AI Milestone or Economic Quagmire?
OpenAI's o3 Model: Soaring Performance, Soaring Costs
Last updated:

Edited By
Mackenzie Ferguson
AI Tools Researcher & Implementation Consultant
OpenAI's latest o3 model has shattered previous benchmarks, boasting an 87.5% score in high-compute mode on the ARC-AGI test. However, the impressive performance comes at a high cost, with even low-compute tasks priced around $20 each. As the tech world buzzes, concerns arise over the economic viability and accessibility of such powerful AI technology, especially with the 'mini' version expected in January 2024. We explore the implications of these advancements in AI.
Introduction to OpenAI's o3 Model
OpenAI's latest advancement in artificial intelligence, the o3 model, has sparked significant interest and debate in the tech community. This model represents a leap forward in AI capabilities, particularly in its performance on benchmarks that measure advanced reasoning. Unlike its predecessors, o3 incorporates 'test-time compute' functionality, allowing it to consider multiple possible answers before committing to one. This not only enhances accuracy but also comes at a high computational cost. While OpenAI claims impressive performance improvements with o3, questions about its practicality due to the associated costs persist. This introduction will explore the groundbreaking elements of the o3 model while addressing the economic and accessibility concerns it raises. In the following sections, we delve into these aspects, drawing from expert opinions, related events, and public reactions.
Difference Between o3 and Previous Models
OpenAI's latest model, known as o3, marks a significant leap in AI technology over its predecessors, such as o1. The o3 model is distinct because it employs a method called 'test-time compute,' which allows it to assess multiple potential outcomes before delivering a final answer. This innovative approach has yielded superior performance, achieving a remarkable score of 87.5% on the ARC-AGI benchmark in its high-compute mode. By contrast, the o1 model managed a score of only 32%, highlighting o3’s advanced capabilities.
Learn to use AI like a Pro
Get the latest AI workflows to boost your productivity and business performance, delivered weekly by expert consultants. Enjoy step-by-step guides, weekly Q&A sessions, and full access to our AI workflow archive.
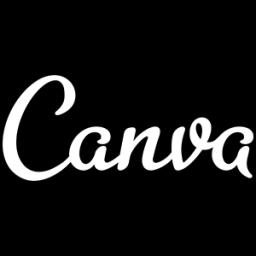
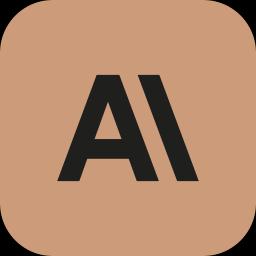
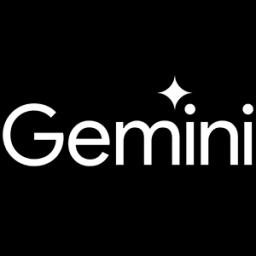
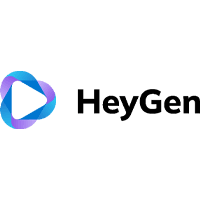
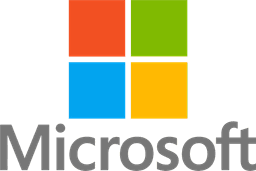
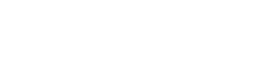
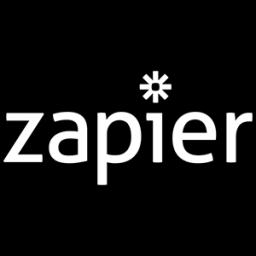
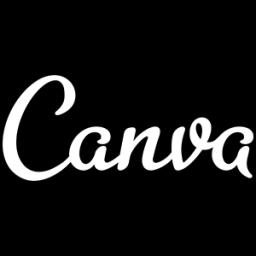
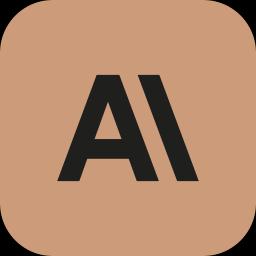
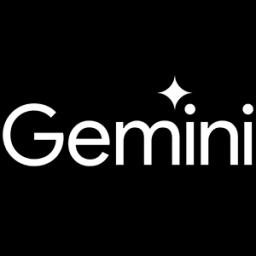
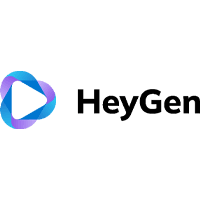
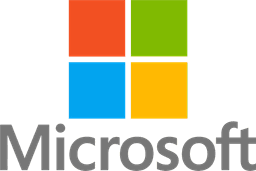
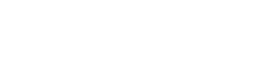
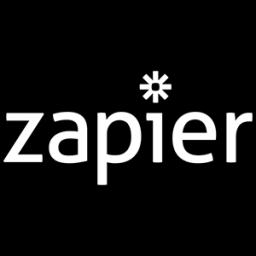
Despite its impressive performance, the o3 model comes with substantial cost implications. The high-compute mode of o3, while powerful, incurs costs exceeding $1,000 per task, a stark contrast to its low-compute version which, while still costly at approximately $20 per task, represents a more economically viable option. These costs provoke questions regarding the economic sustainability of deploying such a resource-intensive model, especially when weighed against its available computational power and accuracy.
The introduction of o3 also ignites a debate about the balance between cost and benefit in AI applications. Critics point out the potential diminishing returns of scaling AI models to such sophisticated levels, particularly if the economic and practical accessibility remains limited to well-funded organizations. Furthermore, there’s skepticism in the community about whether the advancements in o3 truly edge closer to artificial general intelligence (AGI), especially considering the specific areas it exceeds in such as scientific reasoning and coding, yet still struggles with simpler, human-trivial tasks.
While o3 has not yet been released to the public, anticipation is high for the forthcoming 'o3-mini' variant, anticipated to launch in January. This version is expected to offer a more balanced approach, providing many of o3's capabilities in a more accessible and economic format. The reduced computational costs and adjustable reasoning levels could make advanced AI power available to a broader range of applications and users, potentially democratizing access to such technologies.
Cost Analysis of o3 Model Usage
OpenAI's recently released o3 model presents a noteworthy progression in AI technology, reflecting substantial improvements in performance when compared to its predecessors. The o3 model employs an innovative "test-time compute" approach, which empowers it to explore an array of possibilities prior to arriving at an answer. While this method contributes to its enhanced accuracy, it inevitably results in increased computational costs. In benchmark evaluations, the high-compute mode of o3 achieved an impressive 87.5% on the ARC-AGI test compared to the mere 32% scored by the previous o1 model. However, this higher performance comes at a cost, with the high-compute version amounting to over $1,000 per task and the less intensive low-compute version costing about $20 per task. Despite its remarkable performance metrics, the o3 model is not yet available for public use, with a "mini" version expected in January.
Learn to use AI like a Pro
Get the latest AI workflows to boost your productivity and business performance, delivered weekly by expert consultants. Enjoy step-by-step guides, weekly Q&A sessions, and full access to our AI workflow archive.
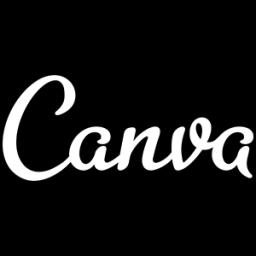
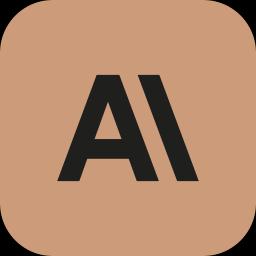
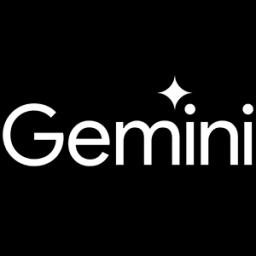
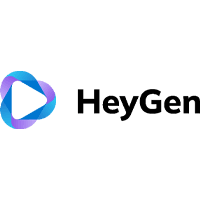
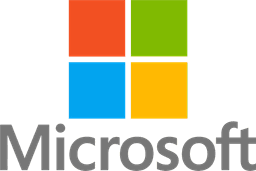
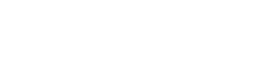
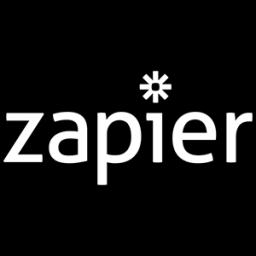
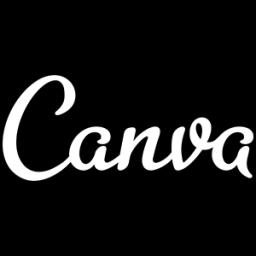
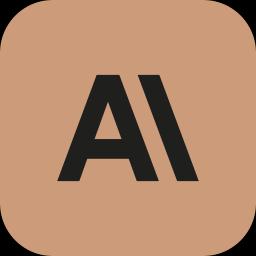
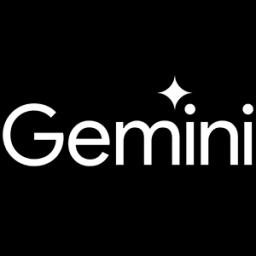
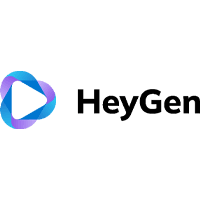
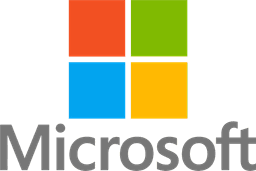
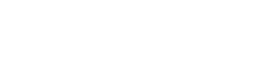
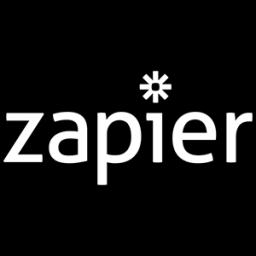
Availability and Release Date of o3
The anticipation around OpenAI’s new model, o3, is palpable as discussions about its availability and release dates gain momentum. The model promises groundbreaking advancements in artificial intelligence but is yet to be released to the public. Enthusiasts and AI experts alike are particularly eager for the "mini" version, projected to be released in January, which is expected to bring more practicality and accessibility to its users. This impending release generates a buzz in the tech community as potential users weigh the cost implications against the potential benefits.
Performance of o3 on ARC-AGI Benchmark
The performance of OpenAI's latest model, o3, on the ARC-AGI benchmark showcases a remarkable advancement in AI capabilities, achieving an impressive score of 87.5% in its high-compute mode, far surpassing the 32% scored by its predecessor, o1. This achievement demonstrates the potential of o3's "test-time compute" feature, which allows the model to explore multiple possibilities before arriving at a solution, thus greatly enhancing its accuracy. While these advancements mark a significant step towards more capable AI systems, they come with substantial trade-offs in computational costs, raising critical discussions about the balance between enhanced performance and economic viability.
The o3 model's notable performance on the ARC-AGI benchmark signifies its potential to redefine AI application boundaries, particularly in fields requiring complex reasoning and precision, such as scientific research and advanced software development. The improved performance is attributed to the extensive computational analysis conducted during the model's operation, a process that opens up new avenues for AI utilization but also intensifies concerns over the sustainability of such powerful models in real-world applications due to their high operational costs. The exceedingly high cost-per-task in the high-compute version, reported to be over $1,000, prompts a re-evaluation of AI deployment strategies, especially concerning its integration into existing commercial technologies like ChatGPT Plus.
Despite these cost-related challenges, the model also offers a lower-cost version which still achieves a robust 76% on the ARC-AGI benchmark, making it significantly more accessible while maintaining a solid performance profile. This option could potentially bridge the gap for users and organizations unable to afford the high-compute version. Furthermore, discussions about the upcoming "mini" version of o3 suggest an interesting pivot towards developing more cost-effective and universally accessible AI solutions, facilitating wider adoption and fostering innovation across various sectors. However, the current trade-off between cost and performance continues to fuel debates on the feasibility and equity of deploying such sophisticated AI models in a broad spectrum of applications.
As the development and application of AI models like o3 continue to progress, it is crucial to navigate the challenges of high computational costs and ensure that advancements in AI do not exacerbate existing technological divides. The substantial achievements of o3 in specialized computational tasks highlight the model's potential to considerably impact various fields, yet they underscore the importance of creating sustainable AI ecosystems that are both economically feasible and accessible to a diverse range of users. Moving forward, it will be important for AI researchers and developers to focus on lowering computational demands and costs, thereby meeting both performance and economic accessibility goals.
Economic Implications of o3's High Costs
The economic implications of OpenAI's newly released o3 model, hailed for its impressive performance, are significant and multifaceted. A key feature of the o3 model is its 'test-time compute' capability, which allows it to explore multiple possibilities before answering questions. This approach significantly enhances its accuracy but comes with a marked increase in computational costs, such as charging over $1,000 per task for its high-compute mode and about $20 per task for lower computing demands.
Learn to use AI like a Pro
Get the latest AI workflows to boost your productivity and business performance, delivered weekly by expert consultants. Enjoy step-by-step guides, weekly Q&A sessions, and full access to our AI workflow archive.
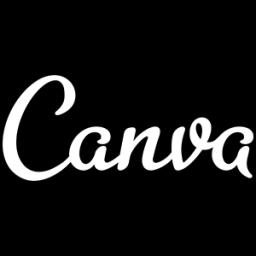
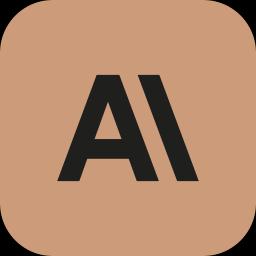
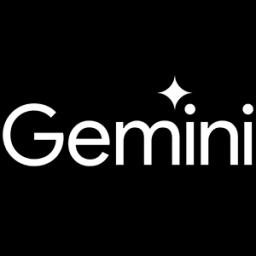
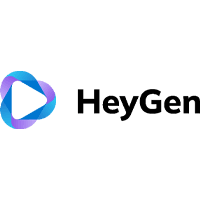
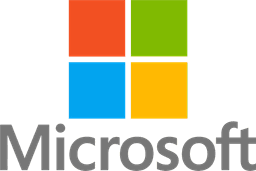
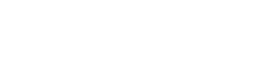
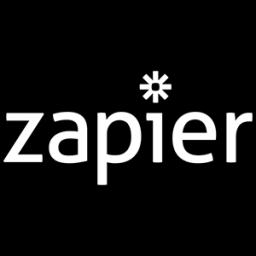
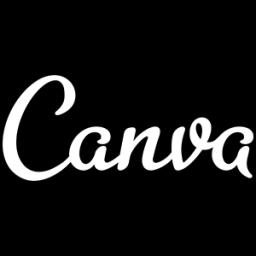
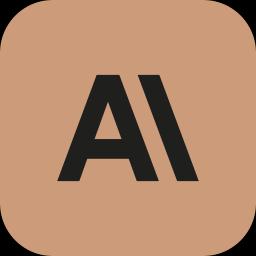
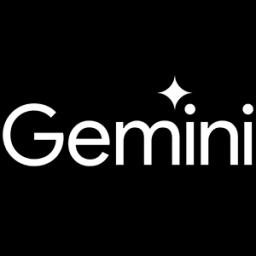
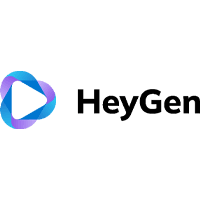
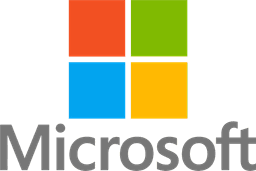
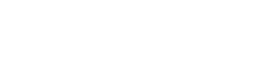
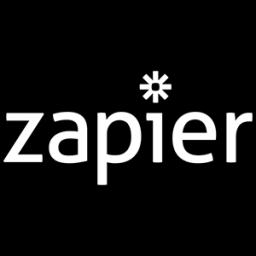
This cost structure has raised critical questions regarding the economic viability of leveraging such advanced models in practical applications, particularly for user-facing products like ChatGPT Plus. The high expenses linked to o3 could present a barrier, limiting accessibility mainly to well-funded organizations and potentially widening the technological gap between these entities and smaller companies or individual users.
The release has also sparked public discourse concerning the diminishing returns of AI scaling and the broader implications in terms of accessibility and equity. In response, experts suggest that as AI technologies advance and scale, associated costs might decrease, increasing their economic feasibility. Nonetheless, currently, the high computational demands and financial costs of the o3 model pose challenges to its widespread adoption within the industry.
Additionally, there is an anticipation of shifts within the job market as AI integration continues to evolve, with increasing demand for high-skill roles associated with AI technologies. Furthermore, the financial dynamics surrounding AI development may prompt increased investment in computational infrastructure to support future advancements.
Overall, the economic landscape shaped by the o3 model underscores the need for balanced innovation and accessibility. It also emphasizes the importance of developing strategies to mitigate the cost barriers associated with adopting such cutting-edge AI models, ensuring broader economic inclusivity and benefit.
Related Events in the AI Industry
The launch of OpenAI’s o3 model has stirred significant discussion within the AI industry, largely due to its advanced capabilities and the high costs associated with its computational demands. The model’s impressive performance on benchmarks such as the ARC-AGI—where it scored 87.5% in high-compute mode—has been both praised and scrutinized. This performance is a considerable improvement over its predecessor, o1, which scored a mere 32%, highlighting the dramatic advancements in AI capabilities brought about by o3’s “test-time compute.” However, the economic demands of utilizing o3, with costs exceeding $1,000 for high-compute tasks, have sparked widespread debate regarding the model's economic sustainability.
Other leading tech companies are facing similar challenges and opportunities in the wake of these developments. For example, Google has delayed the launch of its anticipated Gemini Ultra model due to resource constraints, a move that underscores how these computational needs are impacting project timelines industry-wide. Concurrently, Anthropic’s development of constitutional AI to align models with human values during training reflects a strategic pivot toward reducing costs at the training stage, potentially offsetting the need for expensive fine-tuning in future applications.
Learn to use AI like a Pro
Get the latest AI workflows to boost your productivity and business performance, delivered weekly by expert consultants. Enjoy step-by-step guides, weekly Q&A sessions, and full access to our AI workflow archive.
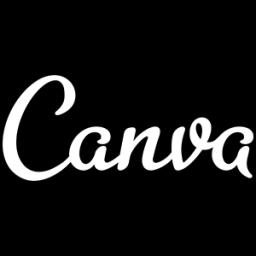
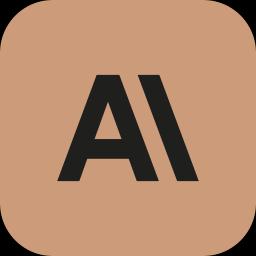
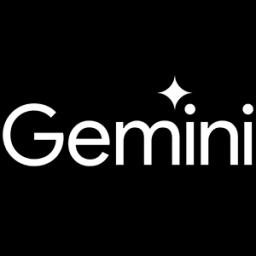
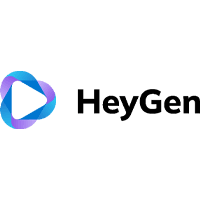
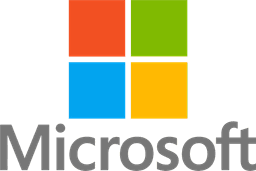
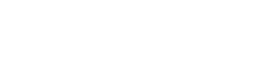
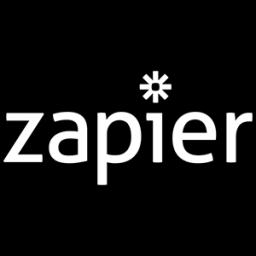
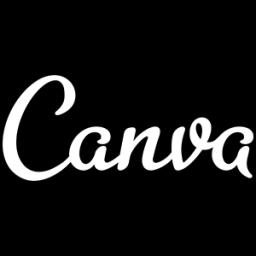
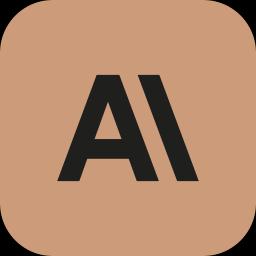
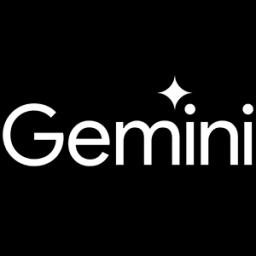
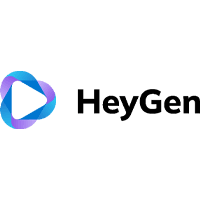
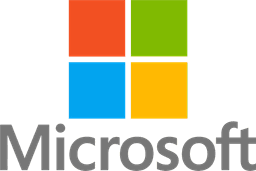
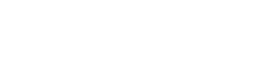
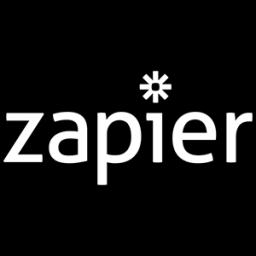
The AI industry is also dealing with hardware bottlenecks, as evidenced by Nvidia’s H100 GPU shortages, which have strained AI development capabilities. The shortfall highlights the dependency on specialized hardware for training and scaling large AI models, further emphasizing the importance of robust, scalable infrastructure in supporting the industry's growth. In contrast, Meta’s decision to open-source its Llama 2 model aims to democratize AI development, providing accessibility to researchers and smaller enterprises that might otherwise be unable to shoulder the costs associated with developing cutting-edge technologies.
Furthermore, the U.S. government's investment in AI infrastructure, with initiatives like the National AI Research Resource, demonstrates a commitment to supporting innovation while managing costs. These strategic moves within the industry and by governmental bodies suggest a future wherein economic considerations and resource management will be pivotal in determining the trajectory of AI development. The landscape indicates an evolving balance between technological advancement and practical, sustainable application that is responsive to broad industry needs and constraints.
Expert Opinions on OpenAI's o3 Model
OpenAI's third-generation model, known as 'o3', introduces a novel approach in AI with its use of 'test-time compute,' a process allowing the model to explore various possibilities before finalizing its answer. This approach leads to significant improvements in performance accuracy, particularly evident in its 87.5% score on the ARC-AGI benchmark using high-compute mode. However, such advancements come at a steep financial cost, with tasks in high-compute mode costing over $1,000 each. This has sparked discussions on the economic viability and practicality of deploying such advanced models in everyday applications.
Expert opinions are divided regarding the o3 model's impact and potential. François Chollet, creator of the ARC-AGI benchmark, acknowledges the model's impressive capabilities, describing its scores as a significant leap in AI development. However, he cautions against prematurely viewing the model as having achieved artificial general intelligence (AGI) and highlights its struggles with tasks that are trivial for humans. His sentiment is echoed by Gary Marcus, a notable AI critic, who calls for more independent scientific evaluations and emphasizes the need for broader comparison with other AI models developed outside of commercial labs.
The introduction of the o3 model raises significant accessibility and equity concerns due to its high operational costs, estimated to range from $17 to $3,000 per task. This high cost presents a barrier to widespread adoption, potentially exacerbating existing digital divides by limiting access to well-resourced organizations. Meanwhile, potential users are anticipating the release of a 'mini' version of the model, expected to offer more practical and affordable computational solutions by adjusting reasoning levels and balancing power against cost.
Public reaction to the o3 model reflects a mixture of enthusiasm and skepticism. While the model's performance in specific domains like coding and scientific reasoning is celebrated, there are notable concerns about its economic viability, with fears that its high costs could limit its broader applicability and increase the technological gap between different sectors. Social media discussions highlight these issues, while looking forward to the release of o3-mini, which promises more accessible capabilities to a wider audience.
Learn to use AI like a Pro
Get the latest AI workflows to boost your productivity and business performance, delivered weekly by expert consultants. Enjoy step-by-step guides, weekly Q&A sessions, and full access to our AI workflow archive.
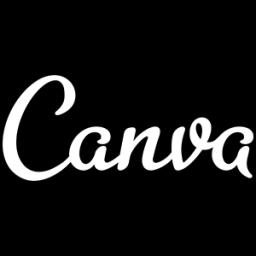
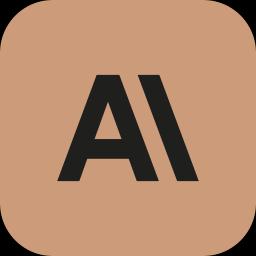
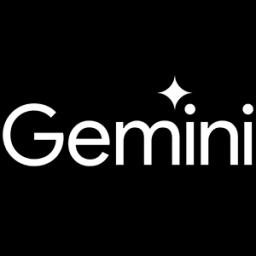
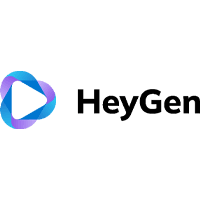
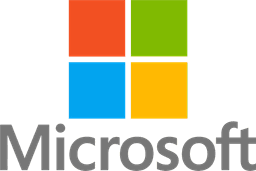
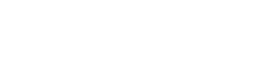
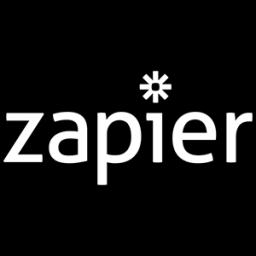
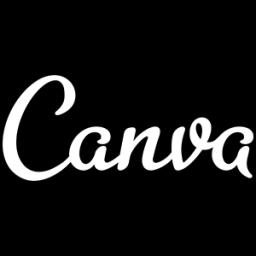
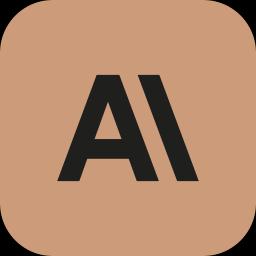
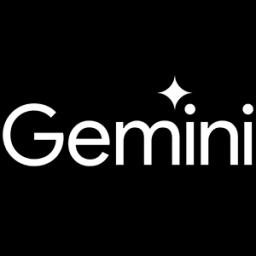
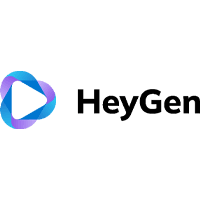
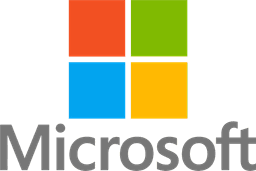
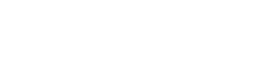
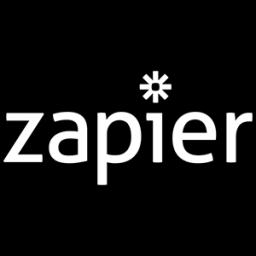
Looking forward, the o3 model's cost implications suggest a reshaping of the AI landscape. Economically, there could be a widening gap between well-funded and smaller organizations, though costs might decrease as the technology matures. The high expense currently favors entities that can afford extensive computational infrastructure, but future advancements might democratize access to powerful AI tools. These shifts could lead to significant societal, political, and technological changes, driving further innovation while prompting discussions on AI governance and regulation.
Public Reactions to the o3 Model
The debut of OpenAI's o3 model has certainly stirred public emotions, split between awe for its breakthrough capabilities and apprehension regarding its steep costs. On one hand, the o3's ability to perform complex scientific reasoning and coding tasks well beyond average human capacity has been met with fascination and applause, marking it as a major leap in AI capability. This is a testament to o3's adoption of 'test-time compute,' a method allowing the model to explore numerous possibilities before arriving at answers, thus maximizing its performance.
However, the financial implications are undeniable, with the high-compute variant of o3 demanding over $1,000 per task, a figure that raises eyebrows among the public and stakeholders alike. This factor not only sparks debates about its accessibility and economic feasibility but also brings to the forefront issues related to digital equity. There is a palpable concern that such premium costs might widen the tech divide, benefiting affluent organizations while sidelining smaller enterprises and communities.
Many skeptics also voice apprehensions about o3's purported capabilities, questioning the authenticity of claims surrounding Artificial General Intelligence (AGI). These critics argue that while o3's benchmarks may be remarkable, they shouldn't be mistaken for true general intelligence, which requires a broader spectrum of skills and understanding.
Amid these discussions, excitement builds around the scheduled release of 'o3-mini,' a version anticipated to be more budget-friendly and practical for everyday purposes. The flexibility offered by this iteration, especially in terms of adjustable reasoning levels that provide a balance between computational demand and cost, is seen as a promising option for consumers from diverse backgrounds.
Social media platforms and forums are buzzing with speculative discussions about the long-term implications of o3. While some users remain hesitant about diving into such a costly tool, others speculate on future cost reductions as the technology evolves. Meanwhile, influencers critique the media buzz as inflated, yet acknowledge the potential, transformative power of o3 once economic and technological balance is achieved.
Learn to use AI like a Pro
Get the latest AI workflows to boost your productivity and business performance, delivered weekly by expert consultants. Enjoy step-by-step guides, weekly Q&A sessions, and full access to our AI workflow archive.
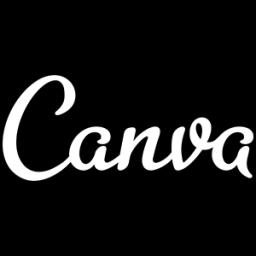
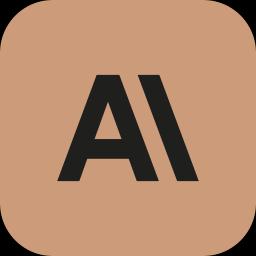
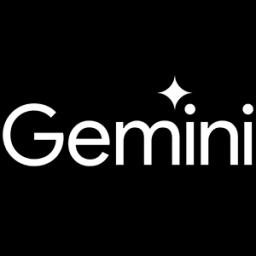
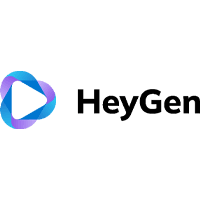
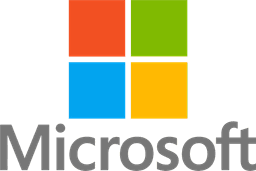
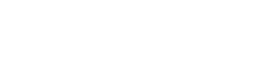
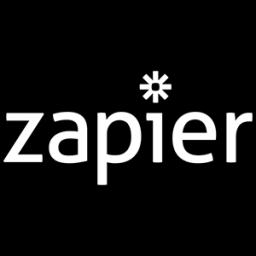
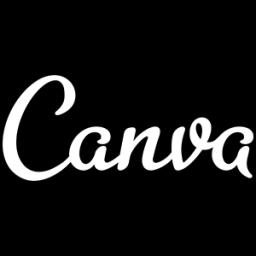
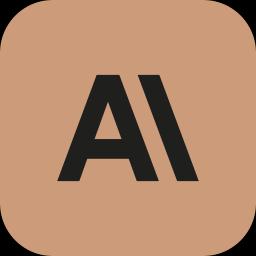
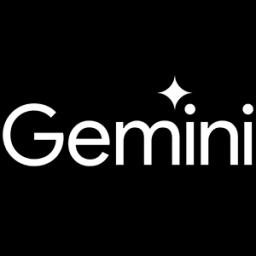
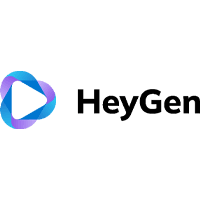
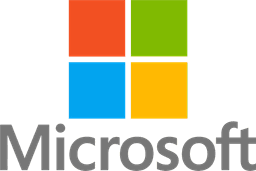
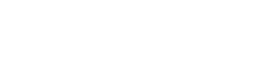
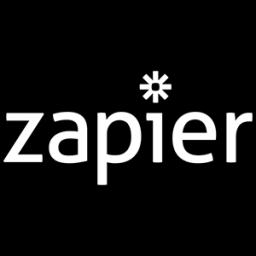
Overall, the rollout of the o3 model represents a vivid snapshot of the current state of AI, punctuated by technological awe, economic scrutiny, and forward-looking curiosity. The interplay of these dynamics reflects a society eager to explore the frontiers of artificial intelligence while grappling with the pragmatic realities it presents.
Future Implications of the o3 Model
The release of OpenAI's o3 model marks a significant milestone in the advancement of artificial intelligence, showcasing capabilities that far exceed those of traditional models. This improvement, however, comes with a steep increase in computational costs, which poses potential implications for the future landscape of AI technology, economy, and society at large.
Economically, the o3 model's high cost, estimated at over $1,000 per task for the high-compute mode, could widen the technological gap. Well-funded organizations might be the primary beneficiaries, while smaller entities without substantial financial resources may struggle to keep pace. Over time, as AI technology matures and scales, there is potential for development costs to decrease. This could democratize access to AI advancements, allowing a broader range of businesses and institutions to benefit from these powerful tools.
From a social perspective, there's an ongoing debate about the digital divide exacerbated by the costly nature of the o3 model. While the model can potentially enhance scientific research and problem-solving by surpassing human performance in specific tasks, its high price raises ethical questions about accessibility and fairness. AI that largely benefits only affluent industries could contribute to societal inequalities, unless deliberate efforts are made to make these technologies more accessible to all.
Politically, the rise of sophisticated AI models like o3 is placing pressure on governments to address these inequities. There is a growing call for policies that ensure equitable access to cutting-edge AI technologies and resources. Additionally, international competition in AI could shift global power dynamics as countries and corporations vie for technological superiority. This heightened focus on AI governance and policy-making will shape how these tools are developed and who benefits from them.
Finally, the technological implications are vast. The o3 model represents an acceleration towards more specialized and high-performing AI applications, particularly in areas like mathematics and coding. However, the pathway to achieving artificial general intelligence remains contested, with experts debating the feasibility and timing of such advancements. With developments like the anticipated "o3-mini" model, there's potential for more adaptable and accessible AI tools that could drive wider adoption and integration into various sectors.
Learn to use AI like a Pro
Get the latest AI workflows to boost your productivity and business performance, delivered weekly by expert consultants. Enjoy step-by-step guides, weekly Q&A sessions, and full access to our AI workflow archive.
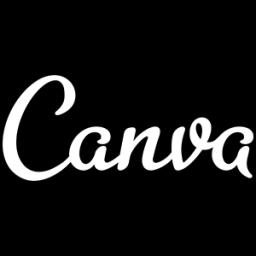
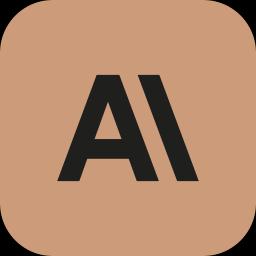
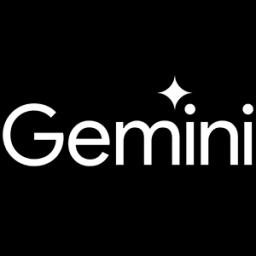
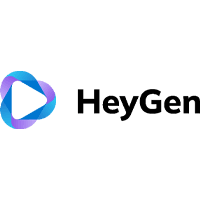
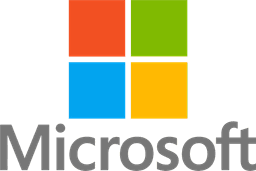
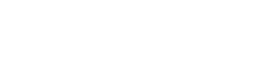
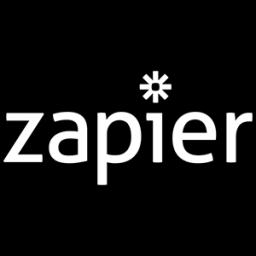
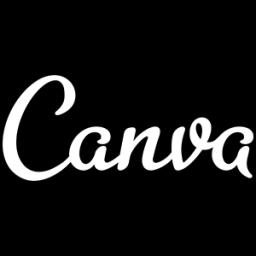
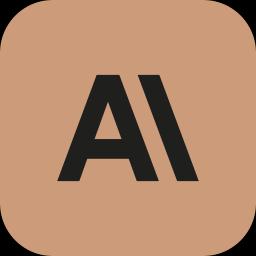
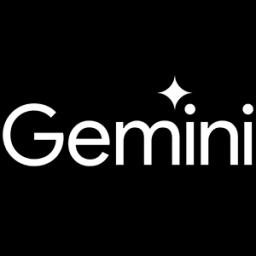
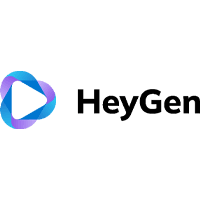
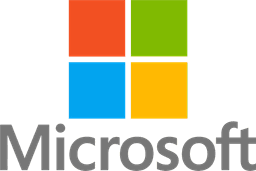
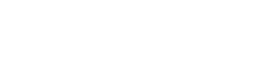
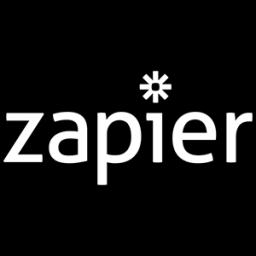