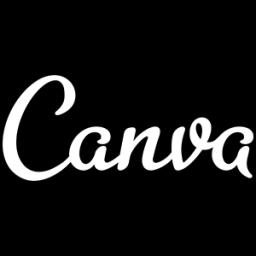
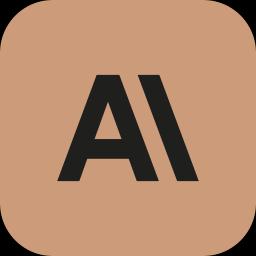
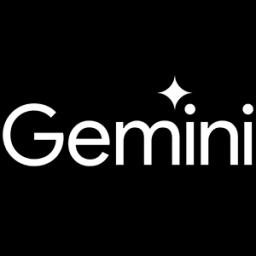
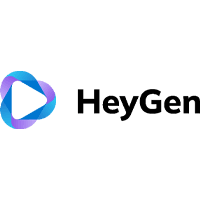
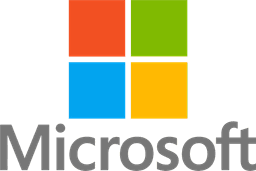
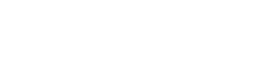
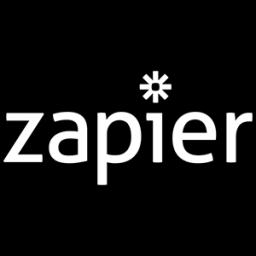
Other major players in the AI field are also exploring ways to refine AI reasoning capabilities. For example, OpenAI is working on enhancing its language models by implementing sophisticated reasoning techniques that resemble TPO's framework, focusing on boosting both the efficiency and accuracy of AI responses. Similarly, DeepMind's 'Socratic AI' is a project aimed at enabling AI systems to handle abstract reasoning and learn from complex tasks. This approach parallels TPO by seeking to deepen AI's reasoning skills to create more contextually aware models.
Experts in the AI realm are taking note of these advancements. Dr. Lance Eliot highlights the potential of TPO in transforming AI outputs across various sectors by reframing AI's processing of information. This enhancement could lead to AI systems that are not only more coherent but also offer contextually aware responses, which are crucial for industries like customer service and knowledge management. However, some critiques have pointed out the demanding nature of TPO, especially in domains like mathematical problem-solving, where its computational complexity may pose challenges. The balance between sophistication and practicality is emphasized as a key consideration for the future development of TPO and similar technologies.
Public reactions to TPO highlight a mixed sentiment. On one side, there's a strong appreciation for its potential to improve AI's logical and creative capabilities without additional human intervention. The analogy of 'showing your work' resonates well as it offers transparency in AI decision-making processes, enhancing trust. On the other hand, skeptics point to concerns related to the adequacy of existing data to support TPO's reasoning enhancement and whether this new form of AI reasoning truly constitutes intelligent thought or merely advanced pattern recognition. Discussions on social media often touch on the fear that such technologies could disrupt job markets focused on human judgment, even as its technical benefits are acknowledged.
Looking ahead, TPO and similar AI reasoning advancements bear significant implications. Economically, they could transform industries like customer service by providing more coherent and cost-effective AI solutions, although this may lead to disruptions in job markets dependent on human reasoning. Socially, TPO could redefine educational paradigms by enabling AI-driven learning models that simulate human reasoning processes, raising questions about potential over-reliance on technology. Politically, the improved reasoning capabilities of AI could revolutionize governance through enhanced decision-making, though it will require careful navigation of ethical considerations to ensure responsible AI deployment. Overall, the evolution of AI reasoning continues to challenge and reshape the interaction between technology and society.
Expert Opinions: Advantages and Limitations of TPO
Meta's Thought Preference Optimization (TPO) represents a significant leap in the field of artificial intelligence, specifically in enhancing the internal reasoning capabilities of generative AI models. One of the primary advantages highlighted by experts is TPO’s ability to refine AI's internal thought processes akin to human cognitive practices. This refinement aims to produce more coherent and contextually aware outputs, which can greatly benefit sectors like customer service and knowledge management. Dr. Lance Eliot and other professionals emphasize that by encouraging AI systems to "show their work," TPO aligns with educational methodologies that encourage transparency and accountability in reasoning. This method is transformative as it provides deeper insights into AI logic, potentially leading to more accurate and reliable responses. Moreover, TPO’s synthetic approach to logic generation can significantly enhance AI's thinking capabilities, opening new avenues for creativity and problem-solving across various domains.
Public Reception: Enthusiasm and Skepticism
Meta's recent advancements in AI, specifically through their Thought Preference Optimization (TPO), have sparked a spectrum of public reactions. On one hand, there is considerable enthusiasm. Many see TPO as a groundbreaking method that substantially elevates the coherence and quality of AI interactions. The public appreciates its potential in enhancing AI's reasoning capabilities without the need for constant human annotations, which could revolutionize fields that rely heavily on logical reasoning and creativity, such as marketing and education. The analogy of AI 'showing its work' resonates well with educational perspectives, enhancing transparency and trust in AI outputs.
Learn to use AI like a Pro
Get the latest AI workflows to boost your productivity and business performance, delivered weekly by expert consultants. Enjoy step-by-step guides, weekly Q&A sessions, and full access to our AI workflow archive.
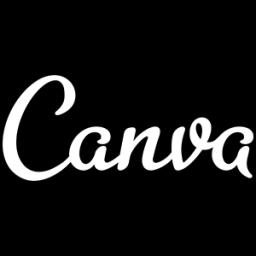
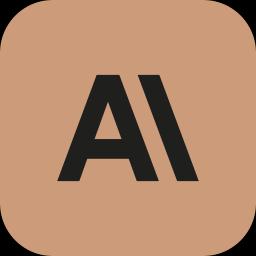
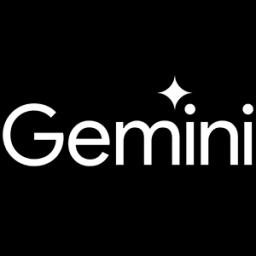
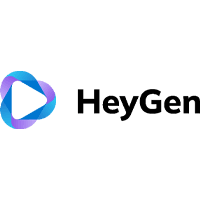
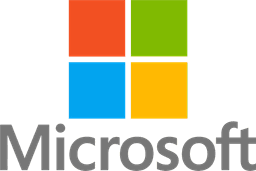
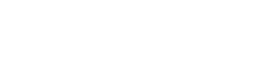
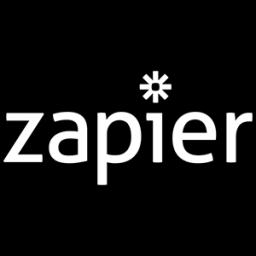
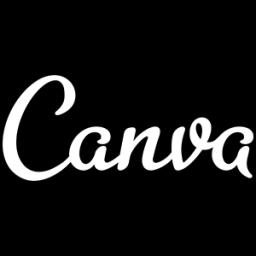
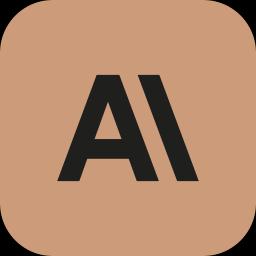
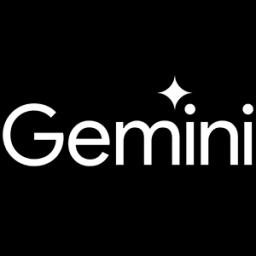
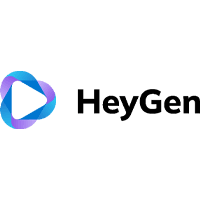
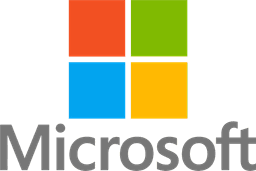
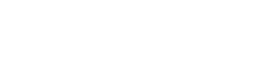
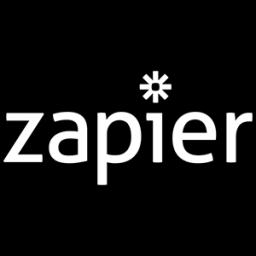
Conversely, skepticism exists alongside the excitement. Critics express concerns about the assumptions underlying the sufficiency of current data in logical reasoning. The concept of AI 'overthinking' potentially leading to greater computational demands is another issue. Moreover, debate continues over whether TPO truly represents AI 'thinking' or if it's merely sophisticated pattern recognition. Additionally, there's anxiety over how such advancements might impact job markets; particularly sectors that hinge on human judgment and decision-making. The dialogue about TPO's broader implications remains active and multifaceted, indicating that while its benefits are acknowledged, many are cautious about its integration and impact on various facets of society.
Future Implications: Economic, Social, and Political Impact
Meta's Thought Preference Optimization (TPO) introduces groundbreaking changes in generative AI, potentially transforming economic landscapes. By enhancing AI's logical reasoning and contextual understanding, businesses in industries like customer service and marketing can achieve unprecedented efficiency and cost-effectiveness, potentially reducing the need for human oversight. However, these advances also pose significant challenges, particularly in job markets where AI could replace roles dependent on human cognitive abilities, thereby unsettling entire sectors reliant on human judgment and decision-making.
In the social sphere, TPO fosters a revolutionary change in educational paradigms by enabling AI to simulate human-like reasoning processes. This advancement promises to better prepare students for an AI-integrated future, as they engage with technology that encourages critical thinking and problem-solving in educational settings. Despite these benefits, concerns about dependency on AI and the potential erosion of independent critical thinking skills among learners highlight the double-edged nature of this innovation.
Politically, TPO's development in AI reasoning holds transformative potential in governance and policymaking. Enhanced AI capabilities can streamline decision-making processes, making them more robust, efficient, and potentially reducing bureaucratic backlogs. Nevertheless, this shift also brings to the fore significant ethical considerations regarding transparency, accountability, and the extent to which AI can and should operate without human oversight in crucial governmental functions. As TPO technologies become more integrated, ensuring responsible AI deployment with stringent ethical guidelines becomes imperative to navigate the complexities of AI in politics.
Conclusion: Balancing Sophistication and Practicality
In conclusion, the introduction of Thought Preference Optimization (TPO) by Meta marks a significant milestone in the ongoing evolution of generative AI. The technique’s ability to enhance both the sophistication and practicality of AI systems represents a crucial step forward, especially in achieving a balance that addresses current technological limitations and societal needs. TPO acknowledges the challenge posed by existing data’s lack of explicit logical structure, thus providing a means to bridge this gap through synthesized internal reasoning.
The practicality of TPO is evident across multiple domains, from improving AI’s reasoning abilities in customer service to enhancing educational technologies. Nonetheless, the sophistication of this method does not come without its challenges; it demands considerable computational resources, which might affect efficiency. Furthermore, different sectors may require tailored adaptations of TPO to fully leverage its potential, particularly where rapid response times are critical.
Learn to use AI like a Pro
Get the latest AI workflows to boost your productivity and business performance, delivered weekly by expert consultants. Enjoy step-by-step guides, weekly Q&A sessions, and full access to our AI workflow archive.
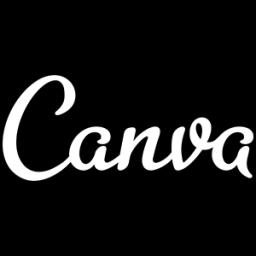
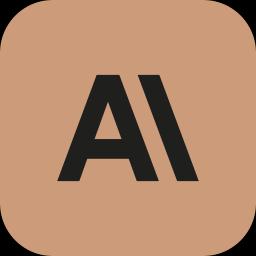
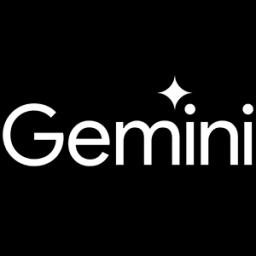
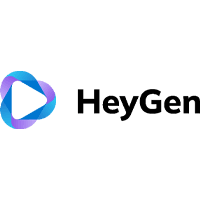
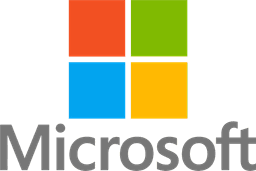
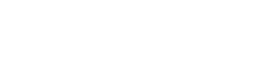
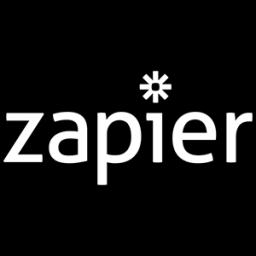
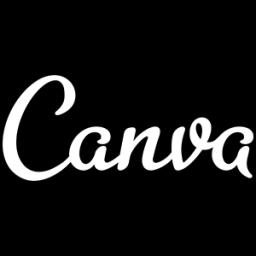
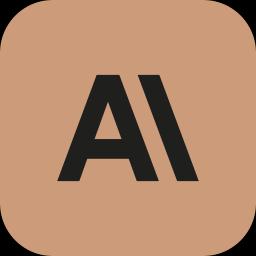
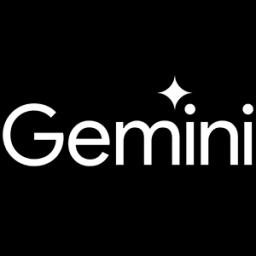
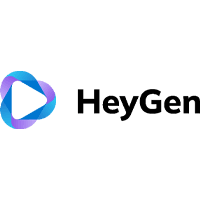
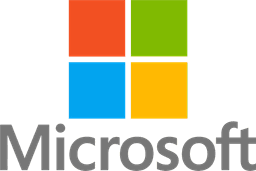
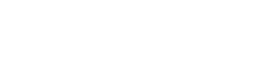
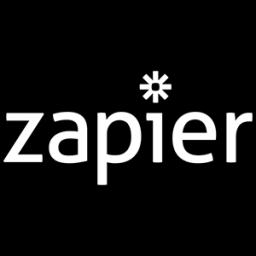
The balance between TPO's sophistication and practical application also raises broader considerations about how AI technologies can be responsibly adopted on a global scale. As AI gains the ability to simulate more complex cognitive processes, it’s imperative to ensure these advancements are aligned with ethical guidelines. The debates surrounding AI's impact on employment, the preservation of human cognitive skills, and governance systems illustrate the intricate dynamics at play.
Ultimately, as we anticipate future advancements, the key will be to synthesize TPO’s ambitious capabilities with everyday practical needs. This balance is crucial to drive the AI industry forward—ensuring that ground-breaking innovations do not outpace our capacity to implement them effectively within societal structures. Thought Preference Optimization could very well redefine the frontier of AI capabilities, provided it is harnessed thoughtfully and inclusively.