Model Theft Alert!
TPUXtract: The Clever Hack That Outs Google Edge TPU Model Secrets
Last updated:

Edited By
Mackenzie Ferguson
AI Tools Researcher & Implementation Consultant
Researchers at North Carolina State University have devised a cunning side-channel attack named TPUXtract, capable of extracting hyperparameters from AI models running on Google Edge TPUs. By harnessing electromagnetic emissions during inference, this method can reveal critical model details such as learning rate and batch size, allowing for near-perfect model reconstruction. While requiring physical access and specialized hardware, this breakthrough highlights pressing vulnerabilities due to unencrypted memory, especially on devices like the Coral Dev Board.
Introduction to TPUXtract Attack
The TPUXtract attack represents a significant advancement in side-channel attacks on AI models, specifically targeting the hyperparameters of models run on Google Edge TPUs. Researchers from North Carolina State University have pioneered this technique, which exploits electromagnetic emissions during the inference stage to reveal sensitive model components such as learning rate, batch size, and pooling size. These hyperparameter values are crucial for the reconstruction and optimization of AI models, allowing nearly perfect model recreation with an accuracy rate of 99.91%. The specificity of this attack to Google’s AI accelerator hardware highlights the critical need for enhanced security measures in these devices.
Understanding Hyperparameters and Their Significance
Hyperparameters are the predefined settings in a machine learning model that have significant influence over the training process and the efficiency of the model. They are fundamentally different from the model parameters, which are learned during training. Examples of hyperparameters include the learning rate, batch size, and pool size, among others. Their importance is underscored by the role they play in model training; they can drastically affect the convergence rate of the model as well as its accuracy, which in turn influences the overall performance of the AI system. Thus, choosing the correct hyperparameters is essential not only for model replication but also for optimization of performance, ensuring that the model can process data efficiently and produce the expected outcomes.
Learn to use AI like a Pro
Get the latest AI workflows to boost your productivity and business performance, delivered weekly by expert consultants. Enjoy step-by-step guides, weekly Q&A sessions, and full access to our AI workflow archive.
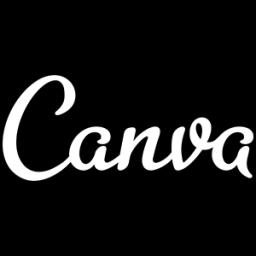
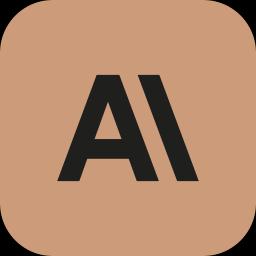
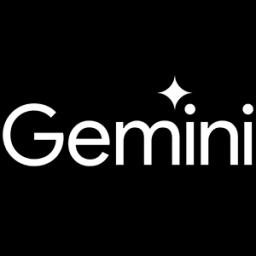
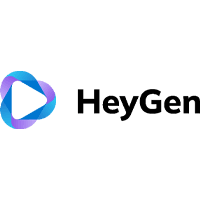
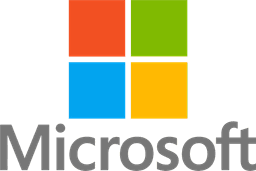
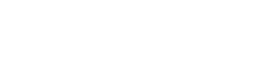
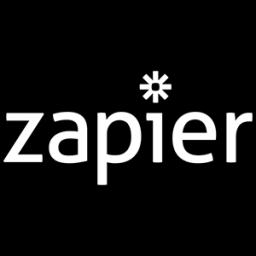
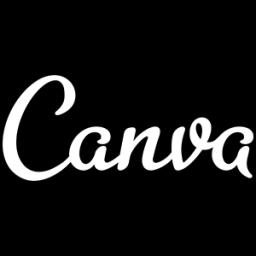
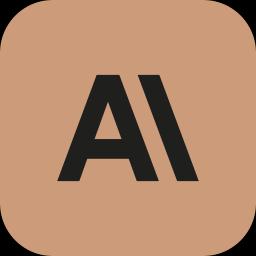
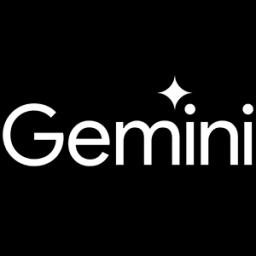
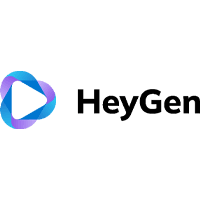
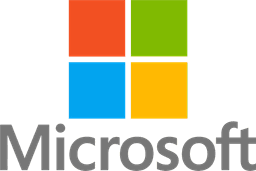
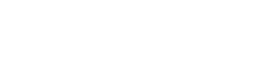
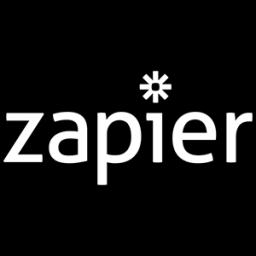
In recent technological advancements, side-channel attacks like 'TPUXtract' have highlighted vulnerabilities in AI system security, specifically focusing on hyperparameters extraction from AI models such as those run on Google Edge TPUs. Unlike traditional model-stealing attacks, TPUXtract emphasizes extracting and making use of hyperparameters via electromagnetic emissions during model inference to deduce crucial aspects like learning rate, batch size, and pool size. This innovative approach stands out as it targets Google Edge TPU exclusively, marking a significant leap in comprehensiveness and efficiency over past methods focused solely on parameter extraction. Such tactics necessitate the use of specialized hardware for electromagnetic measurements, typically requiring physical proximity to the device, enhancing potential security risks in the AI development landscape.
The implications of being able to extract hyperparameters are profound and multi-faceted, affecting economic, technological, and security aspects of AI development. From an economic standpoint, companies face the threat of having their investments in AI research compromised, enabling competitors to recreate proprietary models without incurring similar R&D costs. Furthermore, it presents a security risk as proprietary AI models become susceptible to unauthorized reproduction and distribution, potentially leading to a loss of competitive edge. This threat emphasizes the need for stronger hardware security measures to protect AI accelerators such as Edge TPUs against electromagnetic side-channel attacks.
Addressing these vulnerabilities necessitates a multi-layered approach towards security enhancement. Implementing measures such as memory encryption on devices, investing in research to develop secure hardware design, and crafting countermeasures against electromagnetic side-channel attacks are critical. Practical methods for mitigation suggested by experts include noise injection, layer randomization, and using dummy operations to obscure electromagnetic emissions of AI models, making it harder for adversaries to extract hyperparameters and other sensitive data. Additionally, reinforcing access controls and anomaly detection systems can fortify defenses against such attacks.
This underscores the pressing need for a refined balance between advancing AI technologies and ensuring robust security mechanisms are in place. The shift towards developing secure AI hardware accelerators is vital to mitigate risks associated with model theft and ensure the trust and reliability of AI technologies, especially as they become more integrated into critical and everyday applications. A comprehensive understanding of the threats posed by hyperparameter extraction and the formulation of robust counter-strategies must be prioritized to protect and advance AI systems appropriately.
Learn to use AI like a Pro
Get the latest AI workflows to boost your productivity and business performance, delivered weekly by expert consultants. Enjoy step-by-step guides, weekly Q&A sessions, and full access to our AI workflow archive.
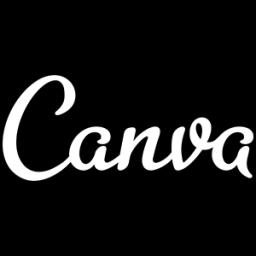
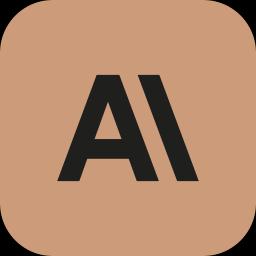
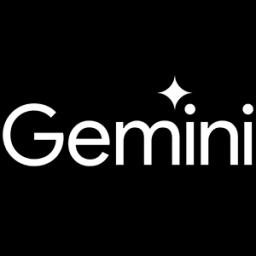
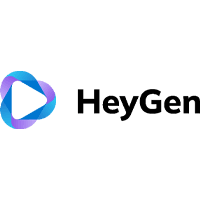
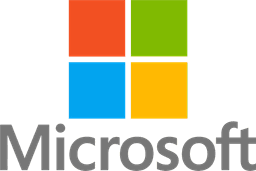
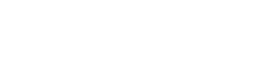
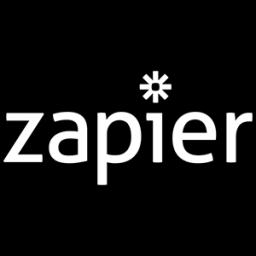
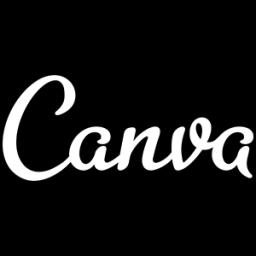
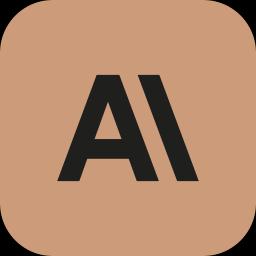
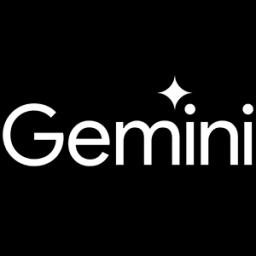
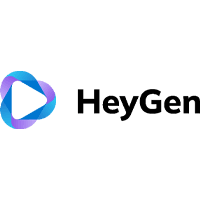
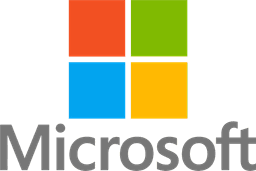
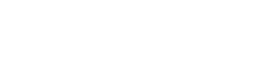
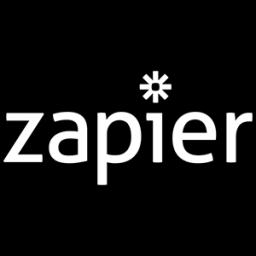
Comparison of TPUXtract with Prior Model-Stealing Attacks
The TPUXtract attack represents a significant advancement over previous model-stealing attacks, highlighting a new dimension in the threat landscape for AI models. Unlike earlier approaches that primarily focused on extracting model parameters, TPUXtract targets hyperparameters, revealing crucial insights into the AI model's functioning. This breakthrough is pivotal as hyperparameters, such as learning rate, batch size, and pool size, play a fundamental role in determining the efficiency and accuracy of AI models. By capturing these details, TPUXtract allows for an almost complete replication of the target model, achieving 99.91% accuracy in reconstruction. This level of precision is unprecedented and underscores the enhanced capabilities of TPUXtract in model theft.
Prior model-stealing attacks typically relied on black-box approaches or direct access to model outputs to infer model parameters. Those methods were either too generic, limiting the fidelity of the copied models, or required substantial computational resources and time. TPUXtract, on the other hand, employs electromagnetic emission analysis during model inference on Google Edge TPUs. This novel use of side-channel analysis not only enables a detailed extraction of hyperparameters but does so with remarkable efficiency, albeit with the necessity for close physical proximity to the device and specialized hardware to measure EM emissions. The technical sophistication and resource requirement for executing TPUXtract make it attractive to adversaries with ample resources, diverging from the historical trend of mass-market model-stealing attempts.
Another key distinction of TPUXtract is its specific focus on Google Edge TPUs, which have not been the target of such specialized attacks before. The vulnerability stems partly from the lack of memory encryption on these accelerators, particularly within the Coral Dev Board. In comparison, most prior attacks concentrated on cloud-based or general-purpose hardware accelerators, where security measures differ significantly. This specificity not only underlines the novel angle of the attack but also signals an urgent need for hardware-focused security solutions in AI device manufacturing. By highlighting these vulnerabilities in Google's edge solutions, TPUXtract paves the way for a reevaluation of security protocols across similar AI deployment frameworks.
Implications of Vulnerabilities in AI Models
The discovery of vulnerabilities in AI models, notably the TPUXtract side-channel attack, underscores a growing concern among developers and companies investing in AI technology. This attack exemplifies the significant risk of proprietary model theft, threatening the value and competitive edge that these models represent. As the capacity to extract hyperparameters with near-flawless accuracy becomes a reality, companies are faced with the daunting challenge of protecting intellectual property while ensuring their developments are not replicated without consent. In the competitive landscape of AI development, the implications of such vulnerabilities extend beyond financial loss to affect reputational standing and long-term strategic planning.
The TPUXtract attack particularly highlights the vulnerabilities inherent in certain AI hardware, such as the Google Edge TPU, where electromagnetic emissions can be exploited to extract critical hyperparameters. This has raised alarms about the security protocols in place to protect against unauthorized access and extraction. The fact that these attacks require comparatively low-complexity techniques points to an urgent need for enhanced security measures within AI architectures, especially those deployed in accessible environments or on consumer devices. The reality of such vulnerabilities demands immediate attention towards developing robust countermeasures to safeguard these advanced technologies.
Moreover, the attack's demonstration of extracting detailed model parameters exposes the broader implications for both developers and users of AI systems. Unauthorized model reconstruction poses severe risks, including the potential for widespread distribution of imitation models that could undermine original innovations. AI developers must grapple with the need to secure their models against increasingly sophisticated adversaries, which in turn could influence business models, making security a cornerstone of product development and launch strategies.
Learn to use AI like a Pro
Get the latest AI workflows to boost your productivity and business performance, delivered weekly by expert consultants. Enjoy step-by-step guides, weekly Q&A sessions, and full access to our AI workflow archive.
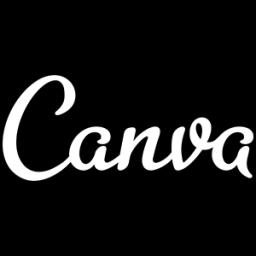
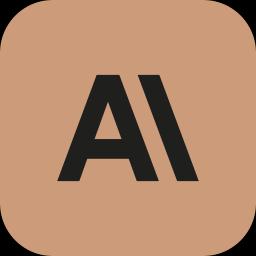
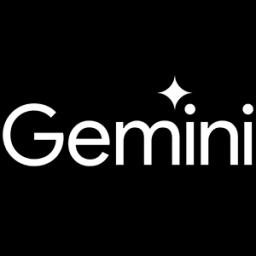
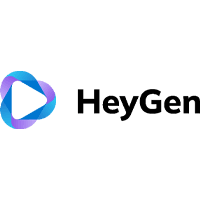
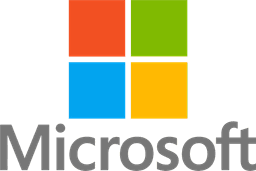
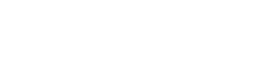
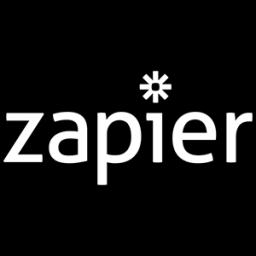
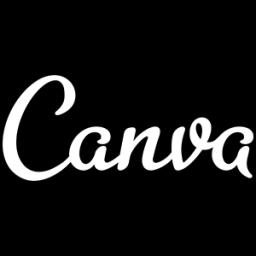
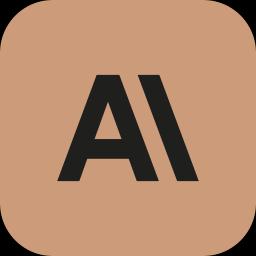
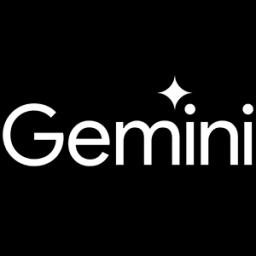
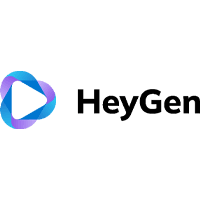
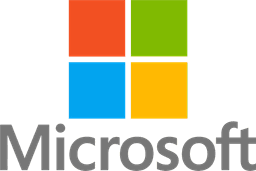
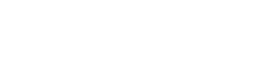
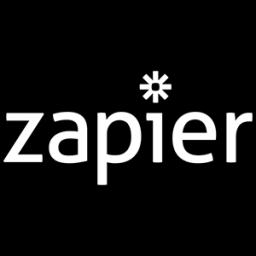
Beyond the technical and economic ramifications, this vulnerability also poses significant societal and ethical questions around data privacy and AI governance. As these models become easier to replicate and modify, there is a risk that sensitive training data—including personal information used during model training—could be exposed. This elevates concerns about trust and transparency in AI technologies, prompting calls for stricter regulations and protective measures to mitigate privacy risks. In parallel, the potential for democratization of AI through unauthorized replication could spur wider accessibility and adoption, reshaping how AI capabilities are leveraged across various sectors.
As the implications of vulnerabilities like those exploited in the TPUXtract attack continue to unfold, they inevitably drive the evolution of legal, political, and technological landscapes. Legal frameworks may need adaptation to address the unique challenges of AI model theft and protection. Politically, the risk of such exploits may influence international relations, pushing nations to view advanced AI models as strategic assets. Technologically, this could herald a new wave of innovation focused on enhancing model resilience against extraction attacks and developing inherently secure AI systems. These developments underscore the pressing need for a collaborative approach to safeguard AI advancements for both current and future applications.
Mitigation Strategies for AI Hardware Vulnerabilities
The paper delves into various strategies that can be employed to mitigate the vulnerabilities of AI hardware, particularly in response to emerging threats such as the TPUXtract attack. The first and foremost mitigation strategy is the implementation of memory encryption, especially on devices like Google's Coral Dev Board, which currently lacks this crucial security feature. Memory encryption can prevent unauthorized access to sensitive data stored in the device, acting as a barrier against data extraction through side-channel attacks.
A comprehensive approach to mitigating AI hardware vulnerabilities involves both hardware and software solutions. On the hardware side, exploring new secure hardware designs is essential. These designs should aim to minimize the chances of electromagnetic emissions during inference, which attackers exploit to extract hyperparameters. From a software perspective, implementing robust encryption for data in use and data at rest can greatly enhance security. Furthermore, regularly updating firmware with security patches can close existing vulnerabilities and protect against newly discovered threats.
To counteract electromagnetic side-channel attacks such as TPUXtract, specialized techniques must be employed. Noise injection is one strategy that involves adding random variations to the electromagnetic emissions generated during AI model processing. This technique can obfuscate the signals that attackers rely on for information extraction. Another method is the use of dummy operations and layer randomization to change the execution pattern of AI models, making it more difficult for attackers to deduce meaningful data. These approaches can significantly reduce the risk of successful side-channel attacks by complicating the data extraction process for would-be attackers.
There's a pressing need for ongoing research into developing countermeasures tailored specifically for AI hardware. This includes not only defending against current side-channel attacks but also anticipating future threats as AI technology evolves. Collaboration between academia, industry, and government entities can drive innovation in this field, leading to the creation of more resilient AI systems. With potential vulnerabilities in hardware being an avenue for significant financial and intellectual property loss, investing in advanced security measures is becoming increasingly crucial for AI developers and hardware manufacturers.
Learn to use AI like a Pro
Get the latest AI workflows to boost your productivity and business performance, delivered weekly by expert consultants. Enjoy step-by-step guides, weekly Q&A sessions, and full access to our AI workflow archive.
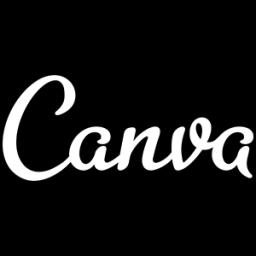
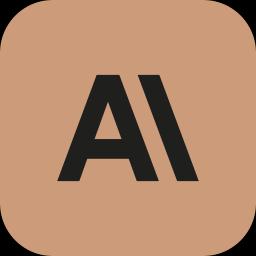
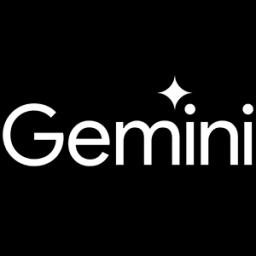
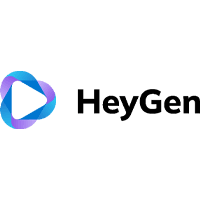
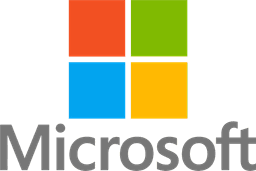
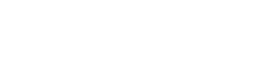
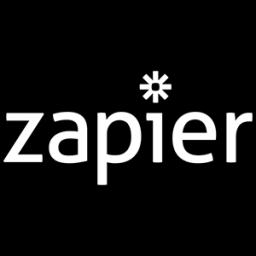
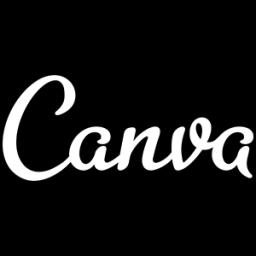
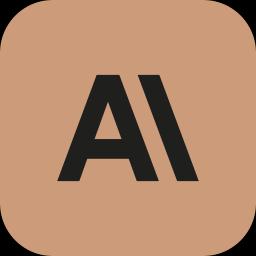
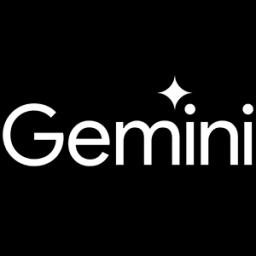
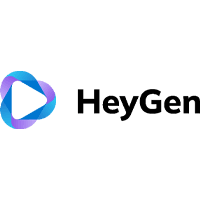
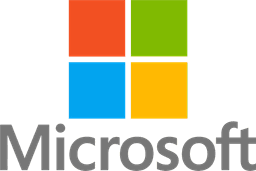
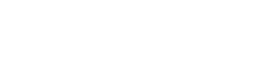
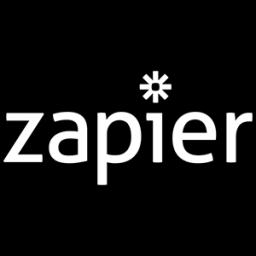
In conclusion, the proactive development and implementation of comprehensive security strategies is vital for safeguarding AI hardware against emergent vulnerabilities like TPUXtract. Such measures not only protect intellectual assets and maintain competitive advantages but also bolster trust in AI technologies by ensuring security and privacy for end-users. As AI models continue to integrate into various aspect of life and industry, the imperative for robust hardware security solutions will only grow more urgent.
Overview of Google Edge TPU and Its Applications
Google Edge TPU is a specialized hardware accelerator designed to facilitate machine learning processes on edge devices, such as smartphones and IoT devices. Specifically, the Edge TPU enables efficient execution of neural networks, thus enhancing the performance of machine learning applications directly on the device without necessitating data transfer to cloud servers. This capability is crucial for applications requiring low latency and privacy, such as real-time image recognition or personal voice assistants.
The TPUXtract attack developed by researchers at North Carolina State University spotlights a significant vulnerability within the Edge TPU infrastructure. By exploiting electromagnetic emissions during the inference process, attackers can extract crucial hyperparameters from AI models, such as learning rate, batch size, and pool size. Although this approach requires physical access to the hardware and specialized equipment, the implications of near-perfect model reconstruction are profound, posing risks to intellectual property and competitive advantage in AI development.
Despite the sophistication of the TPUXtract method, the attack underscores broader concerns about hardware security in AI accelerators like Google's Coral Dev Board. The absence of memory encryption, in particular, is a key weakness exploited by this technique. Consequently, this event has sparked discussions about improving the security protocols on edge devices to safeguard AI models against unauthorized access and replication.
The implications of such vulnerabilities extend beyond just the technical realm, affecting economic, political, and social aspects. Companies may be forced to redirect significant resources toward enhancing AI security, potentially stalling innovation. Moreover, the ease of model theft might drive shifts in how AI capabilities are commercialized, moving towards cloud-based services rather than device-embedded solutions, thereby impacting business models and strategies.
In response to these challenges, experts advocate for a multi-faceted approach to enhance the resistance of AI systems to side-channel attacks like TPUXtract. Recommendations include implementing memory encryption, noise injection, and anomaly detection, as well as pursuing advancements in hardware security to outpace potential adversaries. This calls for concerted efforts in research and development to establish robust defenses in this ever-evolving domain.
Learn to use AI like a Pro
Get the latest AI workflows to boost your productivity and business performance, delivered weekly by expert consultants. Enjoy step-by-step guides, weekly Q&A sessions, and full access to our AI workflow archive.
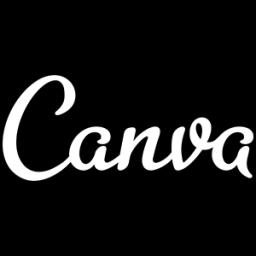
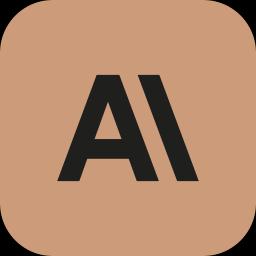
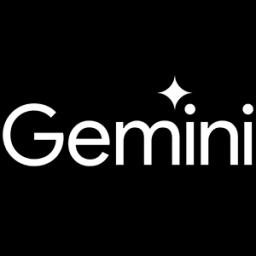
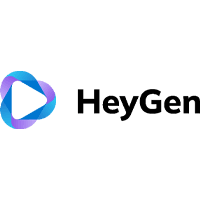
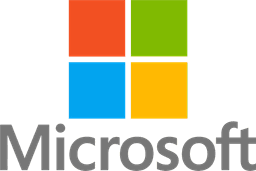
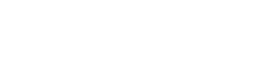
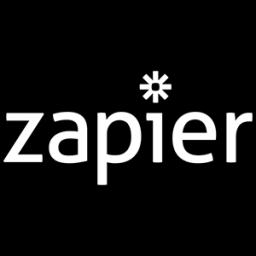
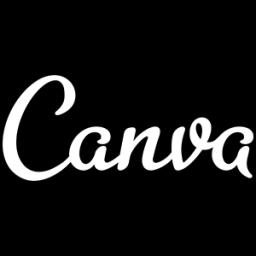
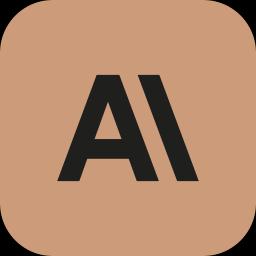
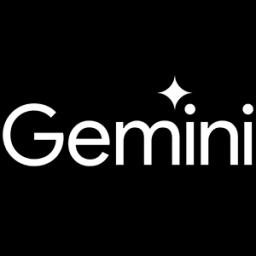
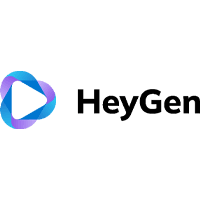
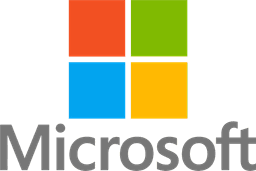
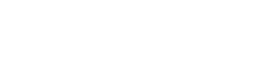
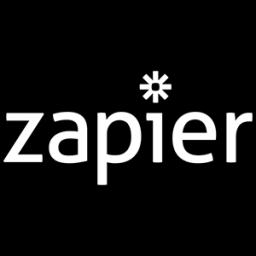
Total Duration and Complexity of TPUXtract Attack
The TPUXtract attack on Google's Edge TPUs represents a significant step in the evolution of side-channel attacks on AI hardware. The complexity and duration of the attack are key considerations that highlight the vulnerability of AI models to such sophisticated methods. At its core, TPUXtract utilizes electromagnetic emissions captured during the inference phase to deduce critical model details such as hyperparameters, including learning rate, batch size, and pool size. These hyperparameters are crucial for replicating AI models and optimizing their performance, making the extraction particularly valuable and dangerous.
The process of attack is demanding, requiring both physical access to the target device and specialized hardware capable of measuring electromagnetic emissions. On average, it takes approximately three hours to extract data per layer of a neural network, with total attack duration heavily dependent on the model's complexity. Researchers tested models ranging from 28 to 242 layers, indicating the attack could span over several days for more complex architectures.
One reason for the effectiveness of TPUXtract is the lack of robust security measures in Google's Coral Dev Boards, where unencrypted memory usage allows for easier access to critical information. This lack of encryption is part of what facilitates TPUXtract’s remarkable 99.91% accuracy in model reconstruction. Given these factors, the attack serves as a stark reminder of the need for improved hardware security measures, not only in Google’s products but across the AI hardware industry.
Historical Context: Related Events in AI Model Security
The development of TPUXtract by researchers signifies a new era in AI model security concerns, where side-channel attacks can effectively compromise the integrity and privacy of AI models. This side-channel attack, specifically on Google Edge TPUs, not only exposes vulnerabilities in AI hardware but also highlights how electromagnetic emissions can be exploited to extract hyperparameters - crucial components that dictate a model's learning capability. This vulnerability reflects the intersection of hardware limitations and advanced model theft techniques, emphasizing the need to revisit hardware design and security protocols.
Historically, AI models have faced various threats, but TPUXtract represents a significant escalation in how detailed and efficient such attacks can become. In parallel, other notable events like the model extraction attack on OpenAI's GPT-4 or the DRAM-based side-channel attack on GPUs demonstrate a persistent threat landscape, where the security of AI systems is continuously tested. These events collectively stress the urgent need for improved hardware and software defenses to protect intellectual property and preserve the integrity of AI innovations in an increasingly hostile cyber environment.
Expert Analysis and Opinions on the TPUXtract Attack
The TPUXtract attack on Google Edge TPUs has sparked significant discussion within the tech community regarding the security of AI models. With researchers from North Carolina State University uncovering a novel side-channel attack that extracts hyperparameters, this revelation underscores the potential risks associated with unprotected AI hardware. By using electromagnetic emissions during inference, TPUXtract can deduce critical model details such as learning rate, batch size, and pool size, boasting a remarkable accuracy rate of 99.91%. However, executing this attack poses challenges as it necessitates physical access to the device and sophisticated equipment for electromagnetic measurement, along with approximately three hours per layer to extract information. This vulnerability is exacerbated by the absence of memory encryption on devices like the Coral Dev Board, making them susceptible to such exploits.
Learn to use AI like a Pro
Get the latest AI workflows to boost your productivity and business performance, delivered weekly by expert consultants. Enjoy step-by-step guides, weekly Q&A sessions, and full access to our AI workflow archive.
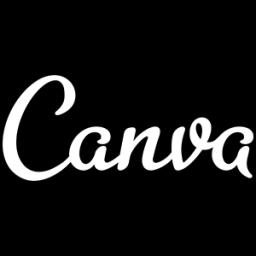
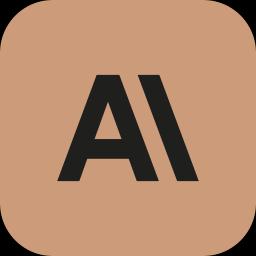
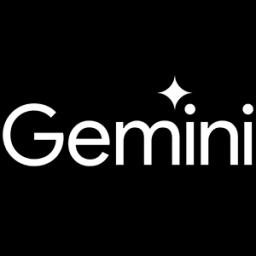
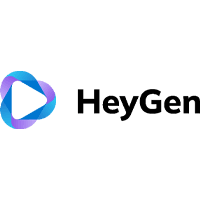
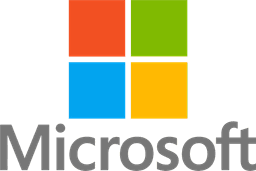
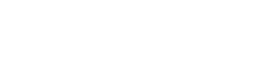
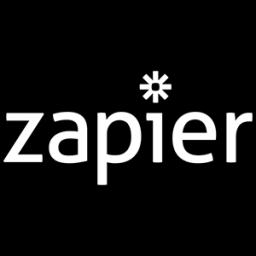
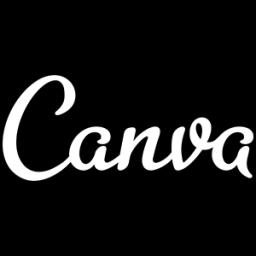
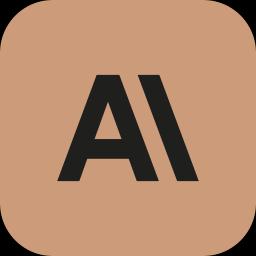
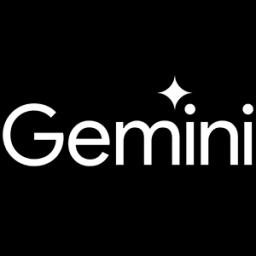
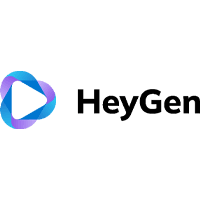
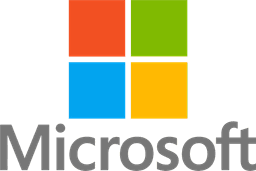
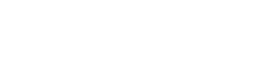
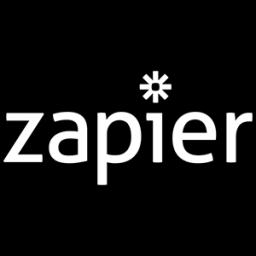
The implications of TPUXtract on AI security are profound. By facilitating the reconstruction of entire AI models, it poses a substantial threat to developers' investments and competitive edges. This vulnerability could lead to unauthorized replication and distribution of proprietary AI technologies. More broadly, it emphasizes the critical need for improved hardware security measures in AI accelerators. Several mitigation strategies have been proposed, including implementing memory encryption, conducting further research into secure device design, and developing defenses against electromagnetic side-channel attacks.
In a series of expert opinions gathered on the TPUXtract incident, Ashley Kurian emphasized the significant threats posed by the attack. She noted that with the ability to extract hyperparameters, attackers could effectively recreate an entire neural network, thus stealing both the model and the training data. While the attack requires specialized and sophisticated equipment, it remains a feasible threat for well-resourced adversaries who wish to acquire valuable AI models strategically. Kurian suggested several mitigation techniques such as noise injection, layer randomization, and employing dummy operations to blur electromagnetic signatures, thus safeguarding against such vulnerabilities.
Scott Bolen, a threat intelligence analyst, highlighted the relatively low complexity of the techniques used in the TPUXtract attack, such as Differential Power Analysis and Cache Timing Analysis, which are sufficient to compromise AI models on Google Edge TPUs. Bolen called attention to the growing importance of hardware security to keep pace with software defenses, advocating for strong access controls, anomaly detection, and exploration of encryption and differential privacy approaches as means of protection.
Public reactions to the TPUXtract attack, based on comments and discussions from platforms such as *The Register*, reveal a mix of alarm and skepticism. Many tech-savvy readers are concerned about the ease with which expensive AI models could potentially be stolen, raising alarms on the need for better intellectual property protection strategies. Meanwhile, some skeptics highlight the practical difficulties of executing such attacks, owing to the need for specialized hardware and physical access to target devices. Nonetheless, the broader discussion continues to stress vulnerabilities in edge devices like the Coral Dev Board and the urgent need for robust security interventions in AI advancements.
As the TPUXtract incident unfolds, it portends significant future implications across economic, social, and political domains. Economically, the ease of model theft may prompt AI businesses to lean towards secure API-based services, rather than embedding models within devices, potentially transforming business models and increasing costs associated with AI security. Socially, there is a risk of exposing training data, which might include personal information, if models are stolen. Politically, AI model theft could instigate stricter regulations, international tensions, and heightened national security measures surrounding AI technologies. Furthermore, this attack is likely to accelerate technological advancements, pushing for innovation in hardware security and the development of new AI architectures resistant to such vulnerabilities.
The legal landscape might also experience shifts as TPUXtract raises concerns over intellectual property battles, necessitating the establishment of new legal frameworks targeted specifically at protecting AI models. These developments point towards an evolving interplay between technology, law, and international relations, as AI continues to proliferate across sectors.
Learn to use AI like a Pro
Get the latest AI workflows to boost your productivity and business performance, delivered weekly by expert consultants. Enjoy step-by-step guides, weekly Q&A sessions, and full access to our AI workflow archive.
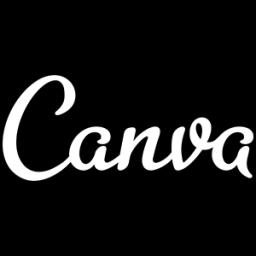
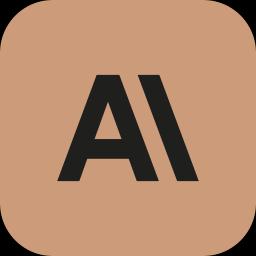
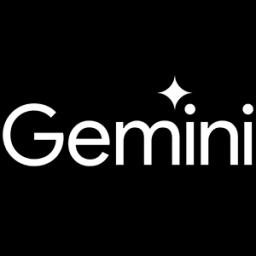
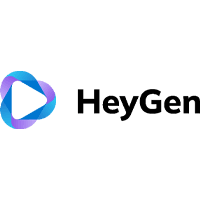
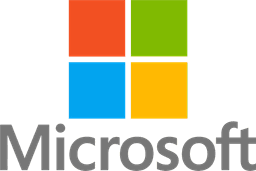
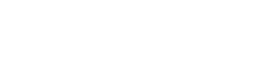
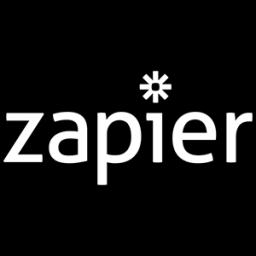
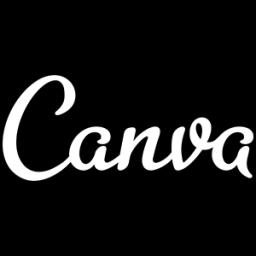
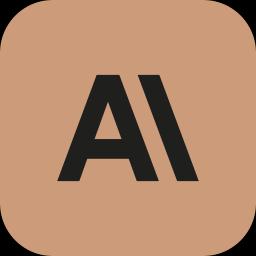
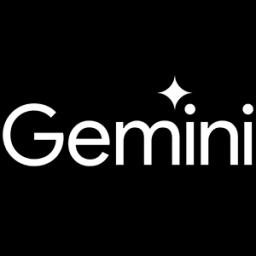
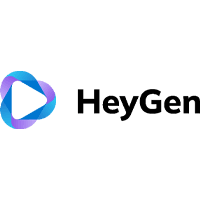
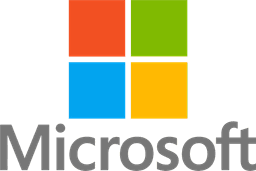
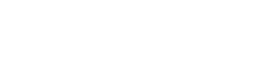
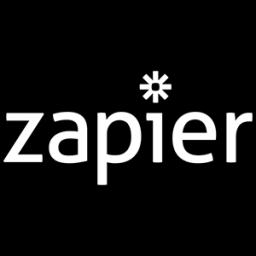
Public Reactions to TPUXtract Vulnerability Discovery
The discovery of the TPUXtract vulnerability has generated a range of public reactions, particularly among tech enthusiasts and cybersecurity experts. Comment sections on platforms like The Register reveal a mix of alarm, skepticism, and concern. Some individuals express alarm over the ease with which expensive AI models can be replicated and the potential for intellectual property theft, which threatens the investments made by AI developers.
There is also skepticism about the practicality of the attack, with discussions focusing on the challenges of obtaining the specialized hardware necessary for electromagnetic measurements and the physical access required to target devices. This skepticism suggests that while the attack is theoretically possible, its execution in real-world scenarios may be limited to well-resourced adversaries.
The discovery of the vulnerability in edge devices like the Coral Dev Board has further fueled security concerns. There is an increasing awareness of the need for enhanced AI model security on similar hardware, as many worry about the implications for AI systems that may not have sufficient hardware defenses against such attacks.
Furthermore, the public discourse includes broader concerns regarding intellectual property protection. As AI models represent significant assets, the discussions highlight the challenges companies face in safeguarding them against unauthorized reproduction and theft.
Lastly, the cybersecurity landscape may need to adapt to new potential threats as outlined by some commenters. The vulnerability underscores a need for sophisticated defenses and mitigation strategies to protect against evolving cyberattacks targeting AI systems.
Future Economic and Social Implications of the Attack
The TPUXtract attack represents a significant challenge and opportunity for both economic and social sectors. Economically, the ability to extract high-value AI model hyperparameters with relative ease could induce a shift in how businesses protect their AI assets. Companies may need to allocate substantial resources to enhance the security frameworks surrounding their machine learning models to safeguard against such vulnerabilities. With rising costs in research and development aimed at improving security, smaller firms might struggle to compete, potentially consolidating AI development in the hands of a few large corporations with robust security postures.
Learn to use AI like a Pro
Get the latest AI workflows to boost your productivity and business performance, delivered weekly by expert consultants. Enjoy step-by-step guides, weekly Q&A sessions, and full access to our AI workflow archive.
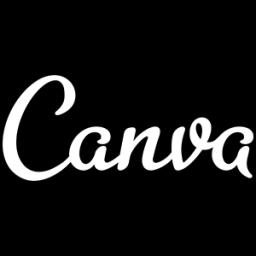
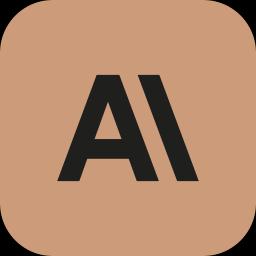
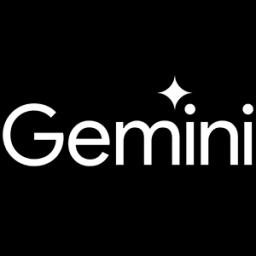
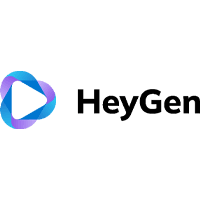
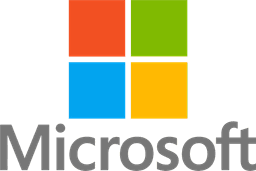
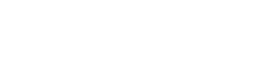
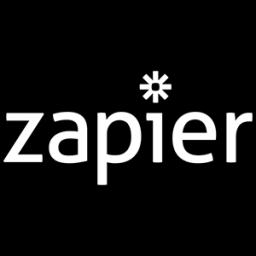
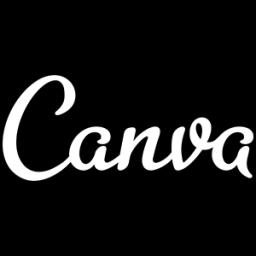
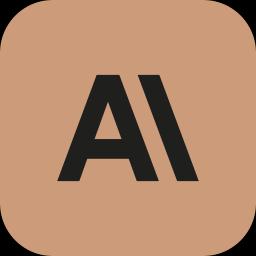
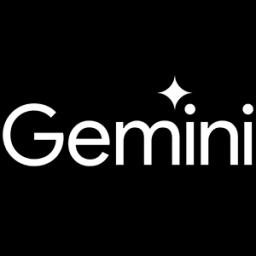
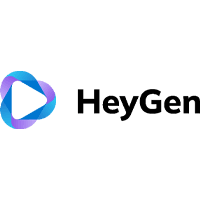
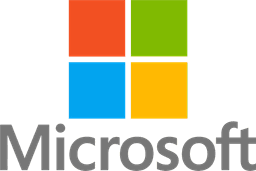
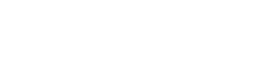
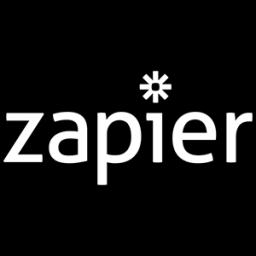
Moreover, this vulnerability might drive a transformation in AI business models. As direct hardware-based AI solutions become more susceptible to attacks, companies might prefer to transition to API-based models, preserving their intellectual property behind secure server environments and reducing the risk of theft. This could lead to a surge in cloud AI service demand, reshaping the industry landscape over time. Additionally, the cybersecurity insurance sector might experience growth as businesses seek specialized coverage to mitigate the financial impact of AI vulnerabilities.
Socially, the repercussions of TPUXtract's implications could stretch far beyond corporate walls. There is a potential effect on privacy, as stolen models may expose sensitive training data embedded within those models, some of which might involve personal or proprietary information. Conversely, the unauthorized access to AI capabilities could lead to democratization in technology deployment, making advanced AI solutions more accessible to wider audiences, albeit through illicit means.
From a political standpoint, the international landscape could become more complex as countries rigorously protect their AI innovations as strategic national assets. The regulatory environment is likely to become more stringent, as governments strive to protect AI-related intellectual property from international theft, potentially leading to the development of stricter guidelines and sanctions.
Technological advancements in AI safety and security are expected to accelerate in response to these challenges. Hardware developers might focus on creating more resilient AI systems designed to thwart such model-stealing attacks, incorporating built-in security measures that go beyond software-level defenses. Concurrently, legal systems around the world may catch up to these technological strides, evolving new intellectual property laws specifically addressing and protecting AI models.
Regulatory, Political, and Technological Advancements Post-TPUXtract
The discovery of TPUXtract, an advanced side-channel attack targeting Google Edge TPUs, marks a pivotal moment in the intersection of AI technology and security. This attack, developed by researchers at North Carolina State University, is capable of extracting hyperparameters from AI models by analyzing electromagnetic emissions during inference. These hyperparameters, crucial for AI model replication and optimization, include settings like learning rate, batch size, and pool size. The vulnerability it exposes is compounded by the Coral Dev Board's lack of memory encryption, emphasizing the need for robust security protocols in AI hardware. As TPUXtract necessitates physical access and specialized equipment, it underlines the importance of securing not just digital but also physical and environmental aspects of AI technology. The attack’s efficiency, with a potential 99.91% model reconstruction accuracy, represents both a technical achievement and a significant security concern. This event underscores the urgent need for advancements in hardware security, memory encryption, and the development of countermeasures against electromagnetic side-channel attacks.
Legal Implications and Potential Battles over AI Model Theft
The emergence of attacks like TPUXtract raises critical questions about the legal landscape surrounding AI model theft. As AI models become pivotal intellectual property assets for tech companies, the risk of unauthorized extraction and replication poses severe challenges for maintaining competitive advantages. Legal implications of such vulnerabilities are vast and complex, highlighting a pressing need for clear legal frameworks that define the rights and responsibilities of AI developers and users.
Learn to use AI like a Pro
Get the latest AI workflows to boost your productivity and business performance, delivered weekly by expert consultants. Enjoy step-by-step guides, weekly Q&A sessions, and full access to our AI workflow archive.
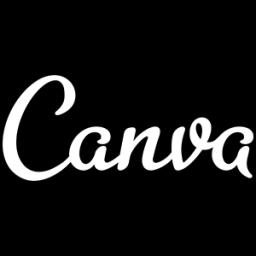
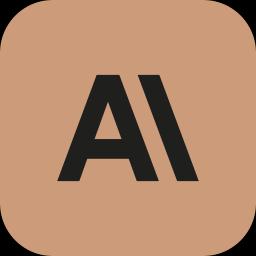
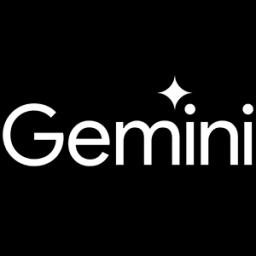
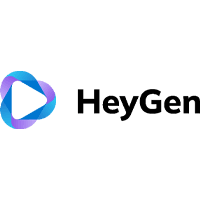
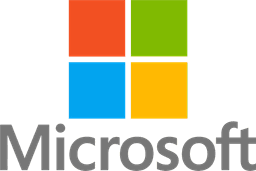
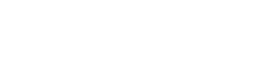
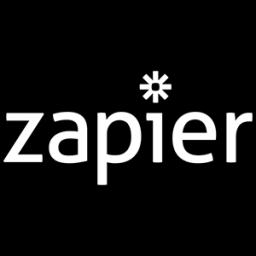
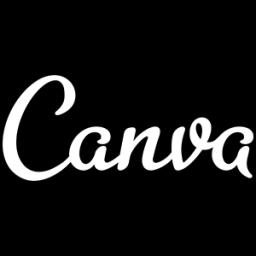
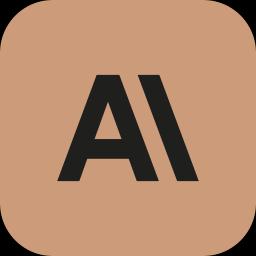
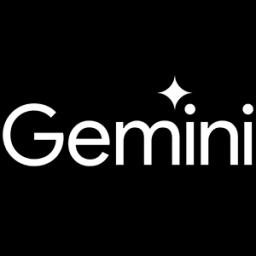
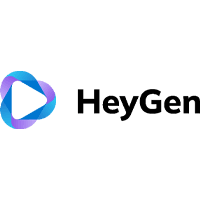
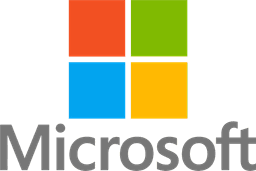
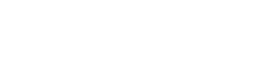
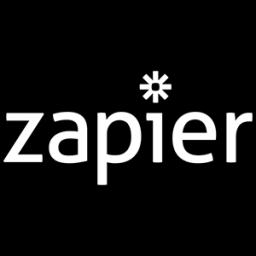
Several experts argue that existing intellectual property laws might not be fully equipped to address the nuances of AI model theft. Traditional IP protections, such as patents and copyrights, may fall short for AI models, as they often focus on the final product rather than the underlying algorithms and hyperparameters involved. This gap necessitates the development of new legal instruments tailored to the AI industry's needs.
Potential legal battles could arise as companies seek to protect their models from theft and exploitation. In the absence of specific AI model protection laws, companies might turn to trade secrets or contractual agreements to safeguard their innovations. However, these measures can be limited in preventing model extraction by sophisticated attacks like TPUXtract, especially when adversaries have access to specialized tools and techniques.
The global nature of AI development adds another layer of complexity to the legal discourse. Variations in IP laws and enforcement across countries could lead to international disputes over AI model ownership and rights. Such disputes might further necessitate international cooperation and agreements to establish harmonized regulations and protect AI intellectual property across borders.
Ultimately, addressing the legal implications of AI model theft requires a collaborative effort from policymakers, legal experts, and the tech industry. By working together, stakeholders can create a robust legal framework that not only protects AI models but also encourages innovation and fair competition in the rapidly evolving AI landscape.