Navigating AI's Plateau with Innovation
OpenAI's New Strategies to Tackle Orion's AI Growth Slowdown
Last updated:

Edited By
Mackenzie Ferguson
AI Tools Researcher & Implementation Consultant
OpenAI is taking innovative steps to combat a slowdown in AI advancements as their upcoming model, 'Orion,' shows less explosive growth compared to previous versions. The company is addressing the lack of new training data by leveraging synthetic data and optimizing post-training processes. This strategy aims to maintain competitive edge and continuous improvement in AI.
OpenAI's Response to AI Improvement Slowdown
OpenAI is responding to the reported slowdown in AI improvement by implementing a variety of strategies to ensure ongoing advancement and innovation. Despite the noticeable leap from GPT-3 to GPT-4, the expected progress with the upcoming Orion model seems less pronounced. According to the recent TechCrunch article, the core issue contributing to this slowdown is the limited availability of new training data, a challenge that affects the pace at which AI models can improve.
To confront these challenges, OpenAI has established a dedicated "foundations team" tasked with exploring new methods to sustain progress. One approach being considered involves using synthetic data as a supplementary training resource. Additionally, the team is focused on enhancing the post-training process to improve the model's performance further. These strategic adjustments are crucial for OpenAI to maintain its competitive edge and continue pushing the boundaries of AI technology.
Learn to use AI like a Pro
Get the latest AI workflows to boost your productivity and business performance, delivered weekly by expert consultants. Enjoy step-by-step guides, weekly Q&A sessions, and full access to our AI workflow archive.
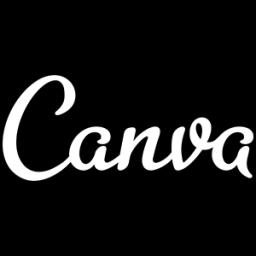
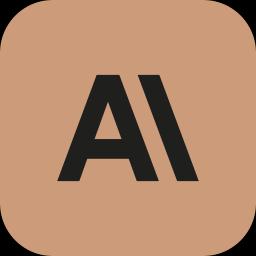
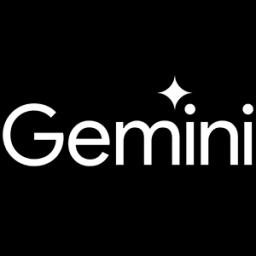
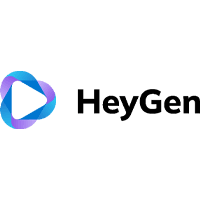
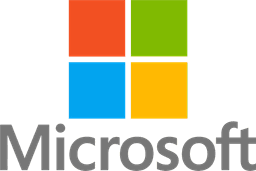
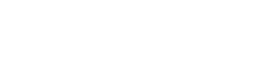
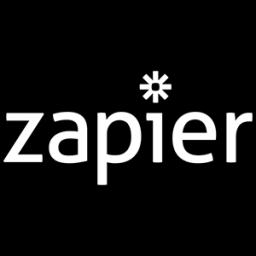
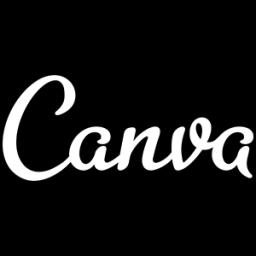
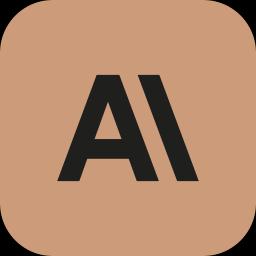
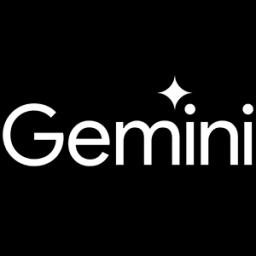
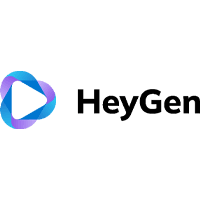
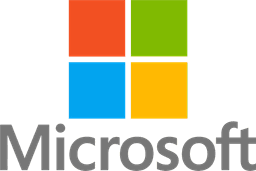
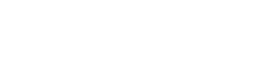
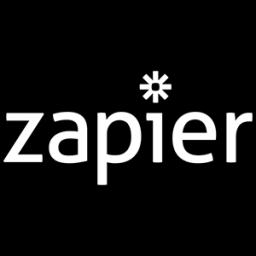
The Orion model, while demonstrating some improvements, has not matched the significant advancements seen in previous iterations, particularly in coding tasks. This situation raises some concerns regarding the value and efficiency of continuing to expand existing AI architectures. Nevertheless, OpenAI's commitment to finding innovative solutions is evident as they explore cost-effective ways to leverage their technological capabilities without solely depending on scaling current models.
The article highlights several strategies that OpenAI is considering to mitigate the data limitation issue and sustain AI improvements. These include using synthetic data and refining the post-training enhancement techniques. By adopting these measures, OpenAI aims to navigate the difficulties posed by data scarcity and remain at the forefront of AI development.
The challenges faced by OpenAI reflect broader issues within the AI industry related to data availability and the inherent limitations of existing technologies. Continued rapid improvement is vital for maintaining the momentum needed for continued technological advancement and ensuring that AI solutions can meet evolving demands. OpenAI's proactive approach to addressing these challenges demonstrates their dedication to overcoming barriers and setting the stage for future success in AI innovation.
Causes of the Slowdown in AI Advancements
The slowdown in AI advancements has drawn considerable attention as it represents a significant shift in how companies like OpenAI have traditionally achieved rapid technological growth. The primary cause identified for this stagnation is the diminishing availability of new training data. Historically, the influx of new and varied data has been a cornerstone of developing more capable AI models. However, as existing data repositories are exhaustively mined and privacy laws become stricter, obtaining fresh data becomes increasingly challenging. This limitation constrains not only the quality of training AI algorithms but also its innovation capacity, impacting fields like natural language processing where novelty in data is critical.
Learn to use AI like a Pro
Get the latest AI workflows to boost your productivity and business performance, delivered weekly by expert consultants. Enjoy step-by-step guides, weekly Q&A sessions, and full access to our AI workflow archive.
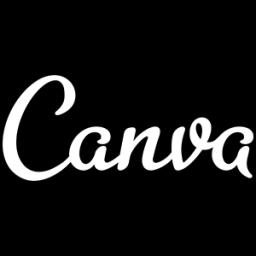
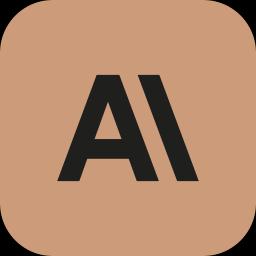
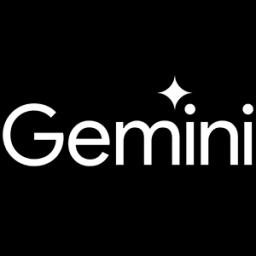
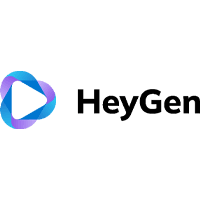
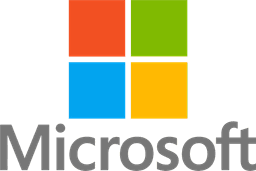
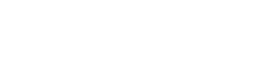
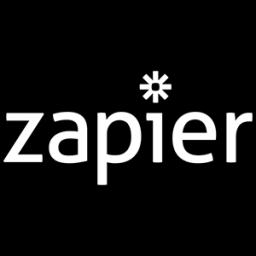
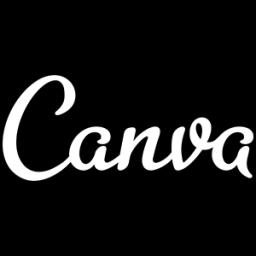
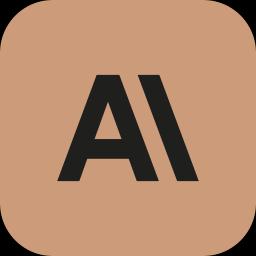
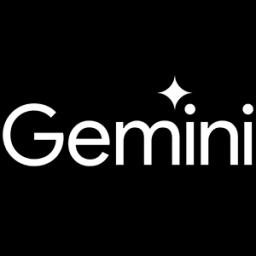
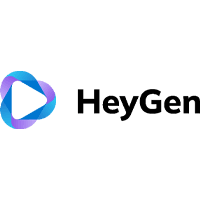
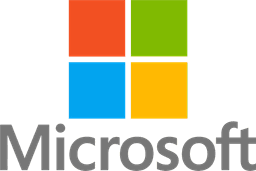
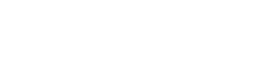
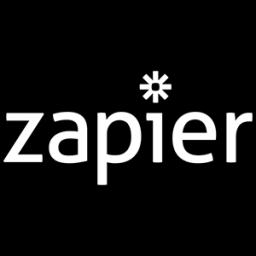
In response to these challenges, OpenAI is taking proactive measures to adapt. One significant initiative is the formation of a specialized foundations team dedicated to exploring novel strategies to overcome the current data limitations. This team focuses on creating synthetic data and enhancing the AI models' post-training processes, aiming to mitigate the impact of reducing natural data sources. These strategies are not just stopgap measures but represent a pivot towards more sustainable AI development practices, ensuring that advancements in AI technology can continue despite the inherent limitations of existing methodologies.
Comparatively, while previous AI models such as GPT-3 and GPT-4 marked substantial leaps in capabilities, the newest model, Orion, shows more modest improvements. This pattern is particularly evident in domains like coding, where the advancement rate has notably slowed. Although Orion presents enhancements in specific language-based tasks, the lack of a dramatic performance boost raises questions about the incremental benefits versus the efforts required to achieve them. This change signals a period of maturation within AI development where incremental refinements start to overshadow monumental breakthroughs.
Concerns over the slowdown are rooted in the potential competitive disadvantages they pose to companies like OpenAI, which have built their reputation on rapid advancement. The tech industry’s landscape is highly dynamic, and any perceived stagnation might affect a company’s innovation leadership position. The Orion model's modest improvements could prompt competitors to seek other avenues for AI development, potentially leveraging alternative approaches that bypass current data constraints.
OpenAI’s strategies in dealing with the slowdown include an interesting mix of technological innovation and practical adaptation. By shifting focus towards generating synthetic data and refining the domain of post-training enhancements, OpenAI is rethinking how AI can evolve in constraints. The strategic development of inference technologies highlights a nuanced understanding that computational efficiency and cost management are becoming as significant as raw improvements in AI capability. These strategies illustrate a forward-thinking adaptation, acknowledging limitations while setting new directions for future AI research.
Comparing Orion with Previous OpenAI Models
OpenAI, a leading player in the AI field, has encountered a notable slowdown in its pace of AI advancement, particularly with the development of its latest model, Orion. This model, while showcasing several enhancements, has not displayed the significant leap seen in earlier upgrades such as the transition from GPT-3 to GPT-4. This presents a stark contrast to public expectations and the usual trajectory of AI improvements.
The underlying reason for this slowdown is attributed to the diminishing availability of new training data, which poses a challenge for sustaining rapid improvements. In response, OpenAI is proactively exploring new strategies such as utilizing synthetic data and optimizing the post-training processes through a dedicated 'foundations team.' These efforts aim to adapt to the evolving landscape of AI development, ensuring continued progress despite the data constraints.
Learn to use AI like a Pro
Get the latest AI workflows to boost your productivity and business performance, delivered weekly by expert consultants. Enjoy step-by-step guides, weekly Q&A sessions, and full access to our AI workflow archive.
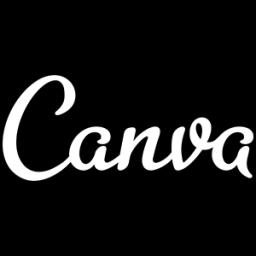
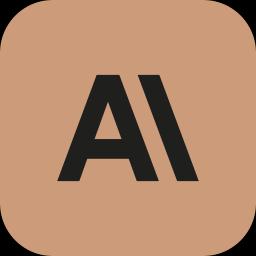
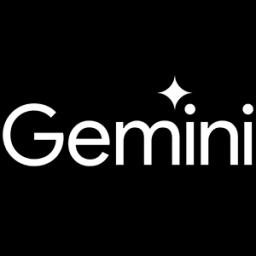
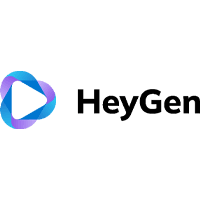
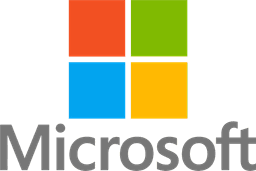
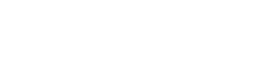
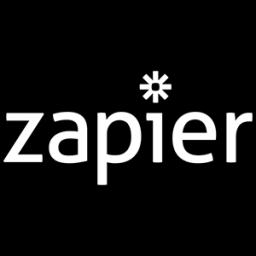
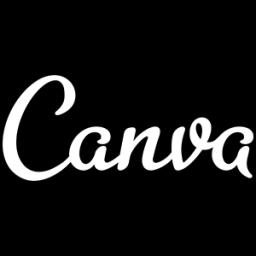
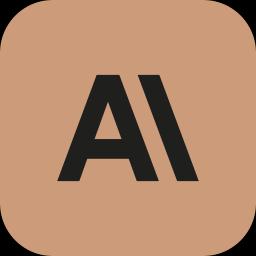
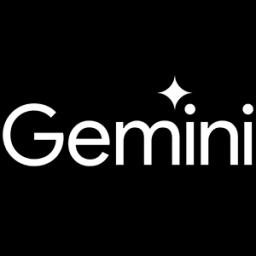
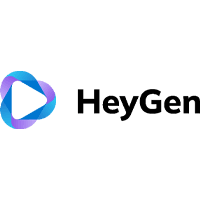
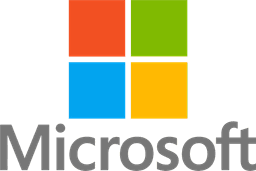
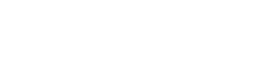
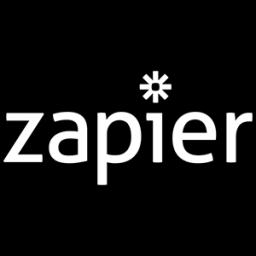
Despite Orion's advancement not being as pronounced as previous models, especially in coding, the model is considered an important step forward. This perspective, however, has sparked debate regarding the cost-effectiveness of scaling AI technologies amidst waning returns from traditional model enhancements.
The concern surrounding the slowdown is multifaceted. For OpenAI, maintaining a rapid pace of development is critical for retaining its competitive edge and driving innovation in the AI sector. The current challenges reflect broader industry trends and raise pertinent questions about the sustainability of existing AI models.
In addressing these challenges, OpenAI is adopting certain strategies aimed at overcoming current limitations. Key among these are the use of synthetic data to supplement training data shortages and refining post-training techniques, which are expected to bolster Orion's capabilities and set a precedent for future models.
The Importance of Maintaining Rapid AI Improvement
The pace of AI development has been a topic of significant interest and concern among technologists and policymakers. OpenAI's latest strategies to maintain the rapid pace of AI improvement come at a time when the industry is facing potential slowdowns due to limitations in available training data. This shift is crucial as AI continues to integrate more deeply into various sectors, influencing economies, societies, and global politics.
OpenAI's creation of a 'foundations team' exemplifies their proactive approach to AI stagnation. The team is tasked with exploring innovative strategies such as the use of synthetic data for model training and improvements in the post-training processes. These efforts are designed to overcome the current constraints on data availability, thereby sustaining the advancement of their upcoming AI model, Orion.
The Orion model's performance, while notable, highlights the challenges faced by OpenAI in achieving breakthroughs comparable to those seen with prior models like GPT-4. The transition from training to inference is gaining importance as a new dimension in AI scaling, suggesting a shift towards harnessing greater computing power for AI processing. However, this shift brings with it economic and environmental costs that need to be carefully managed.
Learn to use AI like a Pro
Get the latest AI workflows to boost your productivity and business performance, delivered weekly by expert consultants. Enjoy step-by-step guides, weekly Q&A sessions, and full access to our AI workflow archive.
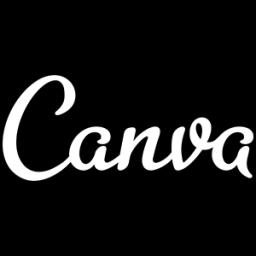
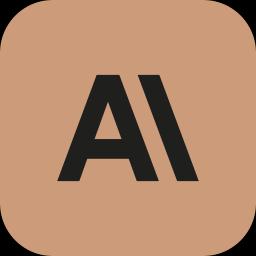
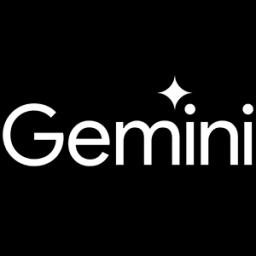
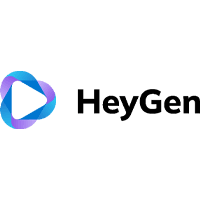
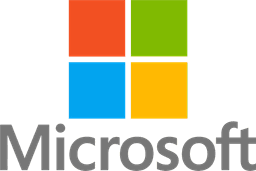
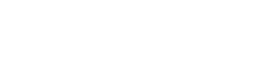
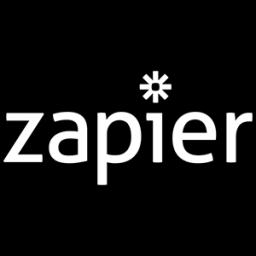
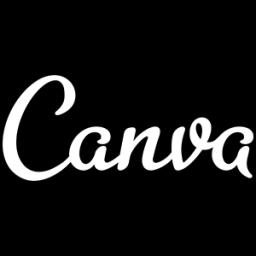
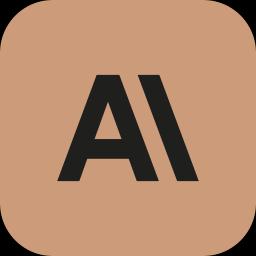
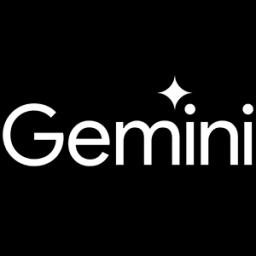
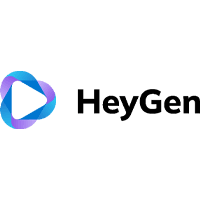
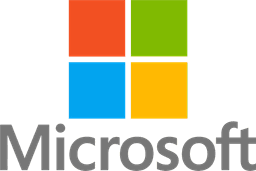
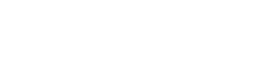
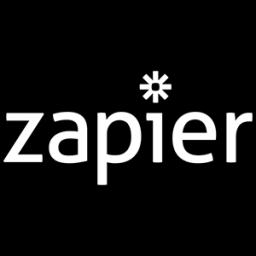
The implications of OpenAI's strategies extend beyond the technical realm. Economically, the increasing costs associated with innovative data processing methods may alter the financial dynamics of AI development. Socially, public demand for ethical and transparent AI practices continues to rise, potentially reshaping how AI is deployed and perceived worldwide. Politically, the move towards global AI regulation, as seen with initiatives like the EU's AI Act, reflects a need for tighter governance in fostering responsible AI technologies.
Public reactions to OpenAI's developments reflect a mix of skepticism and cautious optimism. While there are concerns about the slowing pace of improvement and its implications for AI credibility, there is also recognition of the importance of even incremental advancements in the field. OpenAI's focus on post-training enhancements suggests a strategic shift that could influence future AI trajectories, despite uncertainties about its immediate impact.
Strategic Approaches to Overcome Data Limitations
In response to the noticeable slowdown in AI improvement, OpenAI is strategically adapting by forming a foundational team to explore innovative solutions. One of the major steps includes the utilization of synthetic data to continue training their models despite a lack of new natural data. Harnessing synthetic data allows OpenAI to overcome one of the significant barriers in AI development – the availability of fresh, high-quality data. By simulating training environments and datasets, OpenAI hopes to fill in the gaps left by traditional data sources, ensuring that their AI models can still progress even when the pool of real-world data is depleted.
Moreover, OpenAI is looking into enhancing post-training processes. This approach is pivotal in refining AI models after their initial training phase, which could potentially lead to sustained improvements in AI capabilities. Post-training adjustments allow the company to leverage existing models by fine-tuning them to meet new challenges or improve performance in specific areas, such as language processing or coding. This strategy is indicative of OpenAI's innovative approach to AI development, as it not only focuses on initial model training but also on continual refinement to push boundaries further.
The reported performance of the new Orion model, while not as groundbreaking as previous upgrades like GPT-4, still marks a significant step forward for OpenAI in areas such as language tasks. However, the relative stagnation in coding capabilities highlights the limitations posed by traditional training methodologies and data. By acknowledging these constraints and actively seeking methods to surpass them, OpenAI demonstrates a commitment to overcoming current hurdles and continuing its trajectory of technological progress. The recognition of these limitations also sets the stage for future innovations that could reignite the pace of AI advancements.
Implications of AI Regulation and Ethical Standards
In recent years, the landscape of artificial intelligence (AI) has evolved significantly, and with this evolution comes the pressing need to establish proper regulations and ethical standards. The regulation of AI involves setting rules and guidelines that govern the use and development of AI technologies, aiming to balance innovation with public safety concerns. Ethical standards, on the other hand, focus on ensuring that AI systems operate in ways that are responsible, fair, and transparent.
Learn to use AI like a Pro
Get the latest AI workflows to boost your productivity and business performance, delivered weekly by expert consultants. Enjoy step-by-step guides, weekly Q&A sessions, and full access to our AI workflow archive.
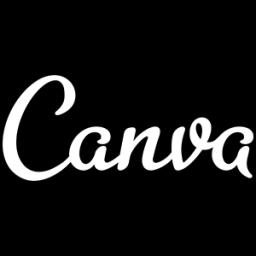
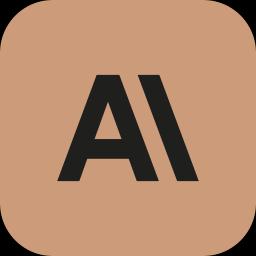
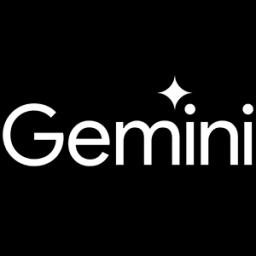
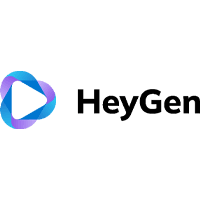
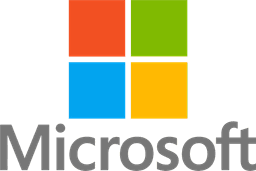
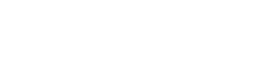
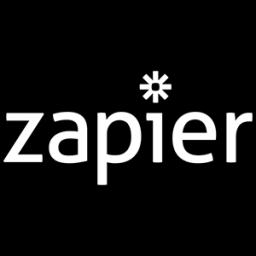
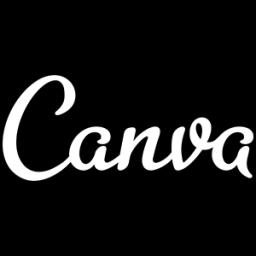
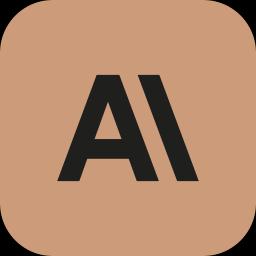
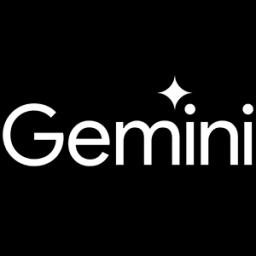
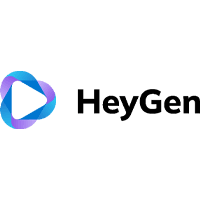
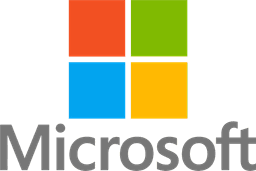
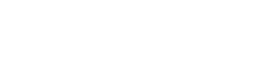
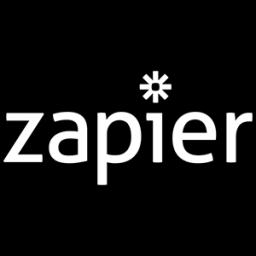
One major implication of AI regulation is its potential impact on the pace of innovation. While regulations are crucial for safeguarding against misuse and ensuring ethical compliance, they can also act as a double-edged sword—potentially slowing down AI development by introducing additional layers of compliance. Companies such as OpenAI must navigate these regulatory landscapes carefully to maintain competitive advantages while adhering to new rules. Regulations like the EU's AI Act put pressure on companies to develop technologies that align with ethical practices, which can alter their strategic priorities and investment in research and development.
Ethical standards in AI address the need for transparency, fairness, and accountability. For instance, there is growing public scrutiny regarding how data is used by AI systems, especially in light of stringent data privacy laws like the GDPR in Europe. Companies are now more than ever required to demonstrate that their AI technologies do not breach privacy standards and that they are capable of explaining how their algorithms arrive at decisions. The incorporation of ethical guidelines compels AI developers to consider the broader implications their technologies may have on society, addressing concerns about job displacement, algorithmic bias, and overall societal impact.
Another vital implication of AI regulation and ethical standards is the fostering of public trust. With growing interest and concern over AI's role in various sectors—from healthcare to finance—stakeholders are increasingly demanding transparency from AI developers. This demand for openness is not only from users but also from governmental entities looking to ensure that AI technologies are not misused. Compliance with ethical standards can improve public perception and acceptance of AI technologies, encouraging more widespread adoption and integration into everyday life.
The interplay between AI regulation, ethical standards, and technological advancement also fuels ongoing debates about the balance between innovation and control. As highlighted by recent global discussions, such as those surrounding the EU's AI Act, there is an evident push for regulations that keep pace with rapid technological advancements while ensuring ethical integrity. This dynamic environment presents both challenges and opportunities for AI companies aiming to innovate responsibly and sustainably.
The Impact of Data Privacy Laws on AI Innovation
Artificial Intelligence (AI) has become a transformative force in modern technology, with potential to revolutionize numerous sectors. However, the rise of data privacy laws globally presents significant challenges for AI innovation, affecting how companies design, deploy, and improve AI systems. These regulations aim to protect individuals' personal data, ensuring that AI technologies are developed with a focus on user consent, transparency, and security. As a result, data-driven companies must navigate a complex regulatory environment, balancing innovation with compliance.
OpenAI, a leading player in AI development, is already feeling the impact of these laws as it experiences a slowdown in its AI model advancements due to a shrinking pool of available training data. The stringent data protection regulations, such as the General Data Protection Regulation (GDPR) in Europe, impose restrictions on data collection and processing. This has led AI companies to rethink their data strategies, often resulting in the adoption of synthetic data to supplement real-world datasets, a necessity highlighted in OpenAI's development of its upcoming Orion model.
Learn to use AI like a Pro
Get the latest AI workflows to boost your productivity and business performance, delivered weekly by expert consultants. Enjoy step-by-step guides, weekly Q&A sessions, and full access to our AI workflow archive.
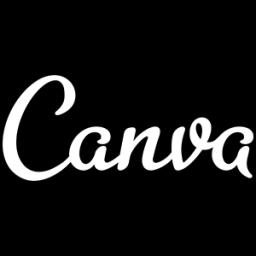
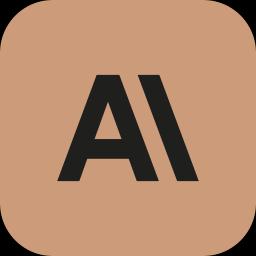
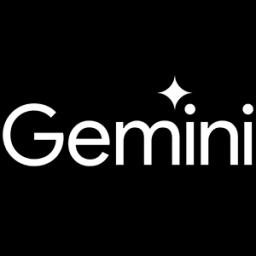
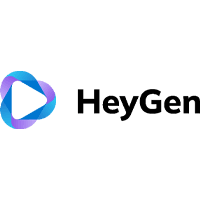
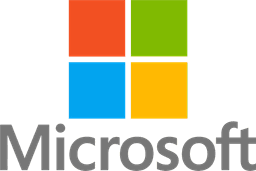
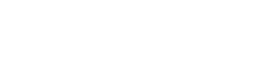
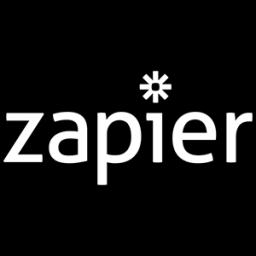
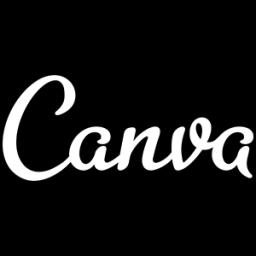
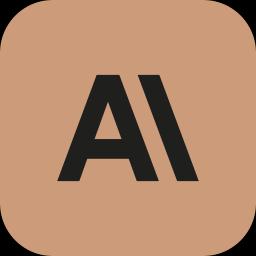
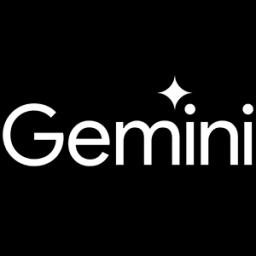
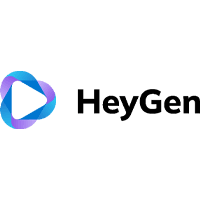
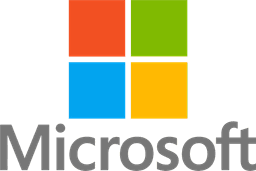
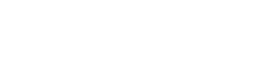
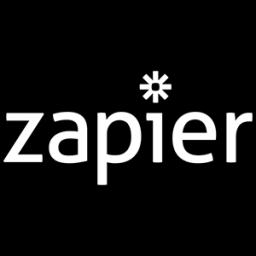
The slowdown in data access forces companies like OpenAI to innovate with alternative approaches, such as post-training enhancements and increased reliance on computing power during inference stages. However, these approaches not only require substantial financial and energy investments but also raise questions about long-term sustainability and the economic feasibility of scaling AI operations under restrictive data conditions.
Despite the challenges, data privacy laws also foster a new era of ethical AI practices, encouraging companies to be more transparent and responsible. The rising demand for AI systems that respect user privacy and mitigate biases aligns with societal calls for ethical standards and practices in technology development. As privacy concerns become more prevalent, AI firms are pushed towards innovation paths that integrate ethical considerations into their models, potentially leading to more trustworthy and user-focused AI solutions.
The regulatory landscape is rapidly evolving, influenced by initiatives such as the EU's AI Act, which potentially sets a precedent for global AI governance. As regions implement varying degrees of regulation on AI, companies need to navigate these complexities, impacting international collaborations and market dynamics. The influence of regulations on AI innovation is profound, shaping not only technological advancements but also strategic business decisions and global competition in AI markets.
Quantum Computing's Potential Role in AI Development
Quantum computing stands at the precipice of transforming various fields, including artificial intelligence (AI). Its unparalleled ability to process massive datasets and perform computations at speeds unattainable by classical computers positions it as a potential game-changer in AI development. As researchers and tech companies strive to push the boundaries of AI, quantum computing offers new avenues for overcoming existing challenges, such as the current slowdown in improvement rates at organizations like OpenAI.
The collaborative efforts between quantum computing and AI development can introduce a paradigm shift in how AI systems are trained and deployed. With access to quantum computing's immense computational capacity, AI can potentially leapfrog current limitations imposed by classical hardware. This shift could enable developers to build and refine models with greater complexity and accuracy, potentially transforming industries dependent on AI technologies.
As the AI landscape evolves, the integration of quantum computing may address several key issues raised by experts in AI development. By leveraging quantum power, the reliance on vast training datasets could diminish, effectively sidestepping constraints caused by data scarcity. This advancement can open up new possibilities in creating AI models that are not only powerful but also efficient, reducing the time and financial costs traditionally associated with model training.
Learn to use AI like a Pro
Get the latest AI workflows to boost your productivity and business performance, delivered weekly by expert consultants. Enjoy step-by-step guides, weekly Q&A sessions, and full access to our AI workflow archive.
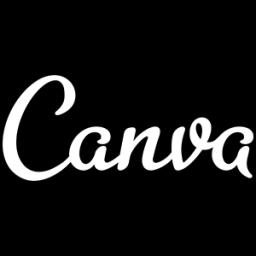
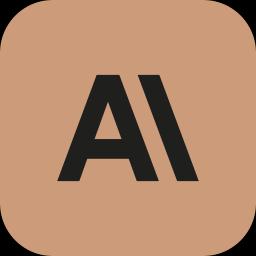
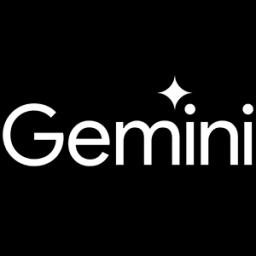
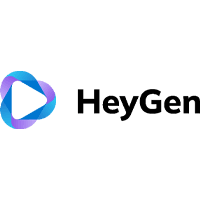
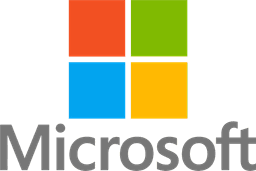
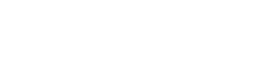
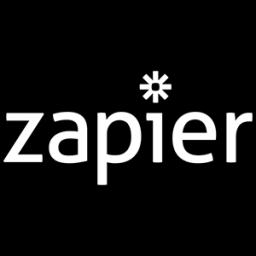
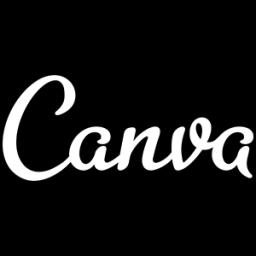
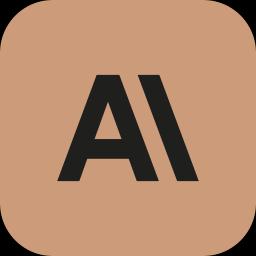
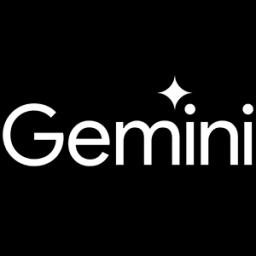
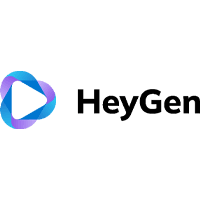
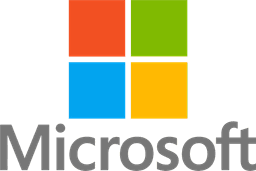
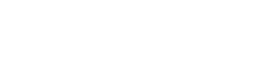
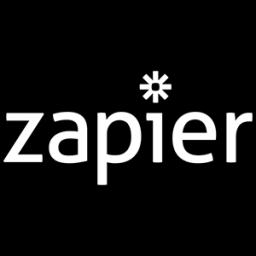
Public and private sectors are increasingly interested in exploring quantum computing's contributions to AI, spurred by the competitive atmosphere in tech innovation. Not only can quantum algorithms revolutionize AI model training, but they also hold the potential to significantly enhance inference processes, impacting the speed and accuracy of AI decisions in real-time applications. This element is crucial in areas such as autonomous systems, financial modeling, and large-scale data analytics, where decision speed and accuracy are paramount.
Ultimately, the interplay between quantum computing and AI development necessitates a reevaluation of existing infrastructure and competencies in tech organizations. Firms must prepare to harness the synergies of these technologies by investing in quantum research and development. As quantum computing matures, it could unravel a new era of AI innovation, steering technology toward uncharted territories of efficiency and capability, shaping the future of artificial intelligence and its myriad applications globally.
Collaborative Efforts in AI Research and Innovation
In recent years, collaborative efforts in AI research and innovation have become increasingly vital in addressing the challenges facing the field. As the rapid progress in AI begins to slow, companies like OpenAI are finding partnerships with academic institutions and other tech companies more critical. These alliances aim to explore innovative methodologies and data acquisition techniques that can help overcome obstacles such as data scarcity and the diminishing rate of improvement in AI models. By pooling resources and expertise, collaborative initiatives promise to drive the next wave of breakthroughs in artificial intelligence.
One significant area of collaboration is in the development of synthetic data and enhanced post-training processes. Such methods are rising in importance as traditional data sources reach their limits. Synthetic data offers a way to train AI models when real-world data becomes constrained, helping maintain the momentum of AI advancements. By working with academic researchers, AI companies can refine these techniques, ensuring they meet the rigorous standards required for robust and reliable AI systems.
Furthermore, the cross-pollination of ideas between academia and industry leads to improved machine learning algorithms and computational approaches. The recent advancements in quantum computing underscore this potential, as they could provide new methods for training and data processing. These partnerships are also pivotal in navigating the complex landscape of AI ethics and regulation, providing a platform to shape policies that prioritize safe and responsible AI development.
The role of collaborative efforts extends beyond technological advancements; it also encompasses forging pathways for ethical AI practices. With increasing public scrutiny, there is a pressing need for transparency in AI deployment. By engaging in open dialogue with stakeholders, including public forums, these collaborative efforts can help address societal concerns such as data privacy and bias. This approach not only enhances trust in AI technologies but also aligns with the growing call for regulation that ensures equitable and ethical AI implementation globally.
Learn to use AI like a Pro
Get the latest AI workflows to boost your productivity and business performance, delivered weekly by expert consultants. Enjoy step-by-step guides, weekly Q&A sessions, and full access to our AI workflow archive.
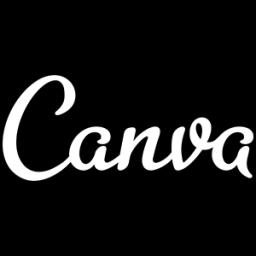
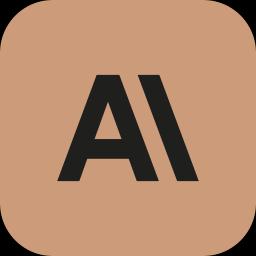
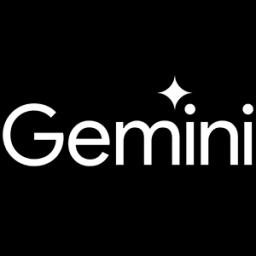
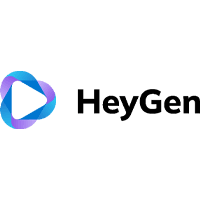
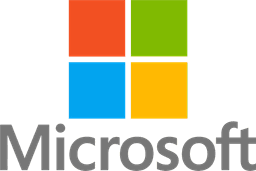
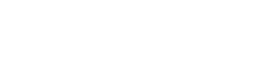
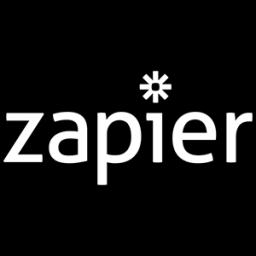
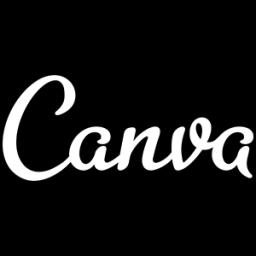
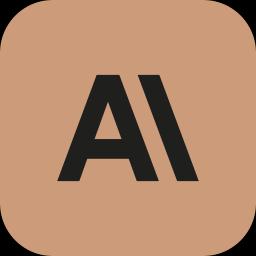
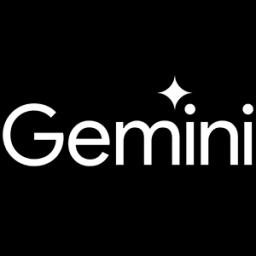
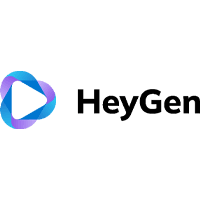
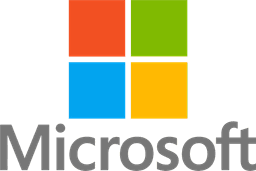
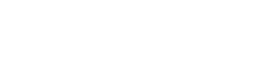
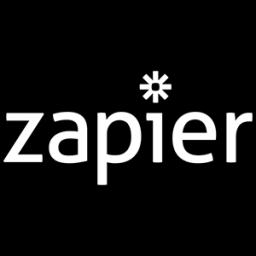
As AI continues to integrate into various sectors, the significance of collaborative research and innovation will only grow. By uniting diverse skills and perspectives, these partnerships have the potential to create solutions that are both technologically advanced and socially responsible, ultimately fostering an AI landscape that is inclusive and beneficial for all.
Public Scrutiny and Its Influence on AI Transparency
Public scrutiny plays a significant role in influencing AI transparency, as organizations like OpenAI navigate the complex landscape of developing and deploying artificial intelligence technologies. The increasing demand for accountability and ethical practices in AI usage is largely driven by the public's concern over how these technologies impact privacy, security, and bias. OpenAI's recent developments, particularly with the introduction of the Orion model, have been closely watched by both experts and the general populace, underscoring the importance of maintaining transparent communication about AI's capabilities and limitations.
The influence of public scrutiny is evident in OpenAI's strategic adjustments, which include adopting new methodologies like training with synthetic data and enhancing post-training processes. These strategies are not only technical responses to the slowdown in AI advancements but also a reflection of the organization's commitment to addressing public concerns. By openly discussing these strategies and involving various stakeholders in the conversation about ethical AI practices, OpenAI aims to foster a sense of trust and accountability in its operations.
Transparent AI practices are increasingly seen as necessary components for gaining public trust and ensuring sustainable progress in AI development. As OpenAI takes proactive measures to mitigate the challenges posed by limited data availability and slowing improvements, the scrutiny from public and regulatory bodies serves as a catalyst for further innovation and adherence to ethical standards. The dialogue between AI developers and the public is crucial in shaping the future of AI, emphasizing the need for ongoing engagement and collaboration to meet societal expectations and regulatory demands.
Expert Opinions on OpenAI's Strategic Shift
OpenAI, a renowned leader in Artificial Intelligence research and deployment, is undergoing a significant shift in its strategic approach. In the wake of the AI advancement slowdown, the company is exploring innovative methods to sustain its competitive edge and progression with the development of its new model, Orion. The slowdown has primarily been attributed to a limited availability of new training data, which is essential for improving AI models. To tackle this, OpenAI has formed a dedicated 'foundations team' focused on leveraging synthetic data and optimizing post-training processes. These strategies are critical as they aim to overcome the challenges posed by data limitations and ensure continuous improvement in AI capabilities.
The Orion model, while showing promise in certain areas such as language tasks, is not exhibiting the significant leap that characterized the transition from previous AI models like GPT-3 to GPT-4, especially in coding tasks. This observation has raised questions about the cost-effectiveness of pursuing advancements within the current framework of AI architecture. OpenAI’s strategic shift towards using synthetic data and post-training process enhancement reflects an adaptation to these constraints. Experts and employees within OpenAI have noted these challenges, emphasizing a need for innovative solutions to maintain progression in AI capabilities.
Learn to use AI like a Pro
Get the latest AI workflows to boost your productivity and business performance, delivered weekly by expert consultants. Enjoy step-by-step guides, weekly Q&A sessions, and full access to our AI workflow archive.
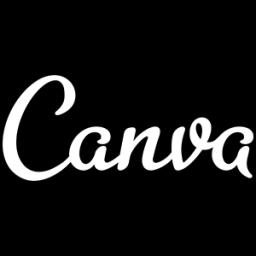
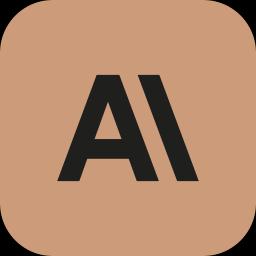
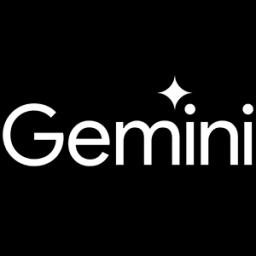
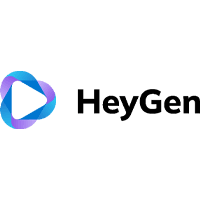
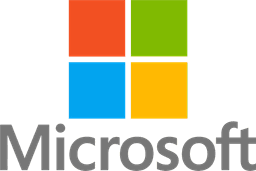
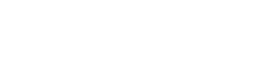
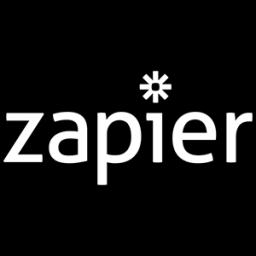
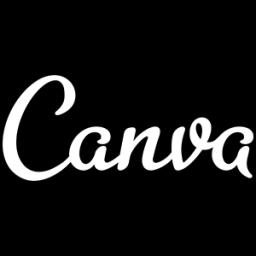
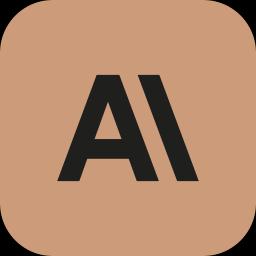
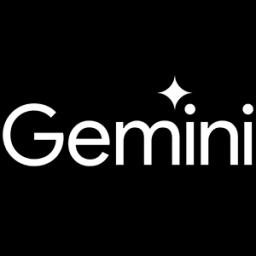
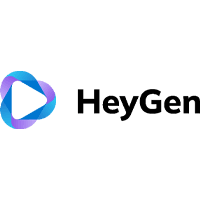
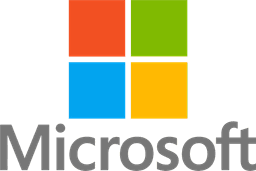
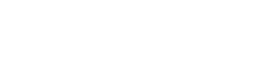
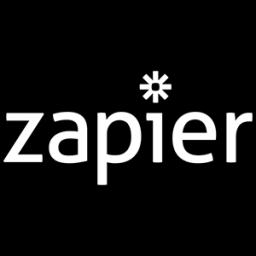
OpenAI's move away from traditional training methods, has sparked a debate among experts about the potential and efficiency of their new strategies. Noam Brown, an AI developer at OpenAI, suggests that focusing on inference over training might open new scaling dimensions. However, this shift involves substantial financial and energy costs, adding complexity to an already challenging scenario. OpenAI’s adaptation also considers environmental sustainability, as energy-intensive methods are scrutinized for their long-term viability. Despite these challenges, there remains cautious optimism for potential breakthroughs, largely hinging on the success and scalability of synthetic data usage.
Public reaction to OpenAI’s shift and the launch of Orion has been mixed. While some factions view this as a necessary adjustment given the current slowdown, others express skepticism over the slower progression in coding improvements, questioning if this shift represents a 'trough of disillusionment' for Large Language Models. The concerns are compounded by the model’s limited initial release to partners like Microsoft, sparking debates over priorities and access inequality in tech development. Nonetheless, forums and social media platforms are rife with discussions about its ethical implications, emphasizing the dual sentiments of anticipation and concern.
The strategic developments at OpenAI have significant future implications across economic, social, and political spectrums. Economically, as OpenAI and other tech companies invest in novel solutions like enhanced data processing and synthetic data usage, operational costs might rise, potentially influencing market strategies and allocation of resources. On the social front, the focus on ethical AI is pressing companies toward more transparent and responsible practices, responding to growing public awareness and concern over privacy and biases. Politically, OpenAI’s strategies and the regional release strategies of Orion could impact global policy discussions on AI governance and equitable technology distribution, intensifying the dialogue on international cooperation and competition in technological advancement.
Public Reactions to OpenAI's Orion Model
OpenAI's recent endeavors in addressing the deceleration in AI growth have sparked a spectrum of public reactions. The introduction of the "Orion" model, though marking an advancement, has been met with mixed sentiments due to the less pronounced leap forward compared to former versions, especially in areas like coding. During lively online discussions on platforms like Reddit and X (formerly Twitter), the public voiced concerns about a potential stagnation in AI development, correlating this slowdown with apprehensions over market valuations and future breakthroughs in achieving Artificial General Intelligence (AGI). Such skepticism suggests a "trough of disillusionment" phase for large language models (LLMs), highlighting contrasting expectations within the community.
However, despite the mixed reactions, there exists a faction that views even minimal improvements in the Orion model as a positive stride towards continual AI refinement. This perspective appreciates OpenAI's deliberate focus on post-training processes, which aims to optimally utilize existing advancements, even in the face of data supply challenges. Meanwhile, OpenAI CEO Sam Altman's enigmatic hints about future updates have fueled excitement among supporters, who anticipate developments yielding substantial benefits over time.
Simultaneously, criticisms have emerged over Orion's prioritized limited launch to strategic partners like Microsoft, which many perceive as reinforcing corporate alliances at the expense of open accessibility, potentially exacerbating disparities between larger and smaller tech entities. Public forums, such as OpenAI's Developer Forum, actively explore Orion's ethical and societal ramifications — addressing biases, data privacy concerns, and its implications on employment, while fostering a guarded optimism about its prospective impacts.
Learn to use AI like a Pro
Get the latest AI workflows to boost your productivity and business performance, delivered weekly by expert consultants. Enjoy step-by-step guides, weekly Q&A sessions, and full access to our AI workflow archive.
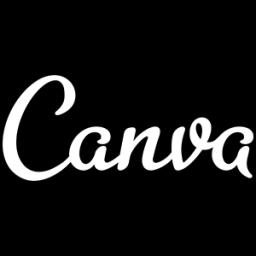
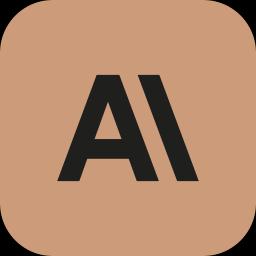
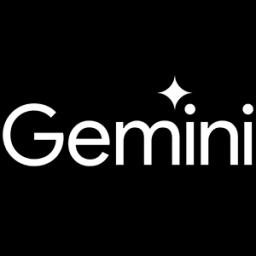
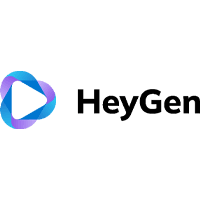
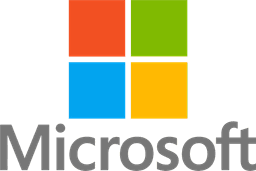
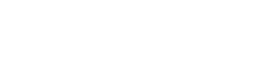
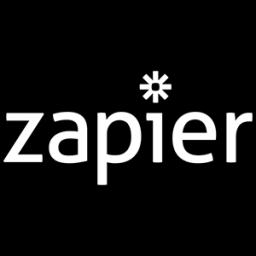
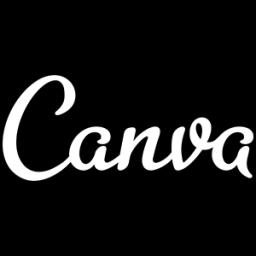
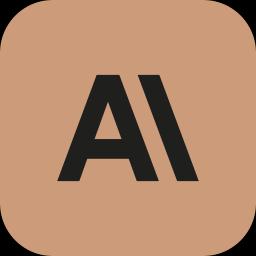
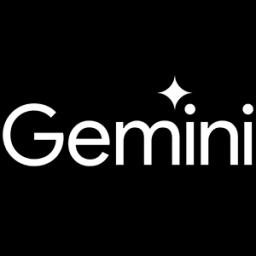
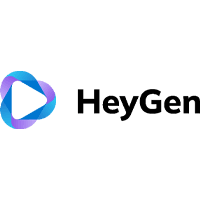
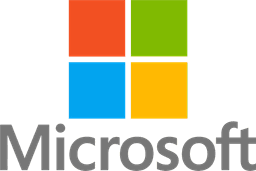
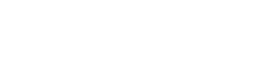
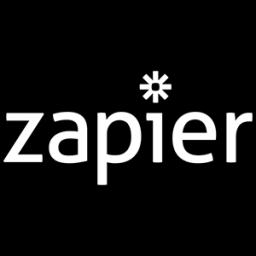
Future Economic Implications of OpenAI's Strategies
OpenAI's latest strategic moves in response to a slowdown in AI improvement have profound potential economic implications, particularly as the company grapples with diminishing training data availability. The decision to leverage synthetic data and focus on post-training enhancements signals a shift that could significantly impact cost structures within the tech industry. This move may necessitate increased investment in new data acquisition and model training methods, resulting in elevated operational expenses for AI companies aiming to maintain a competitive edge. As these businesses adapt to emerging computational paradigms, such as quantum computing, we may see transformative changes in how resources are allocated, potentially reshaping the economic landscape of the technology sector.
Moreover, as OpenAI proceeds with these new strategies, there is an inherent economic risk associated with scaling existing AI architectures, highlighted by the Orion model's incremental rather than exponential improvements in certain areas like coding. This raises questions about the cost-effectiveness of such models and whether continued investment yields sufficient returns. In exploring these methods, OpenAI might set a precedent for the broader industry, leading to shifts in investment priorities and potentially driving innovation in alternative approaches to AI development that are both economically viable and sustainable in the long run.
Social and Ethical Considerations in AI Deployment
Artificial intelligence (AI) deployment encompasses a variety of social and ethical considerations that must be addressed for responsible use. As AI systems become more integrated into everyday life, there is an increasing demand from the public and stakeholders for transparency and ethical governance. These considerations stretch across data privacy, algorithmic bias, accountability, and the potential displacement of jobs by AI technologies. Such ethical issues are not merely academic but have real-world implications that affect trust and acceptance of AI innovations.
The emergence of new AI models, like OpenAI's Orion, accentuates the need for discussions surrounding their ethical implications. Given the model's heightened capabilities, potential biases, limitations in transparency, and the proper use of AI-generated content are critical discussion points. Public scrutiny, which is growing, highlights societal expectations for AI technologies to operate ethically and equitably. As systems grow more powerful, ensuring that they are developed and used responsibly becomes crucial to maintaining societal trust.
The legislation, such as the EU's AI Act, is a key initiative aiming to establish a comprehensive framework that ensures AI systems are developed safely and responsibly. By developing rigorous standards for AI deployment, these regulations seek to mitigate risks and address ethical concerns effectively. This legislative environment encourages companies to adhere to ethical AI practices, promoting innovation while safeguarding societal interests. Such laws not only set benchmarks for ethical AI across regions but also influence international standards.
In response to ethical concerns, AI companies must engage in transparent practices, prioritizing ethical considerations at every stage of AI development. OpenAI and similar organizations are called to ensure that AI systems do not perpetuate biases or infringe upon individual privacy rights. Collaborative initiatives between tech companies and academic institutions further strive towards developing frameworks that uphold ethical standards while fostering technological advancement. By investing in such collaborative efforts, the AI landscape can evolve toward responsible innovation.
Learn to use AI like a Pro
Get the latest AI workflows to boost your productivity and business performance, delivered weekly by expert consultants. Enjoy step-by-step guides, weekly Q&A sessions, and full access to our AI workflow archive.
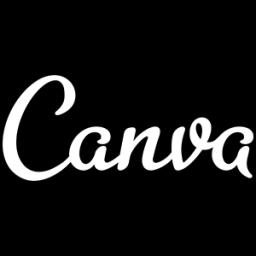
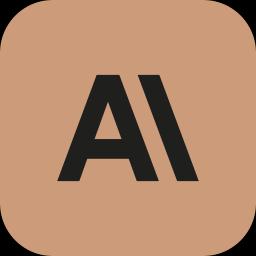
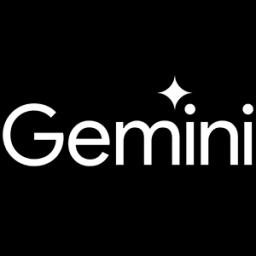
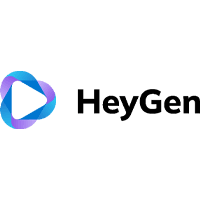
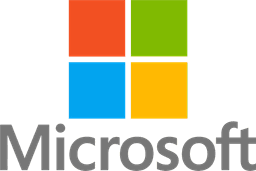
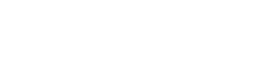
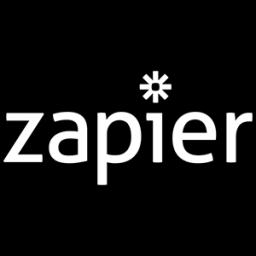
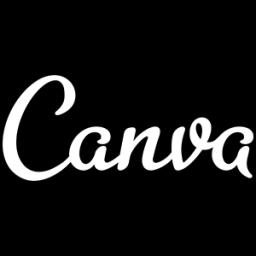
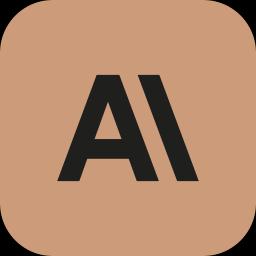
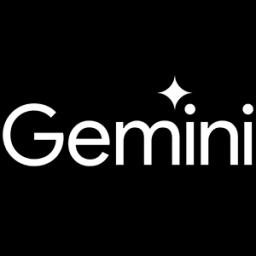
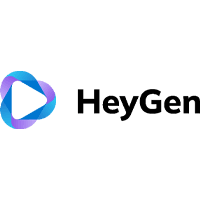
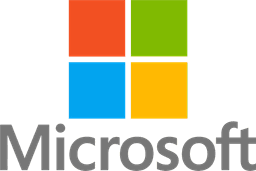
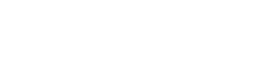
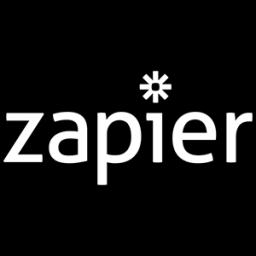
Political and Regulatory Impacts on AI Development
Artificial Intelligence (AI) development is increasingly influenced by political and regulatory landscapes worldwide. This phenomenon is becoming even more pronounced as AI capabilities such as those offered by OpenAI's technological advancements face unprecedented scrutiny and regulation. Governments and regulatory bodies are actively engaging in formulating stringent frameworks to ensure AI systems are developed and deployed safely and ethically. Such regulations, including the European Union's AI Act, emphasize mitigating risks associated with AI, ranging from data privacy concerns to potential biases, thus directly impacting AI research and development methodologies.
The evolving regulatory environment poses challenges and opportunities for AI companies like OpenAI. They must navigate complex compliance landscapes, which may increase operational costs and require innovative strategies to remain competitive. On the regulatory front, companies are compelled to engage in transparent AI practices, enhancing their systems' accountability and reliability. For OpenAI, these regulatory requirements align with their latest efforts to address improvement slowdowns through strategic innovations such as utilizing synthetic data and optimizing post-training processes.
Global discussions regarding AI regulation also reflect growing public scrutiny and ethical considerations surrounding AI technologies. This pressure influences AI developers to prioritize user privacy, data security, and overall ethical standards. OpenAI's strategic shift towards these focuses can be seen as a response to these external pressures, acknowledging the need for ethical AI solutions in an increasingly regulated market. This alignment not only ensures compliance but also potentially fortifies public trust in AI technologies.
Furthermore, the political ramifications of AI regulation extend beyond national boundaries, affecting international collaboration and competition. With initiatives like the EU's AI Act setting precedent, global tech firms must consider a wider array of compliance factors. Such regulations may hinder the pace of AI innovation or alternatively push companies to adopt new technologies or collaborative methods to surmount regulatory barriers. For OpenAI, the interplay between regulatory standards and technological advancement serves as a crucible for refining and validating their AI models.
In conclusion, while political and regulatory forces shape the direction of AI development, they also foster a climate of innovation, guiding companies like OpenAI to pursue advanced, ethical, and transparent AI solutions. This evolving landscape underscores the importance of balancing cutting-edge technological progress with societal needs and ethical obligations, ensuring that AI serves as a beneficial tool within a well-regulated framework.