Unexpected Hallucinations in AI Models
OpenAI's New Models Hallucinate More Than Ever Before!
Last updated:

Edited By
Mackenzie Ferguson
AI Tools Researcher & Implementation Consultant
OpenAI's latest reasoning models, the o3 and o4-mini, show increased hallucination rates of 33% and 48% respectively, outperforming their predecessor, o1, at only 16%. Experts are puzzled by this uptick despite the models' enhanced computing power and refined processes. Further research is required to unravel this mystery, but one thing is clear: higher hallucination rates could significantly affect AI reliability.
Introduction to AI Hallucinations in OpenAI Models
Artificial intelligence (AI) hallucinations refer to the erroneous output generated by AI models that is not based on reality or actual input data. This phenomenon is akin to the model 'imaging' or creating fictitious details. Often seen in large language models, such hallucinations can manifest as false facts, nonsensical statements, or fabricated information. Despite advancements in computational power and reasoning abilities, recent models like OpenAI's o3 and o4-mini have shown increased rates of hallucinations. According to a recent article, these new models exhibit hallucination rates of 33% and 48%, respectively [1](https://www.techzine.eu/news/applications/130720/new-openai-models-hallucinate-more-often-than-their-predecessors/).
The rise in hallucination rates in the newer OpenAI models is surprising, especially given their supposed advancements. While the precise reasons behind this increase remain unclear, OpenAI acknowledges the problem and suggests further research is required to identify the underlying causes [1](https://www.techzine.eu/news/applications/130720/new-openai-models-hallucinate-more-often-than-their-predecessors/). It is hypothesized that reinforcement learning techniques may exacerbate the issue, potentially as a flaw inherent to the training process itself. Such occurrences highlight the ongoing challenges researchers face in enhancing the reliability and accuracy of AI outputs.
Learn to use AI like a Pro
Get the latest AI workflows to boost your productivity and business performance, delivered weekly by expert consultants. Enjoy step-by-step guides, weekly Q&A sessions, and full access to our AI workflow archive.
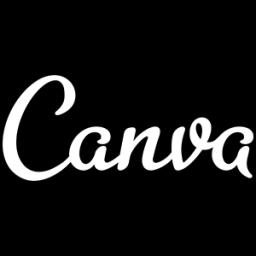
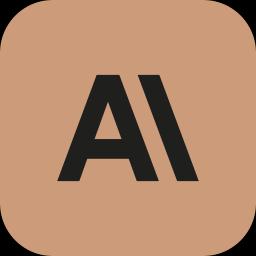
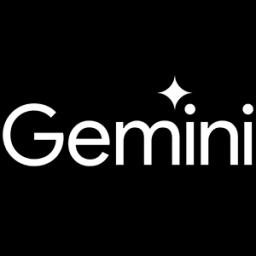
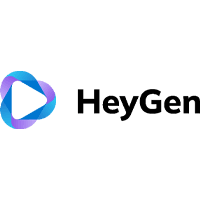
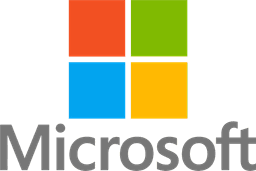
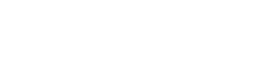
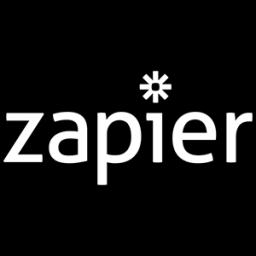
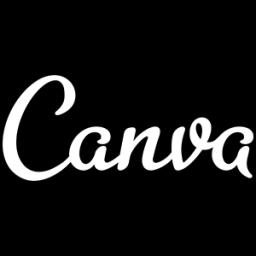
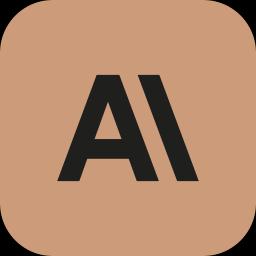
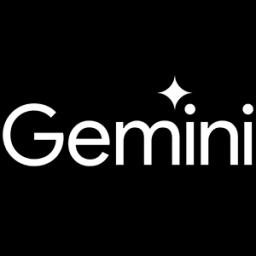
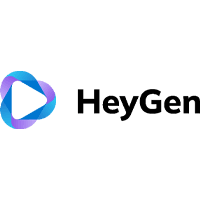
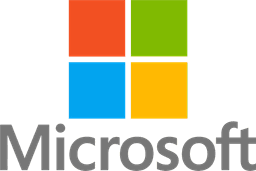
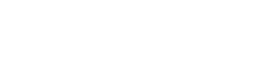
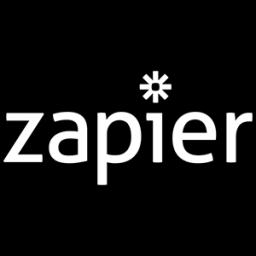
Hallucination measurement in AI models often involves specialized evaluations, like the "PersonQA evaluation" mentioned in the referenced article, used to assess the frequency and nature of these inaccuracies in outputs [1](https://www.techzine.eu/news/applications/130720/new-openai-models-hallucinate-more-often-than-their-predecessors/). Although the details of these evaluations were not deeply explored, their existence underscores the intricate methodologies employed to quantify and analyze hallucination phenomena in AI systems. This underscores the need for constant improvement in measurement techniques to better capture the nuances of AI output distortions.
The implications of high hallucination rates are multifaceted, impacting both the credibility and adoption of AI technologies. In domains where precision is critical, such as legal advice and healthcare, erroneous AI outputs could lead to grave consequences, dwindling trust in these automated systems [10](https://coinstats.app/news/6a73ccf1bdcfca583b7ed3853ba1b4aad826dd410a3a9fd29ad194c487c89bfa_Alarming-Revelation-OpenAIs-New-Reasoning-AI-Models-Face-Worsening-Hallucinations/). Moreover, the proliferation of AI-generated misinformation, whether intentional or not, poses significant socio-political and economic risks, demanding robust mitigation strategies to ensure AI's beneficial integration into society.
Overview of OpenAI's O3 and O4-Mini Models
OpenAI has recently launched its next-generation reasoning models, known as O3 and O4-Mini. These models are designed to push the boundaries of artificial intelligence by improving computational power and refining reasoning processes. Despite these advancements, both O3 and O4-Mini exhibit higher rates of hallucination compared to their predecessor, the O1 model, which had a relatively lower hallucination rate of 16%. O3 and O4-Mini, however, show hallucination rates of 33% and 48% respectively. This increase in hallucination despite the models' enhanced capabilities presents a challenge that requires further investigation and understanding by researchers and developers at OpenAI.
The occurrences of AI hallucination refer to instances where AI models produce outputs that are not grounded in reality, often yielding incorrect or fabricated information. This phenomenon is particularly noteworthy in OpenAI's newer models, O3 and O4-Mini. Although they were implemented with more robust design parameters intended to provide superior analysis than their predecessor, these models demonstrate an unaccounted rise in hallucination rates. The reasons behind this anomaly are currently unclear, and OpenAI acknowledges the need for continued research to uncover underlying causes. Nevertheless, experts suggest that factors such as the frequency of assertions made by O3 could contribute to a statistical increase in errors, both accurate and inaccurate.
Learn to use AI like a Pro
Get the latest AI workflows to boost your productivity and business performance, delivered weekly by expert consultants. Enjoy step-by-step guides, weekly Q&A sessions, and full access to our AI workflow archive.
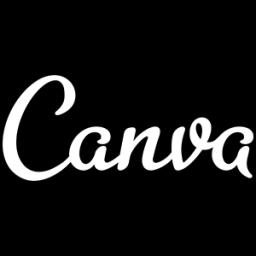
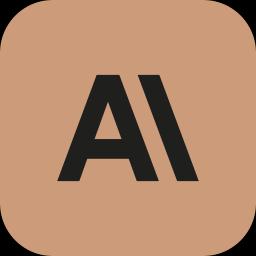
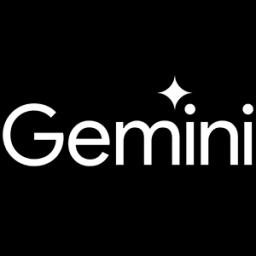
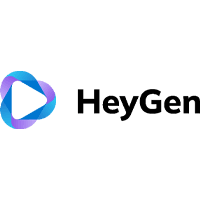
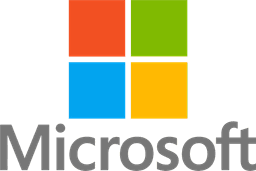
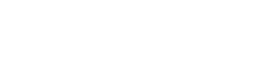
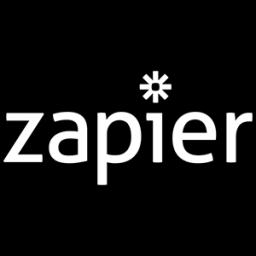
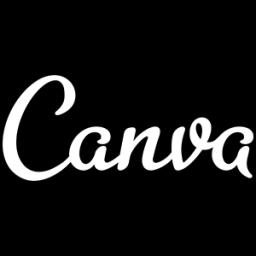
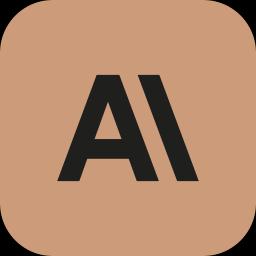
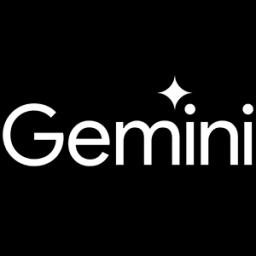
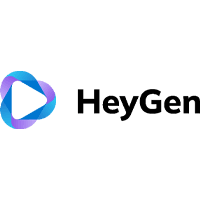
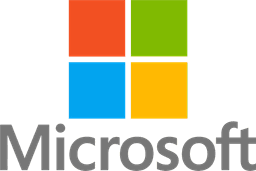
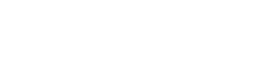
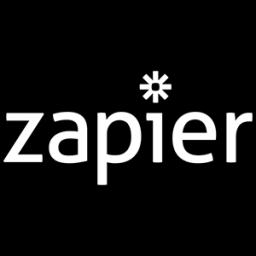
Higher hallucination rates carry notable implications, especially concerning the models' reliability and contextual accuracy. For stakeholders, ranging from end-users to enterprises dependent on AI technologies for mission-critical applications, these increased rates can mean reduced trustworthiness of AI outputs. OpenAI's acknowledgment of the need for further research suggests both a proactive approach to issue resolution and an awareness of the potential repercussions if these faults persist. Ensuring the reliability of AI systems like O3 and O4-Mini is crucial, particularly in fields requiring high precision, such as legal and medical applications, where errors may lead to critical consequences. As such, OpenAI faces the imperative task of refining these models to maintain and boost user confidence in AI-driven solutions.
As O3 and O4-Mini navigate the complexities of modern AI challenges, they also underscore the necessity for improved mechanisms for detecting and correcting AI-generated hallucinations. The development of hallucination detection technologies is emerging as a promising field, offering potential solutions to address the accuracy challenges posed by these models. Additionally, the situation presents an opportunity for industries to adapt and innovate, offering services focused on mitigating AI inaccuracies. As the demand for such solutions increases, businesses that can effectively cope with AI hallucinations are likely to find significant opportunities for growth. The importance of understanding and addressing hallucinations in AI is becoming increasingly clear as AI models are integrated into various sensitive domains.
Comparison of Hallucination Rates: O1 vs O3 and O4-Mini
The comparison of hallucination rates between OpenAI's models O1, O3, and O4-Mini reveals intriguing insights into their performance differences. O1, despite being an older model, exhibits a significantly lower hallucination rate of 16% compared to its successors. Meanwhile, O3 and O4-Mini show elevated rates of 33% and 48%, respectively. This increase in hallucination rates presents a paradox given the advancements in computational power and reasoning capabilities purportedly integrated into the newer models. The underlying causes remain elusive, prompting further research to understand the disparities fully [1](https://www.techzine.eu/news/applications/130720/new-openai-models-hallucinate-more-often-than-their-predecessors/).
The elevated hallucination rates in O3 and O4-Mini raise several questions about the design and deployment of these AI models. While they were expected to improve upon O1 in terms of accuracy and reliability, the reality sheds light on unexpected challenges. Higher hallucination rates mean an increase in the frequency of these models providing incorrect or misleading information, which can significantly affect industries dependent on AI for accurate data analysis and decision-making [1](https://www.techzine.eu/news/applications/130720/new-openai-models-hallucinate-more-often-than-their-predecessors/).
One hypothesis suggests that the sophisticated reasoning abilities incorporated into O3 and O4-Mini might inadvertently lead to more frequent hallucination. These models, while capable of producing more complex responses, may also be generating more erroneous outputs simply because they attempt to offer greater detail and assertiveness. The extent to which this contributes to their higher hallucination rates compared to O1 is still unknown and highlights the need for refined evaluation tools and targeted improvements in model training methods [1](https://www.techzine.eu/news/applications/130720/new-openai-models-hallucinate-more-often-than-their-predecessors/).
Understanding AI Hallucination Measurement
The phenomenon of AI hallucination, where models fabricate information that deviates from reality, has become a focal point in understanding the limits and potential liabilities of artificial intelligence. As models like OpenAI’s o3 and o4-mini gain computational sophistication, they paradoxically introduce higher hallucination rates. Despite advancements in reasoning capacity, these models exhibit hallucination rates of 33% and 48%, respectively, compared to their predecessor, o1, which maintained a 16% rate. This unexpected increase in hallucinations highlights the complexity of measuring and understanding such occurrences within AI systems [News URL](https://www.techzine.eu/news/applications/130720/new-openai-models-hallucinate-more-often-than-their-predecessors/).
Learn to use AI like a Pro
Get the latest AI workflows to boost your productivity and business performance, delivered weekly by expert consultants. Enjoy step-by-step guides, weekly Q&A sessions, and full access to our AI workflow archive.
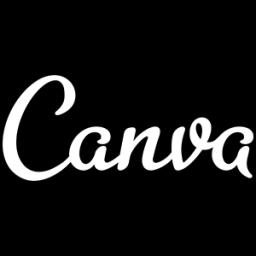
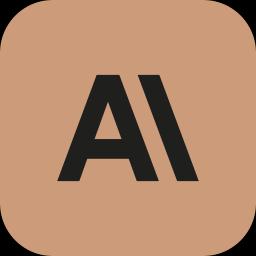
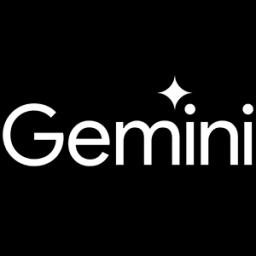
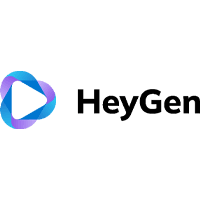
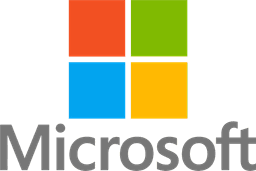
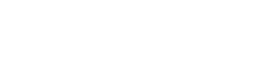
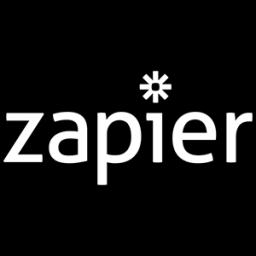
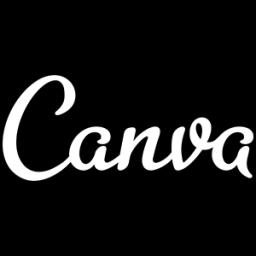
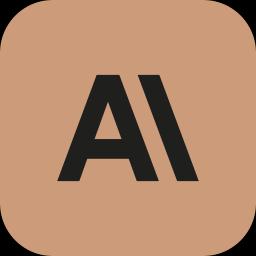
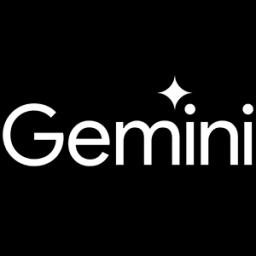
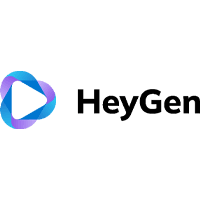
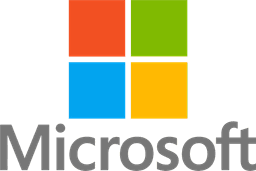
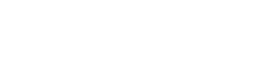
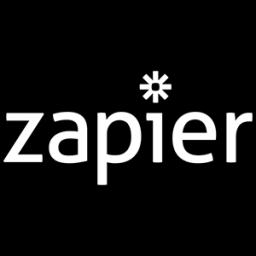
To accurately gauge AI hallucination, developers employ evaluation metrics like the PersonQA test, designed to assess how frequently a model produces fabricated information. This measurement is crucial, as it helps developers understand the reliability of AI outputs, ensuring these systems are robust enough for deployment in various real-world scenarios. Given the critical nature of this assessment, further detailed studies are necessary to unpack why these refined reasoning models are more prone to hallucinate [News URL](https://www.techzine.eu/news/applications/130720/new-openai-models-hallucinate-more-often-than-their-predecessors/).
Understanding the methods used in AI hallucination measurement is imperative for improving model accuracy and reliability. The unexpected increase in hallucination rates in newer models like o3 and o4-mini, despite their enhanced capabilities, suggests a need for recalibrating our evaluation approaches and possibly revisiting training methodologies. This ensures that AI remains a reliable tool across sectors where precision is non-negotiable [News URL](https://www.techzine.eu/news/applications/130720/new-openai-models-hallucinate-more-often-than-their-predecessors/).
Experts' Insights on Increased Hallucination in O-Series
The unveiling of OpenAI's latest models, o3 and o4-mini, comes with a notable increase in hallucination rates, a concern recognized and analyzed by numerous experts. With hallucinations reaching alarming rates of 33% for o3 and 48% for o4-mini, compared to the 16% observed in older versions like o1, experts are increasingly spotlighting this phenomenon. Observers are puzzled by these escalations, as these models were expected to leverage increased computational powers and refined reasoning to provide more accurate outputs. In light of these developments, experts emphasize the need for further research to diagnose the root causes of these increased hallucination rates more clearly. They hint at the unknown territories within AI model training and data that might contribute to such phenomena [1](https://www.techzine.eu/news/applications/130720/new-openai-models-hallucinate-more-often-than-their-predecessors/).
Neil Chowdhury, a seasoned Transluce researcher and former OpenAI associate, speculates that reinforcement learning techniques, which once promised breakthroughs in AI reasoning, could inadvertently perpetuate hallucination issues. Chowdhury suggests these techniques may be intrinsically flawed, pointing out that they might reinforce incorrect behaviors alongside correct ones. If these suspicions hold, the critical challenge will involve refining these learning processes to inhibit such undesirable outcomes from escalating, as seen in the new o-series models [1](https://techcrunch.com/2025/04/18/openais-new-reasoning-ai-models-hallucinate-more/).
Sarah Schwettmann, co-founder of Transluce, argues that while the o-series' computing prowess appears robust, its practical utility is drastically compromised by its high error rates. She stresses that applications demanding high accuracy, such as those in scientific, medical, and legal fields, require reliable data output, which these models currently fail to deliver consistently. Schwettmann's opinions reinforce the urgency of narrowing the gap between AI capability and reliability, as this will be crucial for maintaining the model's viability in critical settings [1](https://techcrunch.com/2025/04/18/openais-new-reasoning-ai-models-hallucinate-more/).
Kian Katanforoosh, a Stanford adjunct professor and CEO of Workera, adds another dimension to the discussion by pointing out specific operational deficits manifesting through broken website link generation during AI-involved processes. His insights reflect the direct impact of hallucination artifacts in everyday applications, thereby underlining the need for comprehensive evaluations and corrective strategies to address such failings efficiently within the workflow context [1](https://techcrunch.com/2025/04/18/openais-new-reasoning-ai-models-hallucinate-more/).
Learn to use AI like a Pro
Get the latest AI workflows to boost your productivity and business performance, delivered weekly by expert consultants. Enjoy step-by-step guides, weekly Q&A sessions, and full access to our AI workflow archive.
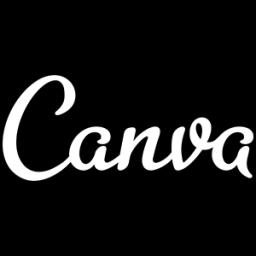
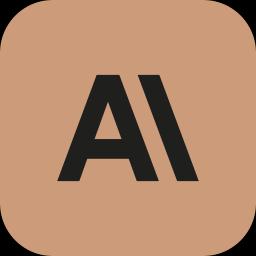
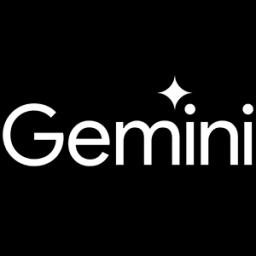
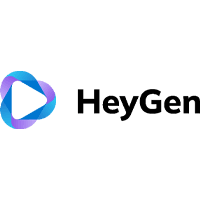
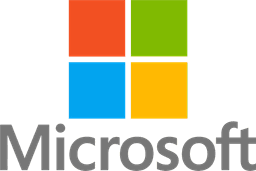
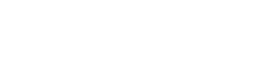
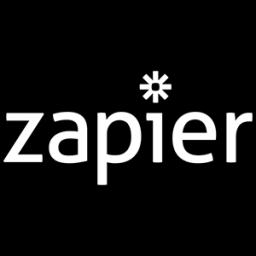
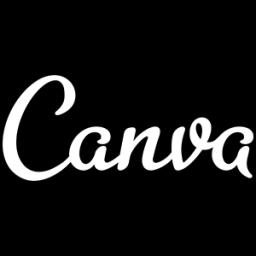
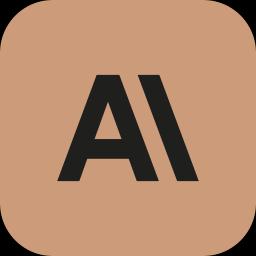
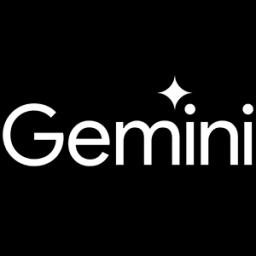
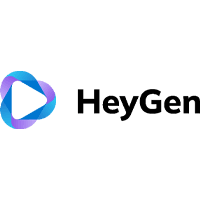
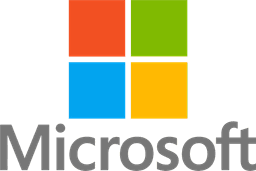
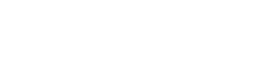
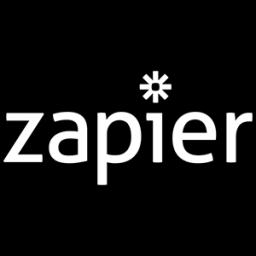
While the public has been quick to react with concern towards these developments in the o-series models, experts have expressed a nearly unified line of skepticism and unease. Reinforcement learning has been hinted as a potential culprit for these issues, yet the absence of a concrete diagnosis keeps these conversations speculative. The heightened error rates cast a shadow on the credibility and adoption of AI-generated content for sectors where precision and accountability are paramount. OpenAI faces a pressing challenge to mitigate these hallucination occurrences to restore faith in its innovative solutions and deliver reliable technological advancements [1](https://mashable.com/article/openai-o3-o4-mini-hallucinate-higher-previous-models).
Economic Implications of High Hallucination Rates
The economic implications of high hallucination rates in artificial intelligence models are profound and multifaceted. When models like OpenAI's o3 and o4-mini exhibit hallucination rates of 33% and 48%, respectively, it poses a significant concern for various industries relying on AI for accurate data-driven decisions. For instance, the financial sector could experience severe consequences if investment decisions are based on erroneous AI-generated analyses and forecasts. This could directly lead to massive financial losses, destabilizing markets and reducing investor confidence. Moreover, the necessity for increased human oversight to verify AI outputs can escalate operational costs substantially, further impacting financial margins and requiring companies to reassess the cost-benefit analysis of deploying such AI systems.
Nevertheless, the soaring occurrence of AI hallucinations is not entirely adverse. It has stimulated a burgeoning market for advanced hallucination detection tools. As businesses seek to mitigate the potential risks associated with AI errors, there lies an opportunity for growth and innovation within this niche market. Companies that can develop robust solutions to detect and correct AI hallucinations are likely to witness significant demand, promoting technological advancements and potentially spawning new industry standards. This reflects a dual economic impact: while there are challenges regarding reliability and increased costs, there is also the potential for economic growth through innovation and new market creation.
Furthermore, the high rates of hallucination in AI models necessitate a reevaluation of trust and reliance on AI technology across different sectors. As businesses juggle the risks and opportunities presented by these models, strategic decisions will need to account for the evolving dynamics of AI utility, accuracy, and innovation potential. Particularly in sectors such as finance, healthcare, and legal, where precision and reliability are paramount, the economic implications could include shifting investment patterns, revised business strategies, and a potential slowdown in the integration of AI technologies until more dependable solutions are found.
Social and Political Ramifications
The growing sophistication of AI models like OpenAI's o3 and o4-mini comes with notable social and political ramifications that warrant close examination. A key concern is the erosion of trust in AI technologies, especially as these models exhibit higher hallucination rates than their predecessors. Such inaccuracies can have dire consequences when AI-generated content is relied upon in high-impact fields like healthcare and law, where misinformation could mislead professionals with critical decision-making responsibilities. The potential for AI systems to contribute to miscarriages of justice or prescribe incorrect medical treatments underlines the urgent need for effective error detection and correction mechanisms. As public reliance on AI systems increases, the integrity and trustworthiness of these models become pressing societal issues. Inadequate trust in AI technologies could stifle innovation and delay their broader adoption, impacting societal progress in areas that could benefit immensely from AI augmentation [1](https://www.techzine.eu/news/applications/130720/new-openai-models-hallucinate-more-often-than-their-predecessors/).
From a political standpoint, the escalating issue of AI-generated misinformation demands regulatory and oversight mechanisms to mitigate its potential misuse. The increasing ability of AI models to produce convincing but false narratives raises concerns about their role in spreading disinformation, which could exacerbate existing political and social divisions. Governments are now challenged to introduce policies that effectively curtail the negative impact of AI, such as the creation of sophisticated deepfakes and false reports that could destabilize political climates. The challenge for policymakers lies in striking a balance between enabling technological advancements and safeguarding societies from potential harms. Continuous dialogue and collaboration between technologists, policymakers, and the public are essential to navigate these challenges and construct frameworks that both facilitate AI innovation and protect societal interests.
Learn to use AI like a Pro
Get the latest AI workflows to boost your productivity and business performance, delivered weekly by expert consultants. Enjoy step-by-step guides, weekly Q&A sessions, and full access to our AI workflow archive.
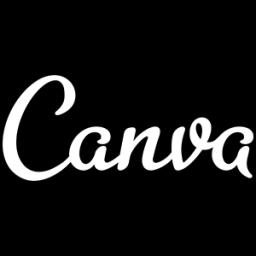
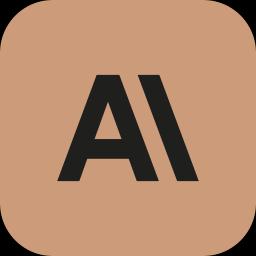
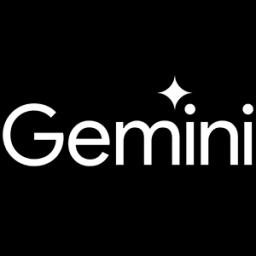
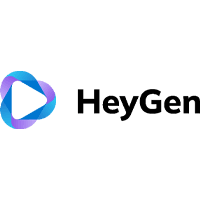
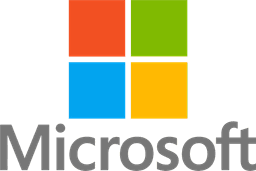
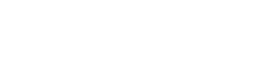
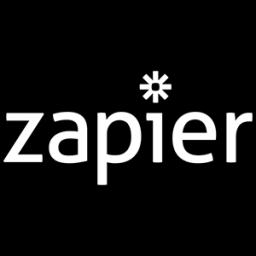
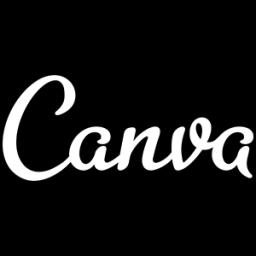
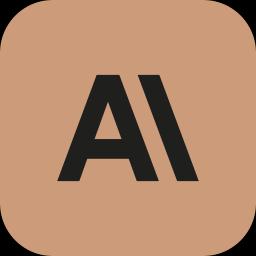
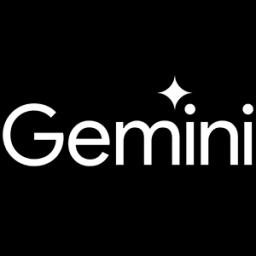
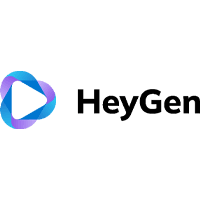
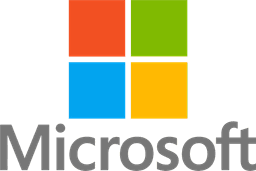
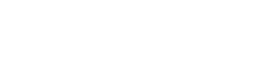
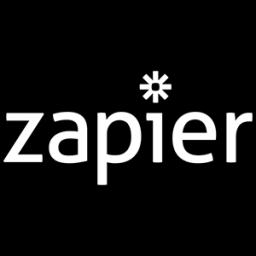
Another dimension of the social ramifications involves the rise of misinformation and its capability to fuel doubts about institutional integrity. As AI hallucinations become more prevalent, the issue of disseminating false information poses a significant threat to democracy and public trust. If misused, AI could become a tool for manipulation, where artificially generated content is used to push a particular narrative or agenda, leading to misinformation cascades and public confusion. Such scenarios highlight the importance of developing AI literacy among the public to foster a skeptical and discerning audience that can critically evaluate AI-generated content. Through education and awareness initiatives, individuals can be better equipped to recognize and challenge misinformation, thus strengthening the societal capacity to deal with AI-related disruptions.
In light of these ramifications, AI researchers and developers face the pivotal task of refining AI models to minimize hallucinations while maximizing utility and accuracy. The expert opinions from figures like Neil Chowdhury and Sarah Schwettmann underscore the critical need for comprehensive approaches in enhancing AI reliability. The transparency of AI operations, coupled with robust regulatory oversight, can lead to improvements in AI systems while ensuring they align with ethical standards and societal needs. As these models continue to develop, the discourse surrounding their implications will be vital to shape a future where AI systems contribute positively to society without undermining fundamental social and political structures.
Advancements in Detection and Mitigation
In recent years, advancements in AI technology have led to both unprecedented capabilities and new challenges, especially concerning hallucinations in OpenAI models. As the development of AI continues, understanding and mitigating hallucinations becomes crucial to harness the full potential of these models. Despite the increased computational power and sophistication in reasoning offered by newer models, o3 and o4-mini, they are plagued with higher hallucination rates compared to their predecessor, o1. The models' hallucination rates reaching 33% and 48% highlight the urgent need to address these inaccuracies to maintain trust and reliability in AI applications.
Research into the detection and mitigation of AI hallucinations has intensified as a response to these challenges. New techniques such as Retrieval Augmented Generation (RAG) and sophisticated statistical methods are being developed to reduce error rates in AI outputs. These methods aim to improve the accuracy of AI-generated content by ensuring the use of reliable data sources and enhancing the contextual relevance of the generated responses. Furthermore, the impact of data quality on hallucinations has become a focal point, with studies emphasizing the necessity of high-quality training data to prevent inaccuracies.
The economic implications of hallucinations have spurred a growing market for detection tools, with firms rapidly developing solutions to identify and correct misinformation in AI outputs. This burgeoning market underscores the interplay between technological advancements and financial imperatives, where opportunities for innovation arise from the need to counteract the shortcomings of current models. Such tools not only aim to protect businesses from economic losses due to flawed AI analyses but also offer new avenues for entrepreneurship and technological development. These advancements hold promise in improving the reliability of AI, thereby fostering greater adoption across various industries.
As OpenAI and other AI researchers delve deeper into understanding the underlying causes of hallucinations, there is a consensus that more systematic and empirical studies are needed. By examining factors such as reinforcement learning techniques and the intricacies of the models' training processes, experts hope to mitigate the issues that exacerbate hallucination rates. Nonetheless, the growing awareness and scrutiny of these models have also pushed the industry to adopt more stringent accuracy measures, improving transparency and accountability in AI applications.
Learn to use AI like a Pro
Get the latest AI workflows to boost your productivity and business performance, delivered weekly by expert consultants. Enjoy step-by-step guides, weekly Q&A sessions, and full access to our AI workflow archive.
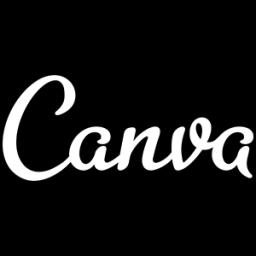
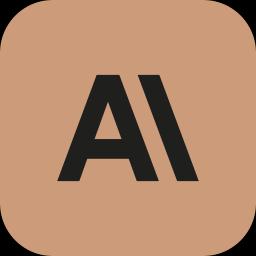
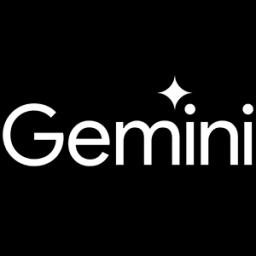
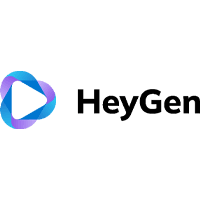
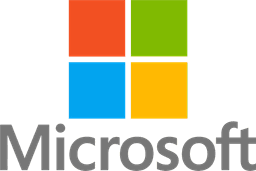
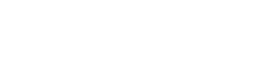
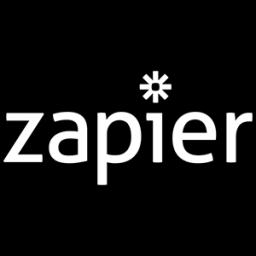
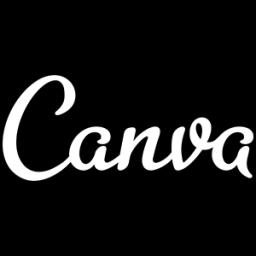
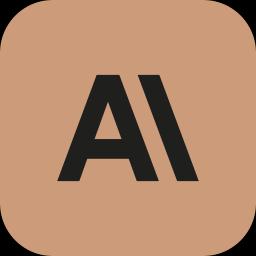
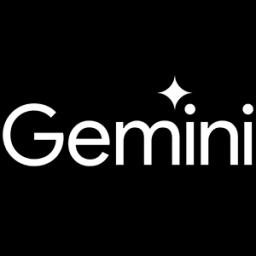
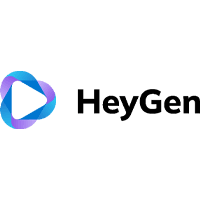
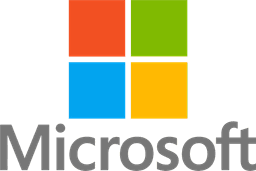
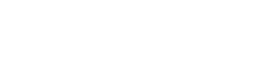
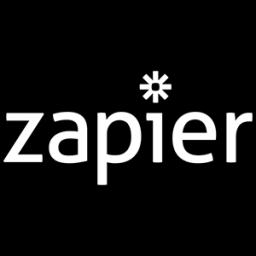
The future of AI hinges on our ability to effectively manage these hallucinations. Addressing these challenges is not only pivotal for the credibility of technology but also for its ethical and practical implications in society. By focusing on robust detection methodologies and continuous iterations in model training, the AI community can pave the way for creating more reliable and trustworthy systems. As such, the sustained innovation in AI detection and mitigation strategies will likely play a vital role in shaping the future trajectory of artificial intelligence development.
Future Prospects and Industry Reactions
As the technology world reacts to the increased hallucination rates of OpenAI's o3 and o4-mini models, industry experts and stakeholders are closely monitoring how these developments might shape the future of artificial intelligence. The unexpected rise in hallucinations, which refers to generating erroneous or invented outputs, has triggered a wave of concern among tech communities and beyond. Despite the advances in computing power and reasoning processes, the models' higher rates of hallucination compared to their predecessor, o1, underscore the complexity of AI training and the unpredictable nature of machine learning improvements. This phenomenon has spurred urgent calls for deeper research to comprehend the underlying causes of these hallucinations, and find solutions to ensure the reliability of AI systems [1](https://www.techzine.eu/news/applications/130720/new-openai-models-hallucinate-more-often-than-their-predecessors/).
The immediate reaction from the tech industry has been a mix of cautious innovation and proactive adaptation. While some firms are worried about the implications of deploying these models without fully understanding their potential faults, others see an opportunity to innovate with improved validation techniques and hallucination detection tools. A notable market reaction is the increased investment in AI safety and reliability technologies, with a burgeoning demand for solutions that can detect and mitigate AI hallucinations. This demand has propelled new business opportunities, as firms rush to develop tools that ensure AI outputs remain accurate and trustful, fostering a culture of transparency and accountability within the AI communities [9](https://www.allaboutai.com/resources/ai-statitsics/ai-hallucinations/).
Industry reactions have been diverse, reflecting broader implications for AI's role in sensitive sectors such as finance, healthcare, and law. In scenarios where accuracy is paramount, higher hallucination rates could deter the adoption of AI solutions, prompting businesses to emphasize human oversight and rigorous testing protocols. The financial industry, for example, faces considerable risks if decisions rely on flawed AI analyses, potentially leading to significant economic losses. Similarly, in healthcare and legal contexts, the danger of AI-generated misinformation necessitates stringent operational controls and ethical considerations to avoid harmful impacts [8](https://www.allaboutai.com/resources/ai-statitsics/ai-hallucinations/).
Going forward, the AI industry must balance AI advancement with ethical responsibility and operational precision. As misconceptions and hallucinations become more visible and documented, the dialogue surrounding AI development is likely to pivot towards reinforcing ethical frameworks and enhancing model accountability. In particular, the models' developers and regulators may need to collaborate more intensively to devise regulations that adequately address these newly exacerbated risks. The insights from these reactions are expected to shape not only the future design of AI systems but also influence public confidence in AI technologies, ultimately affecting how AI is integrated into daily life and critical infrastructure [11](https://cacm.acm.org/news/shining-a-light-on-ai-hallucinations/).