Innovating the Future of AI with Microsoft Phi-4
Microsoft Unveils Phi-4: The New Math Whiz in AI Models!
Last updated:

Edited By
Mackenzie Ferguson
AI Tools Researcher & Implementation Consultant
Microsoft takes AI to the next level with the introduction of Phi-4, a 14 billion parameter generative model that's making waves in mathematical problem-solving. Known for its efficiency and small size, Phi-4 integrates high-quality synthetic and human-generated datasets to pack a punch. Access is limited to research preview on Azure AI Foundry, sparking interest and intrigue, especially following the departure of key developer Sébastien Bubeck.
Introduction to Phi-4
Microsoft's latest generative AI model, Phi-4, is a remarkable advancement in their line of AI models, known as the Phi family. This model represents a significant step in AI technology, particularly in its ability to solve complex mathematical problems. Unlike its predecessors, Phi-4 integrates high-quality synthetic data with human-generated content, which enhances its performance significantly. It boasts a 14 billion parameter architecture, allowing it to handle complex symbolic reasoning tasks efficiently.
Currently, Phi-4 is available under limited conditions, specifically for research purposes on Microsoft's Azure AI Foundry platform. The release of this model occurs under a Microsoft research license agreement, indicating the company's cautious approach towards its distribution and the potential implications it has on the broader AI landscape. The restricted access, however, opens up conversations about Microsoft's strategies in AI research and development, especially with the departure of Sébastien Bubeck, a key developer of previous models in the Phi series.
Learn to use AI like a Pro
Get the latest AI workflows to boost your productivity and business performance, delivered weekly by expert consultants. Enjoy step-by-step guides, weekly Q&A sessions, and full access to our AI workflow archive.
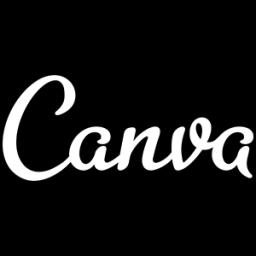
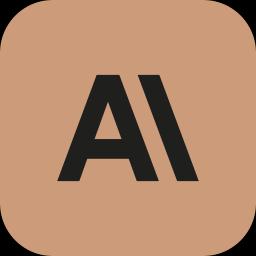
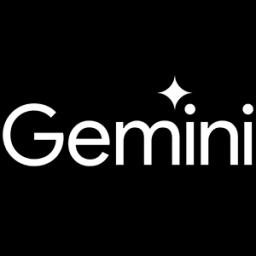
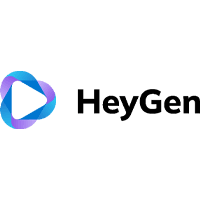
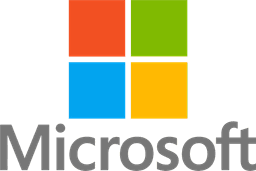
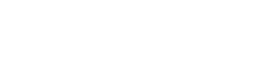
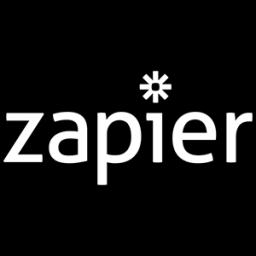
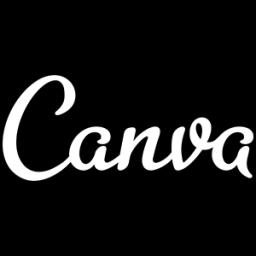
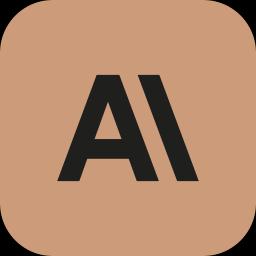
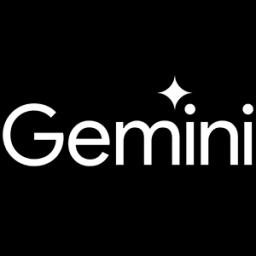
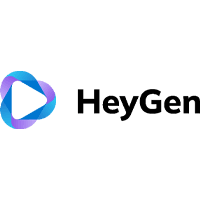
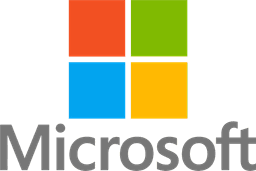
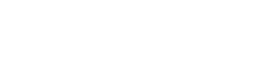
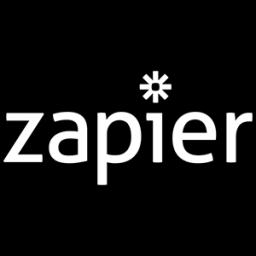
The advancements of Phi-4 compared to earlier models are primarily centered on its improved capabilities in mathematical reasoning. Its efficiency and capacity to perform complex computations make it a strong competitor among other small language models like GPT-4o mini and Claude 3.5 Haiku. While full accessibility remains limited, its potential for impacting fields that rely on mathematical precision is significant.
In the broader AI market, Phi-4 stands as a testament to Microsoft's commitment to refining AI models that are both robust and efficient. The ongoing challenges in AI, such as overcoming the pre-training data wall and leveraging synthetic datasets effectively, highlight this model's significance in tackling such hurdles. As such, Phi-4 does not just reflect technological progress but also marks a pivotal point in AI model development, particularly in how companies approach model training and deployment amidst resource constraints and scaling difficulties.
Advancements in Mathematical Problem-Solving
Microsoft's recent launch of the Phi-4 generative AI model marks a significant leap in mathematical problem-solving capabilities. This model is part of the Phi series and features an architecture with 14 billion parameters, making it adept in solving complex mathematical problems. Despite its formidable capacity, Phi-4 is accessible only under certain conditions, as it is currently available for research purposes on Microsoft's Azure AI Foundry.
The introduction of Phi-4 has sparked interest in its role amid the competitive landscape of small language models, such as GPT-4o mini and Claude 3.5 Haiku. These models are known for their efficiency and affordability, yet Phi-4 distinguishes itself through its powerful math-solving ability. The reliance on high-quality synthetic datasets, combined with human-generated data, enhances its effectiveness, a crucial advancement considering the scaling difficulties faced by AI models.
Learn to use AI like a Pro
Get the latest AI workflows to boost your productivity and business performance, delivered weekly by expert consultants. Enjoy step-by-step guides, weekly Q&A sessions, and full access to our AI workflow archive.
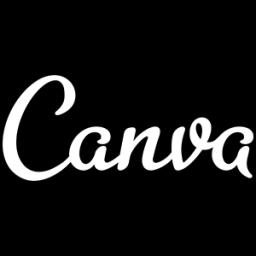
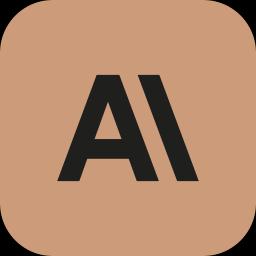
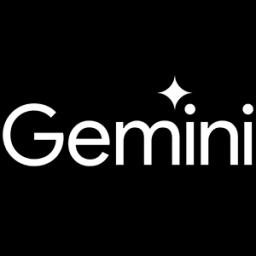
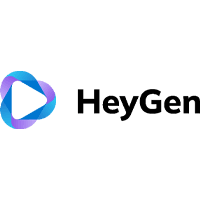
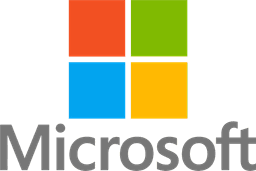
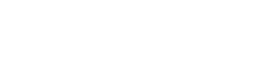
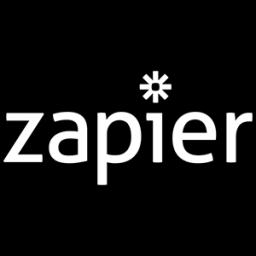
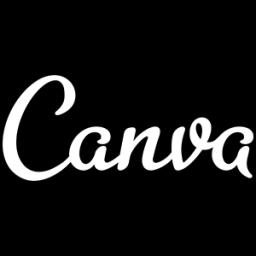
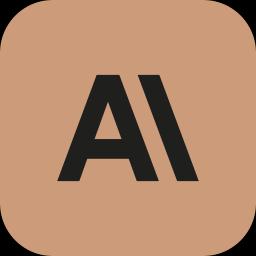
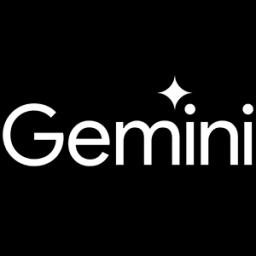
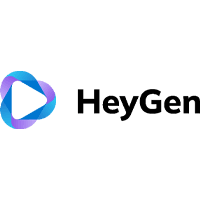
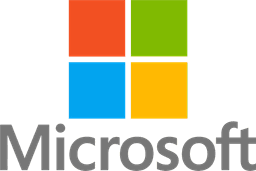
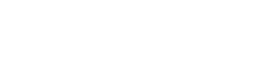
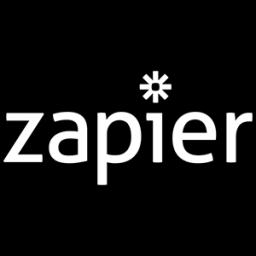
A pivotal developer, Sébastien Bubeck, recently left Microsoft, shifting to OpenAI, which presents questions about potential strategic shifts at Microsoft. His departure highlights broader industry challenges, such as the need for independent innovation in light of difficulties in pre-training data acquisition. The ongoing race for better AI capabilities often involves tackling these data-related barriers.
Furthermore, Natural Language Embedded Programs (NLEPs) are revolutionizing the field by enabling AI to generate and execute Python programs, thus improving accuracy and transparency in symbolic reasoning tasks. This development ties into the industry's focus on advancing mathematical reasoning capabilities, further contributing to the discourse around Phi-4 and similar models.
Overcoming the challenges faced by scaling large language models is crucial. As researchers face diminishing returns from merely expanding size, a transformation focusing on enhancing inference capabilities might reshape how resources are allocated, potentially altering the AI hardware market. This change indicates a shift from the 'bigger is better' mantra to more strategic and efficient model development, where models like Phi-4 become central actors.
Integration of Synthetic Datasets
Synthetic datasets have become increasingly pivotal in the landscape of AI model training, especially with the development of sophisticated language models like Microsoft's Phi-4. These datasets are not just supplements but critical components that enhance model performance by providing varied and extensive data that might be difficult to gather through traditional means. In the case of Phi-4, the integration of high-quality synthetic data alongside human-generated content appears to be a strategic move to improve the model's capabilities in mathematical reasoning and other complex tasks.
The reliance on synthetic datasets addresses significant challenges in the AI industry, such as the 'pre-training data wall,' where acquiring sufficient real-world data for training becomes a bottleneck. By simulating a broader spectrum of data scenarios, synthetic datasets enable models like Phi-4 to develop robust problem-solving capabilities without the extensive need for potentially biased or limited human data. This innovative approach is not only improving model reliability but also setting a new standard in AI research methodologies.
Furthermore, the use of synthetic datasets reflects a shift towards more efficient model development practices. As models continue to grow in scale and complexity, the ability to train them effectively on diverse datasets becomes crucial. This process streamlines the deployment of AI systems capable of handling diverse and unforeseen challenges in the real world, thereby expanding their applicability and potential uses across various fields.
Learn to use AI like a Pro
Get the latest AI workflows to boost your productivity and business performance, delivered weekly by expert consultants. Enjoy step-by-step guides, weekly Q&A sessions, and full access to our AI workflow archive.
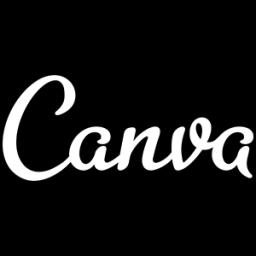
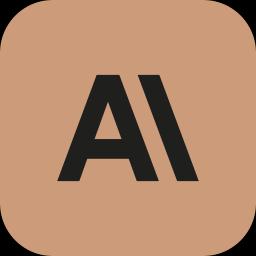
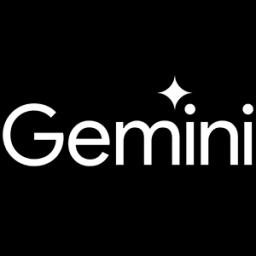
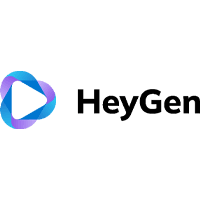
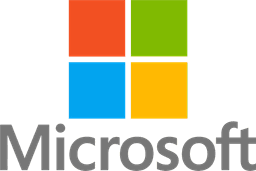
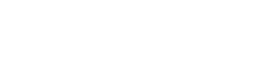
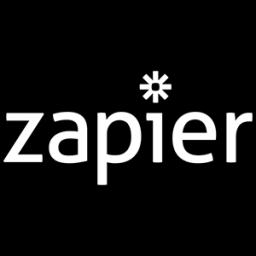
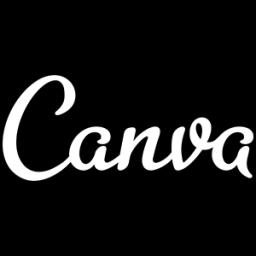
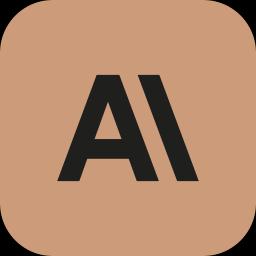
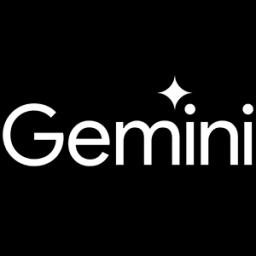
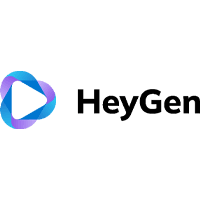
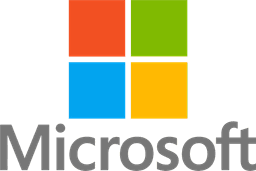
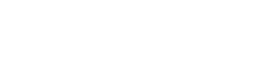
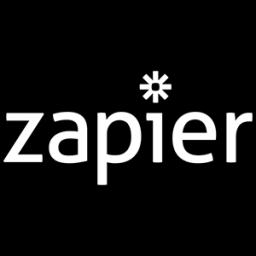
The strategic deployment of synthetic datasets in training leading AI models, such as Phi-4, also raises critical questions about data ethics and integrity. Ensuring the quality and bias mitigation in synthetic datasets is essential to maintain trust and efficacy in AI outputs. As the industry advances, so too must the frameworks that oversee the creation and use of these datasets, ensuring they uphold the highest standards of fairness and accuracy.
Ultimately, the integration of synthetic datasets into AI training regimes is a testament to the evolving landscape of artificial intelligence, where innovation meets necessity. As companies and researchers continue to harness these tools, there is potential for groundbreaking advancements in AI capabilities, driving efficiency and excellence across multiple domains of application.
Research-Oriented Release and Accessibility
Microsoft's unveiling of the Phi-4, a new generative AI model, has not only marked a significant leap in their AI family's evolutionary path but also showcased a strategic shift towards using high-quality synthetic datasets. With a robust 14 billion parameter architecture, Phi-4 excels in mathematical problem-solving, setting itself apart by blending synthetic data with human-generated content. This blend enhances its performance, particularly in handling complex math tasks with higher accuracy than many existing models. The controlled accessibility of Phi-4, restricted primarily for research under Microsoft's Azure AI Foundry platform, suggests a cautious yet deliberate focus on research-oriented developments.
The Phi-4 model's release heralds a new era of competitiveness in the small language model market. It's poised to rival models like GPT-4o mini and Claude 3.5 Haiku, thanks to its unparalleled efficiency and performance. While its availability remains limited, these restrictions are indicative of its trial phase under Microsoft's research license agreements. Meanwhile, the tech industry is abuzz with speculation over potential changes in Microsoft's AI strategy, especially following the exit of essential developer Sébastien Bubeck. These developments are set against the backdrop of broader challenges, including data acquisition hurdles and the imperative of innovative data usage in AI scaling efforts.
The introduction of Natural Language Embedded Programs (NLEPs) marks a groundbreaking approach in enhancing mathematical problem-solving within AI. This technique empowers Phi-4 to generate and execute Python programs, significantly boosting its symbolic reasoning capabilities. The incorporation of synthetic data into training regimens reflects a broader industry trend towards smaller, more efficient models, as companies like OpenAI pivot to alternative data strategies amidst scaling challenges. The approach signifies a move away from the traditional "bigger is better" mindset, acknowledging the limitations faced with expanding model sizes.
Experts have lauded the release of Microsoft's Phi-4 model, highlighting its strategic architecture focused on efficiency. Andrew Ng from JPMorgan praises its smaller size, achieving competitive mathematical reasoning capabilities while optimizing computational resource use. In contrast, MIT's Emilia Rongve stresses the importance of comprehensive testing to ensure scalability across varied applications without compromising accuracy. Google AI expert Jean Liu affirms the strategic pivot to synthetic datasets, viewing it as a pivotal move in enhancing post-training adjustments and reducing data contamination risks. These expert opinions underscore the multifaceted impact of Phi-4 on the AI landscape.
Learn to use AI like a Pro
Get the latest AI workflows to boost your productivity and business performance, delivered weekly by expert consultants. Enjoy step-by-step guides, weekly Q&A sessions, and full access to our AI workflow archive.
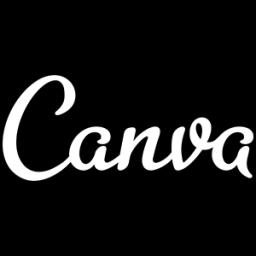
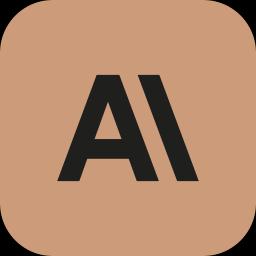
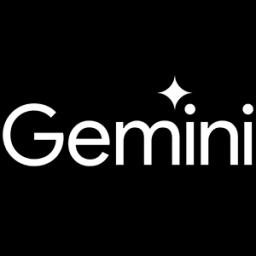
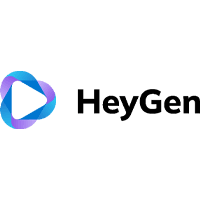
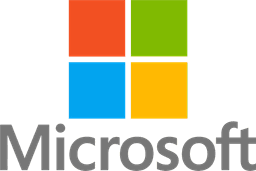
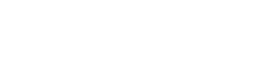
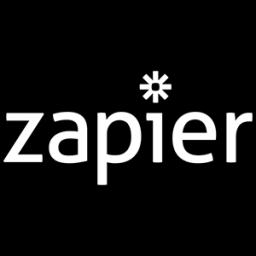
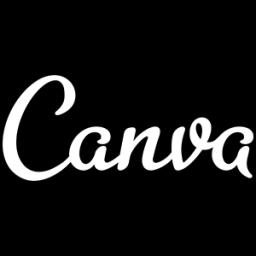
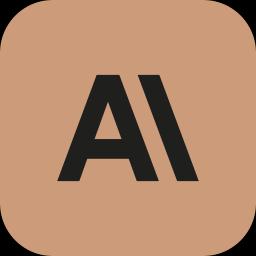
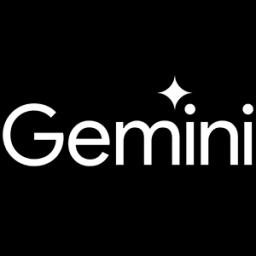
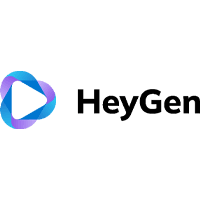
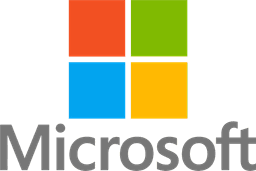
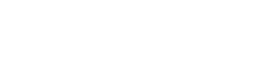
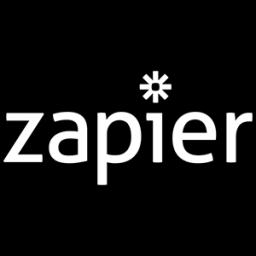
Public response to the Phi-4 model has been a mix of excitement and frustration. Enthusiasts commend its advanced performance, which rivals larger models despite its smaller size, making it an attractive option for mid-sized companies. However, its limited release, available only through a research preview under specific license agreements, has disappointed developers eager for broader usage. This sentiment is echoed across online communities, highlighting a desire for more accessible tools. The uncertainty introduced by Sébastien Bubeck's departure also casts a shadow over its future, fueling discussions on its developmental trajectory.
Looking ahead, the launch of Phi-4 signals substantial potential impacts across various sectors. Economically, its high efficiency and lower computational demands could revolutionize industries dependent on precise mathematical calculations, from finance to engineering. Socially, the democratization of such AI capabilities might promote wider accessibility, although its limited release could slow adoption and erode public trust. Politically, the reliance on synthetic datasets may spark regulatory debates on data ethics and integrity. Furthermore, internal shifts, like Bubeck's departure, could influence policy discussions around tech talent retention. Phi-4's debut thus encapsulates both innovation and the imperative for adaptive strategies to harness its full societal benefits.
Market Competition and Comparison with Other Models
The release of Phi-4 signifies a crucial development in Microsoft's journey through the competitive AI landscape. Comparatively, Phi-4's strengths lie in its specialized application for advanced mathematical tasks, an area where other models such as GPT-4o mini and Claude 3.5 Haiku might not focus as intensely. However, these models have their own unique strengths, such as GPT-4o mini's linguistic versatility and Claude 3.5 Haiku's creative cognition capabilities.
Market dynamics are influenced by Phi-4's entry, particularly due to its focus on efficient computational use, challenging the prevalent 'bigger is better' approach. This paradigm shift supports the trend towards more accessible AI models that mid-sized enterprises can adopt without incurring exponential computational costs. The allusion to 'efficiency kings' by experts reflects a growing appreciation within the AI field for high-performance models that do not excessively burden resources.
The constrained access underlines Microsoft's strategic positioning in AI research, prioritizing innovation and quality applications over widespread, unrestrained availability. This has its drawbacks, as seen in the reaction from AI developers and enthusiasts who crave broader access. Nevertheless, this approach ensures rigorous testing and refinement, supporting Microsoft's long-term objective to maintain cutting-edge technological leadership.
Speculation about the impact of influential developers leaving can have tangible ripple effects in market perception and trust, potentially influencing competitive tactics of other tech giants. Meanwhile, the ongoing conversation around the integration of synthetic datasets signifies potential market shifts towards robust, hybrid data training techniques which benefit models like Phi-4.
Learn to use AI like a Pro
Get the latest AI workflows to boost your productivity and business performance, delivered weekly by expert consultants. Enjoy step-by-step guides, weekly Q&A sessions, and full access to our AI workflow archive.
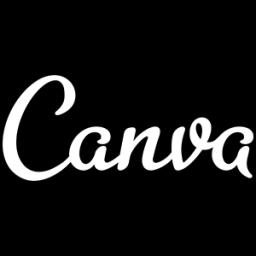
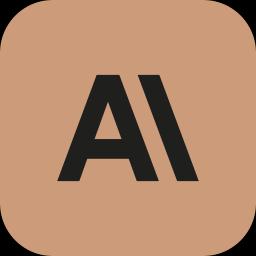
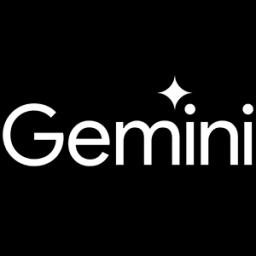
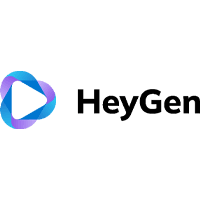
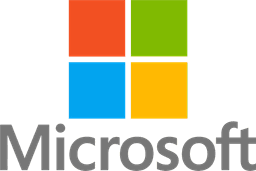
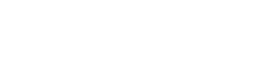
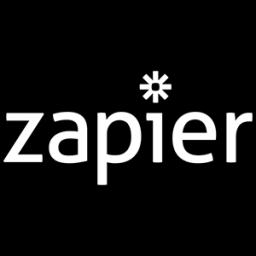
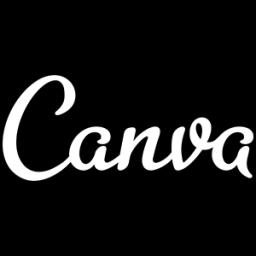
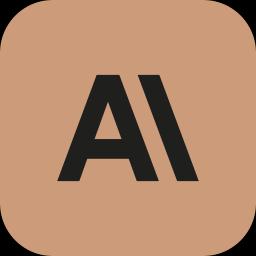
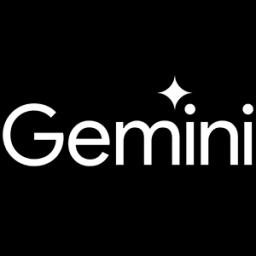
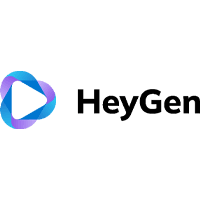
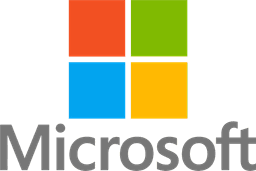
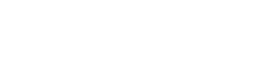
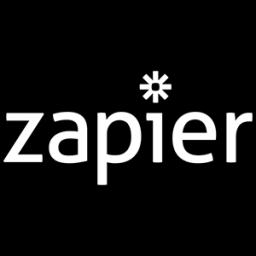
Overall, Phi-4 not only adds a robust tool to Microsoft's AI offerings but also encourages discourse on effective resource utilization, a topic gaining importance as the industry grapples with the pre-training data wall. By offering a competitive edge in specific domains like mathematical reasoning, Phi-4 helps redefine efficiency in model development, projecting a future where smart design could arrange smaller, highly effective models against larger but less nimble counterparts.
Departure of Sébastien Bubeck and its Implications
The recent departure of Sébastien Bubeck from Microsoft to join OpenAI has stirred considerable discussions about its impact on Microsoft's AI strategy, particularly its Phi series of models. Bubeck was instrumental in the development of the earlier models, and his exit poses questions about the future direction and innovation within the Phi model lineup. His profound expertise and pivotal role in shaping Microsoft’s AI tools make his transition a significant event that could potentially disrupt existing projects.
Microsoft's launch of Phi-4, a model released shortly after Bubeck's departure, marks a notable development not just in terms of technological advancement, but also in how Microsoft might navigate the challenges without one of its key AI figures. The model showcases enhanced performance in mathematical reasoning tasks and distinguishes itself with the use of high-quality synthetic datasets to bolster its training. However, the absence of Bubeck raises concerns among industry analysts about the potential shifts in strategy and the continuity of innovative momentum within the company.
The implications of Bubeck's move to OpenAI are multifaceted. There might be a potential exchange of knowledge and collaboration between different AI entities, fostering a more dynamic research environment. Nevertheless, this shift could also intensify competitive tensions. OpenAI, benefiting from his expertise, might leverage his insights to further advance their projects, potentially affecting Microsoft's standing and progression in the field of generative AI.
The industry observes Bubeck’s departure with a mix of curiosity and concern, understanding that such movements in key personnel can herald significant changes in a company’s policy and product development approach. Stakeholders are keenly watching how Microsoft adapts to his exit and whether they will be able to uphold the competitive edge in AI innovation that has been a significant aspect of their growth narrative.
Industry-Wide Challenges and Innovations
The tech industry is constantly evolving, with companies like Microsoft at the forefront, pushing the boundaries of what artificial intelligence (AI) can achieve. One of the central challenges faced by the AI sector is the "pre-training data wall," a metaphorical barrier that limits the amount of quality data available for training effective models. This shortage has driven innovation, particularly in the development and use of synthetic datasets. Synthetic data, which is artificially generated rather than collected from real-world environments, offers a compelling alternative, helping to bypass traditional data limitations while maintaining algorithmic efficacy. This method not only serves as a stopgap solution for data shortages but also opens up possibilities for more controlled and bias-mitigated training processes.
Learn to use AI like a Pro
Get the latest AI workflows to boost your productivity and business performance, delivered weekly by expert consultants. Enjoy step-by-step guides, weekly Q&A sessions, and full access to our AI workflow archive.
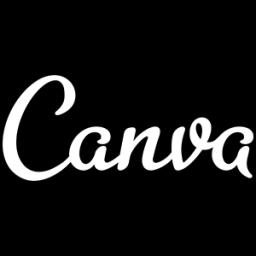
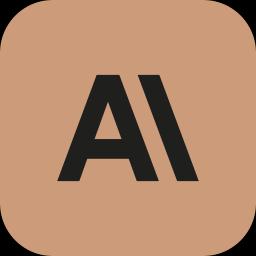
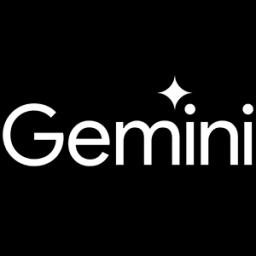
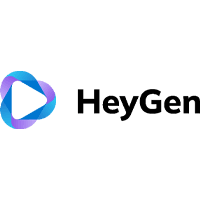
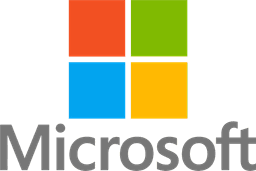
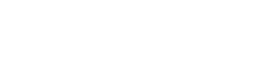
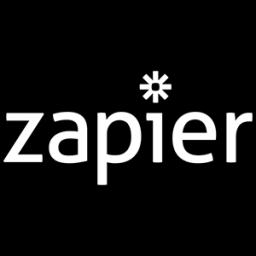
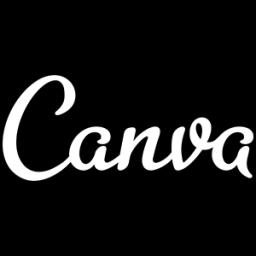
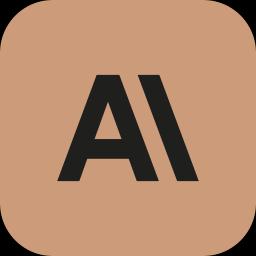
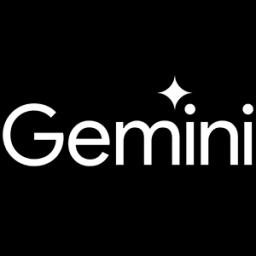
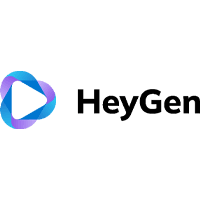
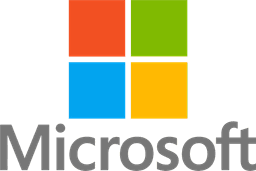
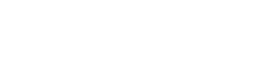
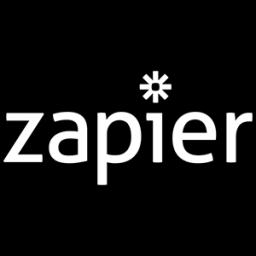
Another crucial challenge within the industry is scaling AI models. The "bigger is better" philosophy, which advocates for increased parameters to boost model performance, is facing scrutiny as it reaches its practical limits. Increasing parameters leads to higher computational costs and energy consumption, contributing to unsustainable environmental impacts. In response, AI researchers are exploring new approaches, focusing on refining inference capabilities to optimize performance without further scaling. This paradigm shift could drive significant changes in how AI models are developed, encouraging efficiency and sustainability over sheer model size.
In tandem with addressing challenges, innovation thrives. Microsoft's recent release of the Phi-4 model exemplifies this balance between overcoming obstacles and pioneering advancements. Phi-4 leverages smaller architecture while outperforming many larger counterparts in mathematical reasoning, signaling a notable shift towards efficiency and specialization. This development is set against the broader backdrop of a competitive market where new techniques like Natural Language Embedded Programs (NLEPs) are enhancing AI's ability to solve complex mathematical problems by allowing models to execute Python scripts for more precise symbolic reasoning.
Furthermore, the integration of synthetic datasets has demonstrated substantial promise, as seen with Phi-4's training regimen, which combines synthetic and human-generated data. This hybrid approach not only aids Phi-4's superior performance but also sets a precedent for future models striving to navigate data availability challenges more effectively. As AI technology continues to evolve, the industry's capacity to innovate in data synthesis and processing will likely define its success in addressing scalability and efficiency issues.
The dynamic landscape of AI innovation is also characterized by evolving competitive dynamics. For instance, smaller models like Phi-4 are emerging as fierce competitors to existing large language models, offering cost-efficient alternatives to businesses eager to harness AI without the expansive resources traditionally required. This shift may intensify market competition, pushing companies to not only innovate technologically but also strategically position their products to meet the growing demands for sophisticated yet accessible AI solutions.
Expert Opinions on Phi-4
Experts across the tech industry have offered a range of opinions on Microsoft's new Phi-4 model. One of the standout features of Phi-4 is its 14 billion parameter architecture, specifically designed to tackle complex mathematical problems. Andrew Ng, Chief AI Scientist at JPMorgan, commends Phi-4's architecture for being a game-changer in efficiency. He highlights how this model, despite having fewer parameters compared to larger models, excels in benchmarks for mathematical reasoning. This advancement showcases a strategic move by Microsoft to optimize both computational resources and operational costs without sacrificing performance.
However, some experts approach the advancements with caution. Emilia Rongve from MIT raised concerns about the scalability of this smaller model across various applications, emphasizing the need for thorough testing to ensure its accuracy and reliability. This input reflects a crucial perspective on whether the model's design can withstand diverse real-world challenges without compromising on quality.
Learn to use AI like a Pro
Get the latest AI workflows to boost your productivity and business performance, delivered weekly by expert consultants. Enjoy step-by-step guides, weekly Q&A sessions, and full access to our AI workflow archive.
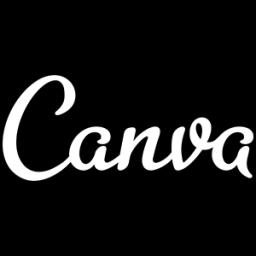
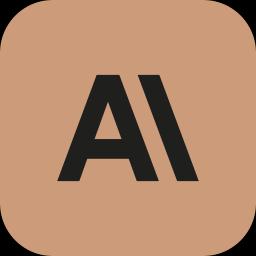
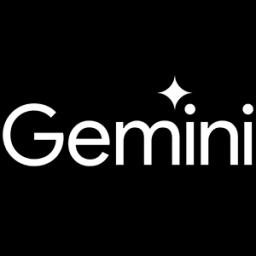
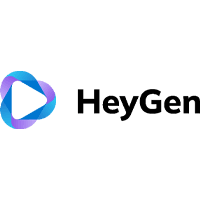
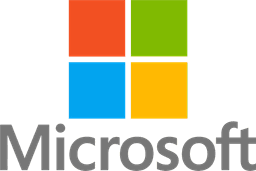
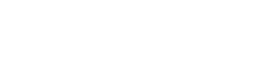
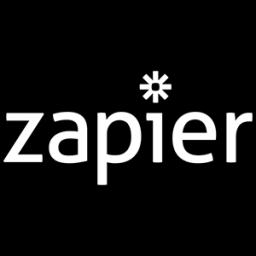
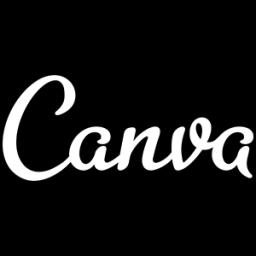
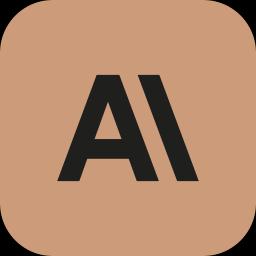
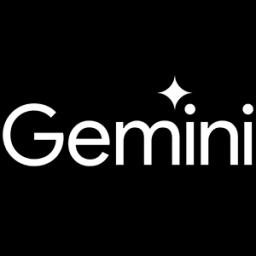
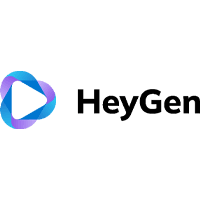
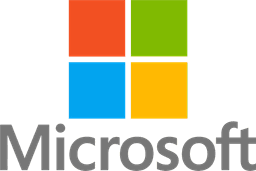
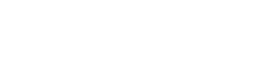
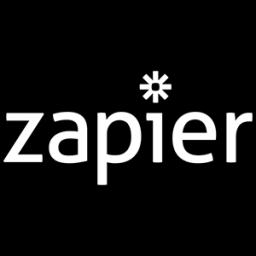
Another element that sparks expert interest is the use of high-quality synthetic datasets in Phi-4. Jean Liu from Google AI points out that this is an excellent strategy to combat data contamination. The incorporation of synthetic datasets alongside traditional human-generated data may lead to more consistent improvements in AI capabilities, particularly in post-training stages. This development in data strategy reflects a growing trend in the AI field towards using synthetic data to enhance learning outcomes.
Public Reactions to Phi-4
Microsoft's announcement of their latest language model, Phi-4, has elicited a wide array of reactions from the public. A significant portion of the tech community has lauded its mathematical problem-solving prowess, especially noting its superior performance compared to larger models like Google's Gemini Pro 1.5. Its efficient architecture, combined with a smaller size, is particularly appealing to mid-sized companies that might benefit from high performance without incurring the infrastructure costs typically associated with larger models.
However, not all feedback has been entirely positive. Many developers express frustration over Phi-4's limited availability, as its release remains strictly for research purposes on the Azure AI Foundry platform. This restriction has been a point of contention, as it prevents broader experimentation and real-world application by developers who wish to leverage its capabilities in various projects. Online communities have been vocal about these limitations, highlighting a widespread demand for more open access.
In various forums such as Reddit's r/LocalLLaMA, Phi-4's performance has been compared with its predecessors. While it has received nods of approval for niche, task-specific efficiency, some users continue to prefer more generalized language models such as Llama 3 for everyday use, indicating a divide in user preferences between hyper-specialization and versatility. Brought to attention is the uncertainty surrounding the development of the Phi series, following the departure of Sébastien Bubeck, a key figure in its creation, which adds a layer of unpredictability regarding the model's evolution and refinement.
Future Implications and Societal Impact
The introduction of Microsoft's Phi-4 model is set to significantly shape future advancements in AI technology and its societal impact. One of the primary implications is in economic sectors leveraging AI for complex computational tasks. Industries such as finance, engineering, and scientific research are likely to benefit from Phi-4’s enhanced capabilities in mathematical reasoning and efficiency. This can potentially lead to a reduction in operational costs, making high-performance AI solutions more accessible to smaller companies that traditionally struggle to afford cutting-edge technology.
Furthermore, Phi-4’s development could be a driving force in democratizing AI technology, offering a wider range of users the ability to solve diverse real-world challenges through AI-driven solutions. However, this democratization is currently hampered by the model's limited access, available primarily under research licenses. If accessibility broadens, it might inspire greater public trust and integration of AI in everyday applications, to the benefit of many societal sectors.
Learn to use AI like a Pro
Get the latest AI workflows to boost your productivity and business performance, delivered weekly by expert consultants. Enjoy step-by-step guides, weekly Q&A sessions, and full access to our AI workflow archive.
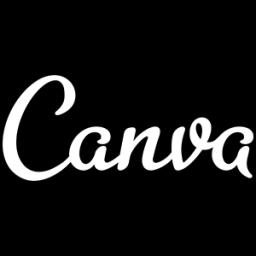
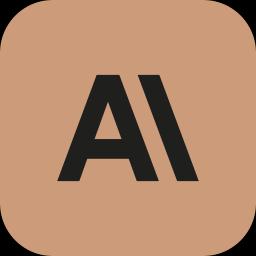
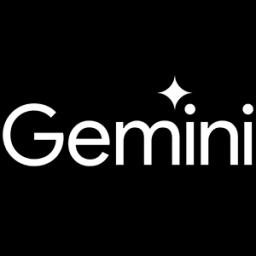
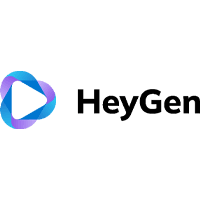
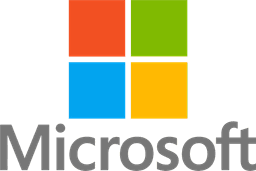
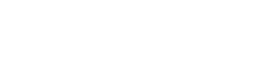
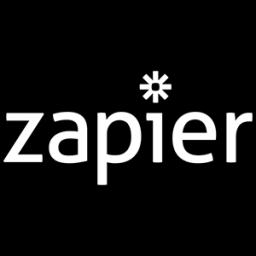
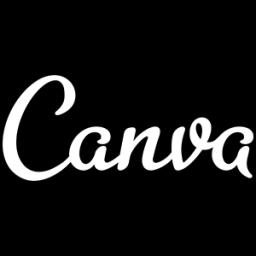
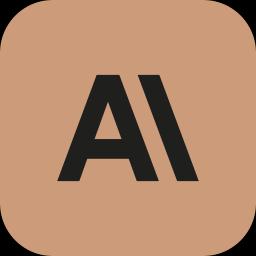
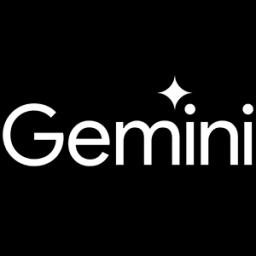
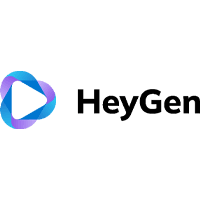
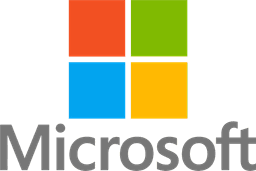
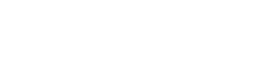
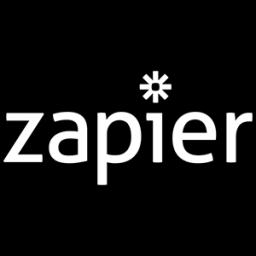
On a socio-political level, Phi-4's reliance on synthetic datasets raises pivotal questions about the ethical use of AI data, potentially igniting regulatory discussions. This situation could prompt the establishment of new guidelines to ensure the ethical and unbiased use of AI, protecting data integrity and fairness in automated systems. Additionally, the model’s launch, following key developer departures like that of Sébastien Bubeck, could influence broader discussions on technological talent retention and the governance of intellectual property in AI development.
Overall, while Phi-4 showcases significant technological advancements, it also highlights existing challenges that must be strategically addressed to maximize the societal benefits of AI. Ensuring that these technologies are equitable and ethically managed will be vital as they increasingly integrate into various aspects of human life.