The Silent Treatment
OpenAI's GPT-4: Unmasking the Mystery Behind the Silence
Last updated:

Edited By
Mackenzie Ferguson
AI Tools Researcher & Implementation Consultant
Dive into the enigmatic world of OpenAI's GPT-4 architecture. From whispers of MoE designs to the challenges of scaling, why is OpenAI keeping mum? We've got the insights and expert takes you need to understand the tech world's latest AI debate.
Introduction to GPT-4 and OpenAI's Transparency
GPT-4, developed by OpenAI, has created a buzz in the AI community not only for its capabilities but also due to the secrecy surrounding its detailed architecture. Despite the widespread curiosity, the company has remained tight-lipped about the specifics of GPT-4, leading to speculation and debate among experts and users alike. Some believe that this lack of transparency hinders scientific research and impedes the ability to accurately assess the model's performance and potential biases. On the other hand, OpenAI's strategic silence may be a protective measure in a highly competitive AI industry where proprietary technology is a valuable asset.
At the core of OpenAI's GPT-4 is what many believe to be a Mixture of Experts (MoE) architecture, a sophisticated setup where numerous expert models work in tandem. This involves multiple models, each possibly specialized in tasks such as coding or interpreting images, combining their capabilities to enhance overall performance. However, this configuration is not without its challenges. The MoE architecture, though beneficial for specialization, significantly escalates operational costs due to the extensive computational power required for its iterative inference processes.
Learn to use AI like a Pro
Get the latest AI workflows to boost your productivity and business performance, delivered weekly by expert consultants. Enjoy step-by-step guides, weekly Q&A sessions, and full access to our AI workflow archive.
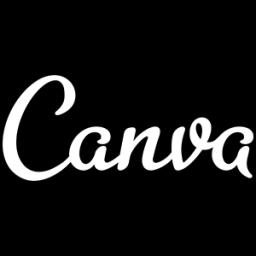
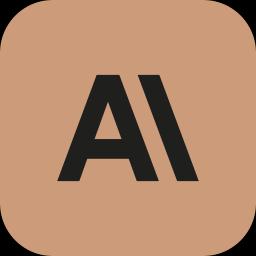
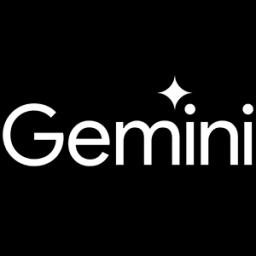
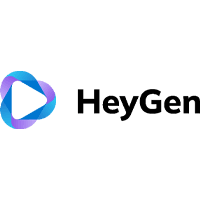
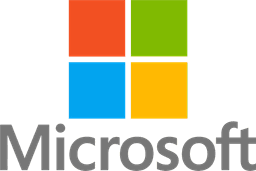
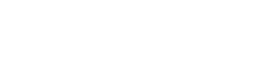
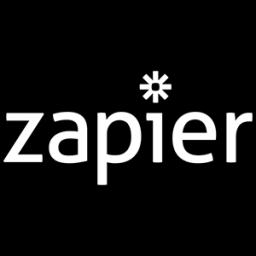
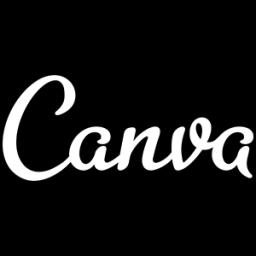
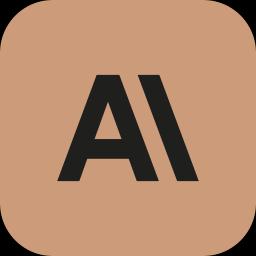
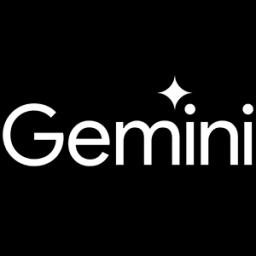
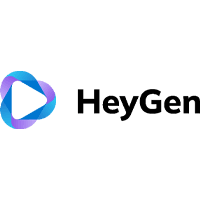
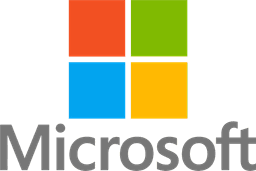
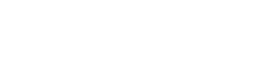
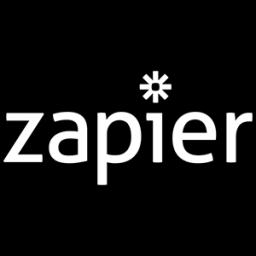
Experts and users have reported various challenges associated with GPT-4's design. These highlight the complexities of scaling such a vast system while maintaining efficiency. Reports of increased cloud costs and a perceived decline in the quality of outputs for applications such as ChatGPT have sparked criticism and concern from the community. Some speculate that the iterative nature of the model's processes, potentially repeated up to 16 times, might be contributing to these issues, necessitating a careful balance between performance and resource consumption.
Public and expert opinion is divided on the impact of OpenAI's non-disclosure on GPT-4. While some praise it as a necessary step to maintain competitive advantage, others call for more openness to foster research and trust in AI technology. User experiences with GPT-4, especially with services like ChatGPT, illustrate this divide. Numerous users claim decreased performance, outlining issues such as factual errors and less inventive responses. Conversely, some have observed improvements or no significant downgrade, suggesting varying expectations and experiences impact perceptions.
OpenAI's stance on transparency has prompted wider discussions about ethics and responsibility in AI. As AI continues to integrate more deeply into society, the call for openness and accountability grows louder. This debate underscores a larger movement within the AI community, pushing for industry standards that balance innovation with ethical considerations. The future will likely see greater pressure on AI companies to disclose more about their technologies, fostering an environment where both proprietary advantages and public interest are respected.
Understanding Mixture of Experts (MoE) Architecture
The Mixture of Experts (MoE) architecture represents a significant advancement in artificial intelligence, providing a novel approach to handling complex tasks by leveraging multiple specialized models. In the context of OpenAI's GPT-4, MoE allows for the integration of eight distinct models, each boasting 220 billion parameters, to form a collective intelligence. This setup is advantageous as it enables the specialization of each expert network within the architecture, addressing specific sub-tasks or domains with precision and efficiency.
Learn to use AI like a Pro
Get the latest AI workflows to boost your productivity and business performance, delivered weekly by expert consultants. Enjoy step-by-step guides, weekly Q&A sessions, and full access to our AI workflow archive.
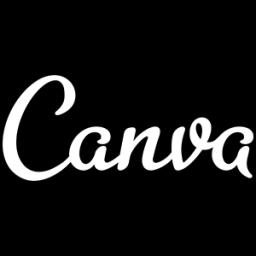
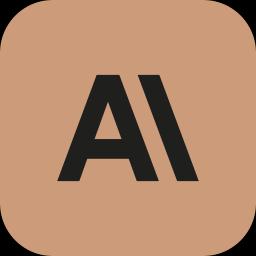
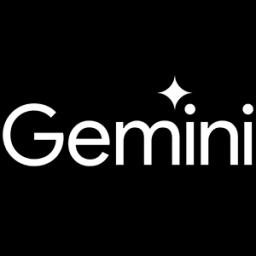
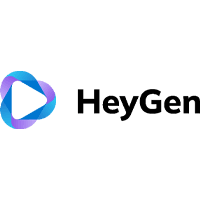
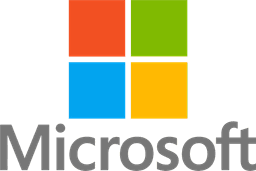
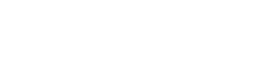
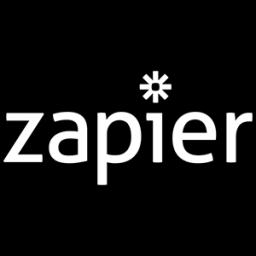
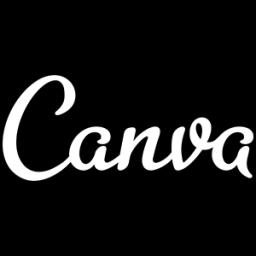
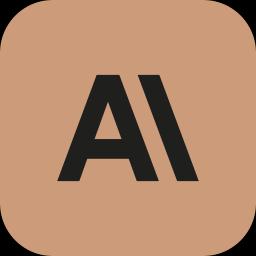
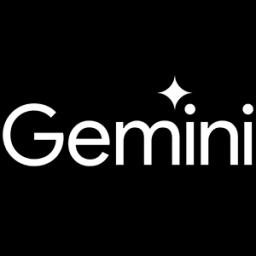
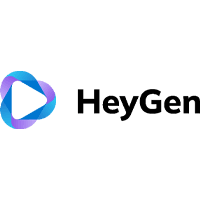
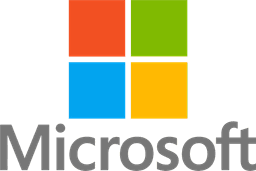
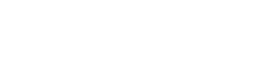
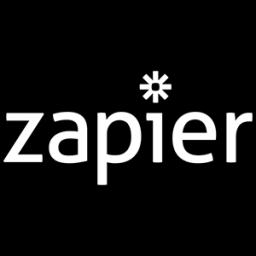
However, employing MoE architectures comes with its own set of challenges, particularly in terms of operational cost and complexity. The need for iterative inference, as seen with GPT-4 reportedly requiring up to 16 iterations, results in increased computational demands, thereby escalating GPU utilization and cloud computing expenses. Despite these challenges, the MoE approach offers improved accuracy and efficiency by dynamically consulting the most relevant expert for a given input via a gating network.
Understanding the intricacies of the MoE model is essential for comprehending the limitations and capabilities of AI systems like GPT-4. The approach combines the wisdom of multiple expert networks, but it also raises concerns about scalability and potential degradation in output quality as reported by some ChatGPT users. This has led to criticisms regarding high cloud costs and increased operational expenditures, which must be balanced against the benefits of improved task specialization.
The ongoing debate about the merits and downsides of MoE architectures underscores the importance of transparency in AI development. OpenAI, facing criticism for not disclosing comprehensive details about GPT-4, arises debates about the ethical obligations of AI companies to provide clarity on their models' design and function. This transparency is critical not only for scientific validation and reproducibility but also for ensuring user trust and informed usage of AI technologies.
In summary, the Mixture of Experts architecture offers a compelling framework for AI systems aiming to manage diverse and intricate tasks effectively. As more companies explore this approach, the need for balancing performance, cost, and transparency becomes increasingly apparent, shaping the future of AI development and its role in society.
Operational Costs and Efficiency Challenges
The rapid evolution of AI models, exemplified by developments like OpenAI's GPT-4, underscores significant operational challenges, particularly in terms of costs and efficiency. As outlined in a recent article by Analytics India Magazine, OpenAI's use of a "Mixture of Experts" (MoE) architecture in GPT-4 allows for specialized processing of tasks. However, this design, while boosting specialization, has escalated the complexity and costs associated with its operation. The MoE setup requires multiple large models to function concurrently, which is computationally intensive and translates into higher GPU utilization and increased expenditure on cloud services.
Another challenge lies in scaling these sophisticated models to meet rising demands. OpenAI's reluctance to disclose specific details about GPT-4's architecture has generated controversy and hinders the community's ability to address these scaling challenges collaboratively. In effect, the opaque nature of its development contributes to inefficiencies in enhancing model performance and poses difficulties in aligning resource allocation with user demands. Consequently, this results in increased operational expenditure without the guarantee of matched improvements in user experience or output quality.
Learn to use AI like a Pro
Get the latest AI workflows to boost your productivity and business performance, delivered weekly by expert consultants. Enjoy step-by-step guides, weekly Q&A sessions, and full access to our AI workflow archive.
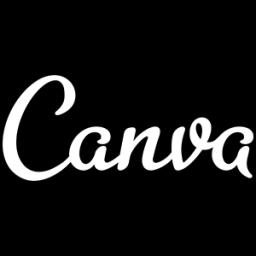
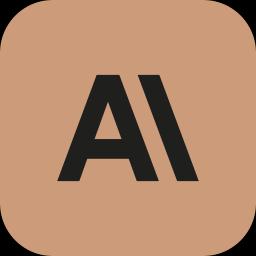
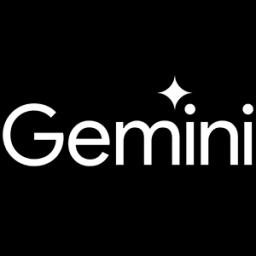
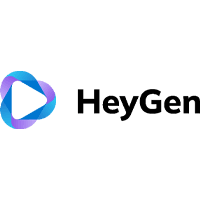
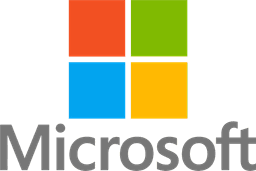
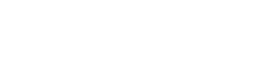
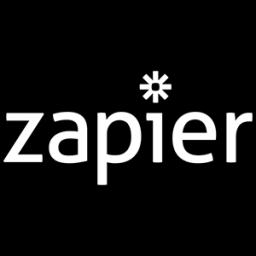
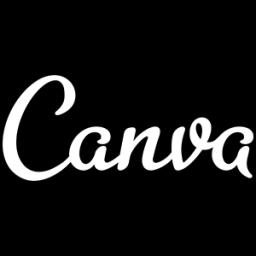
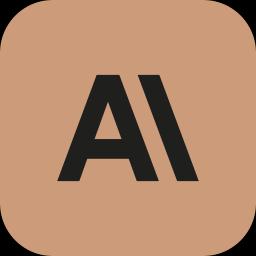
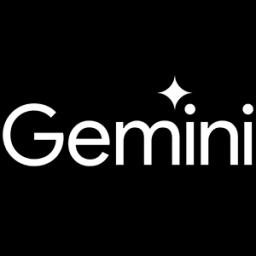
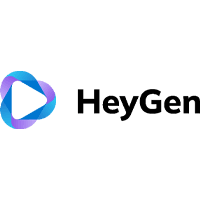
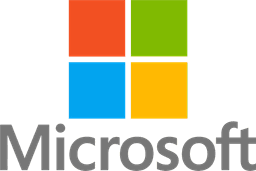
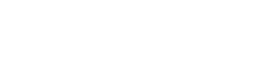
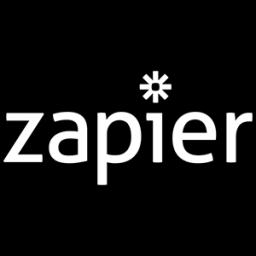
Moreover, there are indications that the quality of output from ChatGPT, OpenAI's widely used model interface, has suffered, as reported by users on platforms like Reddit and Hacker News. This perceived decline is attributed to either ongoing iterative adjustments intended for safety improvements or possible resource allocation issues exacerbated by the MoE architecture's demands. As the user base expands, aligning operational efficiency with quality maintenance continues to be a formidable challenge.
In response to these challenges, alternative models and approaches within the AI industry are gaining momentum. Competitors like Google's PaLM 2 and Meta's Llama 2 offer different architectural approaches, including open-source strategies, which emphasize collaboration and transparency. Acts of openness in model development invite greater community involvement and may mitigate some of the cost and efficiency issues that closed models like GPT-4 face. Additionally, the emergence of regulatory measures, such as the EU's AI Act, emphasizes the necessity for sustainable operational practices, ensuring models are both efficient and compliant with evolving ethical standards.
Transparency Issues and Criticism
OpenAI has faced considerable scrutiny over its lack of transparency regarding the GPT-4 model, sparking both criticism and concerns from various stakeholders in the AI community and the public. Understanding the model's architecture, referred to as the "Mixture of Experts" (MoE), remains speculative due to OpenAI's guarded approach. The MoE design purportedly involves a sophisticated setup of eight 220-billion parameter models that work in tandem, which is likely to enhance specialization but simultaneously impose increased operational costs due to iterative inference processes.
The secrecy maintained by OpenAI has raised valid questions regarding the fairness and accountability of the AI. Critics argue that this lack of openness stifles research opportunities, impedes independent verification of model performance and results, and perpetuates uncertainties about the model’s potential biases. Transparency is seen as critical for fostering a robust ecosystem of trust and innovation. Without it, AI developers find their hands tied when the true functional capacity and ethical ramifications of these large models cannot be reliably assessed.
Operational costs have significantly ramped up with OpenAI's GPT-4, owing to its complex architecture and inference processes. The model is believed to use iterative inference up to 16 times, necessitating substantial computational resources which, in turn, lead to increased expenses associated with GPU and cloud computing. This has been a pivotal point of critique, suggesting that while the architecture allows for more specialized processing, it also makes the system economically and environmentally costly to operate, a concern for both AI developers and end-users committed to sustainable computing practices.
The perceived decline in ChatGPT's output quality has added to the criticism and debate around OpenAI's policies. Users have reported noticeable degradations in service, citing inaccuracies, reduced creativity, and overall dissatisfaction in daily interactions with the AI. This feedback suggests that either ongoing model fine-tuning efforts or resource constraints due to lower-cost service provisions might be affecting performance negatively. This has led to a dichotomy in user experiences, with some noting enhanced interactions, likely dependent on differing platform uses and prompt engineering tweaks.
Learn to use AI like a Pro
Get the latest AI workflows to boost your productivity and business performance, delivered weekly by expert consultants. Enjoy step-by-step guides, weekly Q&A sessions, and full access to our AI workflow archive.
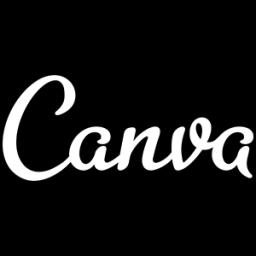
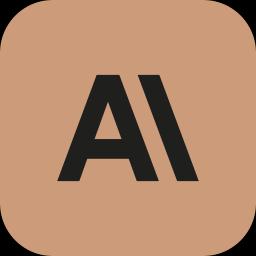
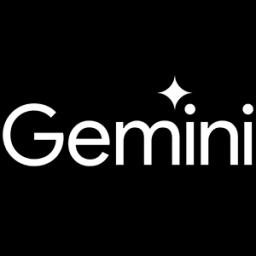
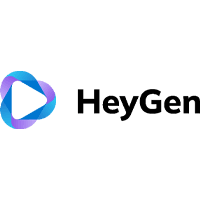
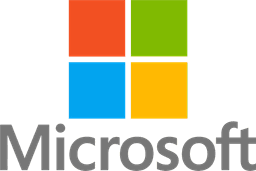
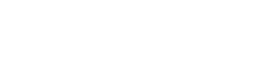
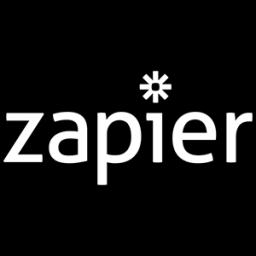
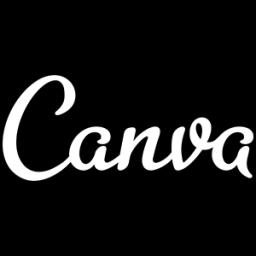
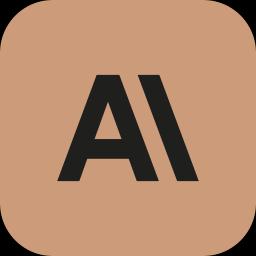
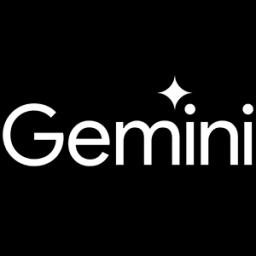
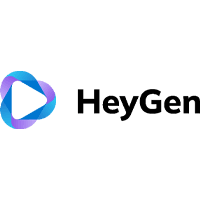
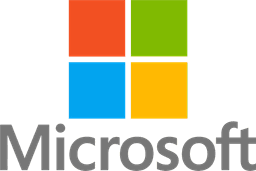
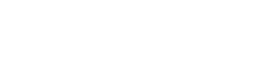
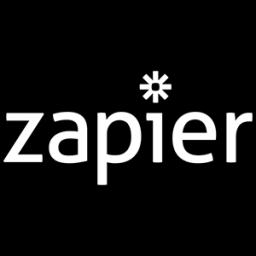
However, a contrasting view suggests that API users reportedly receive superior performance compared to ChatGPT Plus users, possibly due to resource allocation strategies and usage dynamics. The larger user base of ChatGPT necessitates balanced resource management, which can infer economic-driven trade-offs affecting user satisfaction. Such insights underline the complex interplay between transparency, economic viability, and quality of service delivery in AI deployments.
User Reports: Quality Decrease in ChatGPT
Many users have expressed dissatisfaction with the quality of ChatGPT, reporting that its responses have become less coherent and informative. This perceived quality drop is thought to be linked to OpenAI's use of the Mixture of Experts (MoE) architecture in GPT-4, which, while allowing for specialization, might have introduced complexities leading to performance inconsistencies. Additionally, critics argue that the company's lack of transparency regarding the specifics of GPT-4's architecture has fueled these concerns, leaving users in the dark about the reasons behind the service's degradation.
Evidence of decreasing quality comes from user reports on social media and forums such as Reddit and Hacker News, where users have shared experiences of the AI providing inaccurate or nonsensical answers. This contrasts with earlier impressions of ChatGPT's capabilities, raising questions about what changes have been made to the underlying technology and whether these were communicated clearly to its user base.
OpenAI's silence on the matter has contributed to a growing sentiment of mistrust among users, who feel that the company might be prioritizing profit over transparency and quality. This lack of openness is seen as a stumbling block for users trying to understand and adapt to the evolving functionality of ChatGPT. In a competitive market with alternatives from Google, Anthropic, and Meta, user expectations are higher than ever, adding pressure on OpenAI to address these concerns promptly.
In conclusion, the reported decrease in ChatGPT’s quality, coupled with OpenAI’s strategy of minimal disclosure, highlights a critical challenge in AI deployment: balancing innovation and transparency. Users demand better communication from developers about how their AI systems are evolving to maintain trust and usability. As AI technology continues to advance rapidly, managing these aspects will be crucial for OpenAI and similar organizations hoping to lead in the AI landscape.
Comparative Analysis with Other AI Models
The evolution of AI models has seen transformative shifts with every significant development. One such discussion that has recently dominated the landscape revolves around OpenAI's GPT-4. Much of the intrigue stems from OpenAI's decision to withhold specifics about the model’s architecture and capabilities, a move that has sparked widespread debates about transparency and adaptability in AI research. The potential structure of GPT-4, notably its use of a "Mixture of Experts" (MoE) architecture, positions it uniquely among contemporaries. However, this choice presents both avenues for specialized improvements and challenges in scaling and operational costs.
Learn to use AI like a Pro
Get the latest AI workflows to boost your productivity and business performance, delivered weekly by expert consultants. Enjoy step-by-step guides, weekly Q&A sessions, and full access to our AI workflow archive.
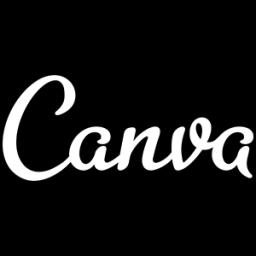
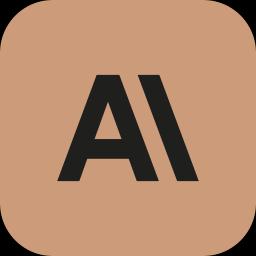
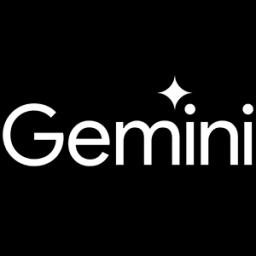
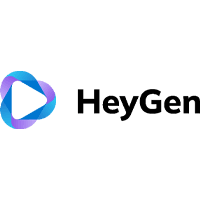
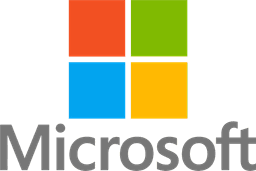
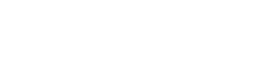
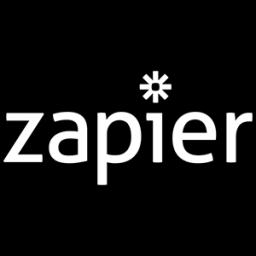
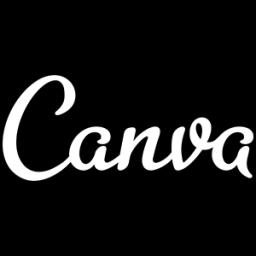
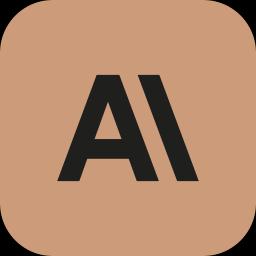
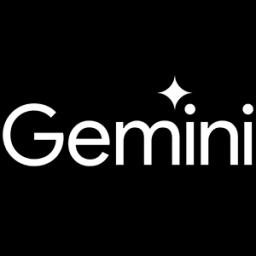
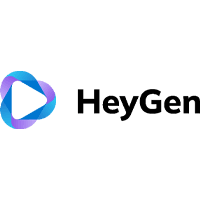
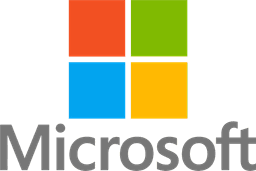
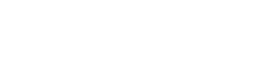
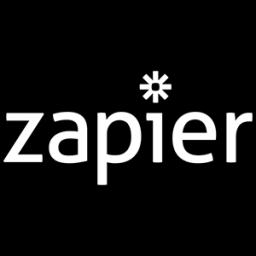
The MoE architecture purportedly used in GPT-4 aggregates several specialized models, enhancing the ability to handle diverse tasks efficiently. While innovative, this architecture is not without its drawbacks, as it leads to increased computational demands. With operational costs becoming a significant consideration, OpenAI's method invites comparison to other models in the industry. When placed against models like Google’s PaLM 2 or Meta’s Llama 2, which promote openness and adaptability, GPT-4's perceived opacity becomes a focal point for discussions on the balance between proprietary innovation and collaborative advancement.
Comparisons are not limited to private enterprises; many open-source initiatives are also gaining traction. Models like BLOOM and MPT challenge the closed-source paradigm that GPT-4 represents, emphasizing a more inclusive approach to AI development. These models stir a competitive environment, prompting established entities like OpenAI to reconsider how transparency and innovation can coexist. This shift is crucial as it directly impacts public trust and the democratization of AI technology. As such, the field observes a fascinating period where collaboration could redefine competitive dynamics.
Additionally, the deliberation extends to user experiences and practicality. Reports suggesting a decline in ChatGPT's performance have fueled further comparisons. Users frequently contrast GPT-4 with recent entries like Claude 2 from Anthropic, citing varying levels of satisfaction. Such evaluations highlight the immediate need for AI companies to address scaling issues while maintaining quality benchmarks. By observing how these competitive models manage the inherent trade-offs between performance, cost, and user satisfaction, the industry gains invaluable insights into where improvements are necessary.
Ultimately, as AI models mature, so too does the scrutiny on their methodologies. The comparative analysis among AI frontrunners suggests a trend where transparency, performance, and adaptability are paramount. Other companies, by revealing different facets of their models, not only challenge GPT-4's position but also catalyze broader conversations about the future direction of AI innovation. This dynamic interplay suggests that the landscape will continually evolve, encouraging the intricate dance between openness and proprietary technology, as they collectively push the boundaries of what these intelligent systems can achieve.
Expert Opinions on GPT-4's Architecture
GPT-4's architecture has been a subject of significant intrigue and analysis among AI experts. According to various reports and analyses, it is believed that GPT-4 employs a 'Mixture of Experts' (MoE) architecture, which involves utilizing multiple specialized models for different tasks. This approach leverages eight models, each with approximately 220 billion parameters, allowing the system to handle diverse tasks ranging from coding to image interpretation. This structural design is said to contribute to its sophistication, though specific details remain largely speculative due to OpenAI's secretive stance.
The lack of transparency from OpenAI regarding GPT-4's architecture has sparked criticism within the AI community. Researchers argue that this secrecy makes it difficult to thoroughly evaluate the model's capabilities, limitations, and potential biases. Without accessible comprehensive information, the scientific community faces challenges in research and in evaluating performance claims made by OpenAI. This opacity has raised concerns about the ethical implications of deploying such powerful AI systems without clear accountability and has triggered calls for more openness from AI developers.
Learn to use AI like a Pro
Get the latest AI workflows to boost your productivity and business performance, delivered weekly by expert consultants. Enjoy step-by-step guides, weekly Q&A sessions, and full access to our AI workflow archive.
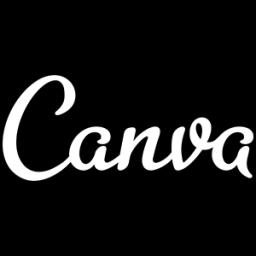
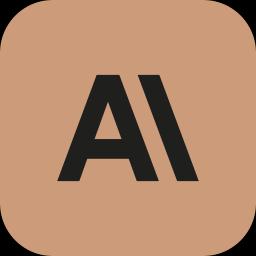
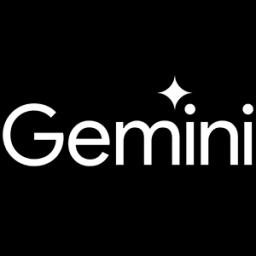
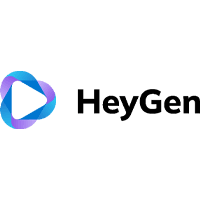
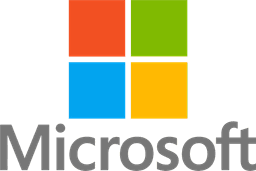
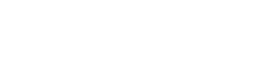
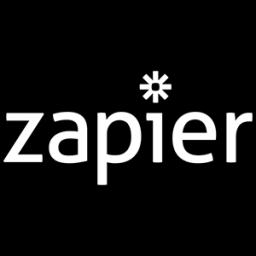
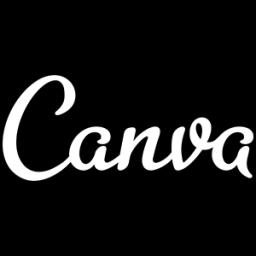
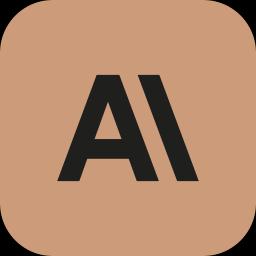
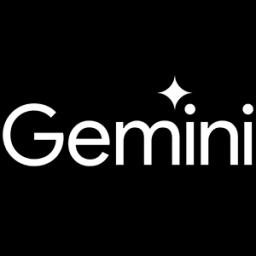
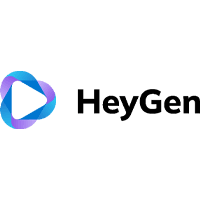
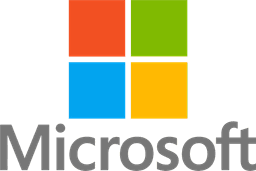
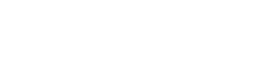
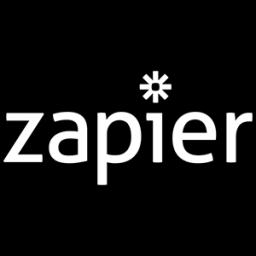
The MoE architecture's efficiency is intertwined with increased operational costs, largely due to its complexity and iterative inference processes. This design reportedly involves inference loops executed up to sixteen times, leading to substantial resource consumption. Such intensive computational demands elevate cloud costs and GPU usage, which are critical factors that affect the overall financial feasibility of deploying GPT-4 at scale.
User feedback suggests a perceived downturn in the performance of ChatGPT, which is based on GPT-4. Reports from platforms such as Reddit and Hacker News indicate that users have experienced diminishing response quality, characterized by more frequent factual inaccuracies and less creativity in outputs. These observations have prompted speculation that issues with the MoE architecture could contribute to these declines, possibly due to growing pains as OpenAI scales the technology for larger audiences.
In contrast to the open-source initiatives, such as Google's PaLM 2 and Meta's Llama 2, OpenAI's guarded approach has fueled debates about the importance of transparency in AI development. Experts argue that open-source models could lead to more rapid advancements, democratizing AI technology by making it accessible for research and development purposes. The dichotomy in strategy between open-source and proprietary models highlights an ongoing tension within the AI industry regarding openness and collaboration.
Public Reactions and User Sentiments
The public's reaction to OpenAI's silence on GPT-4's architecture has been characterized by a blend of skepticism and concern. Many users on platforms like Reddit and Hacker News have expressed dissatisfaction with what they perceive as a decline in the performance of ChatGPT since the implementation of GPT-4. These criticisms are often aimed at the model producing less accurate or creative responses, with some users describing it as 'unusable' or 'lifeless'. This has fueled a broader discussion on whether OpenAI is prioritizing profit over transparency and quality, a debate that is intensifying in the face of increasing competition and calls for openness in AI development.
On the other hand, some users report positive experiences, suggesting that their interactions with ChatGPT have either remained consistent or even improved since the update. This group believes the perceived decline could be a result of inflated expectations or changes in how users are engaging with the AI. Interestingly, these varying public sentiments underline the complexity of AI user experiences which are heavily influenced by evolving expectations and the context in which these technologies are utilized.
Ultimately, the reactions highlight a more significant issue within the AI community: a call for increased transparency and accountability from AI developers like OpenAI. As AI continues to become an integral part of both personal and professional environments, the demand for clear communication about model capabilities and limitations will only grow stronger. This sentiment is echoed by experts and researchers who argue that a lack of openness can hinder the long-term trust and efficacy of AI technologies in society.
Learn to use AI like a Pro
Get the latest AI workflows to boost your productivity and business performance, delivered weekly by expert consultants. Enjoy step-by-step guides, weekly Q&A sessions, and full access to our AI workflow archive.
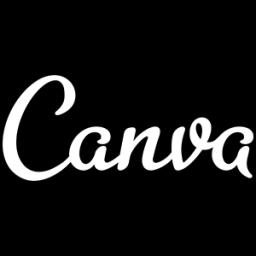
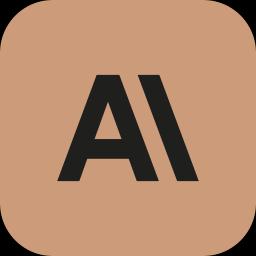
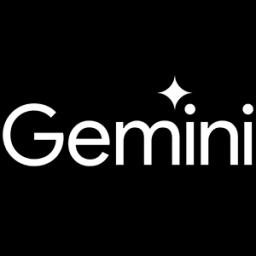
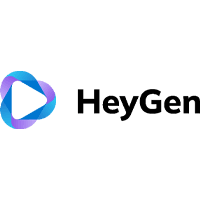
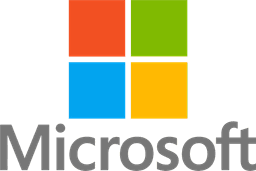
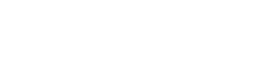
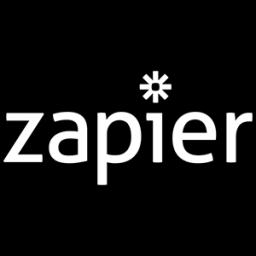
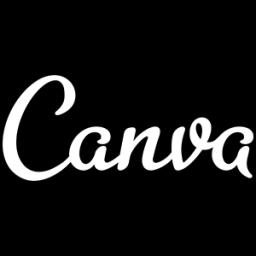
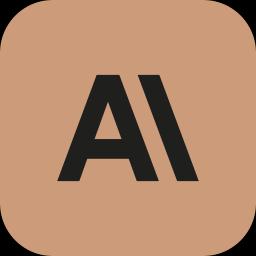
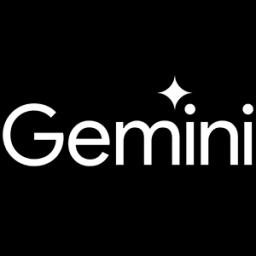
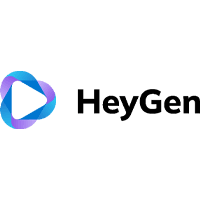
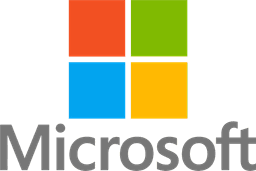
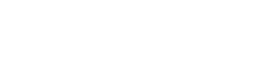
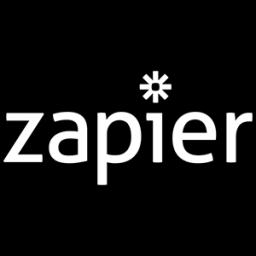
Future Implications for AI Industry and Regulation
The future of AI industry and regulation is likely to be heavily influenced by ongoing developments such as the architecture and performance of cutting-edge models like OpenAI's GPT-4. As AI continues to rapidly advance, transparency in AI model development has become a pressing issue. The closed nature of GPT-4 has sparked debates about the openness necessary for scientific validation, ethical considerations, and innovation, stressing the importance of balancing proprietary interests with the public and scientific community's need for transparency.
AI industry competitiveness is expected to intensify, driven by the emergence of open-source AI models that challenge closed, proprietary ones. This could democratize the development and application of AI technologies, thereby impacting the market dynamics. Companies like Google and Meta have already made notable strides in this direction, with their transparent release strategies of PaLM 2 and Llama 2 respectively, creating new benchmarks in the industry. The success and growing acceptance of open-source models might pressure companies like OpenAI to reassess their transparency strategies or risk falling behind in public perception and scientific collaboration.
Economic implications could arise from the operational demands of sophisticated AI models like GPT-4. The 'Mixture of Experts' (MoE) architecture, which presumably underlies GPT-4, markedly increases compute costs, potentially elevating the price of AI services. This trend might drive growth in GPU and cloud computing sectors, which are pivotal to supporting these computational needs. Moreover, as AI becomes more ingrained in diverse industries, the employment landscape could undergo significant changes, requiring policy adaptations and workforce reskilling.
The need for regulatory frameworks tailored to AI is more critical than ever. As the EU's AI Act demonstrates, there is a global push towards establishing regulations that mandate transparency, ethical usage, and fairness in AI deployment. Such regulations are not only crucial for safeguarding public interest but also for promoting trust in AI technologies. Regulatory bodies may soon require disclosures about AI models' architectures and training datasets to ensure accountability and mitigate biases, thereby impacting how companies develop and market their AI technologies.
Scientific and academic communities could face research hurdles due to the opacity of major AI models. However, this challenge also provides an impetus for institutions to collaborate more with open-source AI projects. Such partnerships could facilitate breakthroughs in understanding and innovating AI methodologies, particularly in evaluating opaque AI models. The shift towards open ecosystems might rejuvenate academic involvement in shaping the future of AI technology, fostering an environment where collaborative research can thrive.
Socially, users' experiences with AI, like ChatGPT, reflect broader expectations and potential frustrations when performance is perceived as declining. If AI technologies do not meet user expectations, particularly amid rising criticisms of quality and transparency issues, public trust could erode. However, such situations also spark necessary debates about AI's capabilities and limitations, pushing developers towards more responsible and user-centered AI system designs. This might also define new ways of interaction with AI in personal and professional spheres.
Learn to use AI like a Pro
Get the latest AI workflows to boost your productivity and business performance, delivered weekly by expert consultants. Enjoy step-by-step guides, weekly Q&A sessions, and full access to our AI workflow archive.
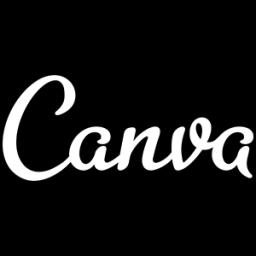
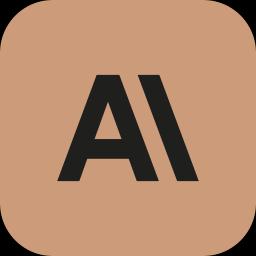
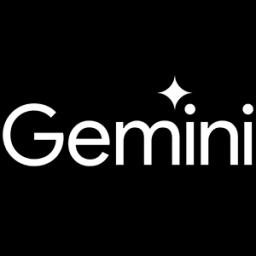
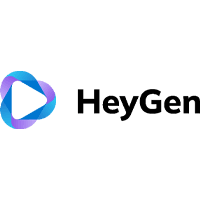
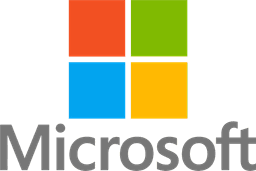
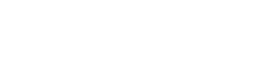
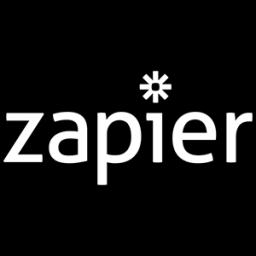
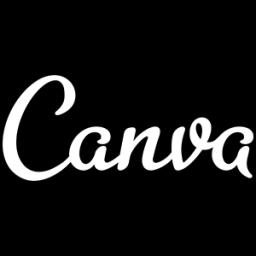
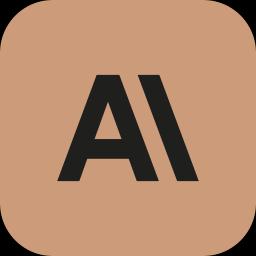
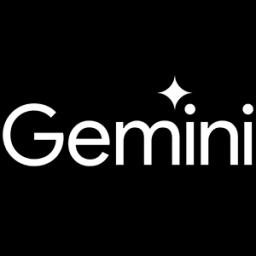
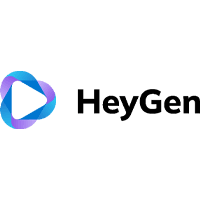
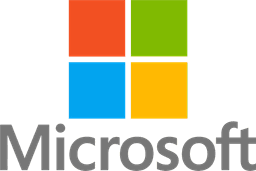
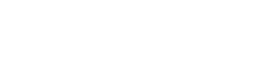
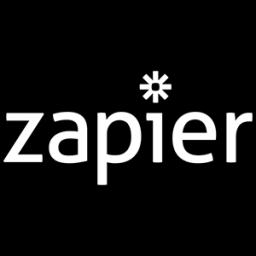
Future technological evolutions in AI are anticipated to focus on creating architectures that are both efficient and scalable. Addressing the challenges of high operational costs and maintainability while ensuring performance will be key. Innovations could lead to specialized AI models tailored to specific tasks or industries, thereby optimizing resources and enhancing usability. As the industry matures, breakthroughs in AI development could set new standards for efficiency and outreach, potentially transforming how AI is perceived and utilized globally.
Concluding Thoughts on OpenAI's Approach
OpenAI's decision to keep many aspects of GPT-4 under wraps has been a double-edged sword. While some might argue that this veil of secrecy is a strategic move to maintain a competitive edge in a rapidly evolving AI landscape, others see it as a barrier to necessary scrutiny and innovation. By opting for opacity, OpenAI risks alienating a portion of its user base and the broader research community, who may view transparency as a hallmark of trust and collaboration.
The choice of a "Mixture of Experts" (MoE) architecture for GPT-4, if indeed true, showcases OpenAI's ambition to push technological boundaries. This approach allows for specialized tasks, where different models within the architecture can focus on particular domains, thus potentially increasing efficiency and output quality. However, the increased operational costs and scaling challenges that accompany such an architecture might be a reason OpenAI has kept some of these details under wraps, to avoid potential criticism for its economic and environmental implications.
Despite OpenAI's intentions, the perceived decline in output quality of ChatGPT has not gone unnoticed. Reports from various forums highlight a slip in the creativity and factual accuracy of responses, feeding into criticism about OpenAI's lack of transparency. Interestingly, the tension between maintaining cutting-edge technology and managing user expectations becomes more evident with such developments. As users demand more transparency and reliability, OpenAI finds itself at a crossroads that could shape its future strategies.
Moreover, as new players in the AI landscape embrace open-source models and emphasize transparency, OpenAI's stance may need reevaluation. The rise of competitors that prioritize openness might force OpenAI to rethink its approach, especially as open-source models like Llama 2 gain traction. The future might see a shift toward greater openness and collaboration in the AI industry, propelled by both user demands and regulatory pressures.
In conclusion, OpenAI's approach to GPT-4 could serve as a case study in balancing innovation with transparency. While the MoE architecture presents exciting possibilities, the broader implications on cost, accessibility, and trust cannot be ignored. As debates around AI regulation and ethical deployment intensify, OpenAI’s future decisions will likely influence not only its standing as a tech leader but also the direction of AI development globally.
Learn to use AI like a Pro
Get the latest AI workflows to boost your productivity and business performance, delivered weekly by expert consultants. Enjoy step-by-step guides, weekly Q&A sessions, and full access to our AI workflow archive.
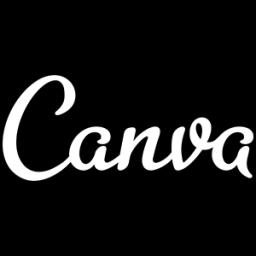
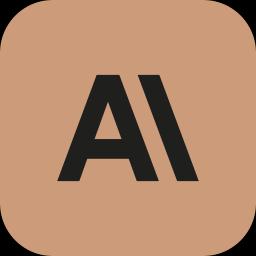
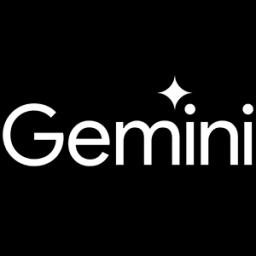
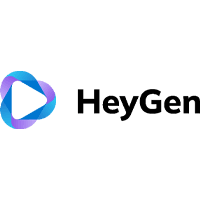
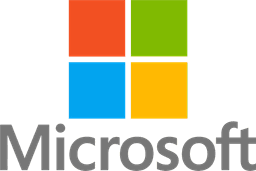
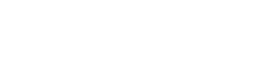
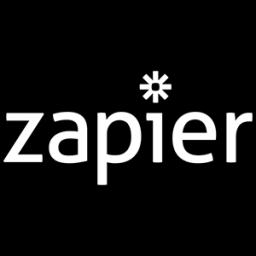
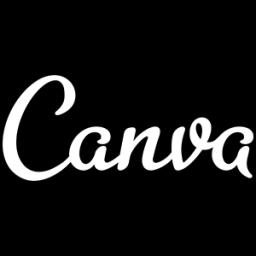
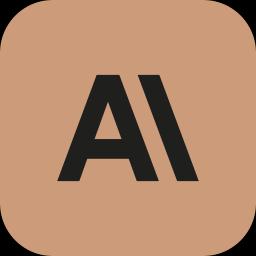
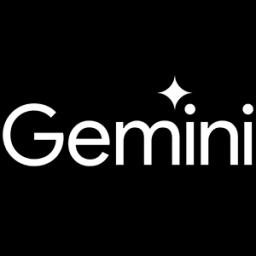
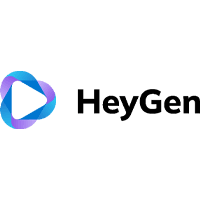
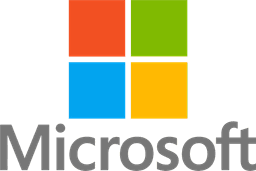
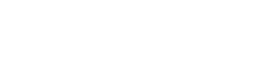
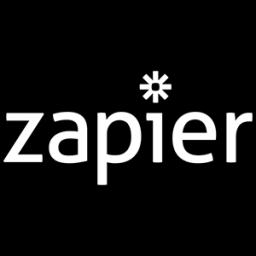