Promises and Pitfalls
Synthetic Data: The Double-Edged Sword of AI Training
Last updated:

Edited By
Mackenzie Ferguson
AI Tools Researcher & Implementation Consultant
The rising trend of using synthetic data for AI training is reshaping the industry landscape. It's hailed as a cheaper, faster solution addressing privacy concerns but comes with risks like amplified biases and the ominous 'model collapse.' Big names like Google and Microsoft are in the race, as regulations and market dynamics evolve.
Introduction to Synthetic Data
The emergence of synthetic data has piqued the interest of various sectors, highlighted by its core advantage of avoiding the costly and ethically problematic nature of acquiring traditional datasets. Companies like Writer, Microsoft, Google, Nvidia, and Hugging Face are paving the way in leveraging this cutting-edge approach for AI model training. Synthetic data, artificially generated to mimic real-world datasets, emerges as a scalable solution that promises to address pressing concerns related to privacy and biases.
However, as much as synthetic data presents opportunities, it rides the boundary with several inherent risks. The quality of artificial data is intrinsically tied to the robustness of the original data it’s derived from. Poor quality or biased original datasets could result in what's known as 'model collapse', where the continual use of such data degrades the model's ability over time. Hence, a heavy reliance on synthetic data mandates vigilant quality control and curation.
Learn to use AI like a Pro
Get the latest AI workflows to boost your productivity and business performance, delivered weekly by expert consultants. Enjoy step-by-step guides, weekly Q&A sessions, and full access to our AI workflow archive.
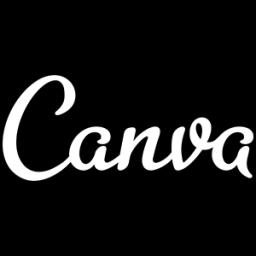
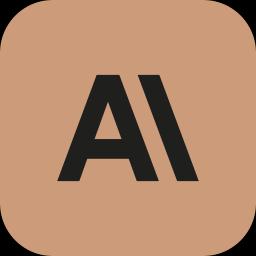
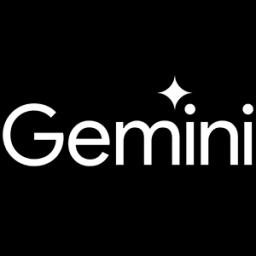
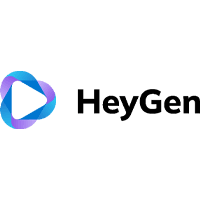
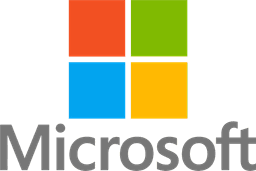
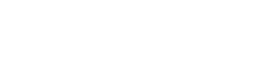
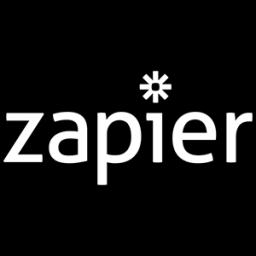
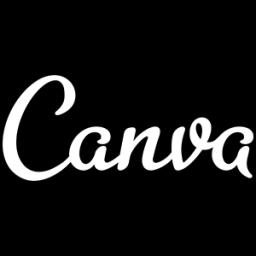
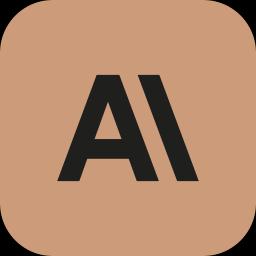
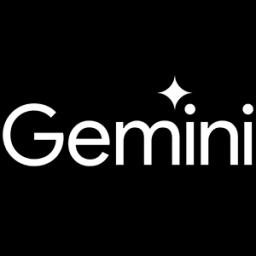
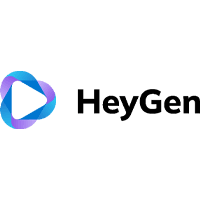
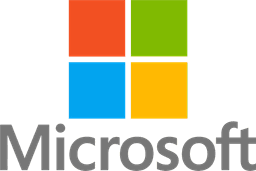
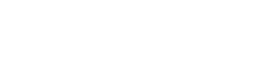
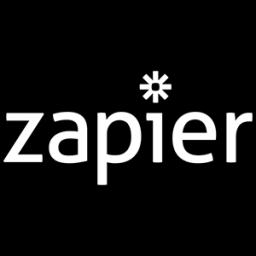
The increasing adoption of synthetic data in AI models hasn't been without debate. Public sentiment hovers between optimism about the technological benefits and apprehension regarding its pitfalls. Concerns about data quality, the potential for amplified biases, and fears of losing model efficacy are common. Despite its novelty and potential, synthetic data is seen as an adjunct rather than a replacement for real-world data, prompting discussions surrounding AI ethics and governance.
The growth in synthetic data use stands poised to reshape the economic, social, political, and technological landscape. Economically, it promises cost reductions in AI development and job creation in new technological realms. Socially, it portends improved privacy standards but simultaneously evokes public scrutiny. Politically, the push for synthetic data sparks discourse around regulations, like the EU's recent propositions, illuminating the essential synergy required between synthetic data and ethical AI advancements. As these changes unfold, the call for balanced AI models relying concomitantly on synthetic and real data intensifies, aiming to maximize efficacy while minimizing risks.
The Need for Synthetic Data
Data is the cornerstone of artificial intelligence development, yet acquiring vast amounts of quality data has become increasingly challenging. The traditional methods of data gathering are not just costly but often raise ethical concerns, especially regarding privacy and bias. This scenario has paved the way for synthetic data, offering a promising alternative that seeks to address these challenges head-on.
Synthetic data refers to artificially generated information that imitates real-world data. It is produced using algorithms that have been trained on real datasets to understand and replicate the patterns within them, leading to the creation of new, synthetic datasets. The emergence of synthetic data is seen as a watershed moment for AI, enabling development in areas where data was previously inaccessible or difficult to obtain.
Learn to use AI like a Pro
Get the latest AI workflows to boost your productivity and business performance, delivered weekly by expert consultants. Enjoy step-by-step guides, weekly Q&A sessions, and full access to our AI workflow archive.
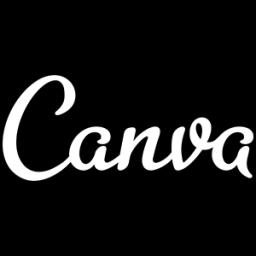
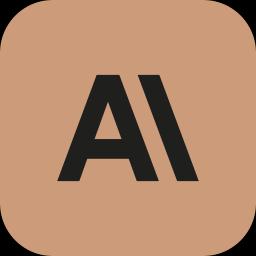
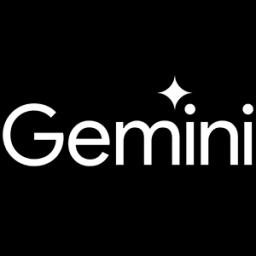
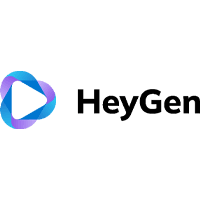
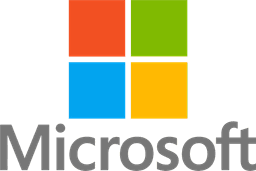
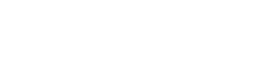
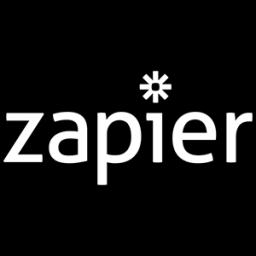
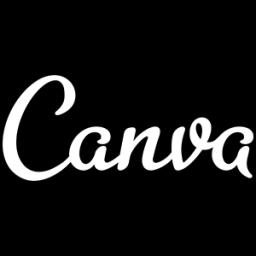
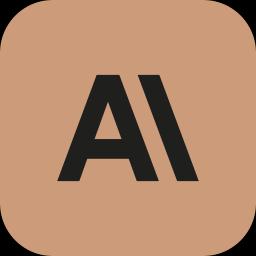
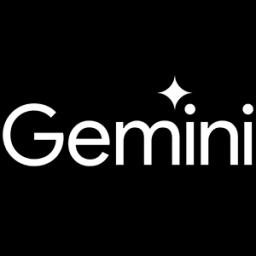
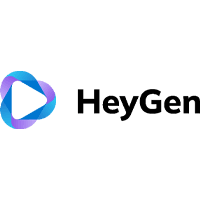
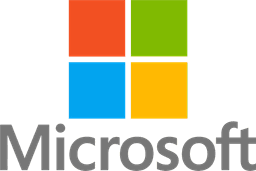
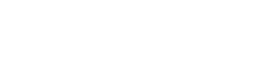
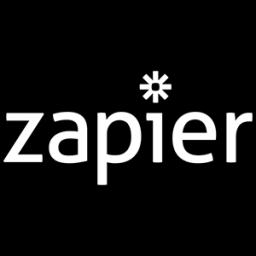
One of the chief benefits of synthetic data is its ability to provide a scalable, faster, and cost-effective solution for data acquisition. Unlike traditional methods, synthetic datasets can be produced rapidly without the need for time-consuming collection processes. Furthermore, because the data is generated artificially, it sidesteps numerous privacy issues, providing an ethical way to enhance AI model training. This advantage is particularly significant given the increasing demand for data and the constraints faced in obtaining it legally and ethically.
Despite its advantages, synthetic data is not without its risks. The quality of the synthetic datasets is heavily reliant on the original data used to train the algorithms. If the original data is biased, those biases can be magnified in the synthetic outputs, potentially leading to skewed or unfavorable outcomes in AI models. Additionally, there's the risk of "model collapse," a phenomenon where AI models become less effective over successive generations when trained primarily on synthetic data. This highlights the importance of combining synthetic with real-world data to maintain model performance and integrity.
Human oversight remains a crucial element of leveraging synthetic data effectively. Quality control and dataset curation are essential to ensuring the reliability and utility of the synthetic data used in AI training. Companies such as Microsoft, Google, and Nvidia are pioneering efforts in synthetic data integration, applying stringent validation practices to mitigate the risks of data inaccuracy and poor model outcomes. These collaborative efforts underscore a growing recognition of synthetic data’s potential, but also of the perils it may bring if not managed carefully.
Benefits of Synthetic Data
In the rapidly evolving landscape of artificial intelligence (AI), synthetic data has emerged as a pivotal tool, offering significant advantages to companies grappling with the challenges of traditional data acquisition. One of the primary drivers for the adoption of synthetic data is its cost-effectiveness and efficiency. In contrast to the expensive and labor-intensive process of collecting real-world data, synthetic data can be generated quickly and at a fraction of the cost. This is particularly beneficial in fields where acquiring real data is fraught with ethical concerns or logistical hurdles, such as in healthcare or financial services.
Moreover, synthetic data offers a promising solution to the ever-pressing issue of data privacy. By generating artificial datasets that mimic real-world data without exposing sensitive information, companies can mitigate privacy concerns that often accompany the use of real data. This capability is especially crucial in an era where data breaches and privacy violations are commonplace, enabling businesses to uphold privacy standards while still harnessing valuable data for AI model training.
In addition to cost and privacy benefits, synthetic data plays a crucial role in addressing biases inherent in real-world data. Because synthetic data can be crafted to represent a more balanced view of the world, it provides a pathway to develop fairer AI models. By eliminating or reducing biases present in the original training datasets, synthetic data helps in creating AI systems that are more equitable and just.
Learn to use AI like a Pro
Get the latest AI workflows to boost your productivity and business performance, delivered weekly by expert consultants. Enjoy step-by-step guides, weekly Q&A sessions, and full access to our AI workflow archive.
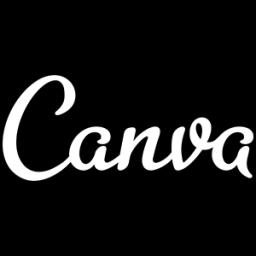
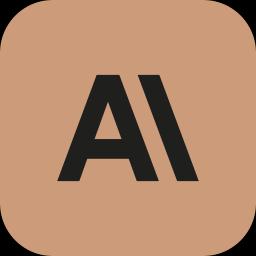
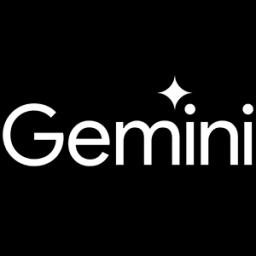
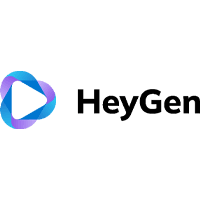
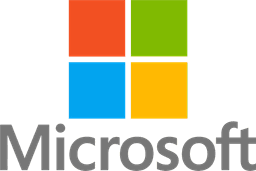
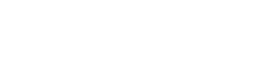
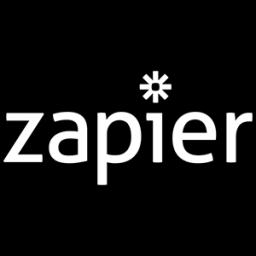
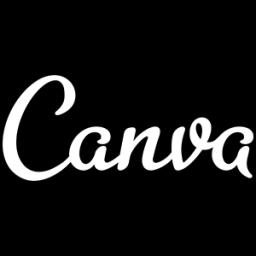
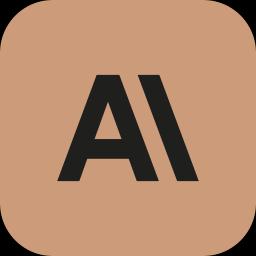
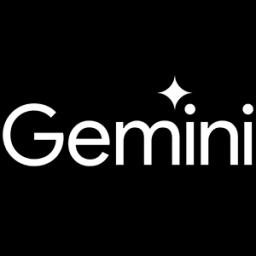
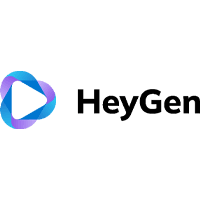
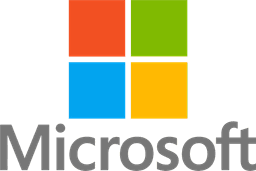
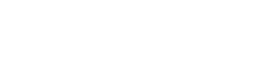
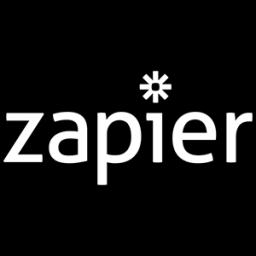
The scalability of synthetic data generation is another compelling advantage. Traditional data collection methods can be painstakingly slow and rarely keep pace with the rapid development cycles of AI technologies. Synthetic data, however, can be scaled up or down with ease, allowing AI models to be trained on vast datasets that would otherwise be unattainable. This flexibility is invaluable for industries that require large volumes of data to innovate and remain competitive.
However, it's important to note that the utility of synthetic data is not without its challenges. The quality of synthetic data is heavily dependent on the original datasets used to create it; if the base data is flawed or biased, these issues can be compounded in the synthetic outputs, leading to 'model collapse' where AI systems underperform due to reliance on low-quality synthetic data. This underscores the necessity for rigorous quality control and the integration of human oversight in the synthetization process to ensure that AI models trained on synthetic data are both reliable and effective.
Risks and Concerns
The utilization of synthetic data in AI model training presents several risks and concerns that stakeholders must navigate. The primary challenge is ensuring the quality of synthetic data. Since synthetic data is generated using algorithms trained on real datasets, its quality is heavily reliant on the original data. If the original datasets contain biases or inaccuracies, these can be exponentially amplified in the synthetic data, leading to compromised AI models.
One significant risk is the phenomenon known as 'model collapse,' where AI models, when trained predominantly on degraded synthetic data, become less functional and more biased. This situation arises particularly when models are recursively trained on synthetic data without adequate incorporation of real-world data. Such a process can result in AI systems that lack creativity and fail to perform accurately in real-world scenarios.
Currently, the AI industry's dependence on human oversight underscores the concerns about synthetic data quality. Human experts remain essential in curating and validating datasets to prevent the propagation of errors and biases. This necessity highlights the inefficiencies and potential pitfalls if synthetic data were to be used without comprehensive quality checks.
Additionally, the potential privacy advantages offered by synthetic data may lead companies to assume a false sense of security. Although synthetic data can mitigate privacy concerns by not relying on personal information, overlooking the importance of quality and ethical data handling can paradoxically expose models to new privacy risks.
Learn to use AI like a Pro
Get the latest AI workflows to boost your productivity and business performance, delivered weekly by expert consultants. Enjoy step-by-step guides, weekly Q&A sessions, and full access to our AI workflow archive.
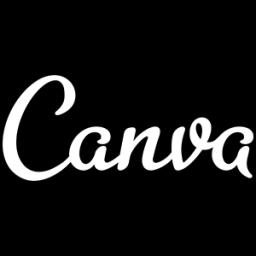
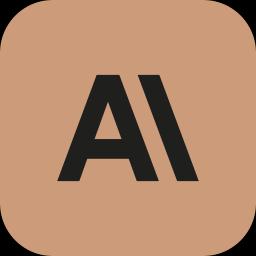
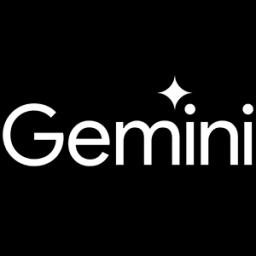
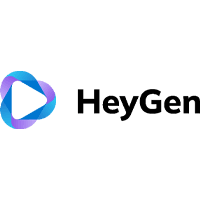
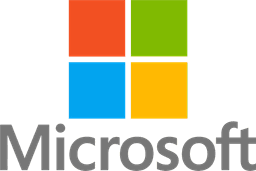
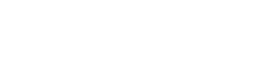
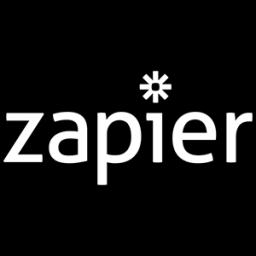
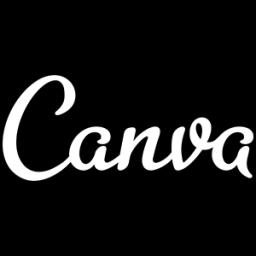
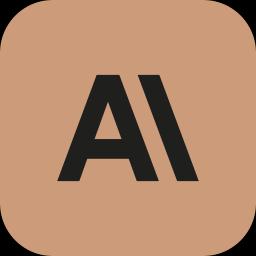
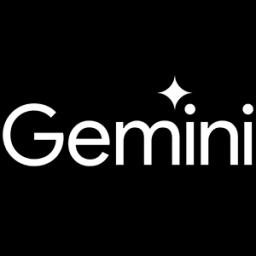
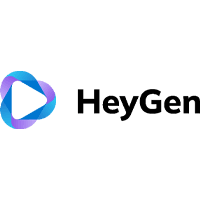
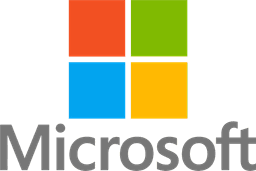
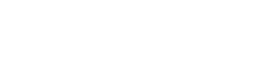
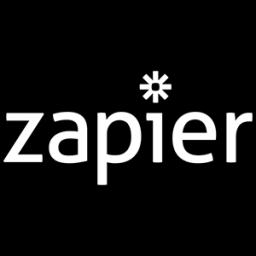
Moreover, as more companies, including tech giants like Google, Microsoft, and Nvidia, incorporate synthetic data into their AI training, there is a growing need for standardized regulations. These regulations would help ensure the ethical use and management of synthetic data, protecting against misuse and abuse that could have far-reaching societal implications.
Beyond these immediate concerns, the public's perception of synthetic data use in AI is mixed, with some viewing it as a necessary innovation to solve data scarcity issues, while others worry about its potential to perpetuate and exacerbate biases in AI systems. This divide suggests that any future strategy involving synthetic data must involve transparent practices and robust public engagement to address both technological ambitions and societal apprehensions.
Current State of Synthetic Data Usage
The use of synthetic data has become increasingly prevalent in the development of artificial intelligence (AI) models. Companies like Microsoft, Google, Nvidia, and Hugging Face are harnessing this technology to address the growing demand fueled by the high costs and ethical challenges associated with traditional data collection methods. Synthetic data is essentially artificially generated information that imitates real-world data, created by algorithms learning from existing datasets to generate new, similar data points. Despite its growing popularity, the use of synthetic data is not without challenges. The quality of synthetic data is inherently tied to the original datasets used to train the generative algorithms. If the initial data contains biases or inaccuracies, these can be amplified in the synthetic data, potentially leading to a phenomenon known as "model collapse." In such scenarios, models trained on low-quality synthetic data may become less innovative, more biased, and less effective over time. There is also an ongoing need for human oversight to ensure data quality and effective curation. Synthetic data offers numerous benefits. It is a more cost-effective, faster, and scalable solution for generating data while addressing privacy concerns that often accompany the use of real-world datasets. It can also be instrumental in mitigating biases present in training data. However, the risks associated with its use underline the need for it to supplement rather than replace real-world data entirely.
Recent initiatives and regulatory actions highlight the significance of synthetic data in AI development. In November 2024, Google announced a significant initiative to advance synthetic data generation techniques, aiming to tackle data scarcity while ensuring privacy standards. Similarly, the European Union proposed new regulations in October 2024 to govern the use of synthetic data, emphasizing quality assurance and bias mitigation. These moves reflect the strategic importance placed on synthetic data in shaping the future of AI. In the field of healthcare, synthetic data is making notable impacts. Researchers at Stanford University have demonstrated its potential in enhancing diagnosis of rare diseases through medical imaging AI models, heralding transformative possibilities in healthcare applications. Similarly, financial institutions like JPMorgan Chase are leveraging synthetic data in their fraud detection systems to improve accuracy while complying with stringent data privacy laws. These developments underscore synthetic data's utility in various sectors, fostering advancements that real-world data alone cannot easily achieve.
Industry Adoption and Success Stories
The adoption of synthetic data across various industries is rapidly gaining traction, thanks to its cost-effectiveness and scalability. Synthetic data, being artificially generated by algorithms, serves as a clever alternative to traditional data sources that often pose ethical and financial challenges. Companies like Writer, Google, Microsoft, Nvidia, and Hugging Face are at the forefront of this innovation, leveraging synthetic data to advance AI development, mitigate privacy concerns, and curb biases inherent in real-world datasets.
Success stories in synthetic data usage are emerging across different fields. For instance, in November 2024, Google announced a pivotal initiative dedicated to refining synthetic data generation techniques to address data scarcity while upholding privacy standards. This move signifies a strategic investment in the future of AI training. Moreover, the financial sector witnessed an impactful adoption of synthetic data by JPMorgan Chase in their fraud detection systems, enhancing accuracy and compliance with stringent privacy regulations. This trend is further amplified by the revolutionary progress in medical imaging, where Stanford University showcased how synthetic data substantially improved the diagnosis of rare diseases by AI models, highlighting potential life-saving applications.
Learn to use AI like a Pro
Get the latest AI workflows to boost your productivity and business performance, delivered weekly by expert consultants. Enjoy step-by-step guides, weekly Q&A sessions, and full access to our AI workflow archive.
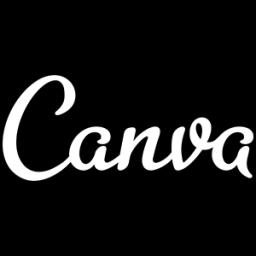
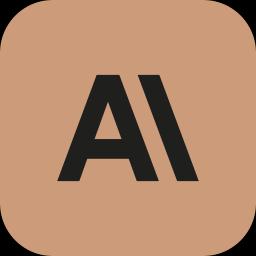
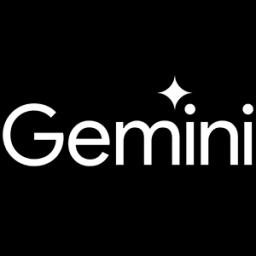
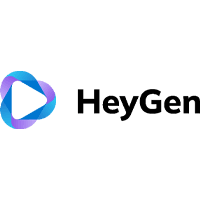
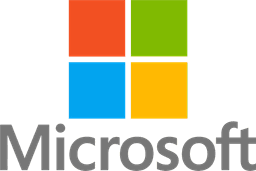
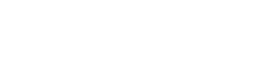
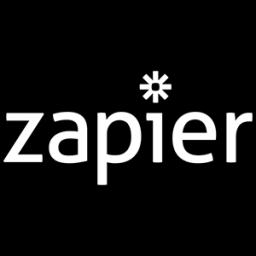
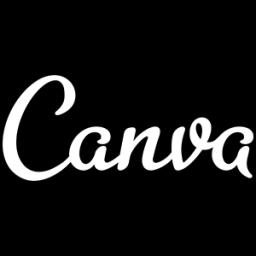
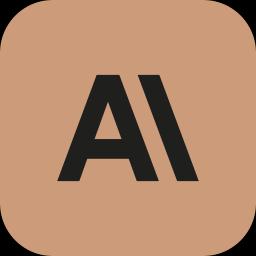
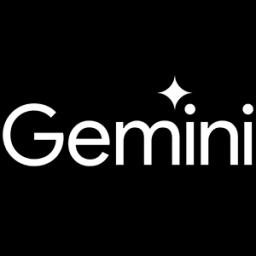
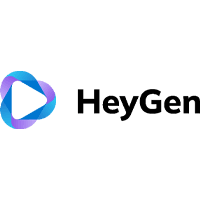
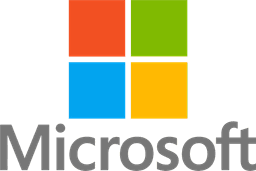
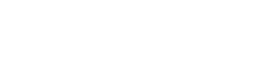
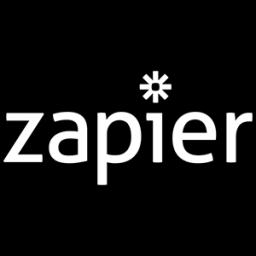
The implications of these success stories stretch beyond individual sectors. The global synthetic data market is projected to soar to $3.5 billion by 2030, indicating a robust economic impact. This growth is expected to democratize AI development, fostering innovation among smaller companies and startups. Additionally, synthetic data’s ability to protect privacy by minimizing reliance on personal information paves the way for social advancements, particularly in sensitive fields like healthcare. Politically, new regulations such as the EU Synthetic Data Regulation are poised to shape the landscape of AI, emphasizing the importance of quality control and bias mitigation in synthetic data usage.
Technologically, synthetic data promises to accelerate AI development, enabling breakthroughs in specialized applications and challenging scenarios. However, these benefits come with the cautionary tale of 'model collapse,' which underscores the necessity for rigorous quality assurance practices. Environmentally, synthetic data contributes to reducing the carbon footprint of AI training processes, marking a sustainable shift in AI development practices. As synthetic data continues to evolve, the challenge remains to balance its transformative potential with the ethical and quality concerns it surfaces.
Expert Opinions on Synthetic Data
The rapid advancement of AI technologies has necessitated new approaches to data acquisition, with synthetic data emerging as a prominent solution. Among industry leaders, Dr. Emily Zhao, an AI Ethics Researcher at Stanford University, emphasizes the delicate balance required between data efficiency and ethical integrity. Zhao advocates for combining synthetic with real-world data, which can effectively tackle challenges like data scarcity and privacy without compromising model reliability. "Synthetic data, while an innovative tool, requires stringent checks to avoid pitfalls such as bias amplification or model collapse," states Zhao.
Another key opinion comes from Dr. Alex Thompson, a Senior Data Scientist at IBM, who stresses the importance of data quality over quantity. According to Thompson, synthetic data must be meticulously curated to ensure it mirrors the variability of real-world scenarios. IBM's experiences underscore the necessity for comprehensive quality control and dataset curation, strategies pivotal in producing trustworthy AI models that do not merely operate on large datasets but are also representative and unbiased.
Prof. Sarah Chen from MIT contributes to the discourse by shedding light on the potential dangers of recursive data generation, which she argues could progressively lead to model deterioration. Her research reveals a preventative strategy through integrating synthetic data with real data to safeguard against model collapse, promoting stability in AI models even as they scale. This balanced methodology resonates with industry best practices, highlighting the need for holistic approaches in AI model training.
Dr. Michael Patel, leading research at Hugging Face, champions the environmental and economic benefits of synthetic data. Patel notes that, by leveraging synthetic datasets, AI development not only becomes more cost-effective but also significantly reduces its carbon footprint—an increasingly crucial consideration as technology evolves. His work with smaller language models proves that synthetic data offers a path to more sustainable AI innovations, setting a practical precedent for future endeavors in the field.
Learn to use AI like a Pro
Get the latest AI workflows to boost your productivity and business performance, delivered weekly by expert consultants. Enjoy step-by-step guides, weekly Q&A sessions, and full access to our AI workflow archive.
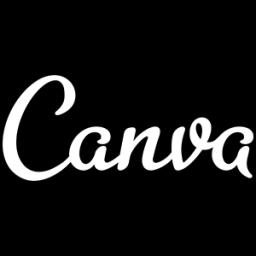
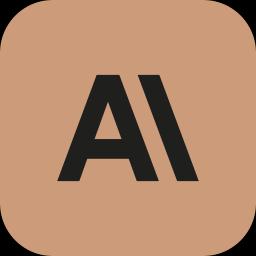
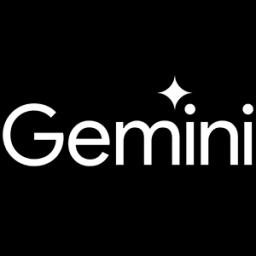
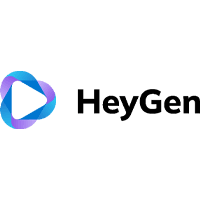
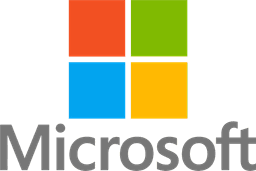
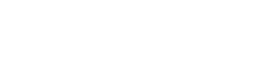
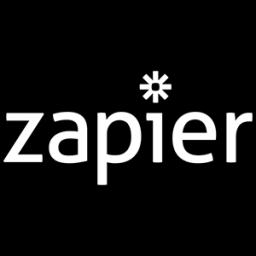
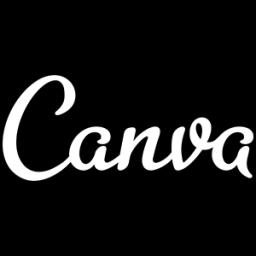
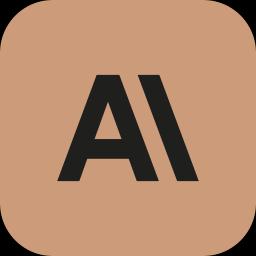
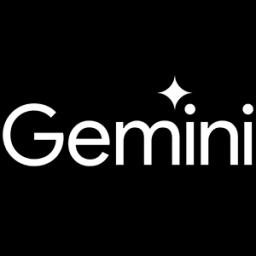
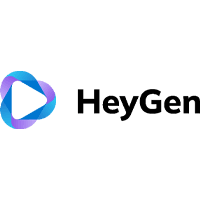
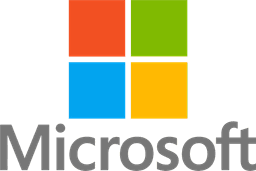
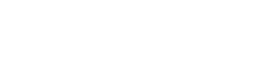
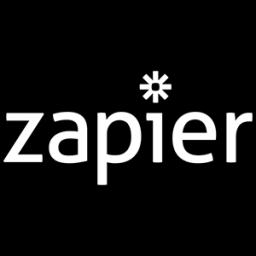
Public Reactions and Debates
Despite the apparent benefits of synthetic data, the public's reactions have been divided, sparking debates across various platforms. While some view synthetic data as a revolutionary solution to data scarcity and privacy issues, others raise alarms about its potential drawbacks.
On the positive side, many see synthetic data as a promising tool that can overcome the limitations associated with the acquisition and processing of real-world data. The ability to quickly generate large datasets that preserve privacy without the ethical quandaries involved in data sharing is a significant plus. Indeed, synthetic data allows for the creation of customized datasets tailored for specific AI model needs, something that excites both developers and end-users.
However, there are concerns regarding the quality and reliability of synthetic data. Critics argue that if synthetic data is not accurately generated, it may introduce or amplify biases within AI models. This could lead to distorted outcomes, especially in applications where bias already poses a significant risk, such as law enforcement or human resources.
Furthermore, the concept of 'model collapse'—where models trained excessively on synthetic data degrade in performance on real-world tasks—is a point of apprehension among experts and laypersons alike. This has spurred numerous discussions on the importance of ensuring high-quality synthetic datasets and the necessity of blending them with real-world data to preserve model integrity.
The lack of clear signals to differentiate AI-generated content from human-generated data is another concern. Without such distinctions, the authenticity and impact of AI outputs become harder to assess, complicating the judgment of their real-world applicability.
Nonetheless, amid these concerns is a general consensus that synthetic data, when used judiciously and alongside real-world data, can be a highly effective and beneficial tool. This sentiment is driving innovation yet urging caution as AI developers and policymakers navigate the evolving landscape of synthetic data.
Learn to use AI like a Pro
Get the latest AI workflows to boost your productivity and business performance, delivered weekly by expert consultants. Enjoy step-by-step guides, weekly Q&A sessions, and full access to our AI workflow archive.
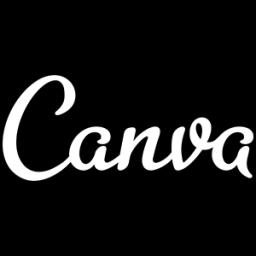
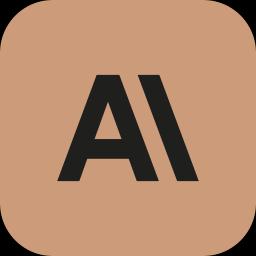
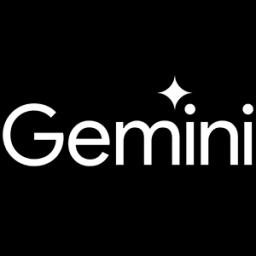
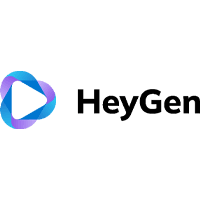
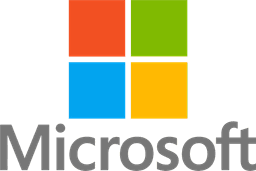
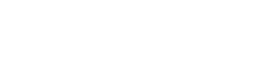
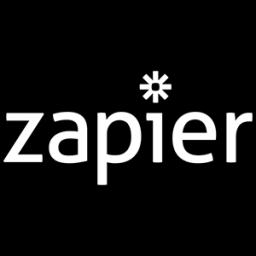
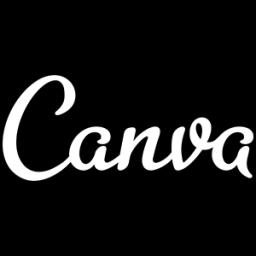
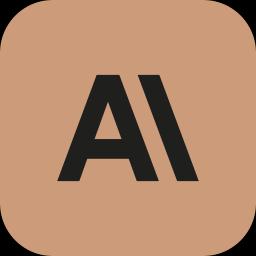
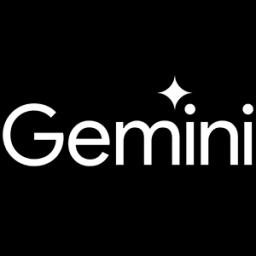
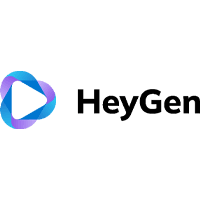
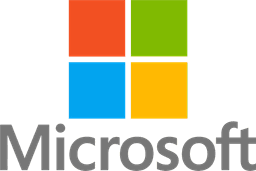
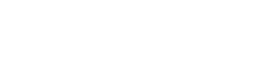
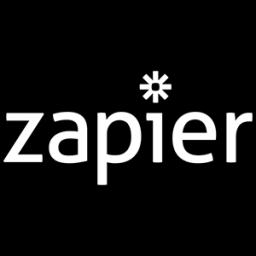
Future Implications and Trends
As synthetic data becomes a mainstay in AI model training, its future implications and trends are drawing increasing attention. The potential economic, social, political, technological, and environmental impacts are profound and multifaceted.
Economically, the synthetic data market is poised for rapid growth, possibly reaching $3.5 billion by 2030. This growth is largely driven by the cost reduction benefits synthetic data offers, making AI development more accessible to smaller companies and startups. Moreover, new job opportunities are emerging in the fields of synthetic data generation, curation, and quality control. This shift not only democratises AI development but also paves the way for new areas of employment.
Socially, synthetic data promises to enhance privacy protections by reducing reliance on personal information, which is crucial as privacy concerns remain a barrier to data utilization in AI. In the healthcare sector, synthetic data could lead to significant advancements, particularly in diagnosing rare diseases. However, alongside these benefits comes increased public scrutiny and debate over the ethics and impacts of AI-generated content on society.
Politically, the landscape is evolving with regulations like the EU Synthetic Data Regulation. Such directives are set to shape the future of AI, encouraging stringent quality and bias-checks. Countries leading in synthetic data technologies may gain geopolitical leverage, as synthetic data is increasingly discussed in the context of AI ethics and governance in political arenas.
From a technological standpoint, synthetic data can accelerate AI model development across industries. However, there is the looming risk of "model collapse"—the degradation of model performance due to poor-quality data—which underscores the necessity of managing synthetic data quality effectively. On the brighter side, there's potential for breakthroughs in AI capabilities, particularly for specialized tasks and scenarios that require large amounts of data which is either rare or costly to gather.
Environmentally, synthetic data contributes to a reduced carbon footprint in the realm of AI training, thanks to more efficient data generation processes. This eco-friendly aspect of synthetic data makes it an appealing choice for companies aiming to reduce their environmental impact.
Learn to use AI like a Pro
Get the latest AI workflows to boost your productivity and business performance, delivered weekly by expert consultants. Enjoy step-by-step guides, weekly Q&A sessions, and full access to our AI workflow archive.
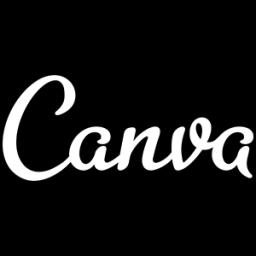
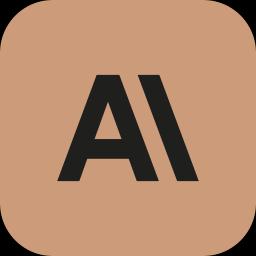
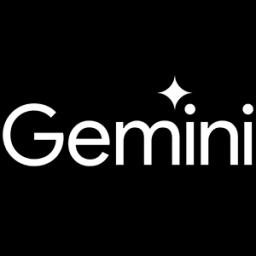
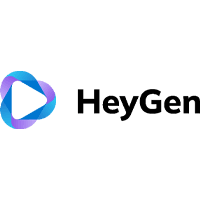
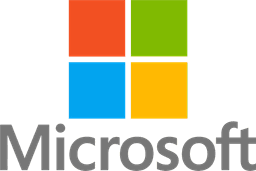
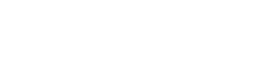
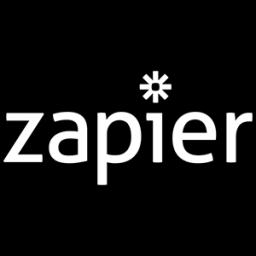
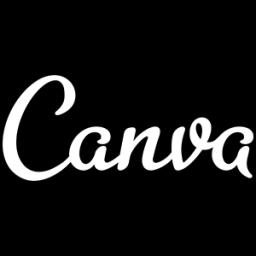
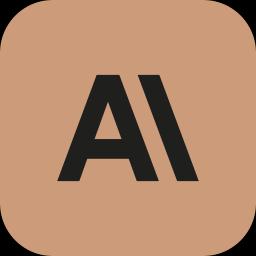
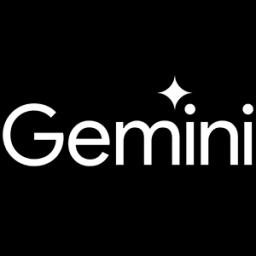
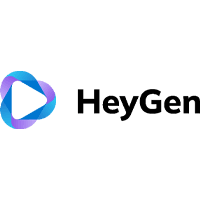
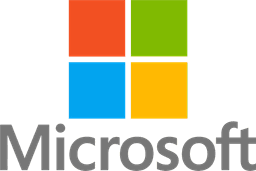
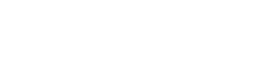
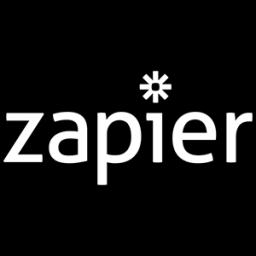
While the potential of synthetic data is vast, realizing its benefits will require a balanced approach. This involves combining synthetic and real-world data and implementing robust quality control measures to ensure data integrity and model reliability. As synthetic data continues to rise in prominence, the ability to harness its advantages while mitigating its risks will be crucial to the future of AI development.
Conclusion
The landscape of AI model training is undergoing a significant transformation with the integration of synthetic data. While synthetic data presents numerous advantages, such as cost efficiency, scalability, and enhanced privacy protection, it also comes with its own set of challenges. As companies like Microsoft and Google leverage these benefits, it becomes crucial for stakeholders to carefully navigate the potential pitfalls that accompany synthetic data usage.
The primary risks associated with synthetic data center around its dependence on the quality of original datasets. If not adequately managed, synthetic data can amplify existing biases and lead to problems such as model collapse, where AI models lose their effectiveness over time. Therefore, the role of human oversight in monitoring and curating these datasets is more important than ever to ensure AI models remain reliable and unbiased.
The rapid adoption of synthetic data in various sectors underscores its importance and potential. The medical field, for instance, is witnessing breakthroughs in rare disease diagnosis thanks to synthetic data, while the financial industry has seen improvements in fraud detection systems. Despite these advancements, concerns about the quality and ethical implications of synthetic data persist, prompting ongoing discussions and regulatory considerations worldwide.
Public opinion remains divided on the widespread use of synthetic data. While many are optimistic about its role in resolving data scarcity issues, others worry about its ability to replicate the complexity and nuance of real-world data. This dichotomy highlights the need for transparent practices and the continuous evaluation of synthetic data's impact on society.
Looking ahead, the future of synthetic data in AI development is poised with both opportunities and responsibilities. Economic projections indicate robust market growth, while social and political landscapes adapt to new norms of privacy and regulation. The key to sustainably leveraging synthetic data lies in a holistic approach that integrates real-world datasets and adheres to strict quality controls, ensuring that AI progresses without compromising its integrity.
Learn to use AI like a Pro
Get the latest AI workflows to boost your productivity and business performance, delivered weekly by expert consultants. Enjoy step-by-step guides, weekly Q&A sessions, and full access to our AI workflow archive.
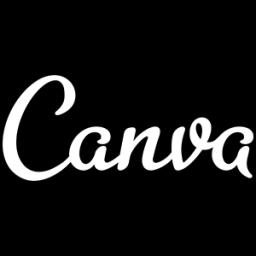
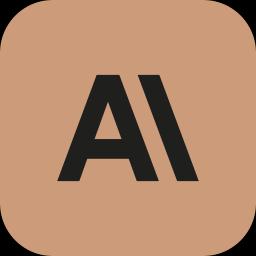
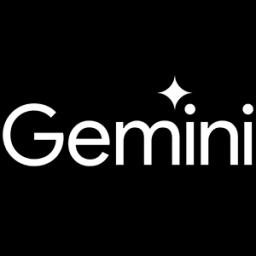
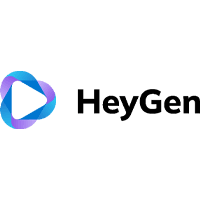
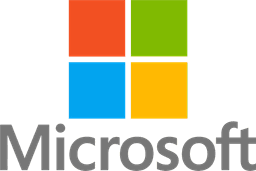
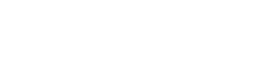
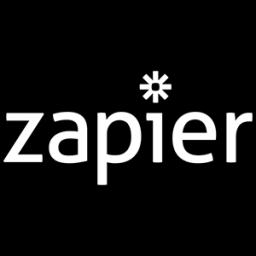
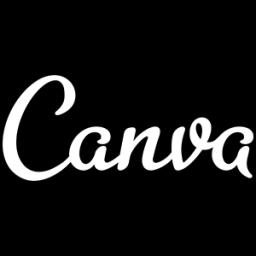
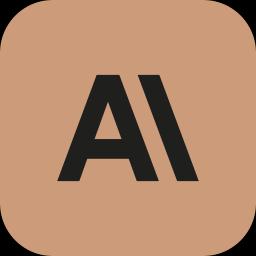
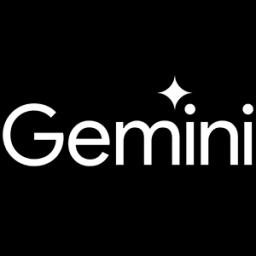
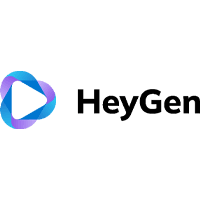
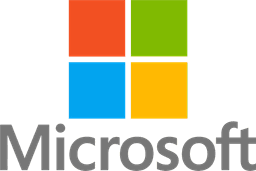
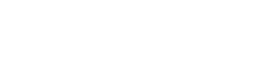
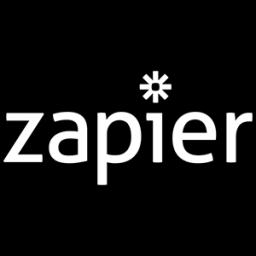